Inflammation plays a vital role in protecting the host from toxins and infection and resolves once these insults have been removed. However, under the presence of triggers, including chronic infection, unhealthy lifestyles and adverse psychological states, an acute inflammatory response can develop into systemic chronic inflammation, which causes tissue degeneration and diseases(Reference Furman, Campisi and Verdin1). Experimental and observational studies have shown that chronic inflammation can increase the risks of diabetes, CVD, cancers, sarcopenia, frailty and all-cause mortality(Reference Ferrucci and Fabbri2).
Healthy dietary patterns, including the Mediterranean diet and Dietary Approaches to Stop Hypertension (DASH), have been associated with lower concentrations of C-reactive protein (CRP) based on the results of systematic reviews and meta-analyses of randomised controlled trials (RCT)(Reference Koelman, Egea Rodrigues and Aleksandrova3,Reference Soltani, Chitsazi and Salehi-Abargouei4) . These dietary patterns have common features: higher intakes of fruit, vegetables, fish, nuts and whole grain combined with lower intakes of red meat, processed foods and refined grains. However, some issues should be resolved for dietary recommendations. First, less remains known about the effects of individual food groups on chronic inflammation, and the results of previous studies examining the effects of individual food groups do not necessarily agree with those of dietary pattern analyses(Reference Salas-Salvadó, Garcia-Arellano and Estruch5,Reference van Bussel, Henry and Ferreira6) . Because food group intakes are often correlated with each other, food groups suggested to affect inflammatory biomarkers by dietary pattern analyses may have inconsistent effects in isolation(Reference Gaesser7). These inconsistent isolated effects with dietary pattern analyses may arise when the findings of dietary pattern analyses examined in one population are applied to another population with a different dietary pattern from the former. Second, relatively few studies have examined the associations between individual food groups and chronic inflammation in Asian populations(Reference Zuñiga, Rebello and Oi8,Reference Yamashita, Yatsuya and Muramatsu9) . Dietary patterns in Japan (e.g. high consumption of white rice and green tea) are distinct from those in other countries(Reference Suzuki, Goto and Ota10). Therefore, more food group analyses should be conducted to clarify the roles of individual food groups on chronic inflammation in the Japanese population.
Therefore, the present cross-sectional study aimed to investigate the associations between individual food groups and high-sensitivity C-reactive protein (hsCRP) among community-dwelling Japanese adults.
Methods
Study design and participants
The present cross-sectional study was based on baseline data from the Japan Multi-Institutional Collaborative Cohort (J-MICC) Study. The J-MICC Study is an ongoing prospective cohort study aiming to detect gene–environment interactions that can lead to lifestyle-related diseases, including cancers(Reference Takeuchi, Naito and Kawai11).
From February 2004 through March 2014, baseline surveys of the J-MICC Study were conducted in fourteen research areas of Japan. The participants were aged 35–69 years at the baseline surveys and recruited from community inhabitants, employees of companies or local governments, health checkup examinees, and first-visit patients at a cancer hospital. All participants completed a self-administered lifestyle-related questionnaire including items on food group intakes and medical history. Height and weight were measured at the health checkups. A total of 92 560 participants were recruited. In three areas (Shiga, Fukuoka, Kyushu or Okinawa), where 23 378 participants were enrolled, hsCRP was measured. Among these participants, the present study further excluded 2757 individuals who had missing data in at least one item necessary to calculate food group intakes, 247 those who had no data on hsCRP, 114 those who had an hsCRP concentration higher than 1·5 mg/dl, 6439 those who had a history of coronary artery disease, stroke, or cancer, or no data about the history of these diseases and 173 those whose total energy intake was beyond 3 sd from the mean of their sex (males: 742–3269 kcal/d, females: 780–2378 kcal/d). Therefore, a total of 13 648 participants (5126 males and 8522 females) were included in the present analysis (Fig. 1). Individuals with an hsCRP concentration higher than 1·5 mg/dl or co-morbidities were excluded because individuals with diseases that elevate hsCRP levels may have modified their dietary habits after the diseases had developed (reverse causation). Regarding the cut-off of hsCRP concentrations, a review article has reported that hsCRP concentration of healthy individuals is usually lower than 1·0 mg/dl(Reference Adukauskienė, Čiginskienė and Adukauskaitė12). Additionally, a meta-analysis has referred to CRP concentrations of > 0·3 mg/dl as high levels and reported that the relative risk of CVD mortality was about 4·0 at 0·9 mg/dl compared with < 0·1 mg/dl. Therefore, we regarded at least hsCRP levels higher than 1·5 mg/dl as pathological(Reference Ni, Yu and Zhang13). The dataset version 200819 was used in the present analysis.
Exposure
Food group intakes were evaluated using a forty-seven-item FFQ(Reference Tokudome, Goto and Imaeda14) and independent items for beverages. The FFQ included items regarding how often the participants had consumed forty-six foods and non-alcoholic beverages during the previous year. The frequencies of eating rice, bread and noodles at breakfast, lunch and dinner were categorised into six levels, from rarely to every day. For these staple foods, the participants were also asked about portion sizes. Regarding the other forty-three foods and beverages, their frequencies were categorised into eight levels, from rarely to three times/d or more, and their portion sizes were predefined. For alcoholic beverages, their frequencies were categorised into six levels, from rarely to seven times per week, and their portion sizes were also queried. In the present analysis, the following food groups were considered as exposures: rice (one item), bread (one item), red meat (one item: beef/pork), processed meat (one item: ham/sausage/bacon), poultry (one item: chicken), dairy products (three items: butter, milk and yogurt), fish (three items: fish, bone-edible small fish and canned tuna), vegetables (ten items: pumpkin, carrots, broccoli, green-leaf vegetables, other green-yellow vegetables, cabbage, radishes, dried radishes, burdock/bamboo shoots and other vegetables), fruit (two items: citrus fruits and other fruits), nuts (one item: peanuts/almonds), coffee (one item: regular/instant coffee) and green tea (one item). These food groups or the nutrients they contain have been reported to be associated with chronic inflammation(Reference Koelman, Egea Rodrigues and Aleksandrova3–Reference van Bussel, Henry and Ferreira6,Reference Cornelis and van Dam15) .
