Introduction
Climate change has devastating effects on agriculture through increased erratic rainfall patterns and prolonged dry spells (Jew et al., Reference Jew, Whitfield, Dougill, Mkwambisi and Steward2020), ultimately resulting in reduced yield (Schlenker and Lobell, Reference Schlenker and Lobell2010). Sub-Sahara Africa (SSA) has experienced the worst impacts of climate change on agriculture over the past decades (Shahzad and Amjad, Reference Shahzad and Amjad2022), with projections showing intensifying climate shocks such as droughts and tropical cyclones (Burke and Lobell, Reference Burke, Lobell, Lobell and Burke2010; Lobell et al., Reference Lobell, Burke, Tebaldi, Mastrandrea, Falcon and Naylor2008; Pangapanga-Phiri et al., Reference Pangapanga-Phiri, Mungatana, Pangapanga and Nkoka2023). Among the major impacts of current and projected climate change include diminishing agricultural productivity and production (Challinor et al., Reference Challinor, Watson, Lobell, Howden, Smith and Chhetri2014). Smallholder agriculture requires interventions that can increase productivity to meet growing food demands amidst climate change (Cairns et al., Reference Cairns, Hellin, Sonder, Araus, MacRobert, Thierfelder and Prasanna2013). The sixth assessment report of the Intergovernmental Panel on Climate Change (AR6) projects increasing rainfall variability and higher temperatures in southern Africa. Malawi is particularly vulnerable to droughts and floods as epitomized by the El-nino events in 2015/2016 and 2023/2024 seasons, and cyclones Idai and Kenneth experienced in 2019 (IPCC, 2022). Future climate projections indicate that temperature will increase by 2°C on average, and rainfall will reduce by 1.8 and 5.1% in the north and southern regions, respectively, by mid-century in 2050 (CIAT and World Bank, 2018).
Sustainable intensification practices such as conservation agriculture (CA) are considered viable options (Thierfelder, Matemba-Mutasa, and Rusinamhodzi, Reference Thierfelder, Matemba-Mutasa and Rusinamhodzi2015a; Thierfelder and Mhlanga, Reference Thierfelder and Mhlanga2022). CA is a sustainable cropping system that can help reverse soil degradation, augment soil stabilization, increase land productivity (yield), and reduce labor requirements while producing high net returns (Jew et al., Reference Jew, Whitfield, Dougill, Mkwambisi and Steward2020; Ngwira, Thierfelder, and Lambert, Reference Ngwira, Thierfelder and Lambert2013; Thierfelder et al., Reference Thierfelder, Bunderson, Jere, Mutenje and Ngwira2016a; Reference Thierfelder, Rusinamhodzi, Setimela, Walker and Eash2016b). It is built on three core principles of minimum soil disturbance, crop residue retention, and crop diversification (Thierfelder et al., Reference Thierfelder, Baudron, Setimela, Nyagumbo, Mupangwa, Mhlanga, Lee and Gérard2018). CA can cushion farmers from the effects of climate change through its capacity to improve soil fertility, water retention (Thierfelder et al., Reference Thierfelder, Rusinamhodzi, Ngwira, Mupangwa, Nyagumbo, Kassie and Cairns2015b), infiltration capacity (Ngwira, Aune, and Thierfelder, Reference Ngwira, Aune and Thierfelder2014a; Ngwira et al., Reference Ngwira, Johnsen, Aune, Mekuria and Thierfelder2014b), and organic carbon (Eze et al., Reference Eze, Dougill, Banwart, Hermans, Ligowe and Thierfelder2020; Shahzad and Amjad, Reference Shahzad and Amjad2022). Thus, CA can help farmers adapt to and mediate the effects of climate change and weather variability (Komarek, Thierfelder, and Steward, Reference Komarek, Thierfelder and Steward2021; Pangapanga-Phiri and Mungatana, Reference Pangapanga-Phiri and Mungatana2021; Steward et al., Reference Steward, Dougill, Thierfelder, Pittelkow, Stringer, Kudzala and Shackelford2018).
However, the benefits of CA are site specific and the sustained adoption of CA differs across different socioeconomic profiles of farmers (Derpsch et al., Reference Derpsch, Friedrich, Kassam, Hongwen, Derpsch and Consultant2010; Knowler and Bradshaw, Reference Knowler and Bradshaw2007; Giller et al., Reference Giller, Andersson, Corbeels, Kirkegaard, Mortensen, Erenstein and Vanlauwe2015; Roques et al., Reference Roques, Kindred, Berry and Helliwell2022). Most CA trials are often supported by projects; hence, they miss out on the heterogeneity of smallholder farmers (Andersson and D'Souza, Reference Andersson and D'Souza2014; Hermans et al., Reference Hermans, Dougill, Whitfield, Peacock, Eze and Thierfelder2021; Ngoma et al., Reference Ngoma, Angelsen, Jayne and Chapoto2021). Furthermore, different institutions frame CA differently, leading to different CA types and performance at farmer level. As a result, the existing benefits and promotion of CA have so far not translated into widespread adoption among smallholder farmers in SSA countries like Malawi (Dougill et al., Reference Dougill, Whitfield, Stringer, Vincent, Wood, Chinseu, Steward and Mkwambisi2017; Hermans et al., Reference Hermans, Dougill, Whitfield, Peacock, Eze and Thierfelder2021). This leads to pertinent research questions: (i) if CA provides so many benefits for smallholders, why has its adoption not spread like wildfire? and (ii) if stakeholders have promoted CA heavily during the last three decades in SSA given its potential benefits, why has its adoption not picked up more rapidly among smallholder farmers?
Despite arguments for and against CA, its promotion has continued unfettered over the past three decades and adoption among smallholder farmers is rising, although slowly in SSA (Ngoma et al., Reference Ngoma, Angelsen, Jayne and Chapoto2021). There is sustained adoption in areas with long-term promotion (Tufa et al., Reference Tufa, Kanyamuka, Alene, Ngoma, Marenya, Thierfelder, Banda and Chikoye2023), hereafter called sentinel CA sites, suggesting that CA confers benefits to smallholder farmers (Kassie et al., Reference Kassie, Teklewold, Marenya, Jaleta and Erenstein2015; Ngoma, Mason, and Sitko, Reference Ngoma, Mason and Sitko2015, Reference Ngoma, Angelsen, Jayne and Chapoto2021; Tambo and Mockshell, Reference Tambo and Mockshell2018). However, there is dis-adoption and abandonment of some forms of CA in SSA, like in Zambia (Arslan, McCarthy, and Lipper, Reference Arslan, McCarthy and Lipper2013; Ngoma, Reference Ngoma2018), and Malawi (Ngwira, Aune, and Thierfelder, Reference Ngwira, Aune and Thierfelder2014a; Ngwira et al., Reference Ngwira, Johnsen, Aune, Mekuria and Thierfelder2014b; Ngwira, Thierfelder, and Lambert, Reference Ngwira, Thierfelder and Lambert2013; Thierfelder et al., Reference Thierfelder, Bunderson, Jere, Mutenje and Ngwira2016a). Tufa et al. (Reference Tufa, Kanyamuka, Alene, Ngoma, Marenya, Thierfelder, Banda and Chikoye2023) reported dis-adoption rates of up to 60% in Malawi, 25% in Zambia, and 10% in Zimbabwe. Nonetheless, CA sentinel sites provide good sites for case studies to gain deeper insights into CA diffusion and adoption dynamics and to draw lessons for CA scaling. This paper takes a deep dive at Nkhotakota district of Malawi, a sentinel site with a perceived high adoption of CA following its consistent promotion since 2005 by the regional non-governmental organization (NGO), Total LandCare and the International Maize and Wheat Improvement Center (CIMMYT) and other actors.
Suffice to mention that this perceived high CA adoption is not ubiquitous across Malawi, raising questions and interest to understand what could have led to the ‘apparent’ success of CA promotion and the subsequent high adoption in Nkhotakota district. In addition, it is still unclear which specific CA benefits and enablers induced adoption. This study addresses three related research questions: (i) what are the main factors that explain the extent and intensity of CA adoption in Nkhotakota; (ii) what are the main CA benefits that motivate farmers to switch from conventional farming practices to CA? and lastly (iii) to what extent does the host farmer extension approach explain the surge in CA adoption in Nkhotakota district?
The extent of adoption in this study is defined as continuous implementation of the CA practices, namely, minimum tillage (MT), mulching (MU), and crop rotation (CR), for at least 2 years. While the intensity of CA adoption refers to the number of years a farmer continuously implemented any CA practice. These definitions do not necessarily mean use of CA practices on the same plot from year to year, but it could be in different plots if these plots are owned or cultivated by the same household. In addition, the study defines a farmer that undertakes all the three practices of CA as full adopter, otherwise, we consider it as partial CA adoption. Our study used a unique survey design approach where for the treatment group, we randomly sampled respondents among farmers from villages known to have had contact with a CA host farmer. This is the first point of departure from previous studies that used random sampling from the whole population of farmers to assess CA adoption. As such, the findings in this paper should be interpreted to reflect the situation among farmers known to have had some exposure to CA within a CA sentinel site.
We add to literature on CA adoption in southern Africa in two main ways: first, using both quantitative and qualitative approaches, we focus on understanding the role of the host farmer approach in explaining the extent and intensity of CA adoption; and second, we highlight factors that motivated smallholder farmers to move from conventional practices to CA in the study areas. In sum, the study aims to validate methods for successful scaling of CA in Malawi that could provide lessons to other countries and regions in southern Africa.
