184 results
Interleukins in the pathogenesis of influenza and other acute respiratory viral infections
-
- Journal:
- Epidemiology & Infection / Volume 152 / 2024
- Published online by Cambridge University Press:
- 30 September 2024, e109
-
- Article
-
- You have access
- Open access
- HTML
- Export citation
Spatiotemporal dynamics of subarctic intertidal macrobenthos: going their own ways
-
- Journal:
- Journal of the Marine Biological Association of the United Kingdom / Volume 104 / 2024
- Published online by Cambridge University Press:
- 18 March 2024, e32
-
- Article
-
- You have access
- HTML
- Export citation
2 - The Offshore Environment
-
- Book:
- Offshore Wind Energy
- Published online:
- 07 March 2024
- Print publication:
- 14 March 2024, pp 11-76
-
- Chapter
- Export citation
E - Strategist Should Make a Situation’s Natural Dynamics Work for Her
-
- Book:
- Three Faces of Sun Tzu
- Published online:
- 07 March 2024
- Print publication:
- 15 February 2024, pp 298-356
-
- Chapter
- Export citation
E - Strategist Should Make a Situation’s Natural Dynamics Work for Her
-
- Book:
- Three Faces of Sun Tzu
- Published online:
- 07 March 2024
- Print publication:
- 15 February 2024, pp 298-356
-
- Chapter
- Export citation
Design and dynamic analysis of supporting mechanism for large scale space deployable membrane sunshield
-
- Journal:
- The Aeronautical Journal / Volume 128 / Issue 1327 / September 2024
- Published online by Cambridge University Press:
- 08 February 2024, pp. 1974-2002
-
- Article
-
- You have access
- HTML
- Export citation
Use of VEGA data to analyse balloon options for possible subsequent long endurance Venus cloud layer missions
-
- Journal:
- The Aeronautical Journal / Volume 128 / Issue 1324 / June 2024
- Published online by Cambridge University Press:
- 08 February 2024, pp. 1104-1118
-
- Article
-
- You have access
- HTML
- Export citation
4 - Particle Sizes, Shapes, and Trajectories
-
- Book:
- Fluid Dynamics of Particles, Drops, and Bubbles
- Published online:
- 28 July 2023
- Print publication:
- 17 August 2023, pp 130-191
-
- Chapter
- Export citation
7 - Combining Translation and Rotation
-
- Book:
- A Student's Guide to Rotational Motion
- Published online:
- 07 August 2023
- Print publication:
- 03 August 2023, pp 133-156
-
- Chapter
- Export citation
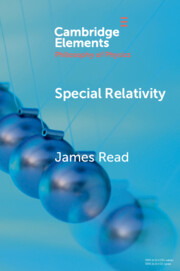
Special Relativity
-
- Published online:
- 26 June 2023
- Print publication:
- 20 July 2023
-
- Element
- Export citation
A reduced-order closed-loop hybrid dynamic model for design and development of lower limb prostheses
-
- Journal:
- Wearable Technologies / Volume 4 / 2023
- Published online by Cambridge University Press:
- 04 April 2023, e10
-
- Article
-
- You have access
- Open access
- HTML
- Export citation
Time-optimal path following for non-redundant serial manipulators using an adaptive path-discretization
-
- Article
-
- You have access
- Open access
- HTML
- Export citation
A network perspective on animal welfare
-
- Journal:
- Animal Welfare / Volume 30 / Issue 3 / August 2021
- Published online by Cambridge University Press:
- 01 January 2023, pp. 235-248
-
- Article
-
- You have access
- Export citation
Chapter 2 - Social Exclusion: Basic Concepts
- from Section 1 - Social Exclusion, Poverty, and Inequality
-
- Book:
- Social Inclusion and Mental Health
- Published online:
- 24 November 2022
- Print publication:
- 08 December 2022, pp 21-34
-
- Chapter
- Export citation
The obliquity of Mercury: Models and interpretation
-
- Journal:
- Proceedings of the International Astronomical Union / Volume 18 / Issue S382 / December 2022
- Published online by Cambridge University Press:
- 16 October 2024, pp. 1-11
- Print publication:
- December 2022
-
- Article
-
- You have access
- Export citation
On the predictability horizon in Impact Monitoring of Near Earth Objects
-
- Journal:
- Proceedings of the International Astronomical Union / Volume 18 / Issue S382 / December 2022
- Published online by Cambridge University Press:
- 16 October 2024, pp. 185-190
- Print publication:
- December 2022
-
- Article
-
- You have access
- Open access
- Export citation
Comparison of Mars rotation angle models
-
- Journal:
- Proceedings of the International Astronomical Union / Volume 18 / Issue S382 / December 2022
- Published online by Cambridge University Press:
- 16 October 2024, pp. 198-204
- Print publication:
- December 2022
-
- Article
-
- You have access
- Export citation