A validation study compared intakes of total energy and twenty-six nutrients estimated using the FFQ with those using a 3-d weighed diet record(Reference Tokudome, Goto and Imaeda16). Log-transformed, energy-adjusted and de-attenuated correlation coefficients for total energy intake and twenty-six nutrients ranged from 0·12 to 0·86 in males and from 0·10 to 0·66 in females. The FFQ also showed high 1-year interval reproducibility for the intakes of total energy, nutrients and food groups(Reference Imaeda, Goto and Tokudome17). Furthermore, another validation study compared food group intakes using the FFQ with those using four 3-d weighed diet records(Reference Imaeda, Goto and Sasakabe18). The energy-adjusted and de-attenuated correlation coefficients between the second FFQ and four 3-d weighed diet records were as follows in males and females, respectively: 0·63 and 0·66 for rice, 0·75 and 0·70 for bread, 0·42 and 0·43 for meat, 0·81 and 0·54 for dairy products, 0·61 and 0·69 for fish, 0·35 and 0·49 for green vegetables, 0·30 and 0·24 for other vegetables, 0·69 and 0·48 for fruit, 0·60 and 0·56 for coffee, and 0·64 and 0·69 for green tea(Reference Imaeda, Goto and Sasakabe18).
In addition to the FFQ, our questionnaire also included items evaluating coffee and green tea consumption. The present analyses used coffee and green tea intakes estimated by these items because they could evaluate a higher amount of drinking beverages than could the FFQ (the amount of coffee and green tea consumption ranged from rarely to five cups/d or more and from rarely to ten cups/d or more, respectively).
Outcomes
At the health checkups, blood samples were drawn in a 7-ml tube for serum and a 7-ml EDTA-2Na-containing tube for plasma and buffy coat. In the Shiga area, serum hsCRP was measured by the latex agglutination method using an N-assay LA CRP-S reagent (Nittobo Medical) and a JCA-BM8060 analyzer (JEOL Ltd). In the Fukuoka and Kyushu or Okinawa areas, the same measurement was conducted by the nephelometry method using an N-latex CRP II reagent (Siemens Healthcare Diagnostics Ltd) and a Behring Nephelometer II analyzer (Siemens Healthcare Diagnostics Ltd). Linear regression showed good agreement between the two measurement methods (Y = 1·0122X + 0·0027, R2 = 0·99 006), where X are hsCRP concentrations measured by the method in the Shiga area and Y are those measured by the method in the Fukuoka and the Kyushu or Okinawa areas.
Covariates
When assessing the associations between food group intakes and hsCRP, the following demographic, lifestyle and dietary factors were adjusted as covariates: age, total energy intake, residential area, educational attainment, smoking exposure, alcohol consumption and total physical activity. These factors have been reported to be associated with chronic inflammation and/or adjusted in observational studies that examined the associations between food group intakes and inflammatory markers(Reference Furman, Campisi and Verdin1,Reference van Bussel, Henry and Ferreira6,Reference Zhang and Zhang19) . In addition to the residual method, total energy intake was incorporated into covariates. This adjustment may reduce random error if total energy intake is associated with hsCRP independent of food group intakes(Reference Willett20). The following continuous variables were converted into categorical variables because possible non-linear associations between these variables and hsCRP should be considered: age, total energy intake, smoking exposure, alcohol consumption and total physical activity. Cut-off values of these categorical variables are presented in Table 1 by sex.