Conservation agriculture in Malawi
The concept of CA is considered relevant in Malawi because of the high rates of land degradation, increasing water stress, small landholding sizes, and low average livestock heads per household, which further suggests low demand for crop residues for animal feed (Holden et al., Reference Holden, Katengeza, Fisher and Thierfelder2018; Ngwira, Aune, and Thierfelder, Reference Ngwira, Aune and Thierfelder2014a; Ngwira et al., Reference Ngwira, Johnsen, Aune, Mekuria and Thierfelder2014b). The majority (over 80%) of smallholder farmers depend on rainfed agriculture, hence, are continuously affected by water stress (Pangapanga-Phiri and Mungatana, Reference Pangapanga-Phiri and Mungatana2021). As a result, the Government of Malawi, international research institutes, and NGOs advocate for CA as an adaptation measure to climate stress. The Department of Agricultural Research Services (DARS) and Bunda College of Agriculture first conducted on-station and on-farm trials on the effects of CA on maize productivity and soil fertility in Malawi in the 1990s (Kassie et al., Reference Kassie, Teklewold, Marenya, Jaleta and Erenstein2015). The Sasakawa Global 2000 promoted CA in Malawi from the late 1990s and other institutions such as the International Maize and Wheat Improvement Centre (CIMMYT), Total LandCare (TLC), Concern Worldwide, World Vision International, and the Government of Malawi Department of Agricultural Extension Services (DAES) continued research and extension of the technology from the early 2000s onwards (Dougill et al., Reference Dougill, Whitfield, Stringer, Vincent, Wood, Chinseu, Steward and Mkwambisi2017; Jew et al., Reference Jew, Whitfield, Dougill, Mkwambisi and Steward2020; Mloza-Banda, Makwiza, and Mloza-Banda, Reference Mloza-Banda, Makwiza and Mloza-Banda2016; Mloza-Banda et al., Reference Mloza-Banda, Cornelis, Mloza-Banda, Makwiza and Verbist2014; Thierfelder et al., Reference Thierfelder, Chisui, Gama, Cheesman, Jere, Trent Bunderson, Eash and Rusinamhodzi2013).
CA was reintroduced in some target areas such as Balaka (South), Dowa (Central), and Mzimba (North) regions of Malawi since 2004. These efforts were led by DARS, the Department of Agriculture Extension Services (DAES), and CIMMYT. Since 2005, TLC, with support from CIMMYT expanded CA promotion to other districts such as Nkhotakota (NKK), Salima, Dowa, and Kasungu (Thierfelder et al., Reference Thierfelder, Bunderson, Jere, Mutenje and Ngwira2016a). For instance, CIMMYT implemented a GIZ- and later IFAD-funded project from 2004 to 2012 on CA systems in the Nkhotakota, Salima, Kasungu, and Dowa districts of Central Malawi and Balaka, Machinga, and Zomba districts of Southern Malawi; a CGIAR-funded program called Sustainable Intensification of Maize-Legume Cropping Systems in Eastern and Southern Africa (SIMLESA) was conducted in six districts of central Malawi, namely Kasungu, Mchinji, and Lilongwe in the mid-altitude agro-ecology and Balaka, Ntcheu, and Salima in the low altitude. From 2015 onwards, CIMMYT supported CA research through a GIZ-funded Climate-Smart Agriculture project and the USAID-funded Africa Research in Sustainable Intensification for the Next Generation (Africa RISING) project in Nkhotakota, Dowa, Salima, Balaka, Machinga, and Zomba districts. At present, scaling of CA systems is still ongoing with support from the CGIAR-funded Regional Initiative Ukama Ustawi: diversification for resilient agribusiness ecosystems in East and Southern Africa and the Global Initiative, Sustainable Intensification of Mixed Farming Systems (SI-MFS).
Different approaches have been used to increase CA adoption among smallholder farmers over the years. They have centered around establishing demonstration plots within promotional areas. In some instances, demonstrations have been accompanied by provision of credit or other technical support for agriculture inputs such as seeds, fertilizer, and herbicides as incentives to encourage farmers to adopt CA. For instance, the Government of Malawi and other partners have used lead farmers or demonstration plots, agricultural fairs, and farmer field schools to show the technical details and procedures of various CA practices (Fisher et al., Reference Fisher, Holden, Thierfelder and Katengeza2018). While TLC and CIMMYT have used the host farmer extension approach to promote the adoption of CA in Nkhotakota district through on-farm demonstration plots under the different projects. In the context of this paper, a host farmer is one of the farmers, trained by a TLC field officer and has his or her own plot to demonstrate CA practices. Furthermore, the host farmer trains and transfers knowledge and skills to other farmers in a village through field days, agricultural fairs, or farmer field schools. The difference between the host and lead farmer approach is that the host farmer has their own plot to demonstrate CA, while the lead farmer does not necessarily need to have any plot to demonstrate CA, except the technical knowledge and transfer they provide to farmers.
Data collection and methods
Farm household survey
We use a household survey of smallholder farmers across three extension planning areas (EPAs) in Malawi's Nkhotakota district, serving as the location for this study (Fig. 1). Covering 4338 square kilometers, with 410,891 inhabitants, Nkhotakota district has a population density of 94 individuals per square kilometer.
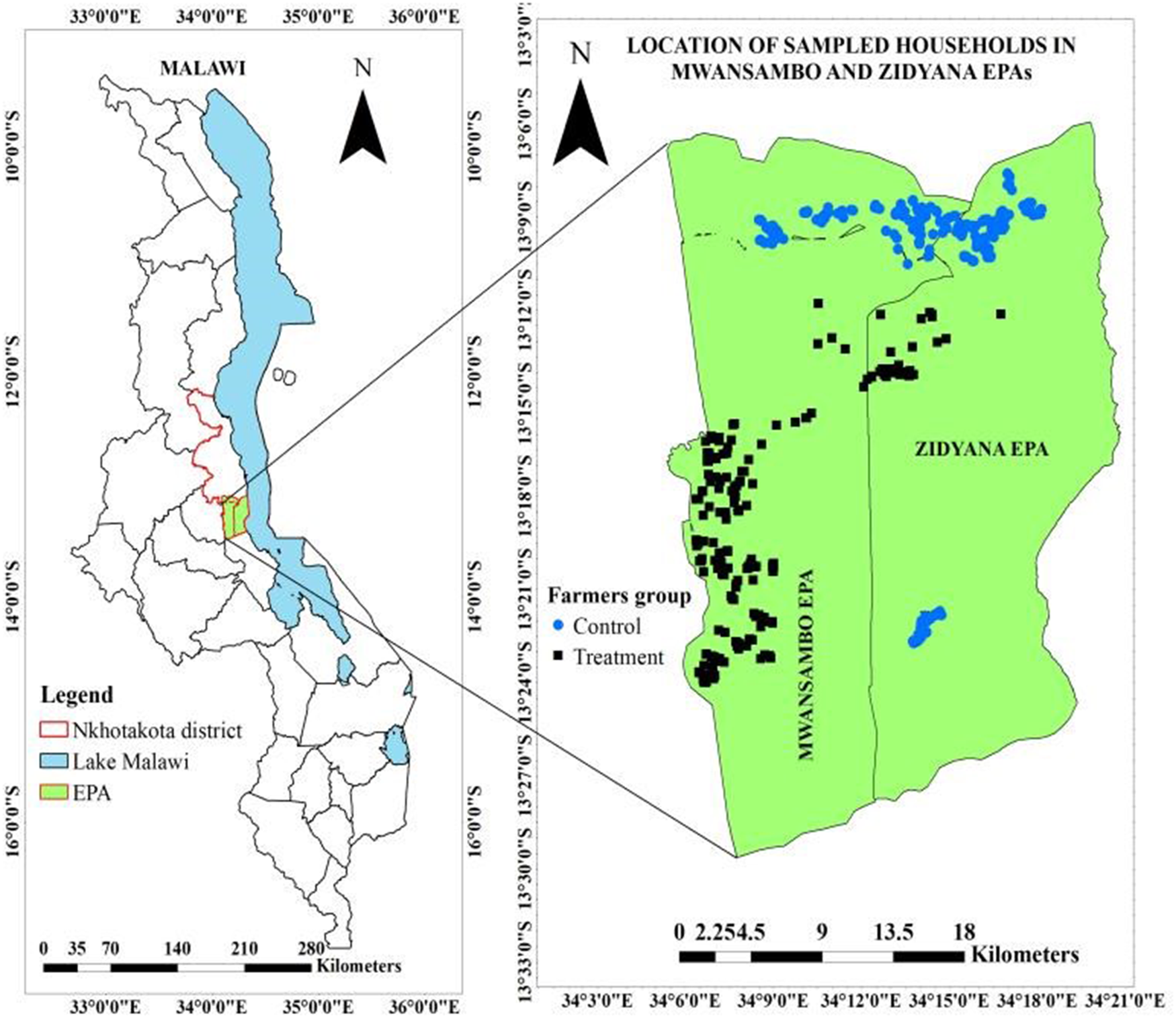
Figure 1. Map of the Nkhotakota district, showing sampled farmers in Mwansambo and Zidyana (treatment, black dots in the center), and Mtosa (control areas, blue dots at the top) extension planning areas.
Women account for 51% of the population. Temperatures for Nkhotakota range, on average, from 19.7°C in June to 29.7°C in November. Nkhotakota is characterized by a unimodal rainfall pattern with a rainy season from November to April. The district receives between 1000 and 1300 mm of rainfall annually (Thierfelder, Matemba-Mutasa, and Rusinamhodzi, Reference Thierfelder, Matemba-Mutasa and Rusinamhodzi2015a; Thierfelder and Mhlanga, Reference Thierfelder and Mhlanga2022) with occasional peaks of over 1600 mm.
