Premenstrual syndrome (PMS) is a common cyclic psychological and somatic disorder affecting a large number of women, which consistently occurs during the luteal phase of the menstrual cycle(Reference Liu, Wang and van Heck1). It is characterized by emotional, behavioural and physical symptoms and can manifest with a wide variety of symptoms, including depression, mood liability, irritability, breast tenderness and sleep disturbances(Reference Naheed, Kuiper and Uthman2). It has been indicated that 47·8 % of women are diagnosed with PMS and the highest prevalence has been reported from Iran, approximately 98 % (95 % CI: 97, 100)(Reference Direkvand-Moghadam, Sayehmiri and Delpisheh3). The high prevalence and great impact on quality of life of the women affected leaves PMS as an unresolved yet important issue in women’s health. The pathology of PMS has not yet been fully-understood. It is proposed that the PMS results from ovulation and may be due to ovarian steroid interactions relating to neurotransmitter dysfunction(Reference Liu, Wang and van Heck1). The potential contributors include central nervous system (CNS) sensitivity to reproductive hormones, genetic background, nutritional indices and psychosocial factors(Reference Hantsoo and Epperson4,Reference Saeedian Kia, Amani and Cheraghian5) . In this regard, nutritional factors are the most modifiable parameters that can be taken into account as a strategy in PMS management.
Little information is available regarding particular nutrients, foods or dietary patterns that might be associated with this syndrome. It seems that the excess or deficiency of certain nutrients might be due to hormonal and neurotransmitter imbalances(Reference Stachowicz and Lebiedzińska6). Furthermore, certain foods, such as vegetables, and dietary patterns including low-fat and high-fibre diets are related to decreased plasma oestrogen level and the duration of premenstrual symptoms(Reference Farasati, Siassi and Koohdani7). Furthermore, studies assessing food-intake have revealed that the excess consumption of sweet-tasting food items, fast foods, deep-fried meals, coffee and alcohol are significantly related to the development of PMS(Reference Farasati, Siassi and Koohdani7).
According to our knowledge, studies regarding the association of empirically derived dietary patterns and the risk of PMS are limited. Conceptually, assessing the association between dietary patterns and the likelihood of PMS is very close to reality, because foods are consumed as part of a whole diet. On the other hand, dietary patterns might be different based on cultures, geography and beliefs of different populations(Reference Kant, Graubard and Schatzkin8,Reference Hu9) . Limited data are available regarding the association between major dietary patterns and risk of PMS in Middle Eastern countries(Reference Mirmiran, Bahadoran and Vakili10). The purpose of the current study was to investigate the association between major dietary patterns and premenstrual syndrome among Iranian women aged 20–45 years in the context of a case-control study.
Methods
Study population
The current case-control study was conducted among women with PMS and healthy women as a control group referring to health centres in 2017. The study population consisted of 225 women with PMS and 334 healthy controls aged 20–45 years. The PMS patients included in the present study were those with confirmed diagnosis of moderate/severe PMS using the Premenstrual Symptoms Screening Tool (PSST), Body Mass Index (BMI) less than 39·9 kg/m2, without specific habits such as smoking, alcoholism, any chronic disease, specific diet, medication therapy or vitamins and minerals supplementation. On the other hand, participants who did not respond to more than 35 items of the Food Frequency Questionnaire (FFQ) or reported total daily energy intake outside the range of 3347–17 572 kJ (800–4200 kcal) were excluded from the analysis. The control group consisted of age-matched women without premenstrual symptoms according to the PSST questionnaire. Similar exclusion criteria applied for the PMS case were used for the control group.
Written informed consent was completed for each individual. The study protocol was approved by the regional bioethics committee (Project number: 395518, Ethical approve number: IR.MUI.REC.1395.3.518). This study is reported based on Strengthening the Reporting of Observational Studies in Epidemiology (STROBE) guidelines(Reference von Elm, Altman and Egger11).
