The exponential rise of social media, where users create online communities to share information for social networking and microblogging including Twitter, Facebook, YouTube, Instagram and Reddit(1), presents an unprecedented opportunity for nutrition research. As of January 2020, there were 3·88 billion worldwide social media users, growing more than 9 % since 2019(Reference Kemp2), with sharing food and eating behaviours one of the most popular online community activities(Reference Lewis and Phillipov3,Reference Lewis4) . Social listening is recognised as an emerging type of communication monitoring as a means of attaining interpersonal information and social intelligence(Reference Stewart and Arnold5), and when applied to social media, it can be further analysed using social media analytics (SMA) to uncover how people behave and talk in relation to food, nutrition and health, thus, providing a future solution or complement to traditional nutrition research methods augmenting the investigation or tracking of trends in changing dietary behaviours.
SMA can provide economies of scale, and most importantly, in real time, surveillance models(Reference Stieglitz, Mirbabaie and Ross6) compared with traditional research methods such as surveys. SMA is an interdisciplinary research field that aims to extend methods of analysis of social media data(Reference Stieglitz, Mirbabaie and Ross6). SMA has grown exponentially in recent years and is well established in the research domains of information systems, business and consumer marketing, political science, crisis identification and communication(Reference Stieglitz, Mirbabaie and Ross6). The popularity of SMA came about because of social media big data, that is characterised by the four Vs of volume, velocity, variety and veracity. The rich data that can be collected at scale and quickly have enabled new SMA use cases. Common applications of SMA are in opinion mining (automatic systems to determine human opinion from text written in natural language), sentiment analysis (using natural language processing (NLP), computational linguistics and text analytics to identify subjective information) and content analytics (often of text and the study of word frequency, distributions, pattern recognition, and visualisation)(Reference Batrinca and Treleaven7). Predictive analytics is often described as the pinnacle of SMA and uses data mining of historical and current social media data, machine learning plus statistical techniques, to predict future or other unknown events or behaviours(Reference Hernandez and Zhang8).
As SMA has evolved, so have developed frameworks including defined steps across data discovery, collection, preparation and analysis(Reference Stieglitz, Mirbabaie and Ross6). SMA often uses established tools across these steps which include in-platform analytic tools (e.g. Application Programming Interface (API)), open source tools (e.g. SentiStrength, NodeXL) which generally require little programming experience, commercial tools (e.g. Radian 6, SAS, IBM Watson Analytics(Reference Hoyt, Snider and Thompson9)) obtained through subscription and often requiring an experienced data analyst or team, and the design of customised machine learning systems and dashboarding requiring far more sophisticated programming capabilities(Reference Gohil, Vuik and Darzi10).
In the field of nutrition research, there is evidence of the use of social media platforms; however, there does not appear to be an established track record of SMA related to the investigation or tracking of dietary behaviours with general population health. Nutrition and health researchers have used social media platforms for recruitment of study participants(Reference Wasilewski, Stinson and Webster11–Reference Arigo, Pagoto and Carter-Harris13) and as part of intervention studies(Reference Loh, Schwendler and Trude14,Reference Jane, Foster and Hagger15) . There is also evidence that nutrition researchers have utilised social media platforms for gathering primary research data; however, in some studies, the researchers have used manual data collection, preparation and analysis methods (such as this example with Instagram posts(Reference Baker and Walsh16)) in comparison with the use of sophisticated machine learning, artificial intelligence and customised programming that define the steps of SMA. In other cases, nutrition and health researchers have relied on traditional qualitative methods such as focus groups (as in this study examining the use of Instagram for healthy eating(Reference Chung, Agapie and Schroeder17)), interviews or surveys(Reference Lyons, Goodwin and McCreanor18) to understand social media user content via self-reporting, as opposed to direct data extraction and analysis with SMA. One example of the use of SMA tools is NLP techniques explored with Reddit posts from an online eating disorder community, and the top techniques, compared with coding by clinical psychologists, were found to have an error rate of only 4 % when assessing if a person required immediate professional help(Reference Yan, Fitzsimmons-Craft and Goodman19). A second example using predictive SMA is the customised dashboard nEmesis machine learning system. This system was designed to automatically detect food outlets that pose a food safety risk, by following any adverse reactions self-reported via Twitter of geotagged diners, and had a 64 % greater effectiveness than traditional methods(Reference Sadilek, Kautz and Di Prete20).
In contrast, in the field of health research, there is evidence from systematic reviews of the use of both SMA applications along with evidence of SMA in investigating general population health behaviours. In a recent review of the popular microblogging site Twitter in health research, SMA was commonly used in cross-sectional content analysis (56 %) and also in longitudinal surveillance (26 %), and to a lesser extent with participant recruitment (7 %) or intervention (7 %)(Reference Sinnenberg, Buttenheim and Padrez21). In this review, the research fields represented by studies were public health (23 %), infectious diseases (20 %) behavioural medicine (18 %) and psychiatry (11 %), with the most common research topics being influenza (8 %), smoking (7 %), cancer (5 %) and Ebola (4 %). A second, recent scoping review on the use of Twitter for data collection with health care consumers concluded that the platform is utilised to search and mine primary research data(Reference Zhang, Albrecht and Scott22). In this review, a wide range of health topics and research questions were explored including health challenges such as pain, migraines and cancer; social discourse of conditions like perceptions or portrayal of seizures; and cyberspace in comparison with real-world phenomena, with data obtained via posts (such as keywords or phrases) or profiles (such as geolocation). Established SMA applications in health behaviours research include as a complementary data source for pharmacovigilance(Reference Lardon, Bellet and Aboukhamis23,Reference Tricco, Zarin and Lillie24) , in the monitoring of changing health habits such as smoking(Reference Krauss, Sowles and Moreno25–Reference Ben Taleb, Laestadius and Asfar30), in public health surveillance such as predicting flu outbreak(Reference Alkouz, Al Aghbari and Abawajy31,Reference Zadeh, Zolbanin and Sharda32) , with sentiment and content analysis such as in distinct, online communities(Reference Sutton, Vos and Olson33,Reference Teoh, Shaikh and Vogel34) and as a predictor of morbidity and psychosocial health such as in suicide risk(Reference Seabrook, Kern and Fulcher35–Reference Thorstad and Wolff39). These findings in health research provide promising support for the application of SMA in nutrition research, with the investigation or tracking trends in dietary behaviours in general population health.
