733 results
Characterizing Veteran suicide decedents that were not classified as high-suicide-risk
-
- Journal:
- Psychological Medicine , First View
- Published online by Cambridge University Press:
- 16 September 2024, pp. 1-10
-
- Article
- Export citation
A combined active control method of restricted nonlinear model and machine learning technology for drag reduction in turbulent channel flow
-
- Journal:
- Journal of Fluid Mechanics / Volume 993 / 25 August 2024
- Published online by Cambridge University Press:
- 13 September 2024, A4
-
- Article
- Export citation
Data-driven transient lift attenuation for extreme vortex gust–airfoil interactions
-
- Journal:
- Journal of Fluid Mechanics / Volume 992 / 10 August 2024
- Published online by Cambridge University Press:
- 10 September 2024, A17
-
- Article
-
- You have access
- Open access
- HTML
- Export citation
Detecting suicide risk among U.S. servicemembers and veterans: a deep learning approach using social media data
-
- Journal:
- Psychological Medicine , First View
- Published online by Cambridge University Press:
- 09 September 2024, pp. 1-10
-
- Article
- Export citation
Thought flow nets: From single predictions to trains of model thought
-
- Journal:
- Natural Language Processing ,
- Published online by Cambridge University Press:
- 06 September 2024, pp. 1-32
-
- Article
-
- You have access
- Open access
- HTML
- Export citation
The promise of machine learning in violent conflict forecasting
-
- Journal:
- Data & Policy / Volume 6 / 2024
- Published online by Cambridge University Press:
- 30 August 2024, e35
-
- Article
-
- You have access
- Open access
- HTML
- Export citation
Virtual laboratories: transforming research with AI
-
- Journal:
- Data-Centric Engineering / Volume 5 / 2024
- Published online by Cambridge University Press:
- 27 August 2024, e19
-
- Article
-
- You have access
- Open access
- HTML
- Export citation
Archival Aerial Photographs of Africa: Present Potential and Imagining a Machine-Learning Future
-
- Journal:
- History in Africa , First View
- Published online by Cambridge University Press:
- 23 August 2024, pp. 1-34
-
- Article
-
- You have access
- Open access
- HTML
- Export citation
Jet mixing enhancement with Bayesian optimization, deep learning and persistent data topology
-
- Journal:
- Journal of Fluid Mechanics / Volume 991 / 25 July 2024
- Published online by Cambridge University Press:
- 20 August 2024, A5
-
- Article
- Export citation
Three-dimensional generative adversarial networks for turbulent flow estimation from wall measurements
-
- Journal:
- Journal of Fluid Mechanics / Volume 991 / 25 July 2024
- Published online by Cambridge University Press:
- 20 August 2024, A1
-
- Article
-
- You have access
- Open access
- HTML
- Export citation
Deep reinforcement learning finds a new strategy for vortex-induced vibration control
-
- Journal:
- Journal of Fluid Mechanics / Volume 990 / 10 July 2024
- Published online by Cambridge University Press:
- 12 August 2024, A7
-
- Article
-
- You have access
- Open access
- HTML
- Export citation
Chapter 25 - Modelling in DOHaD
- from Section 6 - Future Directions
-
-
- Book:
- The Handbook of DOHaD and Society
- Published online:
- 20 June 2024
- Print publication:
- 27 June 2024, pp 279-289
-
- Chapter
-
- You have access
- Open access
- HTML
- Export citation
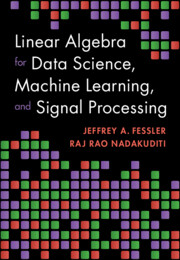
Linear Algebra for Data Science, Machine Learning, and Signal Processing
- Coming soon
-
- Expected online publication date:
- June 2024
- Print publication:
- 16 May 2024
-
- Textbook
- Export citation
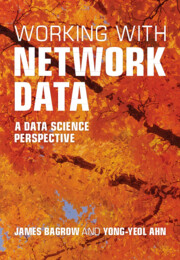
Working with Network Data
- A Data Science Perspective
-
- Published online:
- 06 June 2024
- Print publication:
- 13 June 2024
CLIMATE-RELATED DISCUSSIONS ON SOCIAL MEDIA: CRITICAL LESSONS FOR POLICYMAKERS
-
- Journal:
- National Institute Economic Review ,
- Published online by Cambridge University Press:
- 04 June 2024, pp. 1-8
-
- Article
-
- You have access
- Open access
- HTML
- Export citation
A bidirectional LSTM-based morphological analyzer for Gujarati
-
- Journal:
- Natural Language Processing ,
- Published online by Cambridge University Press:
- 31 May 2024, pp. 1-17
-
- Article
-
- You have access
- Open access
- HTML
- Export citation
2 - Artificial Intelligence and Economics
-
- Book:
- Artificial Intelligence
- Published online:
- 23 May 2024
- Print publication:
- 30 May 2024, pp 22-59
-
- Chapter
- Export citation
5 - Artificial Intelligence, Growth, and Inequality
-
- Book:
- Artificial Intelligence
- Published online:
- 23 May 2024
- Print publication:
- 30 May 2024, pp 107-172
-
- Chapter
- Export citation
The natural course of binge-eating disorder: findings from a prospective, community-based study of adults
-
- Journal:
- Psychological Medicine , First View
- Published online by Cambridge University Press:
- 28 May 2024, pp. 1-11
-
- Article
- Export citation
Dynamic feature-based deep reinforcement learning for flow control of circular cylinder with sparse surface pressure sensing
-
- Journal:
- Journal of Fluid Mechanics / Volume 988 / 10 June 2024
- Published online by Cambridge University Press:
- 28 May 2024, A4
-
- Article
- Export citation