Table 1 Characteristics of participants according to hsCRP quartiles by sex
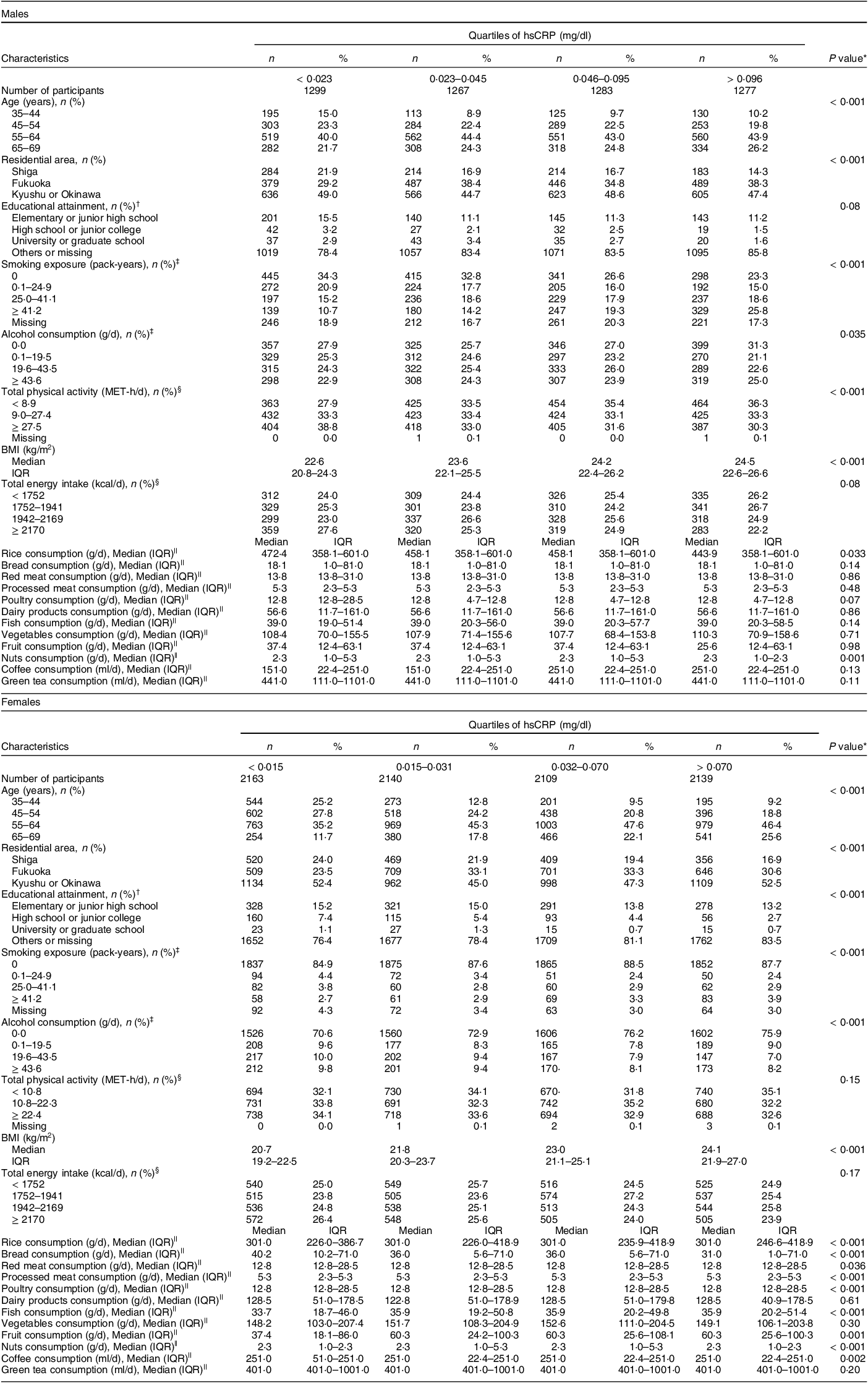
hsCRP, high-sensitivity C-reactive protein; MET-h/d, metabolic equivalent hours per d; IQR, interquartile range.
* Characteriscitcs of participants were compared between quartiles of hsCRP using the Kruskal–Wallis test for continuous variables and the χ 2 of independence for categorical variables.
† Fukuoka and Kyushu or Okinawa area had no data about educational attainment.
‡ These covariates were divided into zero and tertiles above zero.
§ Total physical activity and total energy intake were divided into tertiles and quartiles, respectively.
|| Food group intakes were energy-unadjusted values, which had many tied values, especially among food groups consisting of few items.
Two research areas did not collect data on educational attainment, and thus, all the participants surveyed in those areas were categorised as missing for this covariate. Smoking exposure was calculated as pack-years as follows: (number of smoked cigarettes per d) × (duration of habitual smoking in years)/20. Alcohol consumption was calculated as g/d using the dose and frequency of drinking of each alcohol beverage. Total physical activity was calculated as MET-h/d using the intensity, duration and frequency of three levels of daily total or leisure-time physical activity (daily total physical activity consists of strenuous activity, walking and standing; leisure-time physical activity consists of vigorous, moderate and light activity). BMI was calculated as weight divided by height in metres squared (kg/m2). Self-reported weight and/or height were used to calculate BMI for 337 participants whose weight and/or height had not been measured. Five participants were excluded from the analysis because their self-reported height and/or weight were missing (Figure).
Statistical analysis
All statistical analyses were stratified by sex. Participant characteristics were expressed as geometric means (95% CI) for hsCRP or medians (interquartile ranges) for other continuous variables, and as numbers and proportions (%) for categorical variables. Associations between participant characteristics and the quartiles of log-transformed hsCRP concentrations were examined using the Kruskal–Wallis test for continuous variables because of deviation from normality on quantile–quantile plots or unequal variances. The χ 2 test of independence was adopted for categorical variables. Additionally, characteristics were compared between participants included in the present analysis and those excluded because their total energy intake deviated from the mean of their sex or because they had missing data for at least one item necessary to calculate food group intakes. These comparisons used the Mann–Whitney test for continuous variables and the χ 2 test of independence for categorical variables.
Food group intakes were log-transformed and energy-adjusted using the residual method(Reference Willett20), and Pearson’s correlation coefficients were calculated between food group intakes. The present study defined Pearson’s correlation coefficients of 0·00–0·09 as negligible, 0·10–0·39 as weak, 0·40–0·69 as moderate, 0·70–0·89 as strong and 0·90–1·00 as very strong(Reference Schober, Boer and Schwarte21). Energy-adjusted food group intakes were then categorised into quartiles. Their cut-off values were calculated by exponentiating the estimates of the residual method, which were the sum of an expected log-transformed food group intake for the mean total energy intake and a residual of a log-transformed food group intake(Reference Willett20).