Social media analytics potential with investigating dietary behaviours
To our knowledge, there has not been a literature review which has explored the potential use of SMA in nutrition research with the investigation or trend tracking of dietary behaviours. SMA can provide economies of scale, and most importantly, in real time, surveillance models and is well developed in other fields that indicate promise for nutrition research(Reference Stieglitz, Mirbabaie and Ross6). Conversely, SMA also has known limitations which must be further investigated for applications to the field of nutrition research. For example, sentiment analysis can fail to accurately identify semantics and pragmatics (such as irony and slang) which are commonly used in personal conversations to describe eating behaviours(Reference Hussein40). Finally, as SMA is a fast moving, new area of research, it also presents challenges for health and nutrition researchers which warrant further investigation, such as different skill sets required to plan a study, to derive and analyse data and to conduct a study under existing ethical standards for medical research with human subjects(41).
Given the established track record of SMA with health research and the limited use in nutrition research, the aim of this rapid review is to understand how SMA is currently being used with the investigation or the trend tracking of dietary behaviours in general population health. It behoves us to investigate the studies, platforms, applications, nutrition topics, research disciplines and ethical considerations to map current opportunities and inform future research.
Due to the broad area of investigation, likely heterogenous nature of the study methods, focus on current research due to the rapidly changing landscape of SMA and the aim to draw together timely evidence, a rapid review was the appropriate approach for this knowledge synthesis(Reference Pang42). The aim of this paper was to investigate: How are researchers currently using SMA for investigating dietary behaviours related to general population health?
The key considerations of the rapid review were to:
1. Extract and collate studies that involve dietary behaviours in general population health using SMA on public domain, social media data.
2. Rank the social media platforms by data set size and by number of included studies to assess the scope of use and common research platforms.
3. Collate the included studies by nutrition topics to assess common categories of relevance to public health nutrition.
4. Map each study design for an overview of the scope of SMA applications across the recognised steps of data discovery, collection, preparation and analysis.
5. Explore the types of research disciplines involved and the presence of collaborative research with particular focus on nutrition or health discipline involvement.
6. Record the presence of formal human ethics review or considerations.
Methods
Protocol
The protocol was drafted using guidance in the WHO Rapid Reviews to Strengthen Health Policy and Systems: A Practical Guide(43) and the National Collaborating Centre for Methods and Tools Rapid Review Guidebook: Steps for Conducting a Rapid Review(Reference Dobbins44). An overview of the rapid review approach was recorded with the Open Science Framework on 27 October 2019 and updated on 9 March 2020 and 9 December 2020(Reference Stirling45) with further information on the protocol available via the authors.
Eligibility criteria
Original, full-text research studies published in peer-reviewed journals from January 2014 to February 2020 in the English language were included for review. Due to rapid changes in social media platforms and functions, along with equally rapidly changing technology driving SMA, the authors limited this review to studies published in the prior 5–6 year period in order to obtain data most reflective of current usage. Table 1 outlines the full inclusion and exclusion criteria. Studies were included if they involved SMA investigating individual dietary behaviours related to general population health and not with acute or chronic diseases/conditions or with advertising, campaigns or policy. Nutrition was not required to be the primary focus of studies, and broad fields of research were considered. For the purposes of this review, social media was defined as third-party sites such as Twitter, Facebook, Instagram, Reddit and YouTube and not health-targeted apps, Internet of Things (e.g. wearables) or health-targeted blogs and websites as they often require subscription services with user-generated content not in the public domain or are subject to copyright or privacy policy of the site ownership. Unlike a website or blog hosted and managed by an individual, company or organisation, social media platforms enable users to create online communities to share information, ideas, personal messages and other content (such as videos) typically in the public domain and often available for extraction and SMA often via an API(1). The final eligibility criteria were independently assessed by two authors (ES and JW) who are nutrition scientists and accredited dietitians.
Table 1 Eligibility criteria for the present rapid review of social media analytics in nutrition research, current usage in investigation of dietary behaviours

IOT, Internet of Things.
