101 results
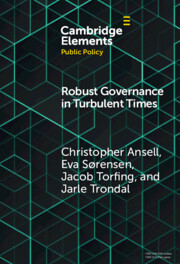
Robust Governance in Turbulent Times
-
- Published online:
- 26 April 2024
- Print publication:
- 16 May 2024
-
- Element
-
- You have access
- Open access
- HTML
- Export citation
Debunking the idea of biological optimisation: quantitative biology to the rescue
-
- Journal:
- Quantitative Plant Biology / Volume 5 / 2024
- Published online by Cambridge University Press:
- 03 April 2024, e3
-
- Article
-
- You have access
- Open access
- HTML
- Export citation
7 - Bounding Damage from Nonignorable Nonresponse
- from Part III - Fighting Nonignorable Nonresponse
-
- Book:
- Polling at a Crossroads
- Published online:
- 29 February 2024
- Print publication:
- 07 March 2024, pp 137-143
-
- Chapter
- Export citation
Establishing the validity and robustness of facial electromyography measures for political science
-
- Journal:
- Politics and the Life Sciences , First View
- Published online by Cambridge University Press:
- 15 January 2024, pp. 1-18
-
- Article
-
- You have access
- Open access
- HTML
- Export citation
Robustness, Scott continuity, and computability
-
- Journal:
- Mathematical Structures in Computer Science / Volume 33 / Issue 6 / June 2023
- Published online by Cambridge University Press:
- 04 August 2023, pp. 536-572
-
- Article
-
- You have access
- Open access
- HTML
- Export citation
A domain-theoretic framework for robustness analysis of neural networks
-
- Journal:
- Mathematical Structures in Computer Science / Volume 33 / Issue 2 / February 2023
- Published online by Cambridge University Press:
- 23 May 2023, pp. 68-105
-
- Article
-
- You have access
- HTML
- Export citation
The elastic origins of tail asymmetry
-
- Journal:
- Macroeconomic Dynamics / Volume 28 / Issue 3 / April 2024
- Published online by Cambridge University Press:
- 27 April 2023, pp. 591-611
-
- Article
-
- You have access
- HTML
- Export citation
Robustness of nonuniform mean-square exponential dichotomies
- Part of
-
- Journal:
- Proceedings of the Royal Society of Edinburgh. Section A: Mathematics / Volume 154 / Issue 2 / April 2024
- Published online by Cambridge University Press:
- 18 April 2023, pp. 525-567
- Print publication:
- April 2024
-
- Article
-
- You have access
- HTML
- Export citation
SENSITIVITY ANALYSIS: ADVANCING THE EFFECTIVENESS OF GLOBAL SENSITIVITY ANALYSIS
- Part of
-
- Journal:
- Bulletin of the Australian Mathematical Society / Volume 107 / Issue 2 / April 2023
- Published online by Cambridge University Press:
- 06 February 2023, pp. 351-352
- Print publication:
- April 2023
-
- Article
-
- You have access
- HTML
- Export citation
FUZZY APPROACHES AGAINST OUTLIERS AND APPLICATIONS IN WIND ENERGY
- Part of
-
- Journal:
- Bulletin of the Australian Mathematical Society / Volume 107 / Issue 2 / April 2023
- Published online by Cambridge University Press:
- 03 February 2023, pp. 346-348
- Print publication:
- April 2023
-
- Article
-
- You have access
- HTML
- Export citation
The hidden contestation of norms: Decent work in the International Labour Organization and the United Nations
-
- Journal:
- Global Constitutionalism / Volume 12 / Issue 2 / July 2023
- Published online by Cambridge University Press:
- 16 January 2023, pp. 246-268
-
- Article
-
- You have access
- Open access
- HTML
- Export citation
Note on Birnbaum and Wan (2020): True and error model analysis is robust with respect to certain violations of the MARTER model
-
- Journal:
- Judgment and Decision Making / Volume 15 / Issue 5 / September 2020
- Published online by Cambridge University Press:
- 01 January 2023, pp. 861-862
-
- Article
-
- You have access
- Open access
- HTML
- Export citation
1 - Introduction
-
-
- Book:
- Robustness and Fragility of Political Orders
- Published online:
- 25 November 2022
- Print publication:
- 24 November 2022, pp 1-24
-
- Chapter
- Export citation
2 - Robustness and Fragility of Political Orders
-
-
- Book:
- Robustness and Fragility of Political Orders
- Published online:
- 25 November 2022
- Print publication:
- 24 November 2022, pp 25-53
-
- Chapter
- Export citation
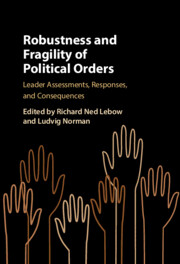
Robustness and Fragility of Political Orders
- Leader Assessments, Responses, and Consequences
-
- Published online:
- 25 November 2022
- Print publication:
- 24 November 2022
10 - Conclusions
-
-
- Book:
- Robustness and Fragility of Political Orders
- Published online:
- 25 November 2022
- Print publication:
- 24 November 2022, pp 270-312
-
- Chapter
- Export citation
On connectivity and robustness of random graphs with inhomogeneity
- Part of
-
- Journal:
- Journal of Applied Probability / Volume 60 / Issue 1 / March 2023
- Published online by Cambridge University Press:
- 05 September 2022, pp. 284-294
- Print publication:
- March 2023
-
- Article
- Export citation
Generating weighted and thresholded gene coexpression networks using signed distance correlation
-
- Journal:
- Network Science / Volume 10 / Issue 2 / June 2022
- Published online by Cambridge University Press:
- 16 June 2022, pp. 131-145
-
- Article
- Export citation
10 - Thinking Outside the Box in the Bourbonnais
-
-
- Book:
- Resilient and Sustainable Farming Systems in Europe
- Published online:
- 21 April 2022
- Print publication:
- 05 May 2022, pp 171-184
-
- Chapter
-
- You have access
- Open access
- HTML
- Export citation
Analysis and modelling of airport resilience, robustness, and vulnerability: impact of COVID-19 pandemic disease
-
- Journal:
- The Aeronautical Journal / Volume 126 / Issue 1305 / November 2022
- Published online by Cambridge University Press:
- 08 April 2022, pp. 1924-1953
-
- Article
-
- You have access
- Open access
- HTML
- Export citation