Associations between the quartiles of energy-adjusted food group intakes and log-transformed hsCRP were examined using multiple linear regression, and their exponentiated coefficients for log-transformed hsCRP were estimated with their 95 % CI. Food group intakes were categorised into quartiles to deal with possible non-linear associations with hsCRP, and quartile 1 was treated as a reference category. Exponentiated coefficients indicate the concentration ratios of hsCRP comparing quartiles 2–4 with quartile 1 of food group intake. The present study established the following three models: (1) a model that incorporated one food group as an exposure and was adjusted for age and total energy intake, (2) a model that was further adjusted for all the covariates listed in the section ‘covariates’ and (3) a model that further incorporated all the other food groups as exposures. All the multivariable models incorporated missing categories for the covariates with missing values (educational attainment, smoking exposure, alcohol consumption and total physical activity). According to a recommendation of a methodological review, both models 2 and 3 were established because model 3 eliminated potential confounding from food groups other than an exposure, but the result may not have been applicable to the whole population because of restrictions in underlying food patterns(Reference Ibsen, Laursen and Würtz22). To address a concern about model 3’s excessive adjustment, we calculated variance inflation factors in model 3. Furthermore, an additional analysis was conducted among food groups whose P trends turned from statistically significant to non-significant after adjusting for other food groups. This additional analysis incorporated only one food group as a covariate based on their correlation (Pearson’s correlation coefficients of 0·10 or higher in absolute values) with exposures of interest. P trend values were also calculated by entering ordinal variables defined as 0, 1, 2 and 3 for quartiles 1–4 of food group intakes, respectively, as continuous variables in the models.
As a sensitivity analysis, multiple imputation was conducted in each sex by combining 868 males and 1470 females excluded from the main analysis using the complete data with 5126 males and 8522 females included in the main analysis. The former participants had missing data for at least one item necessary to calculate food group intakes but satisfied no other exclusion criteria. See Supplemental Text for the detailed methods of multiple imputation. All statistical tests were two-sided, and P < 0·05 was considered statistically significant. Stata 13.1 and 17.0 (StataCorp) was used for statistical analyses.
Results
Table 1 shows the participants’ characteristics according to the quartiles of hsCRP by sex. In males, those with a higher hsCRP concentration tended to be older, to live in Fukuoka, to have higher smoking exposure, to be physically inactive and to have a higher BMI. Regarding food groups, males with a higher hsCRP concentration were more likely to have a lower intake of rice and nuts. In females, those with a higher hsCRP concentration tended to be older, to live in Fukuoka, to have lower educational attainment and higher smoking exposure, to drink less alcohol and to have a higher BMI. Regarding food groups, females with a higher hsCRP concentration were more likely to have higher intakes of rice, fish and fruit and lower intakes of bread, red and processed meat, poultry, nuts and coffee. The exclusion of potential participants according to deviations in total energy intake was not strongly associated with the values of study variables (see online supplementary material, Supplemental Table 1 and Section A of Supplemental Text). However, associations were clear between exclusions according to missingness in at least one item necessary to calculate food group intakes and the values of study variables (see online supplementary material, Supplemental Table 2 and Section A of Supplemental Text).
Pearson’s correlation coefficients showed moderate-strength correlations between red meat and poultry intake in both sexes and between vegetables and fruit in males (see online supplementary material, Supplemental Table 3). Online Supplemental Table 4 shows the estimated ranges of energy-adjusted intakes of food groups by sex. The geometric mean of hsCRP was 0·048 mg/dl (95 % CI 0·047, 0·050) in males and 0·034 mg/dl (95 % CI 0·034, 0·035) in females.
Table 2 shows associations between the quartiles of food group intakes and hsCRP concentrations by sex. In males, model 3, which incorporated all food groups as exposures, provided significant concentration ratios of hsCRP comparing quartiles 4 and 1 among three food group intakes: 1·12 (95 % CI 1·02, 1·22; P trend = 0·014) for processed meat, 1·13 (95 % CI 1·03, 1·24; P trend = 0·025) for fish and 0·83 (95 % CI 0·76, 0·90; P trend < 0·001) for nuts. The results of model 3 were almost the same as those of model 2, which incorporated only one food group intake as an exposure. In females, model 3 showed two positive concentration ratios: 1·11 (95 % CI 1·03, 1·19; P trend = 0·002) for processed meat and 1·19 (95 % CI 1·11, 1·29; P trend < 0·001) for fruit. On the other hand, inverse correlations were found in following four concentration ratios: 0·89 (95 % CI 0·81, 0·97; P trend = 0·010) for bread, 0·86 (95 % CI 0·80, 0·92; P trend < 0·001) for vegetables, 0·90 (95 % CI 0·84, 0·97; P trend = 0·001) for nuts and 0·88 (95 % CI 0·82, 0·95; P trend < 0·001) for green tea. The P trend for rice and poultry was significant in model 2, but non-significant in model 3, and vice versa for processed meat. Variance inflation factor did not indicate excessive multicollinearity in both sexes; they were smaller than four for all the ordinal and continuous variables (see online supplementary material, Supplemental Table 5). In an additional analysis, which incorporated only one food group as a covariate, model 2’s statistically significant P trend was diluted in rice but retained in poultry (see online supplementary material, Supplemental Table 6). The directions of the associations were consistent between the analysis using complete data and that using multiply imputed data (Table 2, see online supplementary material, Supplemental Table 7 and Section C of Supplemental Text).