Information sources and search strategy
One researcher (ES) conducted the systematic search using the keywords ‘social media’ in combination with ‘data analytics’ and ‘food’ or ‘nutrition’ (1 January 2014 until 29 February 2020). A full list of search term keyword synonyms, along with the search strategy across all databases, is displayed in the Supplementary Materials. Social media and data analytics search terms were verified with a research librarian through formative searching and confirmed with data analytics expert author (KL) and also cross-referenced with keywords identified by Taylor et al. (Reference Taylor and Pagliari46). Specific keywords were included for the major platforms such as Facebook, Twitter and YouTube as initial scoping had shown evidence of use in SMA and additional platforms were identified through generic social media keywords. Nutrition-related search terms were purposely kept broad in order to capture studies from a diverse range of research fields that may contain pertinent information on dietary behaviours of interest to public health nutrition. The first subset of nutrition keywords included synonyms for nutrition, diet and health. This subset also contained a targeted list of non-communicable diseases such as ‘diabetes’ and ‘cancer’ to assess if studies on populations with acute or chronic conditions, although not the focus here, contained relevant information on general population health dietary behaviours. The second subset of nutrition keywords were related to general food or eating behaviours such as ‘snack’, ‘takeaway’ and ‘supermarket’ in order to capture studies outside the fields of health, such as hospitality, travel or marketing, that also contained relevant information to this investigation. Searched bibliographic databases included Medline, CINAHL, Scopus, ACM Digital Library and Engineering Village, and all articles were exported into EndNote. The reference lists of all included articles were also hand-searched to capture related texts.
Selection of sources of evidence
The primary author (ES) undertook a first abstract and title assessment to exclude duplicate and irrelevant articles. Full-text articles were then retrieved and screened against the inclusion criteria by two authors who are nutrition scientists and accredited dietitians (ES and JW).
Data charting, data items and synthesis
Data were extracted from eligible studies by ES using data-charting forms jointly developed by three authors (ES, AF and JW), and key study characteristics were discussed by the authors. Data analytics expert author (KL) provided advice on SMA methods as required.
Extracted data items included year, country of origin, social media platform(s), data set size(s), purpose of the study and where available, information on the SMA steps across data discovery, preparation, collection and analysis. The aim of this information was to explore the scope of, and most popular, social media platforms utilised in research by extracting information on the number of studies per platform and the data set size. Secondly, extracting the purpose of the study allowed for collation of the range of nutrition topics under current investigation and alignment with public health nutrition. Thirdly, information on the SMA steps would reveal common applications such as if there was a heavy reliance on SMA for data discovery (such as searching social media data), but less so in advanced analytic applications (such as sophisticated machine learning models and not human coding). Due to the heterogenous nature of the research investigations, diverse research fields and study designs, in-depth comparison of SMA techniques across studies was not warranted, rather the focus was on identifying any common trends across the SMA steps.
Sinnenberg et al. have provided a recent taxonomy to describe the roles of Twitter in health research in order to assign each study to recognised categories in the field of SMA of either content analysis, surveillance (monitoring trends in a particular topic or metric over time), engagement (user interactions with content produced by other users) or network analysis (the connections between users or influencers)(Reference Sinnenberg, Buttenheim and Padrez21). This taxonomy was applied to the included studies in this review, across all social media platforms, given it provided a timely and up-to-date framework that covered appropriate categories for the current field of SMA as confirmed with data analytics expert author (KL). The two additional Sinnenberg categories of recruitment and intervention were outside the scope of this review(Reference Sinnenberg, Buttenheim and Padrez21).
Additional data items were collected on the affiliations or disciplines listed of all study researchers with the aim to explore if current research is driven by particular disciplines or collaborative research. Finally, the presence of institutional ethics board review or ethical mentions was recorded for all included studies to assess ethical considerations, and with the aim to explore any discipline differences in ethical approaches. The final versions of the data-charting forms are displayed in the Supplementary Materials.
Included sources of evidence were not critically appraised in this rapid review as the aim was to capture a broad range of heterogeneous studies to inform a summary of current topics, platforms and other information and not evaluate specific effects on a particular area of public health nutrition.
Results
Selection of sources of evidence
On completion, the original database searches yielded 5220 results (Fig. 1). Duplicate records were removed resulting in 3175 studies. Abstract and title searching was conducted to remove irrelevant articles against eligibility criteria and resulted in 60 retained for full-text review. An additional 10 records were identified through hand-searching reference lists. A total of 70 full-text articles were screened for inclusion in the final review and subsequently 34 of these met the inclusion criteria. Four of the included studies involved extracting posts of user reviews from platforms with major e-commerce or product and service info sharing features (Amazon, Yelp, Weibo and Koubei), which were deemed to meet the criteria of user-generated social media in publicly available data on the Internet. Excluded studies were due to the following reasons: focus was on individuals with acute or chronic conditions and there was a lack of relevant information to general population health dietary behaviours (n 21), studies involved tracking or investigating advertising, campaigns or policy in relation to health and nutrition and not individual dietary behaviours (n 10), in two studies researchers obtained consent and access to the social media accounts of individual participants rather than extracting public domain data, on further review two additional studies were found to have no direct relation to nutrition and one study involved a dedicated health website that was not part of the definition of social media sites in this review.

Fig. 1 Flowchart showing the search for studies included in the rapid review on Social Media Analytics in Nutrition Research: a rapid review of current usage in investigation of dietary behaviours
Synthesis of results
Data characteristics of social media analytics studies involving dietary behaviours
The data characteristics of 34 research articles are described in Table 2, including the social media platform(s), data set size(s), study purpose, Sinnenberg’s Taxonomy and SMA steps. Each study was mapped for the usage of SMA steps across data discovery, collection, preparation and analysis and common tools or processes captured if clearly determinable. The full data-charting and additional details are available in the Supplementary Materials.
Table 2 Characteristics of included studies ranked by original data set size per platform in present rapid review

SMA, social media analytics; API, Application Programming Interface; MALLET, Machine Learning for LanguagE Toolkit; LIWC, Linguistic Inquiry and Word Count; NLP, natural language processing; LDA, linear discriminant analysis; LLDA, local linear discriminant analysis; SVM, support vector machine; WEKA, waikato environment for knowledge analysis.