Data collection
Dietary intake assessment
Dietary intake was assessed using a validated, self-administered, semi-quantitative food-frequency questionnaire (FFQ). Participants reported the frequency of 168 different food and beverage items consumed during the last year. The reliability and validity of the FFQ has been previously reported in the Iranian population(Reference Mirmiran, Esfahani and Mehrabi12). Trained nutritionists filled in the FFQ through face-to-face interviews. Participants were requested to report the frequency of usual food items consumed in the preceding year on a daily, weekly or monthly basis, considering the standard portion size for each food item. The frequency of foods consumed was converted to gram per day using standard household measures. Subsequently, energy and macro and micronutrient intake was determined using the US Department of Agriculture food composition database using the Nutritionist IV programme. We categorized the food items into 36 predefined food groups in order to derive the dietary patterns (Table 1).
Table 1 Food groups used in the principal component analysis
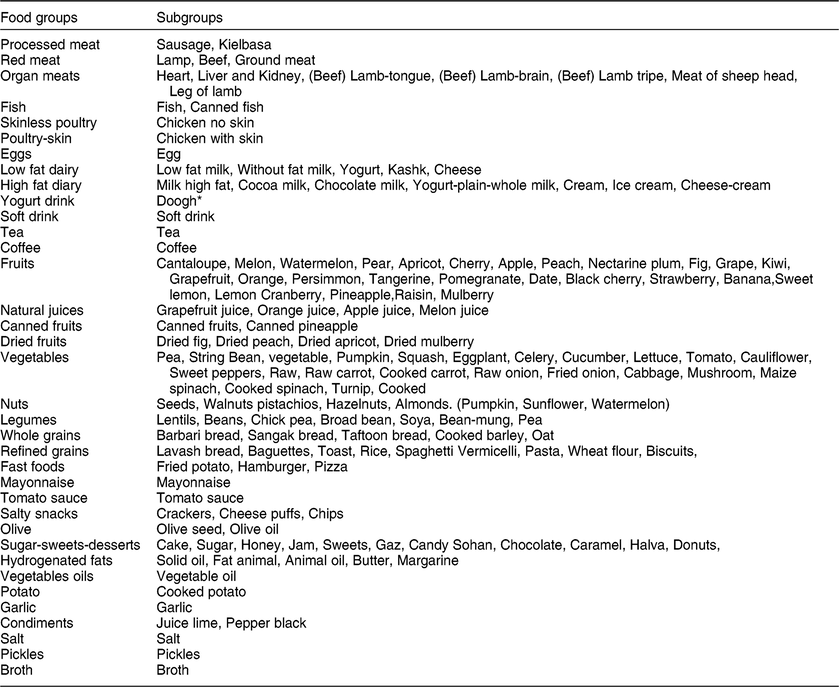
* Doogh is a cold yogurt-based beverage.
Case confirmation
In the current study, PMS was confirmed by the Premenstrual Symptoms Screening Tool (PSST) questionnaire. This tool is a simple, user-friendly screening tool with high reliability and validity for identifying women with Premenstrual Syndrome (PMS)(Reference Hariri, Moghaddam-Banaem and Siah Bazi13) and comprises nineteen items subdivided into two domains: the first domain consists of fourteen items related to premenstrual symptoms, whilst the second domain is composed of five items that assess the functional impact of premenstrual symptoms.
In order to capture the criteria of premenstrual symptoms, the following question was asked ‘Do you experience some or any of the following premenstrual symptoms, which start before your period and stop within a few days of bleeding?’ The symptoms listed were anger/irritability, anxiety/tension, tearful, depressed mood/hopelessness, decreased interest at work, decreased interest at home, decreased interest in social activities, difficulty in concentrating, fatigue/lack of energy, overeating/food cravings, insomnia, hypersomnia, feeling overwhelmed, and physical symptoms (breast tenderness, headaches, joint/muscle pain, bloating, weight gain). In order to assess the functional impact of the premenstrual symptoms, women were asked ‘Have your symptoms interfered with the following five domains: relationship with your family, work efficiency or productivity, relationship with co-workers, social life activities or home responsibilities’(Reference Steiner, Macdougall and Brown14). Each item was rated according to a 4-point Likert scale (0 = absent; 1 = mild; 2 = moderate; 3 = severe). In order to detect moderate to severe PMS, the following three conditions must have qualified. (1) From items 1 to 4, at least one of them must be moderate or severe. (2) At least four items of 1 to 14 should be moderate or severe. (3) In addition to the previous conditions, among the last five items, at least one of them must be moderate or severe.