Using a combination of qualitative and quantitative methods, we interviewed a total of 620 households between October and November 2022. We used a multi-stage cluster sampling procedure, where in the first stage, we purposively chose three EPAs namely, Mwansambo, Zidyana, and Mtosa. CIMMYT and TLC managed CA projects in Mwansambo and Zidyana EPAs since 2005 and never implemented any similar project in Mtosa EPA. On average, the agroecological profiles for the control areas are similar to the treatment areas except for the support from the CIMMYT and TLC CA projects. Mwansambo and Zidyana EPAs are known to have high adoption of CA compared to other areas within the district or across the country. Maize is the most dominant crop farmed by over 90% of the farmers and takes up the bulk of the land under cultivation in the study EPAs. It is followed by groundnuts, planted extensively as a cash crop (National Statistics Office, 2020). Agricultural frontline officers from the government and TLC helped the study team to sample the areas to avoid contamination of information between the two groups.
In the second step, four sections in the treatment areas and two sections in control areas were sampled. In the third step, we randomly selected about 298 and 322 smallholder farmers from the treatment and control areas, respectively. The sampling frame for study participants in the treatment areas were those farmers from villages known to have some contact with any of the host farmers, who worked with TLC. Farmers in the control areas or villages had no known contact with any of the TLC extension staff or TLC-trained host farmers but had some contacts with other farmers and other organizations like the Ministry of Agriculture. However, these organizations were also present in the treatment areas, making the host farmer approach championed by TLC the major missing link from the control areas. To complement the quantitative assessment, the study also consulted 120 farmers through focus group discussions (FGDs), with eight FGDs in the treatment areas. The FGD design accounted for gender disaggregated information, where women and men formed their own groups. Six key informants involving government extension agents, local leaders, lead farmers, and host farmers were also consulted for the study.
The interviews were carried out by well-trained enumerators with a bachelor's degree and experience in agriculture. Primary data were collected using a semi-structured questionnaire. The household survey questionnaire was programmed in a computerized assisted personal interview, the World Bank Survey Solutions, and was deployed to capture data on the socioeconomic status of farmers, agricultural production activities, asset ownership, uses of CA practices, social networks, access to finance, and use of extension services. The server hosting the data was managed and maintained by the Centre of Agricultural Research and Development (CARD) of the Lilongwe University of Agriculture and Natural Resources (LUANAR).
Measuring outcome variables
Two outcome variables, namely, extent and intensity of adoption, are used in this study to understand uptake of CA in Malawi's Nkhotakota district. The extent of CA adoption is measured based on continuous implementation of any of the CA practices on either the same or different plot by a household for at least 2 years. We assume that only households undertaking various CA practices over a long period derive the value for money for investing in CA (Ngoma et al., Reference Ngoma, Angelsen, Jayne and Chapoto2021; Thierfelder et al., Reference Thierfelder, Mupangwa, Chivenge, Rosenstock, Lamanna and Eyre2017; Tufa et al., Reference Tufa, Kanyamuka, Alene, Ngoma, Marenya, Thierfelder, Banda and Chikoye2023). The intensity of CA adoption refers to the number of years a farmer continuously implemented any of the CA practices, like MT, MU, and CR. Full adoption is defined as continuous implementation of all the three CA practices, while otherwise, it means partial adoption. Suffice to emphasize here that continuous implementation in this paper includes use of CA on any plot and not necessarily on the same plot.
Estimation strategy
First, we hypothesize that a smallholder farmer continuously adopts any of the CA practices since they improve land productivity. Based on the cross-sectional data, we estimate the following non-linear regression model:

where A ij is a vector of sustained CA adoption measure = 1 if the household continuously adopts any of the CA practices (j = 1, 2, and 3), namely, MT, MU, or CR, for at least 2 years. The study further assumes that household choices of CA practices influence each other or are jointly determined. Analyzing such choices lends itself to a multivariate probit framework. The study estimates equation (1) based on each CA practice as a binary variable and mutually determined. For instance, agricultural frontline officers stated that MU and MT are adopted jointly, where mulching amplifies the benefits of minimum tillage. Likewise, recent studies, e.g., Tufa et al. (Reference Tufa, Kanyamuka, Alene, Ngoma, Marenya, Thierfelder, Banda and Chikoye2023) have used multivariate probit regression to understand factors affecting CA adoption. Hij denotes the host farmer who provides software or hardware technical support to farmers within their vicinity. Software technical support includes training on the merits and demerits, as well as technical knowledge of CA. While hardware technical support involves demonstration plots where smallholder farmers can visibly see the benefits of CA. In addition, the hardware support includes providing farmers with CA-related inputs such as herbicides and pesticides. Xij represents a vector of household and farm characteristics such as education in years, age in years, gender of plot manager, membership to farmer's group, reliance on relatives or social capital for agricultural support, and landholding size. ${\rm \varepsilon }_{ij}$ is the stochastic white noise, with zero mean and constant variance (Greene, Reference Greene2012). Based on the study hypotheses, the parameter of interest for the study from equation (1) is ωj which captures the relationship between host farmer extension approach and the extent of adoption of CA practices. We assume a positive relationship between the host farmer approach and the extent of CA adoption. Based on the significant covariance between or across various CA practices, the multivariate probit regression model econometrically provides unbiased estimates explaining the positive effect of host farmer approach on CA adoption. However, there could be some selection bias such that the host farmer variable could be endogenous, which may arise from measurement errors, reverse causality, and unobservable heterogeneity. In this study, we assume the use of well-trained enumerators reduced measurement errors.
Second, the study assumes that host farmer approach influences the intensity of CA adoption. Hence, the study hypothesizes a positive relationship between host farmer approach and the intensity of CA adoption as prior defined. Based on the count or duration modeling, the study specifies the following Poison equation (2) (see detail in Greene, Reference Greene2012):

where y ij is the count-dependent variable measured as the number of years smallholder farmers have continuously implemented all or any of the three CA practices. While other variables and parameters are as previously defined. Similarly, the main parameter of interest for the study from equation (2) is γj which indicates the relationship between host farmer approach and intensity of CA adoption.
Results and discussion
Characteristics of the sample
Most of the sample households (80%) were headed by males (Table 1), which is consistent with National Statistics Office (2020), where it is reported that almost one-fifth of households are headed by female farmers in Malawi. Household heads were of productive age, on average 45 years, and were predominantly in monogamous marriages. Households in both the control and treatment areas had an average of six household members, but those from the treatment areas had significantly more adult male members (P < 0.05). Besides, most household heads (69%) in the study area had no formal education qualification. Households that participated in this study were typical farmers, with 90% relying on farming as the main occupation and on average had a total landholding size of 4.17 acres. Cultivable land in the study area averaged 2.48 acres overall, 2.96 acres in the treatment, and 2.02 acres in the control areas. These are similar to results reported by the National Statistics Office (2020). However, the study notes that households in the treatment areas had significantly larger land parcels and cultivated land (P < 0.01).
Table 1. Socioeconomic characteristics of the sample households
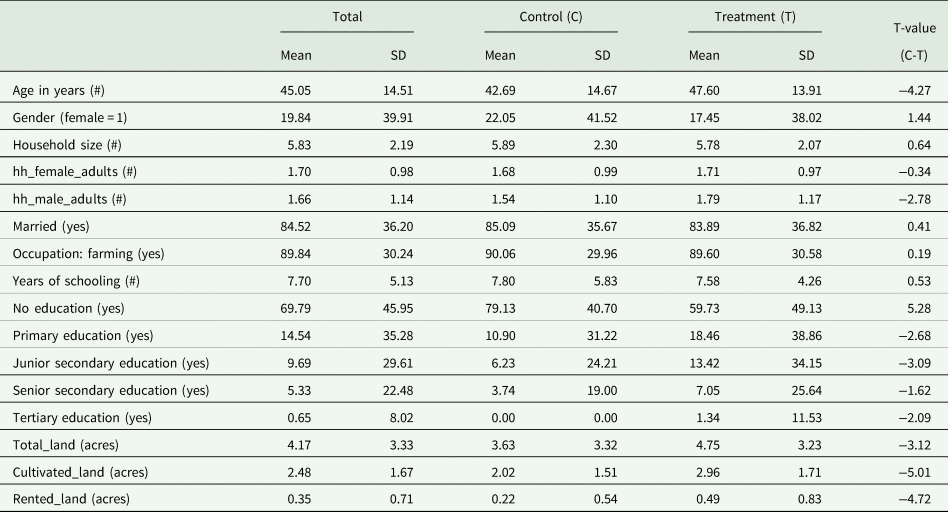
Social networks and access to credit
Social networks are one of the main channels through which agricultural technologies are diffused (Wang, Lu, and Capared, Reference Wang, Lu and Capared2020) through social interaction (Genius et al., Reference Genius, Koundouri, Nauges and Tzouvelekas2014). As such, we hypothesized that the success of the host farmer extension approach largely depends on the social network to facilitate diffusion. In this study, we measured social network following (Dearing, Reference Dearing2021; Rogers, Singhal, and Quinlan, Reference Rogers, Singhal and Quinlan2019; Wang, Lu, and Capared, Reference Wang, Lu and Capared2020; Yu, Reference Yu2022; Zhang, Reference Zhang2018) as: (i) the number of reliable relatives or friends that a household has in leadership positions; (ii) whether there is a household member belonging to a farmer organization; (iii) whether the household is related to the village chieftaincy; and (iv) whether there is a serving member of parliament that hails from the same village as the household head/spouse.