Table 2 Associations between energy-adjusted intakes (residuals) of each food groups and hsCRP by sex
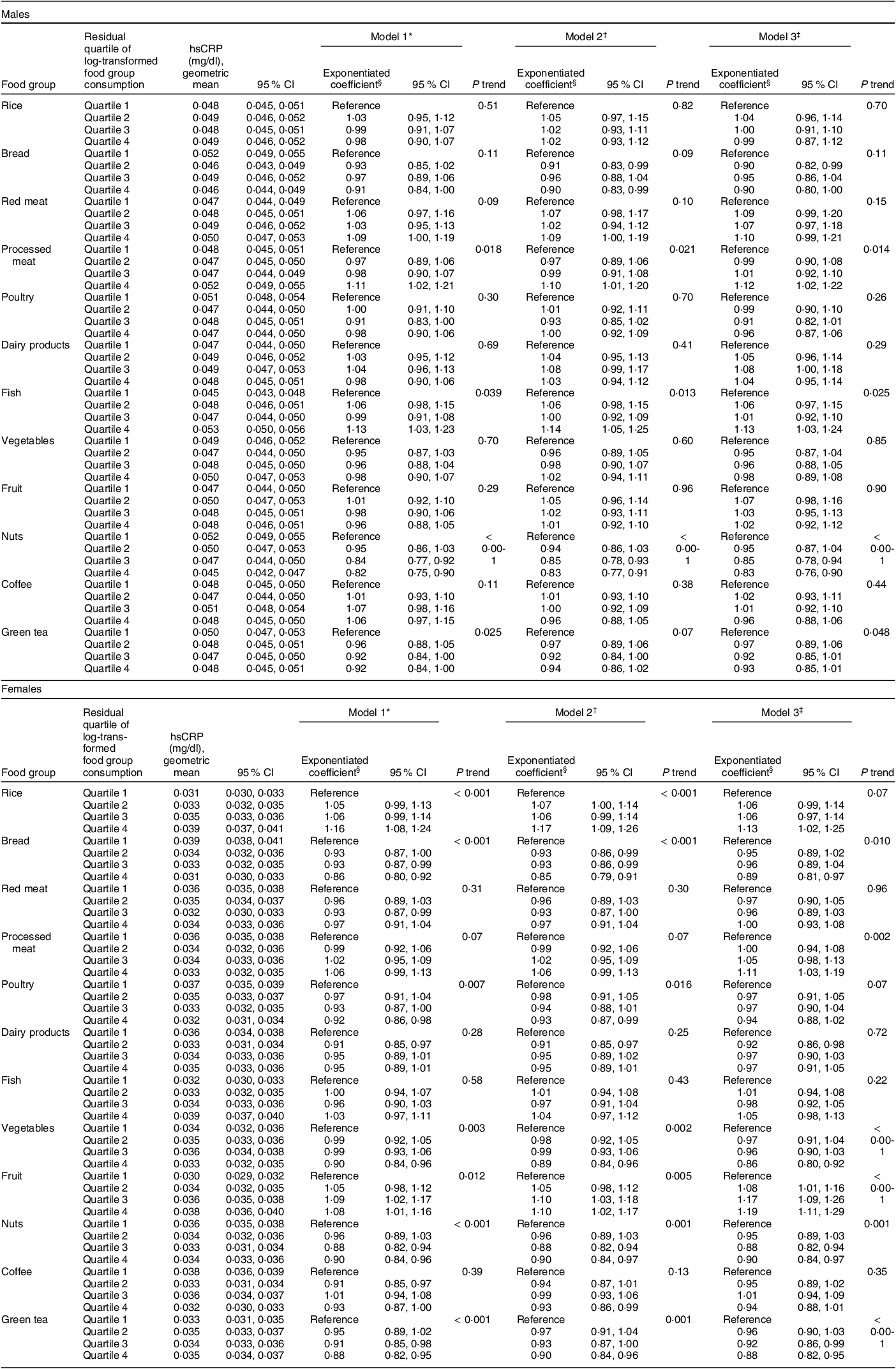
hsCRP, high-sensitivity C-reactive protein; MET-h/d, metabolic equivalent hours per d.
* Adjusted for age (35–44, 45–54, 55–64, 65–69 years) and total energy intake (males: < 1752, 1752–1941, 1942–2169, ≥ 2170 kcal/d; females:< 1443, 1443–1574, 1575–1701, ≥ 1702 kcal/d).
† Adjusted for confounding factors in model 1 and residential area (Shiga, Fukuoka, Kyushu or Okinawa), educational attainment (elementary or junior high school, high school or junior college, university or graduate school, others or missing), smoking exposure (males: 0, 0·1–24·9, 25·0–41·1, ≥ 41·2 pack-years, missing; females: 0, 0·1–9·1, 9·2–19·9, ≥ 20·0 pack-years, missing), alcohol consumption (males: 0·0, 0·1–19·5, 19·6–43·5, ≥ 43·6 g/d; females: 0·0, 0·1–4·7, 4·8–13·7, ≥ 13·8 g/d) and total physical activity (males: < 8·9, 9·0–27·4, ≥ 27·5 MET-h/d, missing; females: < 10·8, 10·8–22·3, ≥ 22·4 MET-h/d, missing).
‡ Adjusted for confounding factors in model 2 and the residual quartiles of other log-transformed food group consumption.
§ Exponentiated coefficients are ratios of estimated hsCRP concentration for the food group consumption of quartiles 2–4 to that for quartile 1.
Discussion
Previous dietary pattern analyses have demonstrated that healthy dietary patterns can lower hsCRP concentrations(Reference Koelman, Egea Rodrigues and Aleksandrova3,Reference Soltani, Chitsazi and Salehi-Abargouei4) . These patterns have common characteristics of higher intakes of fruit, vegetables, fish, nuts and whole grain combined with lower intakes of red meat, processed foods and refined grain. The present study confirmed the associations among processed meat, vegetables and nuts reported by dietary pattern analyses. Interestingly, however, the present study found that bread intake was inversely and fish and fruit intakes positively associated with hsCRP concentrations.
The inconsistent findings of the present study compared with those of the previous dietary pattern analyses may have derived from differences in dietary patterns between the former and the latter. The present study found weak positive correlations among fruit, vegetables and fish but also found positive or no correlations between these food groups and red and processed meat, which differs from those suggested by previous dietary pattern analyses (see online supplementary material, Supplemental Table 3). Moreover, the present participants had high intakes of rice and green tea (see online supplementary material, Supplemental Table 4), which are also distinct from the dietary patterns reported in a previous study(Reference Suzuki, Goto and Ota10). Other possible explanations for the inconsistency with previous dietary pattern analyses are the differences in genetic backgrounds and the accompanying responsiveness of inflammatory processes between the study participants.