Assessment of other variables
Other required information such as age (years), marital status (married/single or widow), education (lower than diploma/diploma (12 years of formal education)/Basic Sciences/post-graduate education), socio-economic status and medication use were obtained using a pretested questionnaire.
Socio-economic status (SES) of the study participants was evaluated based on the following binary variables: education level of both subjects and the family head (academic/non-academic), job of both subjects and the family head (yes/no), family size (fewer than 4/higher than 4), having a car (yes/no), home status (the owner/tenant), and having foreign travel (yes/no) by using self-reported questionnaire, and then a total score was calculated.
Furthermore, physical activity was examined using the International Physical Activity Questionnaire (IPAQ) and expressed as metabolic equivalent-min/week. The validity and reliability of IPAQ has been previously determined(Reference Craig, Marshall and Sjöström15). Participants were asked to recall their type of physical activity and its intensity as well as the duration of activity for the past 7 d. To estimate total daily physical activity level, respective metabolic equivalents (MET) values (for instance, walking = 3·3, moderate-intensity activity = 4·0, vigorous-intensity activity = 8·0) were multiplied by duration time (min), and then summed. Data obtained from the physical activity questionnaire were expressed as daily metabolic equivalents (MET-min/d). Afterwards, continuous values were categorized into high, moderate, and mild levels(Reference Noori, Ghiasvand and Maghsoudi16).
Participants’ weights were measured using a balanced digital scale (SECA, Model no: 710, Germany) to the nearest 0·05 kg. Height was recorded using a wall-mounted tape-meter. Height was measured in a standing position with a tape measure to the nearest 0·5 cm in the normal position. Participant’s weight and height were measured with light clothing and barefoot. Body max index was calculated as weight (kg) divided by the square of height (m2). Hip circumference was measured with a tape measure to the nearest 1 mm at the widest point between hip and buttock. Waist circumference was also measured to the nearest 1 mm at the midway level between the lower rib margin and the iliac crest using a steel tape measure, and waist–hip ratio (WHR) was estimated by dividing waist circumference (cm) to hip circumference (cm).
Statistical analysis
Exploratory factor analysis with Principal component analysis (PCA) as the extraction method was used for extracting the dietary patterns from 36 food groups consumed. Factor analysis is a statistical method used to describe variability among observed, correlated variables (in the current study, food groups) in terms of a potentially lower number of unobserved variables, called factors (in this study; dietary patterns). Factor analysis searches for such joint variations in response to unobserved latent variables (dietary pattern). The observed variables (food groups) are modelled as linear combinations of the potential factors, plus ‘error’ terms(Reference Bartholomew, Steele and Galbraith17).
Scree plot and eigenvalue greater than 1·5 was used for determining the appropriate number of factors. An orthogonal rotation method – i.e. Varimax – was used for improving the interpretability of extracted factors. Finally, three factors were considered as the major dietary patterns and were labelled based on the interpretation of the highly loaded food groups in each factor. For each participant, factor scores of dietary patterns were calculated by using a regression method in which, by summing the intake of food groups weighted by factor loading, each participant received a factor score for each identified dietary pattern. KMO and Bartlett’s tests were used to evaluate the adequacy of sample size and the applicability factor analysis method on our data. Participants were categorized into tertiles based on each dietary pattern’s score. To assess the association between dietary patterns and the chance for developing PMS, the binary logistic regression was used in crude and different multivariable adjusted models. An adjustment for age, marital status, education and socio-economic status (SES) was done in the first model. Further adjustments were conducted for BMI and physical activity in the second model. Energy plus those factors mentioned in the second model were further adjusted in the third model. The first tertile of the dietary patterns scores was considered as the reference category in the logistic regression models.
