769 results
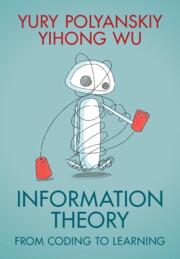
Information Theory
- From Coding to Learning
- Coming soon
-
- Expected online publication date:
- December 2024
- Print publication:
- 02 January 2025
-
- Textbook
- Export citation
12 - Algorithms
-
- Book:
- Ethics in Econometrics
- Published online:
- 14 November 2024
- Print publication:
- 28 November 2024, pp 269-280
-
- Chapter
- Export citation
ActuaryGPT: applications of large language models to insurance and actuarial work
-
- Journal:
- British Actuarial Journal / Volume 29 / 2024
- Published online by Cambridge University Press:
- 21 November 2024, e15
-
- Article
-
- You have access
- Open access
- HTML
- Export citation
Deep learning–based detection and quantification of weed seed mixtures
-
- Journal:
- Weed Science , FirstView
- Published online by Cambridge University Press:
- 21 November 2024, pp. 1-9
-
- Article
-
- You have access
- Open access
- HTML
- Export citation
A Machine Learning-Based Approach for Solving Recurrence Relations and Its use in Cost Analysis of Logic Programs
-
- Journal:
- Theory and Practice of Logic Programming , First View
- Published online by Cambridge University Press:
- 21 November 2024, pp. 1-45
-
- Article
-
- You have access
- Open access
- HTML
- Export citation
Predicting involuntary admission following inpatient psychiatric treatment using machine learning trained on electronic health record data
-
- Journal:
- Psychological Medicine , First View
- Published online by Cambridge University Press:
- 18 November 2024, pp. 1-14
-
- Article
-
- You have access
- Open access
- HTML
- Export citation
Filtered partial differential equations: a robust surrogate constraint in physics-informed deep learning framework
-
- Journal:
- Journal of Fluid Mechanics / Volume 999 / 25 November 2024
- Published online by Cambridge University Press:
- 15 November 2024, A40
-
- Article
-
- You have access
- HTML
- Export citation
Willingness to pay of Portuguese sparkling wine consumers: Econometric and machine learning approaches
-
- Journal:
- Journal of Wine Economics , First View
- Published online by Cambridge University Press:
- 15 November 2024, pp. 1-21
-
- Article
-
- You have access
- Open access
- HTML
- Export citation
Predictors for improvement in personality functioning during outpatient psychotherapy: A machine learning approach within a psychodynamic psychotherapy sample
-
- Journal:
- European Psychiatry / Volume 67 / Issue 1 / 2024
- Published online by Cambridge University Press:
- 15 November 2024, e79
-
- Article
-
- You have access
- Open access
- HTML
- Export citation
Weed Communities of Snap Bean Fields in the United States
-
- Journal:
- Weed Science / Accepted manuscript
- Published online by Cambridge University Press:
- 15 November 2024, pp. 1-30
-
- Article
-
- You have access
- Export citation
Generating Global and Local Explanations for Tree-Ensemble Learning Methods by Answer Set Programming
-
- Journal:
- Theory and Practice of Logic Programming , First View
- Published online by Cambridge University Press:
- 13 November 2024, pp. 1-38
-
- Article
-
- You have access
- Open access
- HTML
- Export citation
Neural networks empowered: a machine learning-enabled, Gyro mmID for enhanced virtual reality and motion tracking applications
-
- Journal:
- International Journal of Microwave and Wireless Technologies , First View
- Published online by Cambridge University Press:
- 13 November 2024, pp. 1-8
-
- Article
-
- You have access
- Open access
- HTML
- Export citation
Watch out for words: the wording of constitutions and constitutional compliance
-
- Journal:
- Journal of Institutional Economics / Volume 20 / 2024
- Published online by Cambridge University Press:
- 11 November 2024, e35
-
- Article
-
- You have access
- HTML
- Export citation
Use of generative artificial intelligence (AI) in psychiatry and mental health care: a systematic review
-
- Journal:
- Acta Neuropsychiatrica , First View
- Published online by Cambridge University Press:
- 11 November 2024, pp. 1-14
-
- Article
-
- You have access
- Open access
- HTML
- Export citation
Recent kernel methods for interacting particle systems: first numerical results
- Part of
-
- Journal:
- European Journal of Applied Mathematics , First View
- Published online by Cambridge University Press:
- 11 November 2024, pp. 1-26
-
- Article
-
- You have access
- Open access
- HTML
- Export citation
Cloudy with a chance of precision: satellite’s autoconversion rates forecasting powered by machine learning
-
- Journal:
- Environmental Data Science / Volume 3 / 2024
- Published online by Cambridge University Press:
- 11 November 2024, e23
-
- Article
-
- You have access
- Open access
- HTML
- Export citation
FloodGNN-GRU: a spatio-temporal graph neural network for flood prediction
-
- Journal:
- Environmental Data Science / Volume 3 / 2024
- Published online by Cambridge University Press:
- 08 November 2024, e21
-
- Article
-
- You have access
- Open access
- HTML
- Export citation
A machine learning assisted preliminary design methodology for bolted composite joints in large structures
-
- Journal:
- The Aeronautical Journal , First View
- Published online by Cambridge University Press:
- 07 November 2024, pp. 1-14
-
- Article
-
- You have access
- Open access
- HTML
- Export citation
Improved machine learning models with a similarity-based approach for remaining useful life prediction
-
- Journal:
- The Aeronautical Journal , First View
- Published online by Cambridge University Press:
- 04 November 2024, pp. 1-21
-
- Article
-
- You have access
- HTML
- Export citation
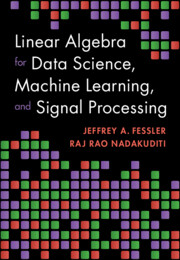
Linear Algebra for Data Science, Machine Learning, and Signal Processing
-
- Published online:
- 01 November 2024
- Print publication:
- 16 May 2024
-
- Textbook
- Export citation