A larger number of farmers in the treatment group had greater social networks than farmers in the control group (Table 2). There were significantly higher number of farmers in the treatment group that: (a) were related to village leadership than in the control group, (b) were members of farmer organizations, and (c) had friends in leadership positions than farmers in the control group (P < 0.05). In terms of access to credit, only 17% of farmers in the sample accessed credit to support various agriculture activities. About 22 and 13% accessed credit in the treatment and control groups, respectively. In terms of what the credit was used for, farmers reported during FGDs that credit was used to purchase inorganic fertilizer, improved seeds, pesticides, or herbicides, and sometimes inputs or equipment used for CA like rippers.
Table 2. Social networks and credit accessibility between treatment and control groups

Extent and intensity of adoption of full and partial conservation agriculture
In line with Rogers, Singhal, and Quinlan (Reference Rogers, Singhal and Quinlan2019) and Zhang (Reference Zhang2018), instantaneous or year-on-year adoption of CA is facilitated through farmers observing, learning, and discussing CA with host farmers in the treatment areas or lead farmers in the control areas. The study's interest is to assess the extent and intensity of CA adoption based on the definitions provided above. As previously pointed out, TLC had contact with host farmers only, who had contact with farmers within their vicinity. Overall, about 31% of the surveyed farmers continuously adopted full CA in the study areas for at least 2 years (Table 3 and Fig. 2). Slightly more than half of the farmers (57%) in the treatment area adopted full CA, while only 7% of farmers in the control group had full adoption of CA practices for at least 2 years. Partial adoption remains most prevalent. The most common combinations of partial CA adoption in the treatment area included mulching and crop rotation (MUCR) (71%), followed by minimum tillage and mulching (MTMU) (67%) and minimum tillage and rotation (MTCR) (60%). While in the control, the common combinations of partial CA adoption included MUMT (16%), followed by MUCR (9%) and MTCR (8%). A χ2 test revealed a significant association between the type of CA adopted by treatment (P < 0.05). When we define partial adoption as the use of MT and other practices, MTMU is most prevalent among the treated and control at 67 and 16%, respectively. Moreover, farmers in the treatment group implemented CR, MU, and MT continuously for 5.12 ± 3.93, 4.60 ± 3.38,, and 3.16 ± 3.49 years (Table 3), suggesting that the length of continuous CA use was significantly higher in the treatment than in the control group (P < 0.05). Similarly, households in the treatment group allocated varying amounts of land toward CA practices, for instance, 2.64 ± 5.25 acres under CR, 1.85 ± 2.15 acres under MT, and 1.74 ± 2.15 acres under MU.
Table 3. Extent and intensity of CA adoption between treatment and control groups
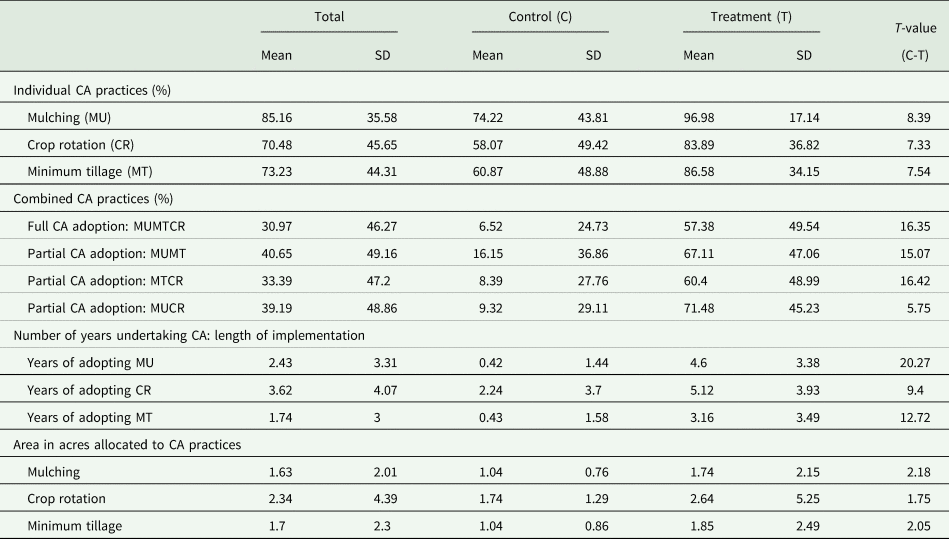
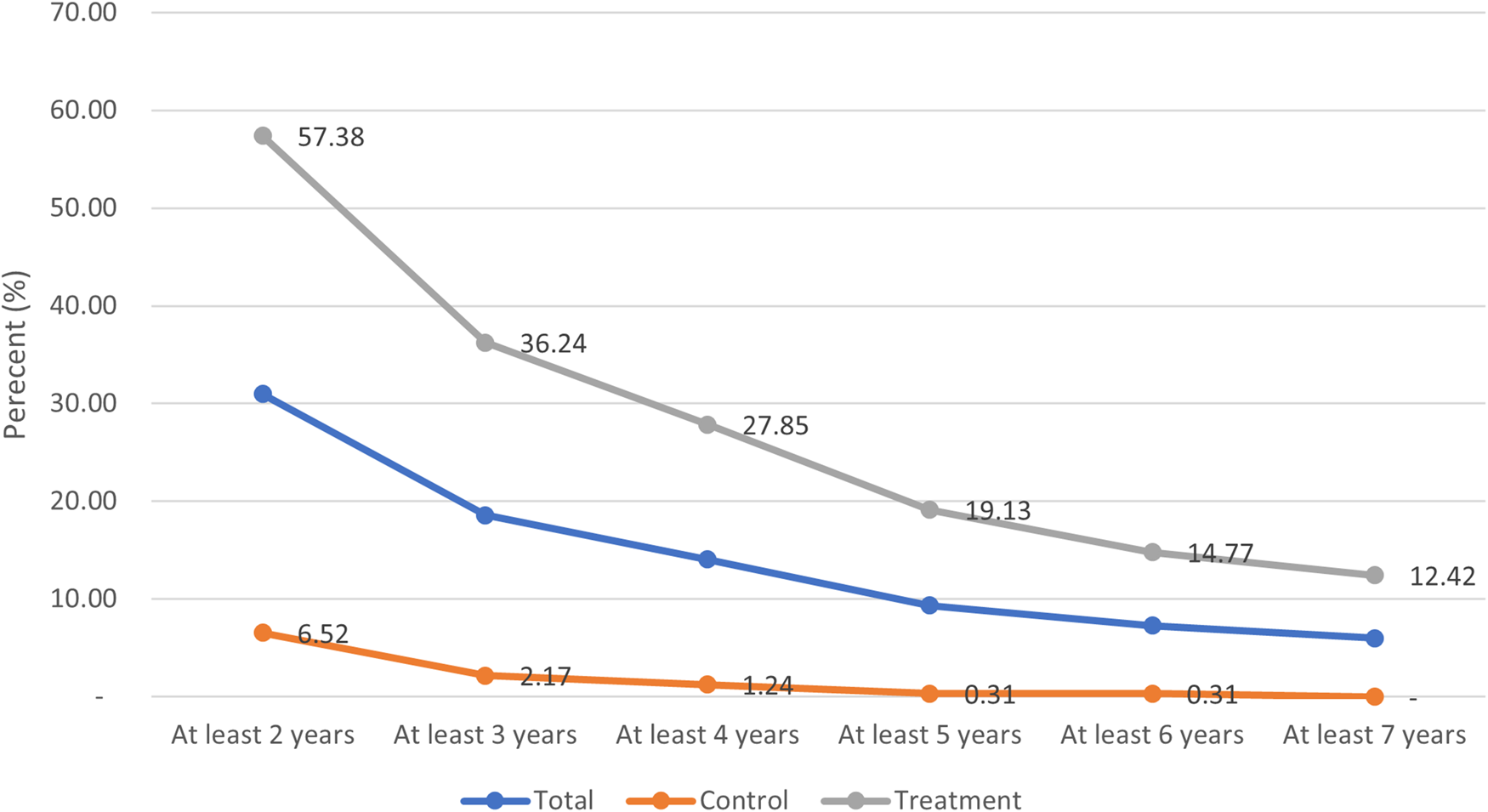
Figure 2. Proportion of farmers adopting full CA over time.
Despite some positives, these results suggest that 69% of the overall sampled farmers did not adopt full CA over the previous 2 years from the 2021/2022 agricultural season. We also find adoption decay, where the proportion of farmers undertaking full CA declines over time. For example, the adoption curve in Figure 2 shows that adoption reduced from highs of 57 and 7% for at least 2 years for treatment and control, respectively, to 12% in the treatment group and practically zero in the control when we condition full CA adoption to at least 7 years (Fig. 2). This suggests the preponderance of dis-adoption as has been suggested by others, e.g., Arslan et al. (Reference Arslan, Mccarthy, Lipper, Asfaw and Cattaneo2014). These results also suggest a critical need to identify levers for persistent adoption.