Quantitative data were compared between case and control groups and across tertiles of dietary patterns using independent t-test, one-way ANOVA and the analysis of covariance (ANCOVA) with Bonferroni correction. The Chi-square test was also used for comparing categorical data between the case and control groups. Statistical analyses were performed using statistical package for social sciences (SPSS) software version 16.0 (SPSS Inc., Chicago, IL, USA). Also, P-values less than 0·05 was considered statistically significant.
Result
Major dietary patterns
The three major dietary patterns identified according to the factor loading are (Table 2): (i) ‘western dietary pattern’, which was highly loaded by fast foods, soft drinks, processed meats, salt, salty snacks, sugar-sweets-desserts, organ meat, broth, poultry-skin, hydrogenated fats, mayonnaise, high fat diary, vegetables oil, tea and red meat; (ii) ‘traditional dietary pattern’, which was high in eggs, tomato sauce, fruits, red meats, refined grains, vegetables, pickles, coffee, natural juices, mayonnaise, skinless poultry, vegetables oils, salty snacks, whole grains and high fat diary; (iii) ‘healthy dietary pattern’, which was high in dried fruits, condiments, nuts, canned fruits, legumes, vegetables, garlic, fruits, natural juices, fish, pickles and yogurt drink. In total, the identified dietary patterns explained 44·8 % of dietary food intakes in this population.
Table 2 Factor loadings matrix for dietary patterns identified by using factor-analysis

Loading factors less than 0·2 are not reported for simplicity.
General characteristics and dietary intake of participants
According to the exclusion criteria, 559 subjects (225 cases and 334 controls) remained for the current analysis. The general characteristics of the study population are shown in Table 3. Individuals in the case group were more likely to be overweight, single, and highly educated, compared with those in the control group. Also, they had greater waist-circumference and hip-circumference and less physical activity (P < 0·05).
Table 3 General characteristics of participants with and without premenstrual syndrome
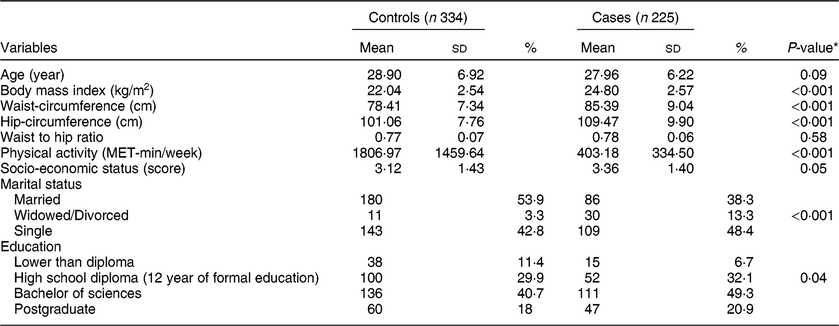
Values are presented as mean ± standard deviation (sd) for continuous and number (%) for categorical variables.
* Resulted from independent samples t-test for quantitative variables and chi-square test for categorical data.
The comparison of the demographic and lifestyle factors of the study population in different levels of the three major dietary patterns is summarized in Table 4. Distribution of participants in terms of age, weight, physical activity level, waist, hip, WHR, BMI, SES marital status and education was significantly different across western dietary pattern categories. In addition, there were significant differences in WHR, physical activity, marital status and education in the healthy dietary pattern category. Furthermore, across different levels of the traditional dietary pattern, participants were significantly different according to age, weight, waist, hip, WHR, BMI and marital status (P < 0·05). In comparison to those in the lowest tertile, individuals in the highest tertile of the western dietary pattern were younger, and had higher mean BMI, waist-circumference, hip-circumference and less physical activity. Comparison of food items in the healthy dietary pattern showed that individuals in the highest tertile were more likely to have physical activity and less WHR. Furthermore, individuals with the highest adherence to traditional dietary pattern were older, and had higher BMI, waist-circumference, hip-circumference and WHR (P < 0·05). Also, 81·4 % of participants with PMS and 18·6 % of healthy subjects were adherent to the western dietary pattern (P < 0·05).