* SMA key:
✓ SMA was described for the relevant step.
X Manual extraction, coding or analysis by humans was described for the relevant step.
✓/x A combination of SMA and manual processes were described for the relevant step.
? The details could not be clearly identified for the relevant step from the paper. Authors were not contacted for additional information.
Social media platforms and data set sizes
Table 3 displays the ranking of social media platforms accessed in included studies with a description of each platform.
Table 3 Social media platforms in included studies in present rapid review

IOT, Internet of Things.
Of the 34 studies, the greatest number utilised Twitter (62 %, n 21), followed by Instagram (21 %, n 7). Facebook (9 %, n 3) and Foursquare (9 %, n 3) had equal next ranking. Amazon.com user product reviews, YouTube, Yelp reviews and Tumblr were used in single studies at times in combination with another platform(s) under investigation. Two studies utilised social media platforms in China, Weibo and Koubei. The majority of studies (88 %, n 30) utilised only one type of social media platform in the research investigation with only four studies (12 %, n 4) using two or more platforms.
The majority of the studies originated in the USA (62 %, n 21) with the remainder across a range of countries and collaborations including UK, Australia, China, Brazil, Uruguay, Bangladesh, Qatar and New Zealand.
Data set sizes ranged from 4 to 80M individual data points of social media content. Twitter studies had the largest data set sizes with 9 studies (26 %, n 9 of total included studies) having data sets of at least 10M tweets. Data sets in the millions were also seen in the Instagram, Weibo and FourSquare studies.
Nutrition topics of relevance to public health nutrition
Included studies were categorised by nutrition topics of relevance to public health nutrition and displayed in Table 4.
Table 4 Categorisation of included studies by nutrition topics of relevance to public health nutrition in present rapid review
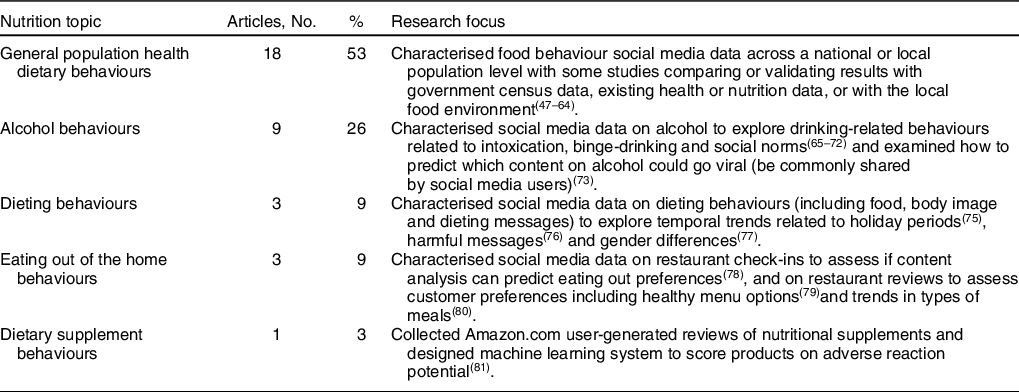
The majority of studies (53 %, n 18)(Reference Shah, Srivastava and Savage47–Reference Nguyen, Meng and Li64) were cross-sectional in design and were categorised as investigations into general population health level, dietary behaviours as part of using social media with the aim to define demographic characteristics or sentiment. Studies in this category ranged from being exploratory in nature to test innovative, machine learning models, through to national population health-level dietary behaviour investigations validated against existing health data or other independent data such as location of fast-food outlets. The most promising studies were able to demonstrate a correlation between the location of high-energetic food mentions on Twitter and independent, government tracked, obesity consumption data(Reference Shah, Srivastava and Savage47) or validated machine learning as a tool for constructing indicators of food intakes compared with government census data(Reference Nguyen, Brunisholz and Yu52). Over two-thirds of studies in this category used Twitter (38 %, n 13) as the social media platform and source of data via tweets, and Instagram (12 %, n 4) and Foursquare (6 %, n 2) also utilised in more than one study.
The next largest category of studies involved insights into dietary behaviours with alcohol consumption (24 %, n 8) related to intoxication, binge-drinking and social norms(Reference Cavazos-Rehg, Krauss and Sowles65–Reference Phan, Muralidhar and Gatica-Perez72). An additional study in this category examined how to predict virality of content promoting drinking(Reference Alhabash, VanDam and Tan73). A post that ‘goes viral’ means one that becomes very popular on a social media platform in a short time period (hours or days), due to it being shared by a significant number of users, often in the millions, which increases the reach and amplitude(Reference Himelboim and Golan74).
The next category identified was dieting behaviours (13 %, n 3) (including food, body image, weight loss and dieting messages) to explore a range of investigations relevant to public health nutrition. These included detecting temporal trends such as patterns of weight loss dieting messages related to periods when weight gain is common, during or after Christmas or in particular seasons such as winter(Reference Turner-McGrievy and Beets75). Blackstone et al. explored posts in two Facebook groups that were dedicated food and exercise programmes led by the fitness industry advertised to ‘jump start’ a healthy lifestyle and found 88·6 % of content promoted harmful messages about dieting restraint, body image and losing body fat/weight(Reference Blackstone and Herrmann76). Finally, a study focused on gender differences and stereotypes in social media using the hashtag #fitspo (fit inspiration) also revealed that 19·6 % of posts were thematically linked to food or dietary behaviours(Reference Carrotte, Prichard and Lim77).