It is worth emphasizing that this result should not be conflated with adoption over time, which in general terms is rising. The main insight from Figure 2 is that farmers that adopt CA do not seem to persist over time. In attempts to explain dis-adoption, farmers highlighted several factors responsible for dis-adoption of CA during FGDs. These include (i) increased weeds and pests perceived to be a result of MT; (ii) lack of by-laws to control grazing, although livestock densities are comparably low in the target areas as compared to other locations in southern Africa; (iii) burning of crop residues by mice hunters or crop residues used as fuel; (iv) perceived high costs of herbicides or pesticides, considered a prerequisite to do CA; (v) high cost of chemical fertilizer, considered important for successful CA implementation; (vi) lack of technical assistance; and (vii) unclear/conflicting extension messages. FGDs also perceived inorganic fertilizer as an important factor for complementing the adoption of CA. Consistent with findings in Mulimbi et al. (Reference Mulimbi, Nalley, Dixon, Snell and Huang2019) and Tufa et al. (Reference Tufa, Kanyamuka, Alene, Ngoma, Marenya, Thierfelder, Banda and Chikoye2023), farmers cited the lack of credit to purchase equipment and inputs as one of major factors that contributed to dis-adoption of CA in the study areas, although this is not only unique to CA.
Perceived CA benefits
Despite the complexity and challenges associated with implementing any technology (Fisher et al., Reference Fisher, Holden, Thierfelder and Katengeza2018) like CA, results from both individual farmers interviews and FGDs revealed that CA is beneficial among adopters, thereby motivating farmers to switch from conventional to CA. Farmers that implemented CA obtained higher yields per acre than farmers that practiced conventional tillage practice (CTP) (see Fig. 3). For maize and groundnuts, the two most common crops in the study area, we find that CA outperformed conventional. For instance, farms under CA obtained 1524 kg ha−1 more maize yield than farms under CTP, implying that CA significantly enhanced maize productivity. Similarly, farmers using CA produced 595 kg ha−1 more groundnuts per ha than farmers undertaking CTP. These results were consistent with Thierfelder et al. (Reference Thierfelder, Chisui, Gama, Cheesman, Jere, Trent Bunderson, Eash and Rusinamhodzi2013), where authors found that plots under CA had substantially higher yield than plots under CTP systems.
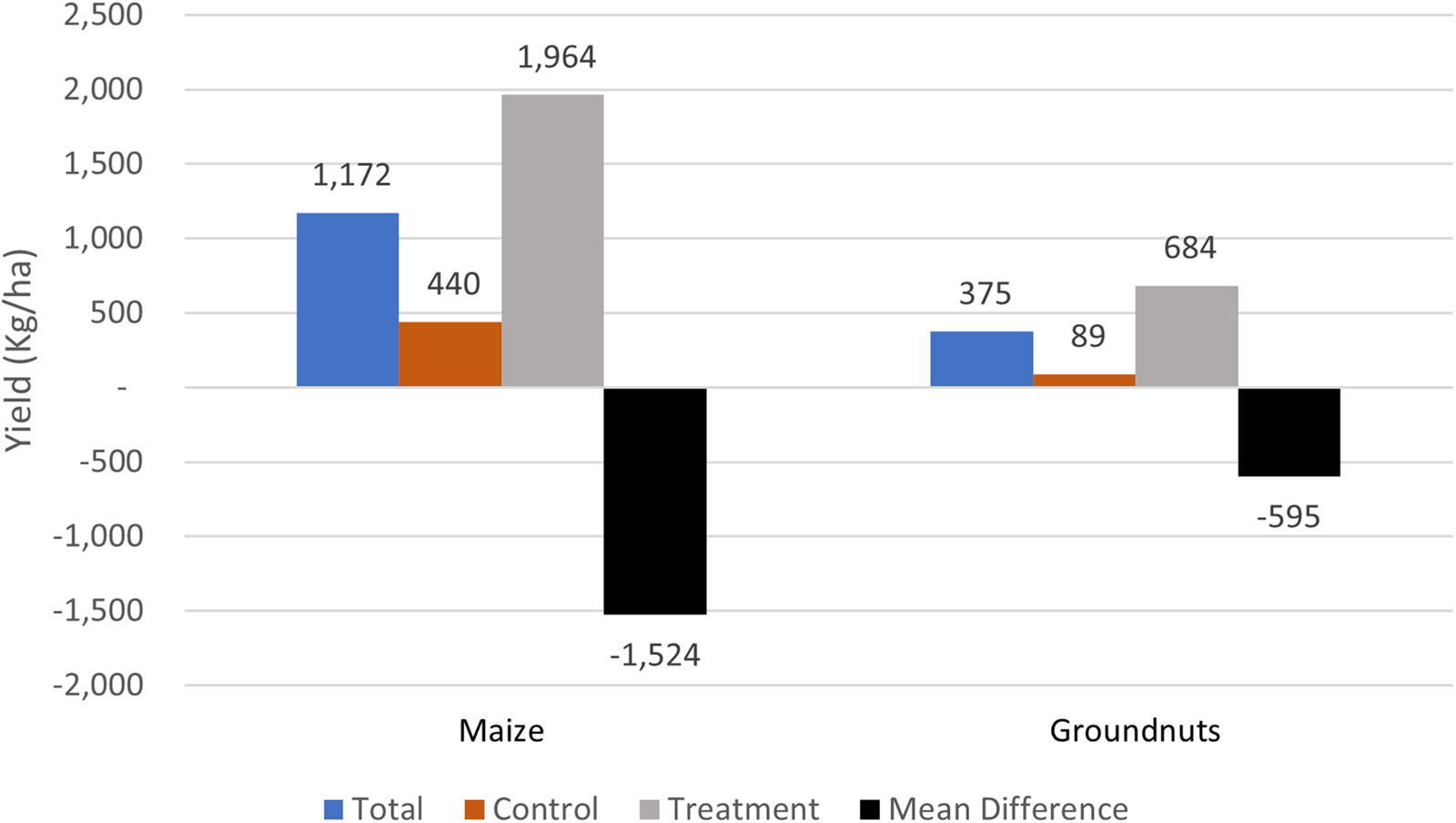
Figure 3. Comparison of farmer reported mean yield (kg) per hectare (ha) between CA and CTP systems.
When broken down by type of benefit, a larger number of participants in FGDs indicated that CR improved soil fertility (86%), reduced pest infestation (64%), and induced higher production (79%) (Fig. 4). Similarly, 71 and 64% of FGDs participants reported that MU preserves soil moisture and promotes soil fertility, respectively. While MT was reported to reduce labor demand during land preparation by 79 and 71%, respectively. These findings are in line with previous studies from sub-Saharan African countries, including Malawi, that show that CA increased grain yields for various crops such as maize and groundnuts (Ngwira, Thierfelder, and Lambert, Reference Ngwira, Thierfelder and Lambert2013; Thierfelder et al., Reference Thierfelder, Bunderson, Jere, Mutenje and Ngwira2016a).
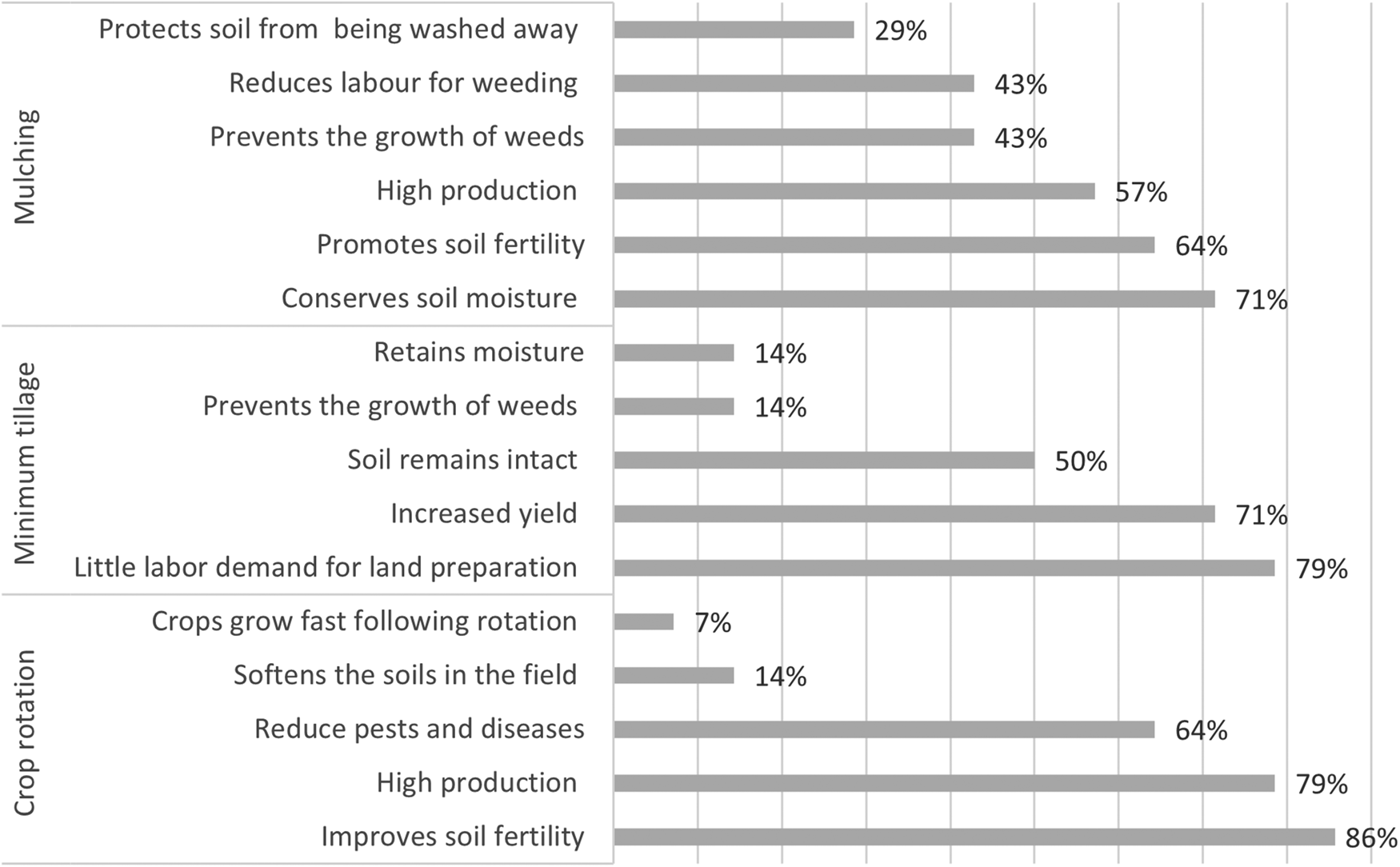
Figure 4. Proportion of farmers that perceived benefits for the three main CA practices in the study area.