In the present study, rice and bread intake would have been mostly occupied by white rice and bread because the consumption of whole grain has been reported to be low among East Asian populations, including Japanese(Reference Micha, Khatibzadeh and Shi23). Although whole grain intake has been linked to reduced inflammation levels in a meta-analysis of RCT(Reference Xu, Wan and Feng24), few studies have examined the effects of refined grain on chronic inflammation. However, both white rice and white bread have a high glycaemic index(Reference Atkinson, Foster-Powell and Brand-Miller25), which has been associated with higher inflammation levels and chronic diseases in systematic reviews of observational studies(Reference Hardy, Garvin and Xu26). The present analysis showed that rice marginally raised, but bread lowered, hsCRP concentrations in females. Three factors could explain this inconsistency. First, the estimated bread intake was far lower than rice intake in the present study (see online supplementary material, Supplemental Table 4), and thus, bread intake may have been insufficient to exert its effect of a high glycemic index. Second, dietary fibre has been shown to improve the degree of inflammation in experimental and interventional studies(Reference Barber, Kabisch and Pfeiffer27). The dose of dietary fibre in white bread (4·2 g/100 g) is higher than that in rice (1·5 g/100 g)(28). Thus, in white bread, the protective effect of dietary fibre on inflammation may surpass the negative effect of its high glycaemic index, and even a relatively low intake of white bread might be sufficient to suppress inflammation.
Red and processed meat has been shown to promote inflammation in several observational studies(Reference Ley, Sun and Willett29,Reference van Woudenbergh, Kuijsten and Tigcheler30) , but others have found an inverse or no association between poultry intake and chronic inflammation(Reference van Bussel, Henry and Ferreira6,Reference van Woudenbergh, Kuijsten and Tigcheler30) . In the present study, processed meat increased hsCRP concentrations, whereas red meat and poultry had marginally positive and negative effects, respectively. Experimental studies have reported that exposing red meat to high temperatures or elevated pH levels produces advanced glycation end products, polycyclic aromatic hydrocarbons and heterocyclic amines, all of which can enhance inflammation(Reference Kim, Keogh and Clifton31,Reference Chiang and Quek32) . Therefore, processed meat could have stronger potential to raise hsCRP concentrations compared with red meat and poultry. Additionally, poultry includes a lower content of heme iron and N-glycolylneuraminic acid than do beef and pork, and these substances can trigger inflammatory processes(Reference Demeyer, Mertens and De Smet33). The non-significant effect of red meat on hsCRP concentrations in the present study may also have derived from lower red meat intakes (the cut-offs of quartile 4 were 27·3 and 27·2 g/d in males and females, respectively) compared with previous studies (the cut-offs of the highest category were 104 g/d in Ley et al.’s study(Reference Ley, Sun and Willett29) and 97·7 g/d in van Woudenbergh et al.’s study(Reference van Woudenbergh, Kuijsten and Tigcheler30)). Although the FFQ used in the present study tended to underestimate meat intake in individuals with higher consumption(Reference Imaeda, Goto and Sasakabe18), the differences in red meat intake between the present and previous studies seemed large (see online supplementary material, Supplemental Table 4).
Dairy products have been shown to have a neutral or a beneficial effect on inflammatory biomarkers in two meta-analyses of RCT(Reference Labonté, Couture and Richard34,Reference Moosavian, Rahimlou and Saneei35) . The present study found no significant associations between dairy products intake and hsCRP, which partly agreed with the findings of previous studies. Although dairy products are rich in SFA, a systematic review of RCT showed that the fat content of dairy products may not affect inflammatory markers(Reference Telle-Hansen, Christensen and Ulven36).
Fish consumption has been linked to lower inflammatory biomarker concentrations in several observational studies(Reference van Bussel, Henry and Schalkwijk37,Reference Emanuele and Meliker38) and to reduced risks of coronary artery disease, stroke and all-cause mortality in two meta-analyses of prospective studies(Reference Bechthold, Boeing and Schwedhelm39,Reference Schwingshackl, Schwedhelm and Hoffmann40) . However, our analyses found that fish intake was correlated with higher hsCRP concentrations in males. The following factors could explain this discrepancy. First, the processing, shipping and storage of fish often involve high or low temperatures, light and oxygen, which degrade cholesterol to form cholesterol oxidation products and promote inflammation(Reference Dantas, Sampaio and Ferreira41). Second, fish contains methylmercury, a proinflammatory substance. Yet, a cross-sectional study reported that blood mercury levels were not associated with CRP concentrations after adjusting for fish intake(Reference Emanuele and Meliker38).
Fruit and vegetables have been shown to ameliorate inflammation in a meta-analysis of observational and interventional studies(Reference Hosseini, Berthon and Saedisomeolia42). In the present study, however, fruit intake was related to a higher hsCRP concentration in females. Although fruit and vegetables contain many anti-inflammatory compounds such as dietary fibre, folate, vitamins and phytochemicals(Reference Hosseini, Berthon and Saedisomeolia42), fruit also has high fructose content. Experimental studies have demonstrated that excessive fructose intake can enhance macrophage infiltration into adipocytes and promote the release of proinflammatory cytokines(Reference DiNicolantonio, Mehta and Onkaramurthy43). Additionally, excessive fructose consumption may induce weight gain(Reference DiNicolantonio, Mehta and Onkaramurthy43). In the present study, however, Spearman’s rank correlation coefficients showed negligible correlations between the residuals of log-transformed and energy-adjusted fruit intake and BMI (males: r = 0·000, P = 0·48; females: r = 0·04, P < 0·001). Further, a systematic review of meta-analyses revealed that raw or dried fruit can decrease the risk of chronic disease and predisposing factors, but that canned fruit may increase the risk of all-cause mortality(Reference Fardet, Richonnet and Mazur44). Finally, the positive association between fruit intake and hsCRP may have derived from limitations of the present FFQ, which included only two items about fruit and did not distinguish raw fruit from canned fruit. These limitations may have led to a lower intake and negated the anti-inflammatory effect of raw fruit. The findings of the present study should be corroborated by cohort studies or RCT, and closer attention may need to be paid to the potential proinflammatory effects of fruit to recommend prudent fruit intake.