A further category characterised social media data on eating out of the home behaviours via studies looking at a range of investigations with restaurant check-ins or reviews. Rahman et al. correlated the analysis of the psycholinguistic content of tweets, the common words written by the user, with Foursquare restaurant check-ins to predict eating out preferences(Reference Rahman, Majumder and Mukta78). In this study, it was revealed that people who frequently use the words ‘health’ are more likely to visit mid-priced restaurants (as opposed to expensive, e.g. indulgent, fine dining or cheaper, e.g. fast food). The two studies on Chinese social media platforms Koubei and Weibo used online restaurant reviews to provide insights into dietary behaviours when eating out, whether healthy options were important to consumers and changing cuisine trends, respectively(Reference Yan, Wang and Chau79,Reference Zhou and Zhang80) .
The single study on Amazon.com designed a surveillance system to monitor dietary behaviours with and flag adverse reactions to dietary supplements via user reviews, as an innovative alternative to traditional methods of therapeutic goods reporting(Reference Sullivan, Sarker and O’Connor81).
Taxonomy
As displayed in Table 2, applying Sinneberg’s Taxonomy(Reference Sinnenberg, Buttenheim and Padrez21) all of the studies included in this review performed some type of content analysis (100 %, n 34), followed by a smaller category also performing or planning for surveillance (12 %, n 4)(Reference Sun, Wang and Li51,Reference Turner-McGrievy and Beets75,Reference Zhou and Zhang80,Reference Sullivan, Sarker and O’Connor81) . One study also performed analysis of social media engagement(Reference Alhabash, VanDam and Tan73). There were no included studies that performed network analysis.
Social media analytics methods
Studies involving SMA methods clearly observed across all steps of data discovery, collection, preparation and analysis were prominent in Twitter (38 %, n 13)(Reference Shah, Srivastava and Savage47,Reference Nguyen, Li and Meng49–Reference Widener and Li53,Reference Karami, Dahl and Turner-McGrievy55,Reference Abbar, Mejova and Weber57,Reference Fried, Surdeanu and Kobourov58,Reference Nguyen, Meng and Li64,Reference Huang, Elghafari and Relia69,Reference Kershaw, Rowe and Stacey70,Reference Alhabash, VanDam and Tan73) with the largest data set at 80M tweets. Two large-scale studies designed sophisticated, customised machine learning systems with the ‘Lexicocalorimeter’ attempting to measure energy content of food tweets(Reference Alajajian, Williams and Reagan50), and Shah et al. designed a machine learning classifier to assess the content of food-related tweets across the whole of Canada with demonstrated correlation with government consumption data(Reference Shah, Srivastava and Savage47). In contrast, a number of studies (approximately 18 %, n 6)(Reference Vydiswaran, Romero and Zhao48,Reference Vidal, Ares and Machín54,Reference Chen and Yang63,Reference Wombacher, Reno and Veil66,Reference Primack, Colditz and Pang68,Reference Carrotte, Prichard and Lim77) across several platforms collected data sets using SMA for data discovery, collection and (often) preparation but relied on manual processes across the steps of data analysis. In these studies, data analyses typically involved human, manual coding, often using a code book designed for the study, and resulted in a much smaller subset of social media data making up the investigation.
The common use of in-platform search tools, along with third-party search tools (such as tagboard.com, Topsy and hashtagify.me), was observed in the data discovery phase to find data with relevant topics, hashtags or key words. In the data collection step, the use of a platform API to mine data such as the Twitter API, Foursquare API, Instagram API and Yelp API was common. In the data preparation step, the use of Stanford Tokenizer was named in two studies, a software that divides text into a sequence of tokens, which roughly correspond to words(82). Across the data analysis step, four studies mentioned the use of MALLET (Machine Learning for LanguagE Toolkit) which is a Java-based package for statistical NLP, clustering, topic modelling, information extraction and other machine learning applications to text(83). In addition, four studies included the use of LIWC (Linguistic Inquiry and Word Count) in data analysis which is a commercial software program used in NLP(84).
Interdisciplinary collaborations
The types of disciplines by the author listed affiliations or disciplines on the publication were grouped into broad categories by health/medicine-related disciplines and non-health-related disciplines including business/computer science and are presented in Table 5.