Notes: Based on 120 focus group discussions participants.
One of the FGD participants in Zidyana EPA narrated that: ‘In times when there was below normal rainfall, crops under CA grew healthy and did not wilt while crops under CTP wilted significantly. Moreover, fields that adopted CA had higher yields than those under CTP.’ Another participant from Mwansambo EPA explained that fields under CA did not require a hoe to remove weeds as in CTP systems. Similarly, Tufa et al. (Reference Tufa, Kanyamuka, Alene, Ngoma, Marenya, Thierfelder, Banda and Chikoye2023) reported that using CA practices such as MU, MT, and CR reduce the effects of climate variability and declining soil fertility through conserving moisture, biofertilization, and changing soil properties. Likewise, Derpsch et al. (Reference Derpsch, Friedrich, Kassam, Hongwen, Derpsch and Consultant2010); Kassam et al. (Reference Kassam, Friedrich, Shaxson and Pretty2009); and Mulimbi et al. (Reference Mulimbi, Nalley, Dixon, Snell and Huang2019) highlight that CA provides better ecosystem functioning and services, which are beneficial to areas affected by climate change. Nevertheless, Thierfelder, Matemba-Mutasa, and Rusinamhodzi (Reference Thierfelder, Matemba-Mutasa and Rusinamhodzi2015a) observed that farmers could derive benefits out of CA adoption only after 2–5 years. Agronomically, this creates a lag in realizing benefits possibly further leading to no adoption or complete abandonment of CA.
Factors determining the extent and intensity of CA adoption
Multivariate probit and Poison regression models were used to estimate the associations between the host farmer approach and the extent and intensity of CA adoption, respectively. Table 4 presents results for the multivariate probit regression for the extent of adoption while Table 5 shows results from the Poison regression for the intensity of adoption.
Table 4. Results from the multivariate probit analysis of factors affecting extent of CA adoption in Nkhotakota
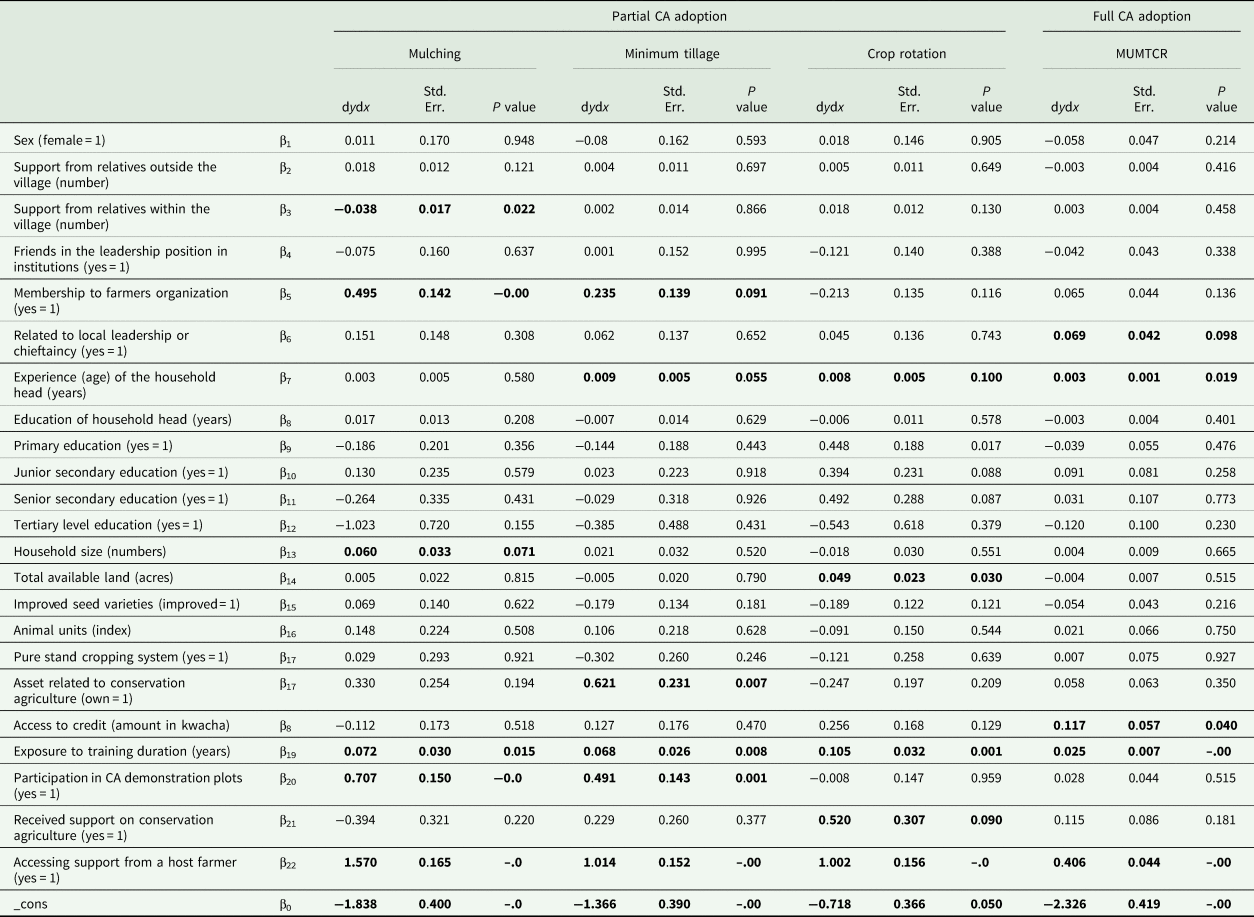
Notes: Significant factors or variables are highlighted in bold.
Table 5. Results from the Poisson regression analysis of factors affecting intensity of CA adoption in Nkhotakota
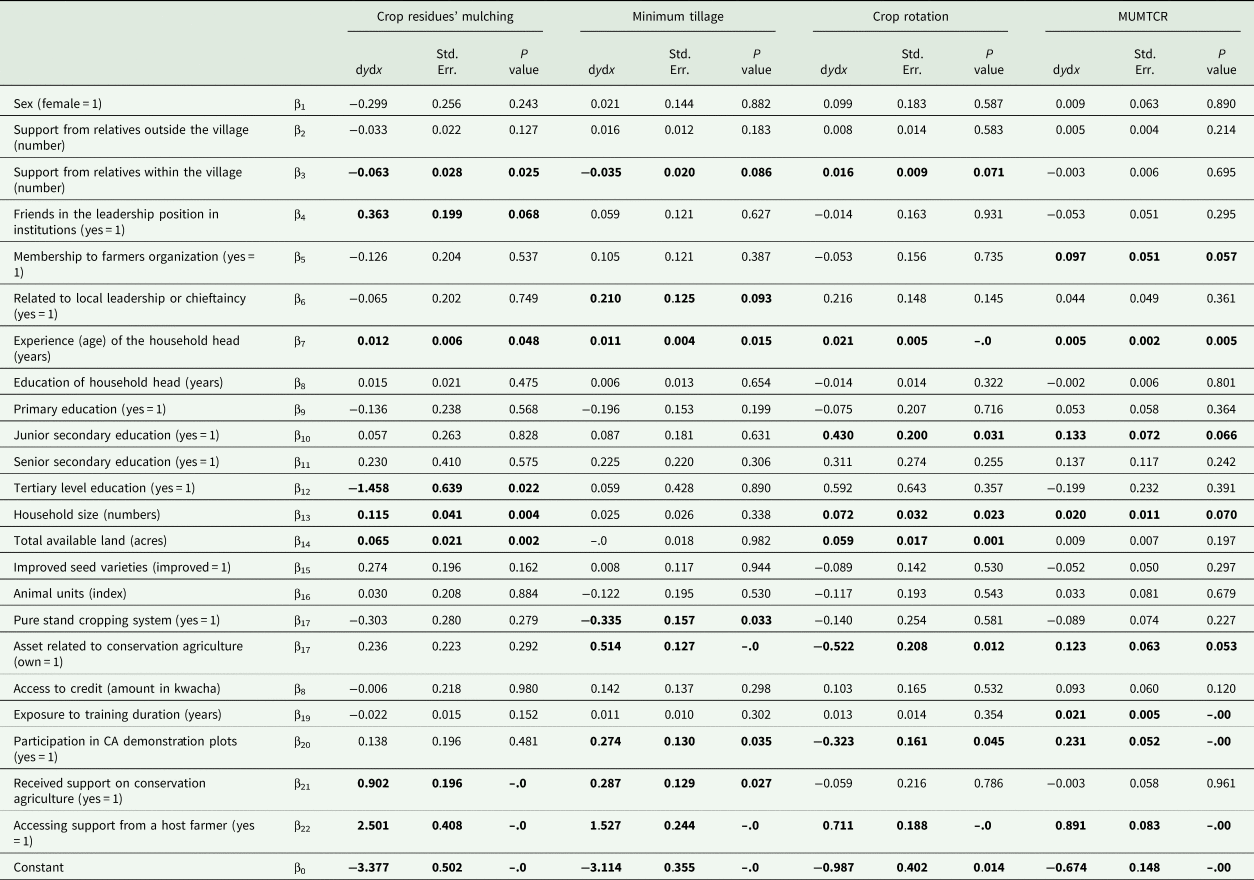
Notes: Significant factors or variables are highlighted in bold.