Table 4 General characteristics of the study participants across tertiles of the major dietary patterns

Values are presented as mean ± standard deviation (sd) for continuous and number (%) for categorical variables.
* Result from Analysis of Variance for quantitative variables and chi-square test for categorical data.
Table 5 illustrates the comparison of macro and micro-nutrients intake in different categories of major dietary patterns. Greater adherence compared with lower adherence to western dietary pattern was significantly associated with higher intake of energy, fat, saturated fatty acid (SFA), MUFA, PUFA, linoleic fatty acid, linolenic fatty acid, oleic fatty acid and Vitamin E, and lower intake of iron, Calcium, Magnesium, Vitamin B1, Vitamin B6, Folate, Vitamin C, and Vitamin D. In addition, participants in the different tertiles of the healthy dietary pattern had significantly different intakes of energy, fat, MUFA, PUFA, linoleic fatty acid, linolenic fatty acid, oleic fatty acid, DHA, Iron, Calcium, Vitamin E, Vitamin B6, Vitamin C, and Vitamin D.
Table 5 Comparison of macro- and micro-nutrients intake across tertiles of major dietary patterns
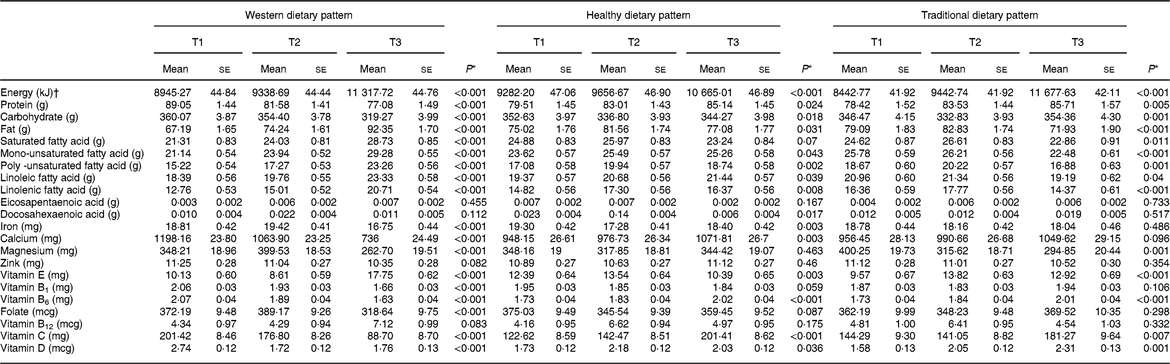
Values are reported as means ± standard errors (ses).
* P-values were obtained by using analysis of covariance; adjustment was made for age and energy intake.
† Adjusted for age.
A similar comparison of nutrients intake in the traditional dietary pattern category showed a prominent difference in terms of energy, fat, SFA, MUFA, PUFA, linoleic fatty acid, linolenic fatty acid, oleic fatty acid, Magnesium, Vitamin E, Vitamin B6, Vitamin C and Vitamin D intake.
The relationship between dietary patterns and PMS
Crude and multivariable adjusted odds ratio with 95 % CI are represented in Table 6. In all fitted models, the lowest categories of adherence to dietary patterns (tertile 1) was defined as the reference. Those in the top tertile of adherence to western dietary pattern had significantly, 1·77 times, more chance of developing PMS compared with those in the bottom tertile of the crude model (OR: 1·77; 95 % CI: 1·05, 2·93). After adjusting for potential important confounding variables, there was a positive significant relationship observed between the chance of PMS and adherence to western dietary pattern in the first model (OR: 2·39; 95 % CI: 1·34, 4·26), second model (OR: 1·92; 95 % CI: 1·03, 4·42), and after adjustment for all confounding variables (OR: 1·49; 95 % CI: 1·01, 3·52).
Table 6 Crude and multivariable adjusted odds ratios (95 % CI for OR) premenstrual syndrome across tertiles of dietary patterns

* Model 1: adjusted for age, marital status, education, and socio-economic status.
† Model 2: adjusted for age, marital status, education, socio-economic status, BMI and physical activity.