Few observational studies have reported the anti-inflammatory effects of nuts(Reference Salas-Salvadó, Garcia-Arellano and Estruch5,Reference Yu, Malik and Keum45) , and these effects have not been confirmed by a meta-analysis of RCT(Reference Xiao, Xia and Ke46). However, nuts are rich in anti-inflammatory substances, including dietary fibre, Mg, phenolic compounds, unsaturated fatty acids and L-arginine(Reference Yu, Malik and Keum45). A meta-analysis of observational studies has also reported that nuts can lower the risk of coronary artery disease(Reference Bechthold, Boeing and Schwedhelm39). Further studies are needed to confirm the potential anti-inflammatory effect of nuts.
In the present analyses, coffee intake had no effect on hsCRP concentrations in either sex, but green tea had an inverse relationship with hsCRP in females. Coffee contains anti-inflammatory and antioxidative compounds such as polyphenols, diterpenes, chlorogenic acids and caffeine(Reference Moua, Hu and Day47). Two meta-analyses of cross-sectional studies have investigated the association between coffee intake and CRP concentrations(Reference Zhang and Zhang19,Reference Moua, Hu and Day47) , but only one found an inverse association(Reference Zhang and Zhang19). The lack of an association found in a previous meta-analysis and the present analysis may have derived from several factors. First, coffee is often consumed with sugar, which could inhibit the anti-inflammatory and antioxidative potential of coffee. Second, individuals who consume higher amounts of coffee may have unhealthy lifestyles. However, in the present study, coffee intake was not associated with hsCRP concentrations, even after adjusting for lifestyle-related factors, including smoking and drinking (models 2 and 3). Third, the moderate coffee consumption seen in the present study may have led to a null association. Yet, a meta-analysis that found no association between coffee consumption and CRP levels included studies with the highest mean coffee intakes of 1037 and 1305 ml/d(Reference Moua, Hu and Day47). Green tea is a rich source of catechins, which also have anti-inflammatory and antioxidative effects. However, two meta-analyses of RCT reported finding no significant effects of green tea or green tea extracts, including catechins, on CRP concentrations(Reference Haghighatdoost and Hariri48,Reference Serban, Sahebkar and Antal49) . The sample sizes of those two meta-analyses were 887 and 377, which may have led to lower statistical powers than that of the present study. On the other hand, the present results may have been affected by residual confounding from unmeasured confounding factors.
Model 2, which incorporated only one food group as an exposure, and model 3, which incorporated all the food groups as exposures, generally showed consistent results. In females, however, the P trends of model 3 were non-significant for rice and poultry, which differed from those of model 2 (Table 2). These diluted associations may have derived from an adjustment from correlated food groups, and this speculation was partly confirmed by an additional analysis adjusted for only one food group correlated with the examined food group, especially in rice (see online supplementary material, Supplemental Table 6). Whereas model 3 has the strength of eliminating potential confounding from other food groups, its limitation of fixing dietary patterns should be mentioned.
The present study has three main strengths: a relatively large sample of community-dwelling populations, the inclusion of multiple food groups as exposures and adjustments for potential confounding factors. Still, six limitations should be mentioned. First, reverse causation may have affected the present study. Although the present study excluded persons with hsCRP concentrations higher than 1·5 mg/dl and/or a history of coronary artery disease, stroke or cancer, we cannot infer causality only from the present results. Second, food group intakes were estimated using an FFQ, and thus, estimated intakes may have included some misclassification, especially in food groups with relatively low validity, such as meat and vegetables. However, food group intakes of the present study other than fruit were generally comparable to those of a study conducted in multiple areas of Japan(Reference Nanri, Shimazu and Ishihara50). Third, the data used in the present study included missing values for many variables. Yet, the results of multiple imputation analysis were materially unchanged from those of the main analysis (Table 2 and see online supplementary material, Supplemental Table 7). Fourth, residual confounding from unmeasured confounding factors may have produced the differences in the results between the previous RCT and our study. Fifth, the present study conducted multiple comparisons. However, since the present study did not aim to generalise the results of one food group to other food groups or those of one sex to another (individual testing), corrections for multiple testing are not appropriate(Reference Rubin51). Sixth, the present analyses were conducted using data from volunteering or participants in health checkups (the response proportion among eligible individuals was 38·5 %), who would be more likely to have high health-consciousness. Therefore, the correlations in general populations may differ from the findings of the present study.
The results of the present study have two implications for clinical practice and research. First, food group analyses can have the strength in clarifying which food groups composing currently proposed dietary patterns are influential on chronic inflammation. Second, our findings suggest that recommendations for individual food groups based on the results of dietary pattern analyses might require some modifications, especially for the Japanese population.
In conclusion, female bread intake was associated with lower and female fruit and male fish consumption was with higher hsCRP levels. These findings, combined with the results from dietary pattern analyses, would be informative to prevent chronic inflammation and its consequences.