Table 5 Extracted information on disciplinary scope as listed on publication* of included studies in present rapid review
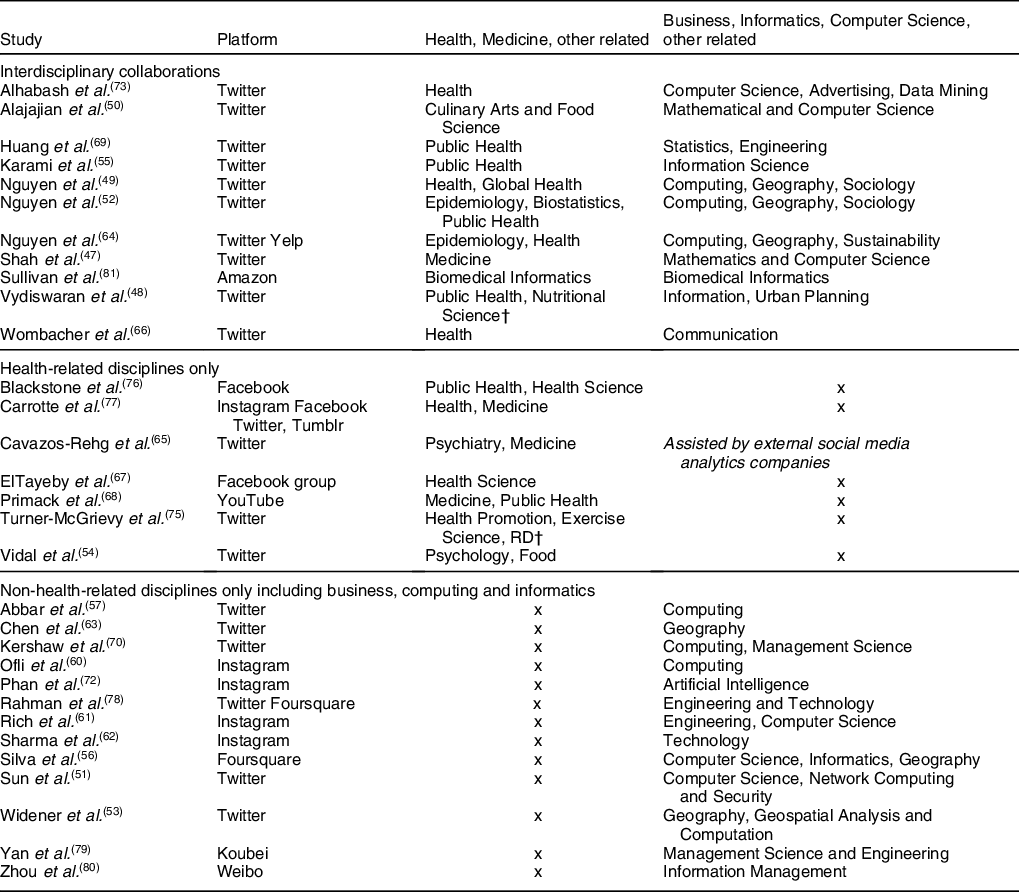
* Studies where author affiliation not clearly identifiable: Fried et al. (Reference Fried, Surdeanu and Kobourov58), Mejova et al. (Reference Mejova, Haddadi and Noulas59), Pang et al. (Reference Pang, Baretto and Kautz71)
† Presence of nutrition or dietetic discipline involvement. RD is the US credential for Registered Dietitian.
Approximately, a third of the studies with available affiliations (32 %, n 11) involved interdisciplinary collaborations between health/medicine or related disciplines, and business/computer science or related disciplines. Over an additional third (38 %, n 13) involved business/computer science or related disciplines only. Under a quarter of studies involved health/medicine disciplines (21 %, n 7) only.
Specific nutrition or dietetics discipline involvement was identified in only two of the studies, with one involving only health/medicine disciplines across health promotion, exercise science and nutrition(Reference Turner-McGrievy and Beets75) and the other a broader interdisciplinary collaboration between public health, nutrition science, urban planning and information disciplines(Reference Vydiswaran, Romero and Zhao48).
Ethics status
Table 6 presents information extracted on the ethics status of included studies. Nearly, three-quarters of the studies (74 %, n 25) did not mention ethical considerations or approvals. Less than a quarter of studies (15 %, n 5) obtained formal human ethics approval(Reference Nguyen, Brunisholz and Yu52,Reference Nguyen, Meng and Li64,Reference Blackstone and Herrmann76,Reference Zhang, Hall and Bastola85) or exemption(Reference Cavazos-Rehg, Krauss and Sowles65) by a relevant institutional review board. In the absence of ethical review process, only four studies (12 %) mentioned ethical considerations(Reference Cavazos-Rehg, Krauss and Sowles65–Reference ElTayeby, Eaglin and Abdullah67,Reference Turner-McGrievy and Beets75) including anonymising data and that social media data were in the public domain.
Table 6 Extracted information on the ethics status of included studies in present rapid review

IRB, institutional review board.
The available data on the type of research disciplines (Table 5) were compared with that of available data in the presence of ethics approval or considerations (Table 6). All studies that obtained formal ethics approval(Reference Nguyen, Brunisholz and Yu52,Reference Nguyen, Meng and Li64,Reference Blackstone and Herrmann76,Reference Zhang, Hall and Bastola85) or exemption(Reference Cavazos-Rehg, Krauss and Sowles65) involved a health-related discipline. Of the twenty-five studies with no mention of ethical approval or considerations, over half (54 %, n 13)(Reference Sun, Wang and Li51,Reference Widener and Li53,Reference Silva, Melo and Almeida56,Reference Abbar, Mejova and Weber57,Reference Ofli, Aytar and Weber60–Reference Chen and Yang63,Reference Kershaw, Rowe and Stacey70,Reference Phan, Muralidhar and Gatica-Perez72,Reference Rahman, Majumder and Mukta78–Reference Zhou and Zhang80) were conducted by business/computing disciplines only, with no health-related discipline involvement. The remaining studies without ethics approval or mentions showed mixed results with involvement of health disciplines only (13 %, n 3)(Reference Vidal, Ares and Machín54,Reference Primack, Colditz and Pang68,Reference Carrotte, Prichard and Lim77) and interdisciplinary collaborations with the presence of health disciplines (25 %, n 6)(Reference Shah, Srivastava and Savage47,Reference Nguyen, Li and Meng49,Reference Alajajian, Williams and Reagan50,Reference Huang, Elghafari and Relia69,Reference Alhabash, VanDam and Tan73,Reference Sullivan, Sarker and O’Connor81) .