First, in this study, we aimed at understanding factors influencing the extent of adoption of the various CA practices in the study area, with particular attention paid to the effects of the host farmer approach. Hence, based on the multivariate regression (Table 4), the study found that accessing information from a host farmer was positively related with both the extent and intensity of adoption in the study area. For instance, farmers getting extension support from the host farmer were generally more likely to adopt any of the individual or full CA practices. We observed that social network factors like getting help from relatives residing outside the village are positively related to adoption of MU, MT, and CR. Similarly, longer duration of CA exposure positively influenced farmer's decisions to adopt MU, MT, and CR. This could be related to the fact that longer exposure might allow farmers to better understand the merits and demerits of CA practices. Demonstration plots for CA also influenced household decisions to adopt MU, MT, except for CR. Increased available total arable land at household level enhanced adoption of CR in the study area.
Second, the study assessed factors associated with the intensity of CA adoption using the Poisson regression (Table 5). A Poisson regression is a duration model (see detail in Greene, Reference Greene2012) hence the dependent variable for this model is the number of years smallholder farmers have continuously implemented CA on their farm. Results suggest that access to CA inputs, project support, and participation in CA projects considerably improved the intensity of MU, MT, and CR adoption in the study area. There is an increasing percentage of farmers in the treatment area continuously implementing CA between 2000 and 2021, with a larger number of farmers adopting full CA after 2010.
Discussion
The role of the host farmer approach in CA adoption
Results revealed that the host farmer approach had a positive effect in enhancing adoption of CA practices among farmers. Not only is this evident as significantly higher numbers of farmers continuously implemented various CA practices but more farmers in the treatment group also adopted full CA than farmers from the control group. The host farmer approach influenced CA adoption by providing CA technical information as was found in Ngwira, Aune, and Thierfelder (Reference Ngwira, Aune and Thierfelder2014a; Ngwira et al., Reference Ngwira, Johnsen, Aune, Mekuria and Thierfelder2014b). In addition, the host farmers established demonstration plots which showcased how CA should be practiced and therefore served as role models for others. During FGDs, farmers indicated that demonstration plots also removed fear for the unknown and debunked some myths regarding CA systems, for example, that practitioners show ‘laziness’ if they do not conventionally till their land.
Unlike other studies that found that the host farmer approach only increases awareness of CA but not adoption (Holden et al., Reference Holden, Katengeza, Fisher and Thierfelder2018), our results suggest that both awareness and adoption increased. Tufa et al. (Reference Tufa, Kanyamuka, Alene, Ngoma, Marenya, Thierfelder, Banda and Chikoye2023) reported that high investments in CA awareness have not yet translated in equally high CA adoption. However, in this paper based on CA sentinel sites shows that longer term CA promotions increased the extent of CA adoption by 57%, basically, because host farmers in the sentinel area closely monitored, followed up, trained, and demonstrated the merits and demerits of CA adoption. This is in line with other scholars (Jumbe and Nyambose, Reference Jumbe and Nyambose2016; Nyanga, Reference Nyanga2012; Olawuyi, Reference Olawuyi2019; Tufa et al., Reference Tufa, Kanyamuka, Alene, Ngoma, Marenya, Thierfelder, Banda and Chikoye2023) who noted that longer-term demonstration of CA increased the likelihood of CA adoption. Likewise, Habanyati, Nyanga, and Umar (Reference Habanyati, Nyanga and Umar2020) found that knowledge about CA is an important precursor to adoption. While Knowler and Bradshaw (Reference Knowler and Bradshaw2007) highlighted that knowledge and information about CA reduced uncertainties and fears associated with CA adoption and its complexities.
The high extent of CA adoption among the treatment farmers could be explained by considering high social capital. Following Rogers, Singhal, and Quinlan (Reference Rogers, Singhal and Quinlan2019)'s adoption and diffusion theory, social networks help farmers discuss various technologies including CA. Usually, farmers would easily believe the success stories experienced by fellow farmers, which implied that they could implement and derive similar benefits as other fellow farmers. In the study area, farmers from the treatment group had higher social networks than farmers from control groups, thereby increasing the likelihood of being exposed to and minimized trust issues regarding CA adoption. During FGDs, it was revealed that adoption of CA in the early phases was highly affected by trust issues. Mulimbi et al. (Reference Mulimbi, Nalley, Dixon, Snell and Huang2019) found that the participation of farmers in associations was believed to lead to greater CA adoption. Increased social networks built more trust and increased CA adoption (Olawuyi, Reference Olawuyi2019). Through the host farmer approach, the concept of ‘baby trial farmers’ was introduced, which helped to transfer knowledge. Such groupings increased farmer interactions and augment the probability of adopting CA (Ngwira, Aune, and Thierfelder, Reference Ngwira, Aune and Thierfelder2014a; Ngwira et al., Reference Ngwira, Johnsen, Aune, Mekuria and Thierfelder2014b).
Even though most farmers from the treatment group had adopted different CA principles, about 43% of them did not adopt the full CA package, i.e., a combination of MT, MU, and CR. This finding was not unique to this study, several studies on CA in SSA (Arslan, McCarthy, and Lipper, Reference Arslan, McCarthy and Lipper2013; Grabowski and Kerr, Reference Grabowski and Kerr2014; Kunzekweguta, Rich, and Lyne, Reference Kunzekweguta, Rich and Lyne2017; Tambo and Mockshell, Reference Tambo and Mockshell2018) have reported that partial adoption of CA principles remain prevalent among smallholder farmers. When farmers were exposed to CA, they developed cropping systems (Nkhoma, Kalinda, and Kuntashula, Reference Nkhoma, Kalinda and Kuntashula2017) that were intermediates between CA and CTP systems (Penot et al., Reference Penot, Domas, Fabre, Poletti, Macdowall, Dugué and Le Gal2015). These intermediate cropping systems were developed to address specific agricultural production constraints encountered by farmers under CA. The partial adoption of CA was not only in terms of practices but also the proportion of cultivated land area under CA (Nkhoma, Kalinda, and Kuntashula, Reference Nkhoma, Kalinda and Kuntashula2017). In this study, less than 50% of farmers reported practicing CA on the entire farm. However, Kassam et al. (Reference Kassam, Friedrich, Shaxson and Pretty2009), Thierfelder et al. (Reference Thierfelder, Baudron, Setimela, Nyagumbo, Mupangwa, Mhlanga, Lee and Gérard2018), Ito, Matsumoto, and Quinones (Reference Ito, Matsumoto and Quinones2007), and Tambo and Mockshell (Reference Tambo and Mockshell2018) reported that farmers fail to realize all benefits from CA under partial adoption.
CA adoption dynamics in sentinel sites
Full CA adoption estimated at 31% overall, 57% in the treatment areas, and 7% in the control areas for at least 2 years in this study stands out from the literature. In the whole of Malawi, these numbers are much lower. For example, a recent study by Tufa et al. (Reference Tufa, Kanyamuka, Alene, Ngoma, Marenya, Thierfelder, Banda and Chikoye2023) that also included parts of Nkhotakota district estimates full CA adoption in Malawi at 2%. There are several reasons for the disparities. We highlight two. First, our study uniquely and randomly sampled households for interview from actual villages known to have had contact with host farmers rather than sampling of random villages that are known or not known to have had CA exposure. The later approach was used by Tufa et al. (Reference Tufa, Kanyamuka, Alene, Ngoma, Marenya, Thierfelder, Banda and Chikoye2023). Second, our study focused on sentinel sites within Nkhotakota district where CA has been consistently promoted for more than 15 years by a technically sound NGO (Total LandCare). As such, our site selection is better able to give a long-term perspective of the effects of CA promotion on adoption. Our estimates have very strong internal validity but are weak on external validity; hence, these estimates cannot be generalized to Malawi as a whole but should be related to the Nkhotakota district.
Despite our targeted surveys in sentinel sites, our adoption estimates are not 100% and there is an appreciable adoption decay, implying that more work is needed to reframe and re-align promotion approaches to further increase CA adoption among smallholder farmers in southern Africa. However, this is not unique to CA as other improved agriculture technologies such as crop diversification, agroforestry, and even mineral fertilizers face the same challenges and are rarely adopted in full and on 100% of the land area in Africa (Thierfelder et al., Reference Thierfelder, Baudron, Setimela, Nyagumbo, Mupangwa, Mhlanga, Lee and Gérard2018). Our results suggesting the existence of an adoption decay are profound, showing declines in adoption from highs of 57 and 7% for adoption for at least 2 years for treatment and control, respectively, to 12% in the treatment group and practically zero in the control when we condition full CA adoption to at least 7 years. This suggests the preponderance of dis-adoption in line with previous literature, e.g., Arslan et al. (Reference Arslan, Mccarthy, Lipper, Asfaw and Cattaneo2014) suggest a need for incentives to induce initial adoption and to sustain it. Such incentives could be payments for environmental services or preferential treatment in fertilizer support programs if CA systems were adopted (Ngoma et al., Reference Ngoma, Angelsen, Jayne and Chapoto2021). An example of such a scheme that has drastically improved the uptake of CA is the Pfumvudza program in Zimbabwe which makes receipt of input subsidies conditional on implementing some elements of CA (Mavesere and Dzawanda, Reference Mavesere and Dzawanda2022).