‡ Model 3: adjusted for age, marital status, education, socio-economic status, BMI, physical activity and energy intake.
On the contrary, individuals in the highest tertile of adherence to healthy dietary pattern were 43 % less likely to have odds of PMS compared with those in the lowest category in the crude model (OR: 0·43; 95 % CI: 0·31, 0·58). This association remained significant even after taking potential confounders into account in model 1 (OR: 0·54; 95 % CI: 0·34, 0·85), and model 3 (OR: 0·31; 95 % CI: 0·17, 0·72). But there was insignificant association in model 2 (OR: 0·59; 95 % CI: 0·29, 1·17).
Furthermore, a similar finding for traditional dietary pattern was shown in the crude model (OR: 2·11; 95 % CI: 1·37, 3·22). In model 1, after adjustment for different confounders, those in the top category had 2·25 times higher odds of PMS (OR: 2·25; 95 % CI: 1·43, 3·55). However, these effects were the reverse in model 2 (OR: 0·96; 95 % CI: 0·47, 1·97) and model 3 after adjustment for all confounding variables (OR: 0·33; 95 % CI: 0·14, 0·77).
Discussion
In the current study three major dietary patterns including western, healthy and traditional dietary habits were identified. Among the dietary patterns obtained, the western dietary pattern – which was characterized by high loading of fast foods, soft drink, processed meats, salt, salty snacks, sugar-sweets-desserts, organ meat, broth, poultry-skin, hydrogenated fats, mayonnaise, high fat diary, vegetables oil, tea and red meat – was significantly associated with a higher likelihood of PMS. In contrast, we found a significant association between a healthy dietary pattern high in dried fruits, condiments, nuts, canned fruits, legumes, vegetables, garlic, fruits, natural juices, fish, pickles and yogurt drink and PMS in the multi-variable adjusted models. In addition, individuals with highest adherence to the traditional dietary pattern high in eggs, tomato sauce, fruits, red meats, refined grains, vegetables, pickles, coffee, natural juices, mayonnaise, skinless poultry, vegetables oils, salty snacks, whole grains and high fat diary also had lower odds for PMS.
Determining dietary patterns allows holistic identification of diet and disease relations and provides further information beyond associations found by the analysis of individual foods and nutrients. To our knowledge, studies evaluating the association of dietary patterns and PMS risk are limited. Previous studies have mostly focused on the relationship between particular foods or nutrients and PMS. They have supported the positive relationship between unhealthy food items, which are highly loaded in the western dietary pattern, such as fast foods(Reference Isik, Ergol and Aynioglu18), and sweet foods and beverages and PMS(Reference Rossignol and Bonnlander19). In contrast, certain healthy food items such as fish might be inversely associated with PMS induced by athletic disturbance(Reference Takeda, Imoto and Nagasawa20). Likewise, specific nutrients such as omega-3 fatty acids have been positively related to the relief of psychiatric and somatic symptoms of PMS(Reference Sohrabi, Kashanian and Ghafoori21).
The results of the current study with 559 participants demonstrated that habitual dietary patterns might contribute to the risk of PMS, despite the complex and vague pathogenesis of PMS. Our findings are in concordance with the results obtained from a previous study(Reference Farasati, Siassi and Koohdani7), which found that the western dietary pattern was associated with an increased risk of PMS. However, it did not identify any significant relationship between either the healthy or traditional dietary patterns and PMS. The emerging evidence has found that higher adherence to the western dietary pattern is associated with inadequate nutrient intake; on the other hand, reports have revealed an association between risk of PMS and low level of nutrients(Reference Saeedian Kia, Amani and Cheraghian5,Reference Serra-Majem, Bes-Rastrollo and Roman-Vinas22) . The western dietary pattern is also positively related to higher level of inflammatory biomarkers, while healthy diets with high antioxidant content, vitamins and phytochemicals results in reduced inflammatory indices(Reference Barbaresko, Koch and Schulze23,Reference Bonaccio, Cerletti and Iacoviello24) . On the other hand, PMS symptoms have been positively associated with some inflammatory markers(Reference Bertone-Johnson, Ronnenberg and Houghton25). Therefore, the effect of western dietary pattern on the risk of PMS might be linked to the induction of inflammation. In addition, the imbalance of oxidant/antioxidant systems may be due to consumption of an unhealthy diet, which eventually enhances the symptoms of PMS(Reference Miller, Erlinger and Sacks26,Reference Duvan, Cumaoglu and Turhan27) . Moreover, adherence to unhealthy dietary patterns might have obesogenic results(Reference Jessri, Wolfinger and Lou28), which may contribute to the development of PMS symptoms(Reference Masho, Adera and South-Paul29).