Discussion
Principal results
This was the first ever rapid review, to the authors’ knowledge, that involved SMA in nutrition research, particularly in the investigation of dietary behaviours. The review identified thirty-four studies involving general population health using SMA on public domain, social media data between 2014 and 2020. Nutrition topics were segmented into the main category of broad population nutrition health investigations with subcategories seen in alcohol consumption behaviours, along with minor categories in dieting behaviours and eating out of the home. It was identified that all studies involved content analysis with some evidence of surveillance and limited evidence of engagement when applying Sinnenberg’s Taxonomy to describe the roles of social media in health research. Moreover, Twitter was found to be the predominant social media platform under investigation with large data set sizes into the tens of millions. Across all platforms, the use of SMA tools were observed in the steps of data discovery, collection and preparation, but to a lesser extent in data analysis. Approximately, a third of the studies involved interdisciplinary collaborations between health/medicine and business/computer science or related disciplines, and only two studies involved nutrition or dietetic discipline involvement. Less than a quarter of studies obtained formal human ethics approval or exemption by a relevant institutional review board and these all were lead or included health-related discipline research team members. These findings reveal existing SMA topics and platforms of relevance to nutrition researchers along with important considerations to inform future collaborative research.
Implications for future nutrition research
A key strength of this rapid review is being the first of a kind knowledge synthesis on SMA in nutrition research, particularly with the investigation of dietary behaviours. For nutrition researchers, it reveals existing social media platforms and categories of nutrition topics to inform further investigations and collaborative research, along with important considerations with ethical standards and technology.
Despite demonstrated capabilities to rapidly collect and analyse millions of individual social media posts and perform social listening or surveillance, this review supports further technological innovation before SMA will augment traditional research methods with investigating or tracking dietary behaviours. However, SMA shows promise for investigating dietary behaviours at a broad population census level and as a tool for mixed methods research. For example, in mixed methods research, SMA could be beneficial as a scoping or scanning tool to investigate dietary behaviours to inform surveys, focus groups or other participant investigations or interventions. SMA could also form an important triangulation instrument(Reference Noble and Heale86) in conjunction with multiple sources of data (such as interviews, focus groups or other sources) to increase the credibility and validity of research findings. One of the most promising studies, using new approaches to NLP, was able to demonstrate a correlation between the location of high-energetic food mentions on Twitter and independent, government-tracked, obesity consumption data(Reference Shah, Srivastava and Savage47). According to Shah et al., their study showed that social media analysis on a large scale, with the use of NLP, can help identify food- and activity-related tweets and is readily available as a close representation of real time(Reference Shah, Srivastava and Savage47). In addition, Nguyen et al. was also able to demonstrate machine learning as a validated tool for constructing indicators of food intakes across local government areas compared with census data(Reference Nguyen, Brunisholz and Yu52). However, in both studies, the approaches were unable to provide in-depth, quantitative data on food or nutrient intakes on par with that obtained from surveys. Technological innovation is required before SMA could be considered as a novel source to augment major public health nutrition surveillance tools like national nutrition surveys. Innovation will likely be needed across all steps of SMA such as being able to more accurately extract posts from individuals and filter those of businesses or brands in data collection, right through to more sophisticated machine learning models in the analysis steps, such as determining dietary behaviours from posts with slang, text abbreviations or colloquial language.
Collaborative and interdisciplinary research was explored in this review as it is often a requirement of research funders and vital in the modern era with the study of complex phenomena(Reference Nyström, Karltun and Keller87). Complementing the capabilities of an expert data analytics team with experienced health or nutrition researchers could enable large data sets to be processed and produce promising insights and outcomes for public health nutrition. Due to the small number of included studies and top line assessment of disciplinary involvement (via assessment of author listed affiliation on each publication), it is not possible to draw conclusions from this rapid review; however, relevant examples were observed in studies with and without health discipline involvement that warrant further investigation. In the multidisciplinary team of Vydiswaran et al. (Reference Vydiswaran, Romero and Zhao48), which included nutrition expertise, Twitter was demonstrated at being able to characterise neighbourhood-level food-related behaviours and attitudes/sentiment in food-related tweets. The researchers concluded that social media data can provide a reliable signal for dietary patterns and food-related attitudes at the census level, despite the noisy nature of user-generated text data, the limited fraction of geolocated tweets and access only to public discussions rather than actual dietary patterns. In contrast, in an example of computer science researchers working in isolation of nutrition-health researchers, there was an emphasis on demonstrating technology processes and at times lack of in-depth questioning or judgements on human dietary behaviours under investigation(Reference Widener and Li53). In this study by Widener et al., researchers from the fields of geography and computation compiled their own narrow list of ‘healthy’ and ‘unhealthy foods’ to inform coding rather than accessing existing, comprehensive, validated dietary assessment instruments(Reference Widener and Li53). In contrast, as another example, in Vidal et al. study, health researchers working in isolation of computer informatics expertise relied on manual content analysis which resulted in a much smaller subset being coded or collated as findings and not the larger data set extracted(Reference Vidal, Ares and Machín54). It is also warranted to point out that specific nutrition or dietetics discipline involvement was identified in only two of the studies(Reference Vydiswaran, Romero and Zhao48,Reference Turner-McGrievy and Beets75) which could indicate an underutilised opportunity for nutrition researchers and collaborative research.