Determinants of non-adoption and dis-adoption of CA
The study revealed that many farmers from the control group did not adopt or dis-adopted CA farming system at some point. These farmers reported socioeconomic, financial, and technical constraints to CA adoption or reasons for dis-adoption, like CA demanding more labor in the initial years of adoption for weed control. These findings conformed to what was reported by other studies (Ngoma et al., Reference Ngoma, Angelsen, Jayne and Chapoto2021; Habanyati, Nyanga, and Umar, Reference Habanyati, Nyanga and Umar2020; Ngwira, Aune, and Thierfelder, Reference Ngwira, Aune and Thierfelder2014a; Ngwira et al., Reference Ngwira, Johnsen, Aune, Mekuria and Thierfelder2014b) that farmers that perceived CA to be labor intensive were likely to dis-adopt or never adopt the technology at all. In line with the FGDs revelations, Habanyati, Nyanga, and Umar (Reference Habanyati, Nyanga and Umar2020) reported that households with large household sizes were more likely to adopt CA because productive labor was available for weeding. During the FGDs, one farmer from Zidyana EPA reported that ‘mulching required more labor, time, and skills especially when laying out crop residues’. This is further complicated by limited availability of biomass that can be used as crop residues to cover entire CA fields.
Farmers in the FGDs reported that high costs of herbicides and pesticides accelerated CA dis-adoption in the study area. Similarly, studies by Thierfelder et al. (Reference Thierfelder, Rusinamhodzi, Setimela, Walker and Eash2016b) and Tsegaye et al. (Reference Tsegaye, LaRovere, Mwabu and Kassie2017) reported that weed control was an important challenge during the early years of CA adoption and Thierfelder et al. (Reference Thierfelder, Mhlanga, Ngoma, Marenya, Matin, Tufa, Alene and Chikoye2024) identify weeds and weed control as the Achilles heel of CA adoption and one where several questions remain unanswered and some answers unquestioned. Tufa et al. (Reference Tufa, Kanyamuka, Alene, Ngoma, Marenya, Thierfelder, Banda and Chikoye2023) report that weeding is the foremost farm operation for which farmers in southern Africa face labor shortages. Thus, farmers may need support in the early years of introducing CA. For example, Ito, Matsumoto, and Quinones (Reference Ito, Matsumoto and Quinones2007) found that farmers were willing to adopt CA if herbicides were accessible. One key informant from TLC indicated that the provision of herbicides had been a crucial impact of the TLC CA project activities. However, Muoni et al. (Reference Muoni, Rusinamhodzi, Rugare, Mabasa, Mangosho, Mupangwa and Thierfelder2014) and Thierfelder et al. (Reference Thierfelder, Mhlanga, Ngoma, Marenya, Matin, Tufa, Alene and Chikoye2024) warn that herbicides may not be affordable for most smallholder farmers and may have undesirable effects on human health and the environment. As such, there is need to explore alternative weed control measures such as biological control (e.g., use of cover crops, microorganisms, integrated weed control) and mechanical weed control (Lee and Thierfelder, Reference Lee and Thierfelder2017).
The lack of technical support to implement CA largely due to poor agriculture extension support systems is another important barrier to adoption (Thierfelder et al., Reference Thierfelder, Bunderson, Jere, Mutenje and Ngwira2016a, Reference Thierfelder, Rusinamhodzi, Setimela, Walker and Eash2016b). Since CA is more complex, capacity building of both farmers and extension agents can therefore foster adoption and implementation of CA. This reinforces that farmer-to-farmer approaches through host farmers could complement other sources of extension to foster adoption. Readers should keep in mind that our study focused on sentinel sites within Nkhotakota district where CA has been consistently promoted for over 15 years. As such, these estimates have high internal validity but low external validity; hence, they cannot be generalized to other parts beyond the study sites.
Conclusion
The devastating effects of climate change on agriculture and livelihoods are more visible now than ever before and they are projected to worsen. Smallholder farming systems need to adapt to the vagaries of climate change to remain productive. Sustainable intensification practices such as CA are promising options. However, lack of widespread adoption despite large investments has led to conclusions that CA systems are unsuitable for smallholder farmers in southern Africa. Sentinel sites where CA has been promoted for a long time, such as Nkhotakota district of Malawi, offer different perspectives because of the perceived high adoption in such areas. Since this high adoption in CA hotspots is hyperlocal and is not ubiquitous, it raises questions and interest to understand what could have led to the perceived success of CA promotion in such areas to inform scaling elsewhere.
This paper took a deep dive at Nkhotakota district of Malawi to study CA adoption dynamics in Mwansambo and Zidyana Extension Plannind Areas (EPAs) for the treatment areas, and Mtosa EPA for the control area. While using survey data from 620 farmers, the study addressed three related questions: (i) what are the main factors that explain the extent and intensity of CA adoption among smallholder farmers; (ii) what are the main benefits associated with CA adoption that motivated farmers to switch from conventional farming practices to CA? and lastly (iii) to what extent does the host farmer approach explain the surge in CA adoption in Nkhotakota district? It is important for readers to keep in mind that our estimates are based on a random sample of farmers who are selected from villages with known contacts with host farmers.
In general, we found that 31% of the surveyed smallholder farmers adopted full CA over at least a 2-year period but there were strong differences between treatment and non-treated areas. About 57% of the farmers in the treatment area adopted full CA, compared to only 7% of farmers in the control area, implying a positive role of the host farmer approach. The adoption estimates from sentinel sites in this paper are much higher than is often reported and highlight the value add of targeted adoption studies.
The main factors that enhanced adoption of CA among farmers in the treatment area were availability of training, extension and advisory services, and demonstration plots by the host farmers. This suggested that CA uptake could be enhanced with increased, targeted, and long-term promotion efforts that include demonstration plots. Thus, in addition to perceived and realized benefits of CA adoption, including enhanced yield, improved soil health, and reduced labor demand during land preparation, the host farmer approach played a critical role in accelerating and strengthening the adoption of CA. However, overall, a larger number of farmers did not adopt full CA, suggesting there are still existing challenges to full CA adoption, namely, (i) labor bottlenecks during weeding, (ii) high cost of herbicides, (iii) lack of knowledge, and (iv) inadequate technical know-how on CA. We also confirm the existence of an adoption decay where the proportion of CA adopters reduces with the length of continuous adoption, suggesting the preponderance of dis-adoption.
Based on the results, we propose three policy recommendations to accelerate the adoption and implementation of CA: first, there is need to continue promoting CA using farmer-centric approaches more consistently, e.g., the host farmer approach. Using a farmer-centered approach facilitates experiential learning and can serve as a motivation for peer-to-peer exchange and learning and can reduce misinformation. The host farmer approach can be strengthened by hosting mega-demonstrations to show farmers that CA can be implemented at scale. This can further strengthen extension delivery to farmers and build trust among farmers on specific CA practices. Leveraging digital technologies for rapid and mass extension delivery is key. Second, CA promotion should allow farmers time to experiment with different CA options before adoption. A key policy question relates to the kinds of support needed in this trial-and-error period. For example, herbicides should be provided to help farmers deal with the weed pressure soon after converting from full to minimum tillage, but this will need to be complemented with training on how to use herbicides correctly to reduce side effects on human health and the environment. Other input support that may be needed in the trial stage include inputs like mechanical rippers where animal traction is unavailable, direct seeding implements, and improved leguminous crop cultivars. How to offer such support without creating economic dependence will be the work of coming years and decades. Lastly, there is need to build and strengthen farmer groups to facilitate easy access to inputs such as leguminous crop seeds for farmers practicing CA. Moreover, these farmer groups could be used as one way of sharing knowledge and information about the merits and demerits of CA and could also be used as conduits to operationalize incentive schemes such as payments for environmental services.
Acknowledgement
The authors would like to acknowledge the support provided by the officials from the Government of Malawi, Nkhotakota District Agricultural Office, Total LandCare, and the farmers from Mwansambo, Zidyana, and Mtosa EPAs. This study would not have been possible without their generous support. Particularly, households who provided access to their information, both in groups and individual interviews on the status of conservation agriculture in Nkhotakota district. We thank several research assistants who facilitated data collection during fieldwork, with enumeration leadership from Lumbani Banda, Gloria Chigona, and Madalitso Nkhata. We thank the editor and two anonymous reviewers for very constructive comments that have helped improve the paper.
Funding statement
The authors would like to recognize financial support provided by USAID through the Africa Research in Sustainable Intensification for the Next Generation (Africa RISING, AID-BFS-G-11–00002) project which funded a consortium of partners and was implemented in Nkhotakota by the International Maize and Wheat Improvement Center (CIMMYT) and national partners. Further support was received by the CGIAR Regional Research Initiative Ukama/Ustawi Diversification for resilient agribusiness ecosystems in East and Southern Africa (ESA) funded by a consortium of CGIAR funding partners and from USAID through the Southern Africa Accelerated Innovation Delivery Initiative (AID-I), Rapid Delivery Hub. Findings, interpretations, and conclusions expressed in this paper are entirely those of the authors and do not necessarily represent the views of USAID, CIMMYT, Total LandCare, or other partners.
Competing interests
None.
Ethical clearance
Not required at the time.