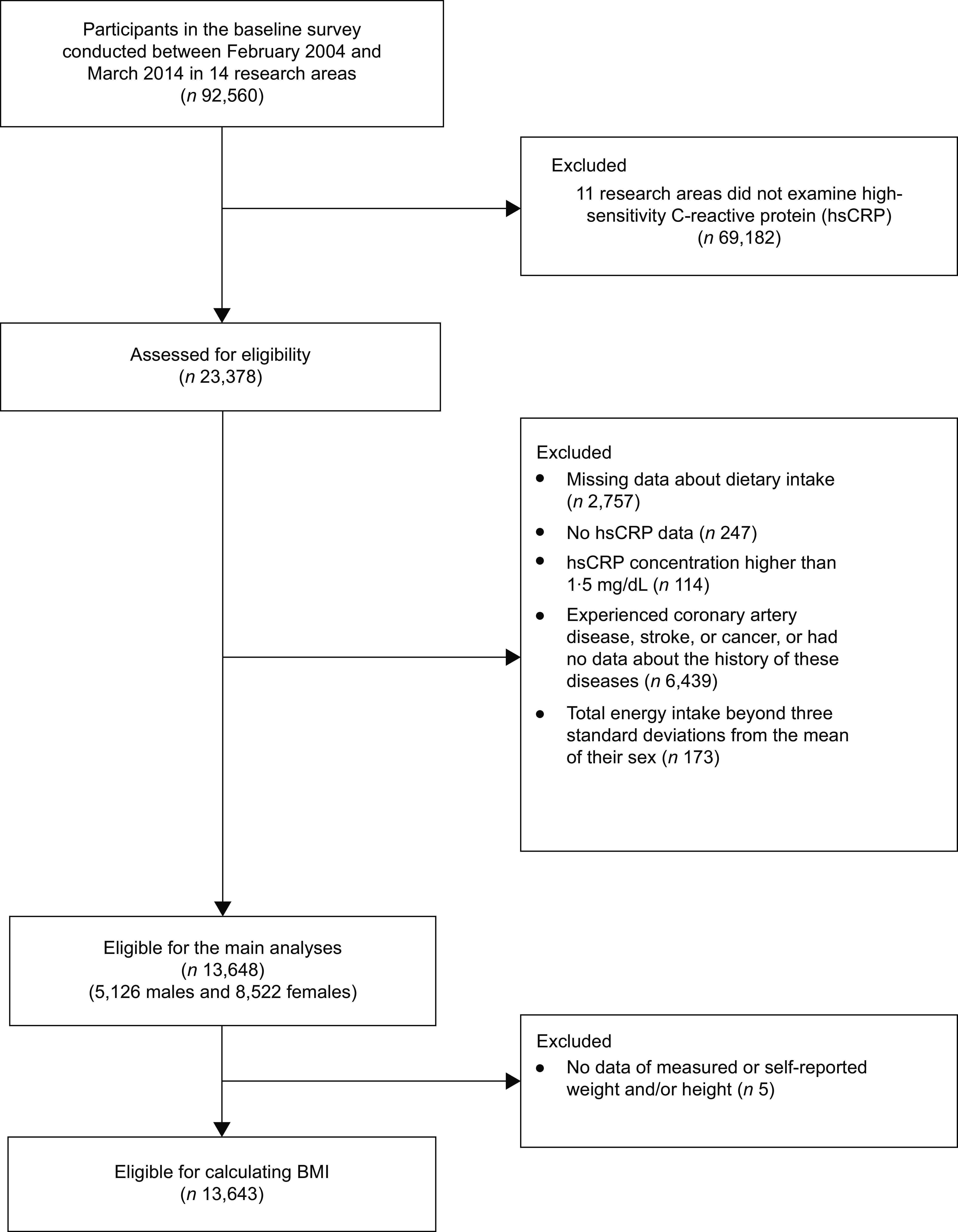
Fig. 1 Flow diagram showing the selection of eligible participants
Acknowledgements
The authors are grateful to the previous principal investigators of the J-MICC Study, Drs Nobuyuki Hamajima and Hideo Tanaka, for establishing and following up the cohort.
Financial support
This study was supported by Grants-in-Aid for Scientific Research for Priority Areas of Cancer (No. 17015018) and Innovative Areas (No. 221S0001) from the Japan Society for the Promotion of Science and by the Platform of Supporting Cohort Study and Biospecimen Analysis (CoBiA, JSPS KAKENHI Grant Nos. 16H06277 and 22H04923) from the Japanese Ministry of Education, Culture, Sports, Science and Technology. None of the funding sources had roles in the design, analysis or writing of this article.
Conflict of interest
There are conflicts of interest
Authorship
T.M. and K.W. designed the research; Y.T., Y.K., Y.K., R.O., M.N., T.T., A.H., H.I., J.O., N.T. and N.M. conducted the research; N.I. and C.G. provided essential materials; T.M. and K.W. performed the statistical analysis; T.M. and K.W. wrote the manuscript; K.M. supervised the J-MICC Study; and T.M. had primary responsibility for the final content. All authors read and approved the final manuscript.
Ethics of human subject participation
This study was conducted according to the guidelines laid down in the Declaration of Helsinki, and all procedures involving research study participants were approved by the ethics committee of Nagoya University Graduate School of Medicine (approval No.: 2010-0939-8) and other institutions participating in the J-MICC Study. All participants provided written informed consent prior to participation.
Data sharing plan: The data used in the present analysis are not publicly available because the participants did not provide consent for data sharing. The data can be available upon reasonable request to Dr Kenji Wakai at the Department of Preventive Medicine, Nagoya University Graduate School of Medicine. Readers who are interested in the FFQ used in the present study can contact Dr Nahomi Imaeda ([email protected]).
Supplementary material
For supplementary material accompanying this paper visit https://doi.org/10.1017/S1368980024001599