Future investigations into social media platforms capabilities by nutrition researchers is warranted, along with keeping abreast of new and emerging platforms that may provide more in-depth or reliable insights into dietary behaviours(Reference Hermann88). Even though this review found that Twitter was the prominent social media platform, it should not be concluded that this represents a preferred tool for investigating dietary behaviours with SMA. Social media tools and the digital landscape are rapidly changing, and the prominence of Twitter could be explained by the ease of extraction of data via the Twitter API(Reference Moessner, Feldhege and Wolf89) and the fact that Twitter, launched in 2006, has been established longer compared with relatively newcomer platforms such as Instagram launched in 2010(Reference Sinnenberg, Buttenheim and Padrez21).
Paramount to nutrition research is the conduction of ethical research according to the Declaration of Helsinki including human ethics review and formal participant consent(41). However, there are significant ethical challenges facing nutrition and health researchers accessing social media data that require further exploration. The rapid rise in the popularity of social media has presented new dilemmas for tech companies and governments as privacy laws, data sharing policies and consumer protections have failed to keep pace(90). In this review, all studies that did obtain formal ethics approval(Reference Nguyen, Brunisholz and Yu52,Reference Nguyen, Meng and Li64,Reference Blackstone and Herrmann76,Reference Zhang, Hall and Bastola85) or exemption(Reference Cavazos-Rehg, Krauss and Sowles65) involved a health-related discipline. However, nearly three-quarters of the studies were found to make no mention of ethical considerations at all, with the majority here involving business/computing disciplines only; however, there were overall mixed results in the presence of health disciplines and ethical approval. These findings complement a recent review into social media data mining and health, highlighting that many researchers do not seek, or view the need to seek, formal human ethics approval(Reference Taylor and Pagliari91).
Nutrition and health professionals also face an array of additional, ethical practice dilemmas when utilising social media for research more broadly, such as when performing surveillance on at-risk communities with no intention-to-treat or with unclear relational boundaries in researcher–participant interactions. This review demonstrated that there was a lack of consistency and frameworks for the ethical assessment of social media data in research. Assumptions were made by some that, given users have chosen to place content in the public domain, social media data are outside ethical jurisdiction. Fundamentally, mining data from public domain social media accounts does involve easily identifiable individuals, at times sharing intimate details of their habits, who do not provide informed consent. Simply complying with company-derived privacy policies, along with established API terms for third-party use, is inordinately insufficient to maintain ethical integrity when collecting and storing personal social media data for nutrition research. It will be vital that these framework gaps, particularly in confidentiality and anonymity, should be addressed, and several groups including those from the US Council for Big Data Ethics and Society, the UK Data Service as well as the UK Society for Data Miners’ are currently working on ethical guidelines for researchers(Reference Taylor and Pagliari91).
Limitations
The number of databases searched was limited to five to complete the rapid review within the time frame set. For this reason, there were also no further searches conducted following the execution of the planned search strategy. Limitations also included English-language-only studies which may have excluded studies from other markets such as China where technology-related research is well established. Assessment of the public health nutrition relevance of studies to the investigation of dietary behaviours was a subjective process by two Accredited Dietitians and may have different interpretations by other nutrition researchers, for example, studies involving user-generated data on cuisine preferences in restaurant reviews may be interpreted as relevant or irrelevant to public health nutrition. Analysis of the disciplinary involvement was performed by listed author affiliations, and this review did not delve deeper into credentials or relevant researcher experience and conclusions could not be drawn on the probable benefits of collaborative research. Charting of the SMA steps uncovered areas of undefined scope and therefore was incomplete and generalised towards top line assessment of the scope of usage across the key SMA steps. Study authors were not contacted to clarify reporting gaps due to the nature of this rapid review. When assessing SMA use in each step across data discovery, preparation, collection and analysis, there were studies where manual coding was charted and SMA was not observed. However, the reasons for manual coding were not assessed and may not represent a lack of SMA expertise, rather there may have been limitations in machine learning capabilities or reliability warranting manual coding, or human coding may have been used to inform machine learning, or a combination, and specific interpretation was outside the scope of this review.
Conclusions
This rapid review was the first knowledge synthesis on SMA in nutrition research, particularly with the investigation of dietary behaviours in general population health using SMA on public domain, social media data and revealed that it is still emerging. The review revealed existing topics and platforms for nutrition and dietetics professionals to inform future collaborative research and to use SMA at a broad population census level, as a scoping tool or complementary, triangulation instrument to traditional research methods. However, careful consideration and planning need to be taken to investigate technological capabilities and maintain ethical integrity. Nevertheless, with the strong track record of innovation from rapidly advancing digital technology, an established track record of SMA in health and other research fields, along with the popularity of sharing eating behaviours on social media, the future of SMA in nutrition research with the investigation of dietary behaviours shows promise.
Acknowledgements
Acknowledgements: Craig Mack provided insights into social listening expertise in commercial organisations. Liz Harris, Senior Research Advisor La Trobe University Library, provided assistance with the search strategy. Emma Stirling is an Advanced Accrediting Practising Dietitian currently undertaking her PhD studies at La Trobe University and is also a Senior Lecturer in the Discipline of Nutrition and Dietetics, Australian Catholic University. Financial support: This research received no external funding. Conflict of interest: None. Authorship: ES, JW, K-L O and AF conceived and designed the study. ES, JW and AF contributed to data acquisition, analysis and interpretation. ES drafted the manuscript. JW and AF assisted with critically revising the manuscript. All authors approved the final version of this manuscript. Ethics of human subject participation: Not applicable.
Supplementary material
For supplementary material accompanying this paper, visit https://doi.org/10.1017/S1368980020005248