As mentioned before, the earlier study that evaluated the relationship between dietary patterns and PMS did not show any relationship between both the healthy or traditional dietary patterns and PMS(Reference Farasati, Siassi and Koohdani7). Participants in the top tertile of the healthy eating plan were 57 % and 46 % less likely to have odds of PMS compared with those in the bottom tertile in the crude and first model, respectively. Interestingly, such associations remained significant in the third model. This finding highlights the beneficial role of healthy food items associated with PMS symptoms.
In the current study, protective association of traditional dietary pattern against PMS was also observed. Although the traditional dietary pattern was shown to be a risk factor for PMS in the crude model, this effect was reversed in the final adjusted model. Therefore, it seems that adjusted factors have a higher contribution to the risk of PMS. Taken together, despite a few unhealthy food items such as red meat, the traditional dietary pattern could be considered as a healthy diet for PMS development.
The current study has several strengths. Although studies examining the association between dietary patterns and PMS are rare, the current study is one of the comprehensive studies with a large number of participants in this field. Second, subjects in this study were selected from individuals referring to healthcare centres, with different socio-economic status. Thus, variability in women’s dietary intakes could cover a wide range of eating habits. In addition, we were able to consider a wide range of confounders for adjustment to reach an independent association in the current study.
There are a number of limitations that should be taken into account. Like all case-control studies, the inherent methodological limitations of such studies make it impossible to draw a causal link between dietary patterns and the risk of PMS. Although case-control studies are efficient in terms of studying the association between diet and disease, the selection and recall biases of such study design must be kept in mind. An additional concern is that women diagnosed with PMS change their diet choices, and this might change the risk estimates. Furthermore, although we assessed energy-adjusted intake of all food groups for extracting dietary pattern scores, the possibility of subject misclassification is unavoidable when using FFQ to assess the long-term dietary intakes.
In conclusion, our results indicated that the western dietary pattern – which includes fast foods, soft drink, processed meats, salt, salty snacks, sugar-sweets-desserts, organ meat, broth, poultry-skin, hydrogenated fats, mayonnaise, high fat diary, vegetables oil, tea and red meat – might be associated with increased odds of PMS. In addition, a protective association of both healthy and traditional dietary pattern against PMS was observed. This study provides important insights into the association between diet and the risk of PMS, and shows that changing diet quality and avoiding unhealthy diets might have desirable effects in reducing the risk of PMS symptoms. However, further studies are needed to confirm these associations and provide the evidence needed to translate these findings into clinical practice.
Acknowledgements
The authors would like to offer their gratitude all the women who kindly participated in this study. Financial support: This research did not receive any specific grant from funding agencies in the public, commercial, or not-for-profit sectors. Conflict of interest: The authors declare no personal or financial conflicts of interest. Authorship: B.M.F., R.G., F.S. and A.F. made substantial contributions to the conception, design, acquisition of data, analysis and interpretation of data and drafting of this study. B.M.F., A.F. and Z.S. participated in drafting the article or revising it. R.G. and M.P. gave final approval of the version to be submitted and any revised version. R.G. is guarantor of this work. Ethics of human subject participation: This study was conducted according to the guidelines laid down in the Declaration of Helsinki, and all procedures involving human subjects/patients were approved by the ethics committee of Isfahan University of medical sciences (Ethical approval number: IR.MUI.REC.1395.3.518). Written informed consent was obtained from all subjects/patients.