45 results
2 - Philosophy of AI
- from Part I - AI, Ethics and Philosophy
-
-
- Book:
- The Cambridge Handbook of the Law, Ethics and Policy of Artificial Intelligence
- Published online:
- 06 February 2025
- Print publication:
- 13 February 2025, pp 40-58
-
- Chapter
-
- You have access
- Open access
- HTML
- Export citation
ON GALOIS GROUPS OF POWER COMPOSITIONAL NONIC POLYNOMIALS
- Part of
-
- Journal:
- Bulletin of the Australian Mathematical Society , First View
- Published online by Cambridge University Press:
- 10 February 2025, pp. 1-15
-
- Article
- Export citation
Chapter 5 - The Archive
-
-
- Book:
- The Cambridge Companion to Literature in a Digital Age
- Published online:
- 29 November 2024
- Print publication:
- 14 November 2024, pp 89-106
-
- Chapter
- Export citation
A - Strategist Should Be Calculating
-
- Book:
- Three Faces of Sun Tzu
- Published online:
- 07 March 2024
- Print publication:
- 15 February 2024, pp 54-109
-
- Chapter
- Export citation
A - Strategist Should Be Calculating
-
- Book:
- Three Faces of Sun Tzu
- Published online:
- 07 March 2024
- Print publication:
- 15 February 2024, pp 54-109
-
- Chapter
- Export citation
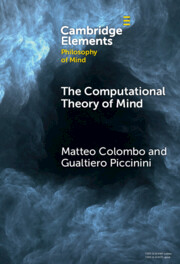
The Computational Theory of Mind
-
- Published online:
- 13 November 2023
- Print publication:
- 07 December 2023
-
- Element
- Export citation
Contemporary art, capitalization and the blockchain: On the autonomy and automation of art's value
-
- Journal:
- Finance and Society / Volume 2 / Issue 2 / 2016
- Published online by Cambridge University Press:
- 09 November 2023, pp. 96-110
-
- Article
-
- You have access
- Open access
- Export citation
Money as a computational machine
-
- Journal:
- Finance and Society / Volume 8 / Issue 2 / 2022
- Published online by Cambridge University Press:
- 09 November 2023, pp. 110-128
-
- Article
-
- You have access
- Open access
- Export citation
Chapter 5 - Chance and Luck
-
- Book:
- Luck, Leisure, and the Casino in Nineteenth-Century Europe
- Published online:
- 24 August 2023
- Print publication:
- 07 September 2023, pp 111-138
-
- Chapter
- Export citation
3 - Beyond Britain and the Book
-
-
- Book:
- The Cambridge History of the Australian Novel
- Published online:
- 28 June 2023
- Print publication:
- 27 July 2023, pp 44-62
-
- Chapter
- Export citation
1 - An Overview of Computational Cognitive Sciences
- from Part I - Introduction
-
-
- Book:
- The Cambridge Handbook of Computational Cognitive Sciences
- Published online:
- 21 April 2023
- Print publication:
- 11 May 2023, pp 3-26
-
- Chapter
- Export citation
37 - Philosophical Issues in Computational Cognitive Sciences
- from Part V - General Discussion
-
-
- Book:
- The Cambridge Handbook of Computational Cognitive Sciences
- Published online:
- 21 April 2023
- Print publication:
- 11 May 2023, pp 1201-1227
-
- Chapter
- Export citation
Chapter 6 - Probability, Belief, and the Richness of Cognition
- from Models of Optimal Beliefs
-
-
- Book:
- The Cognitive Science of Belief
- Published online:
- 03 November 2022
- Print publication:
- 17 November 2022, pp 135-150
-
- Chapter
- Export citation
THE SENSE/REFERENCE DISTINCTION IN CONSTRUCTIVE SEMANTICS
- Part of
-
- Journal:
- Bulletin of Symbolic Logic / Volume 27 / Issue 4 / December 2021
- Published online by Cambridge University Press:
- 28 February 2022, pp. 501-513
- Print publication:
- December 2021
-
- Article
-
- You have access
- Open access
- HTML
- Export citation
19 - Lawyering Somewhere between Computation and the Will to Act
- from Part VI - Fate of the Legal Professions
-
-
- Book:
- The Cambridge Handbook of Lawyering in the Digital Age
- Published online:
- 18 November 2021
- Print publication:
- 25 November 2021, pp 327-357
-
- Chapter
- Export citation
Chapter 10 - Language’s Hopes
- from II - Horizons
-
-
- Book:
- The New Modernist Studies
- Published online:
- 21 January 2021
- Print publication:
- 04 February 2021, pp 200-224
-
- Chapter
- Export citation
Processing APT Spectral Backgrounds for Improved Quantification
-
- Journal:
- / Volume 26 / Issue 5 / October 2020
- Published online by Cambridge University Press:
- 19 August 2020, pp. 964-977
- Print publication:
- October 2020
-
- Article
- Export citation
Social-Computation-Supporting Kinds
-
- Journal:
- Canadian Journal of Philosophy / Volume 50 / Issue 7 / October 2020
- Published online by Cambridge University Press:
- 11 August 2020, pp. 862-877
-
- Article
-
- You have access
- Open access
- HTML
- Export citation
5 - Exploring whole number computation
-
- Book:
- Primary Mathematics
- Published online:
- 05 November 2019
- Print publication:
- 07 August 2019, pp 104-124
-
- Chapter
- Export citation
The Commitment to LOT
-
- Journal:
- Dialogue: Canadian Philosophical Review / Revue canadienne de philosophie / Volume 55 / Issue 2 / June 2016
- Published online by Cambridge University Press:
- 25 July 2016, pp. 313-341
-
- Article
- Export citation