Non-communicable diseases are a growing concern in sub-Saharan Africa (SSA). In this region, the number of cardiovascular deaths has nearly doubled from 1990 to 2015( Reference Mensah, Roth and Sampson 1 ), the prevalence of diabetes has more than doubled from 1980 to 2014( 2 ) and the prevalence of hypertension and obesity are both increasing( 3 , Reference Danaei, Finucane and Lin 4 ). In rural SSA, the proportion of adult deaths due to non-communicable diseases has increased: from 16 % in 2003 to 24 % in 2007 in Tanzania( Reference Narh-Bana, Chirwa and Mwanyangala 5 ) and from 35 % in 2003 to 45 % in 2010 in Kenya( Reference Phillips-Howard, Laserson and Amek 6 ).
One of the potentially modifiable risk factors for non-communicable diseases is diet, which is well documented to affect the risk for many cardiometabolic diseases and cancers( Reference Reedy, Krebs-Smith and Miller 7 – Reference Sotos-Prieto, Bhupathiraju and Mattei 10 ). In SSA, although data remain sparse, it is clear that access to food is increasing but diet quality is worsening. Data from the FAO show that per capita energy intake has increased over the past 30 years( Reference Steyn and McHiza 11 ), snack food and soft drink importation to the fifteen countries that make up the Southern African Development Community has quadrupled over the past two decades( Reference Thow, Sanders and Drury 12 ), and overall dietary quality has worsened( Reference Imamura, Micha and Khatibzadeh 13 ). Reflecting the consequences of the worsening dietary situation in SSA, the Global Burden of Disease study estimates that the proportion of deaths attributable to unhealthy diet in Tanzania has increased from 5·8 % in 1990 to 9·3 % in 2015( 14 , Reference Forouzanfar, Alexander and Anderson 15 ).
As the burden of non-communicable diseases in SSA continues to grow, more research is needed to discover the causes of this rising burden and help governments develop prevention policies. Well-designed public health policies could influence diet through such means as education to inform and change food preferences, healthy food production, food subsidization and taxation( Reference Rees, Dyakova and Wilson 16 – Reference Afshin, Penalvo and Del Gobbo 19 ). However, obtaining reliable information on dietary intake can be challenging because validated tools for quantifying diet in SSA are limited. FFQ are the standard tool used to estimate dietary intake for diet–disease analyses in large cohorts( Reference Willett 20 ). Such questionnaires, which were developed in the 1980s, have been widely used by researchers in the USA and Europe. However, as the validity of FFQ is sensitive to cultural and regional factors( Reference Willett 20 – Reference Cade, Thompson and Burley 22 ), FFQ need to be validated before being extended for use outside the USA and Europe, such as in SSA where diets, availability of foods and dishes, portion sizes, numeracy, literacy and customs differ from those in the USA and Europe( Reference Gibson, Knight and Asante 23 , Reference Mattei, Malik and Wedick 24 ). Within SSA, full-length FFQ intended to measure the entire diet have been validated for use in Mali, South Africa and Botswana( Reference Parr, Barikmo and Torheim 25 – Reference Jackson, Motswagole and Kwape 29 ). However, diets differ between SSA countries, and an FFQ has not yet been validated for use in Tanzania. A previous validation of a Tanzanian FFQ against two 24 h diet recalls provided only correlation coefficients and P values for six broad food groups (fruits, cereals, beverages, vegetables, animal products, fats) based on data from fifty women( Reference Jordan, Hebestreit and Swai 30 ). Here, we report the validity of estimated intakes of energy, twenty-five nutrients and thirteen food groups as assessed by our FFQ compared with two repeated 24 h diet recalls among adults in Dar es Salaam, Tanzania.
Methods
Sampling design and participants
The Dar es Salaam Health and Demographic Surveillance System (HDSS) was initiated in 2011 and collected demographic data on all individuals living in Ukonga, a peri-urban ward of Dar es Salaam( Reference Leyna, Berkman and Njelekela 31 ). For the Dar es Salaam Urban Cohort Hypertension Study (DUCS-HTN), we attempted to contact everyone registered in the HDSS, aged 40 years or older, from two randomly selected neighbourhoods of the seven neighbourhoods in the HDSS (n 4896). Additional information on the DUCS-HTN has been reported previously( Reference Zack, Irema and Kazonda 32 ). We randomly selected 1024 of these potential participants to contact for the DUCS-HTN dietary sub-study. We excluded participants who were pregnant or physically or mentally incapable of participating in the DUCS-HTN. For inclusion in the analytical sample, we required that participants complete an FFQ and two 24 h diet recalls, have fewer than 10 % of their FFQ items missing and that their daily energy intake from the FFQ be within a plausible range of 2092–20 920 kJ (500–5000 kcal).
The Institutional Review Board of the Harvard T.H. Chan School of Public Health and the Muhimbili University of Health and Allied Sciences Ethical Committee approved the study protocol. Written informed consent was obtained from all participants; or, if the participant was unable to sign, a witness signed on behalf of the participant.
Dietary assessments
Trained interviewers conducted face-to-face interviews and physical examinations in participants’ homes from March to June 2014. The six interviewers who conducted dietary questionnaires had experience in public health data collection, but not in collecting FFQ or 24 h diet recalls. The interviewers therefore received one full day of training and written instructions on how to conduct the FFQ and 24 h diet recall. A nutritionist and epidemiologists conducted the dietary data collection training. Interviewers had two days of field practice before commencing data collection, during which supervisors observed interviews and provided feedback to the interviewers to standardize the interview technique between the six interviewers. Interviewers administered the FFQ and the first 24 h diet recall at the first study visit. All interviews and examinations proceeded in the same order: lifestyle questionnaire, blood pressure measurements, FFQ, anthropometric measurements, 24 h diet recall, capillary blood measurements and finally provision of instructions for the 24 h urine collection. A second 24 h diet recall was repeated at the start of the next study visit, a minimum of three days later. The median number of days between the two 24 h diet recalls was 6 (interquartile range 4–15). Eighty-four per cent of participants completed both 24 h diet recalls during the workweek, 15 % completed one on the weekend and one during the week, and 2 % completed both during the weekend.
Interviewers verbally administered the 24 h diet recall to participants. To help standardize participants’ understanding of serving size, the interviewers showed participants plastic sample dishes as well as drawn images of portion sizes (see images in the online supplementary material) for the 24 h diet recalls and the FFQ.
Interviewers verbally administered the 179-food-item semi-quantitative FFQ with specified portion sizes and a recall period of 30 d. The FFQ was slightly modified, through the addition of food items, from the FFQ created by Lukmanji et al.( Reference Lukmanji, Hertzmark and Spiegelman 33 ). Similar FFQ without published validation studies have previously been used in Tanzania( Reference Abioye, Isanaka and Liu 34 ). There were nine options for frequency of consumption, with servings per day calculated from these frequencies as follows: ‘never consumed over past thirty days’ (0 servings/d), ‘1–3 servings per month’ (‘0·067 servings/d), ‘1 serving per week’ (0·143 serving/d), ‘2–4 servings per week’ (0·429 servings/d), ‘5–6 servings per week’ (0·786 servings/d), ‘1 serving per day’ (‘1 serving/d), ‘2–3 servings per day’ (2·5 servings/d), ‘4–5 servings per day’ (4·5 servings/d) and ‘6+ servings per day’ (6 servings/d). Participants selected one frequency of consumption for each of the 179 items in the FFQ. Portion sizes were provided for each food item (see FFQ in the online supplementary material); however, participants could report that they consumed a fraction of a serving size (e.g. ½ or ¼).
For both the 24 h diet recall and FFQ data, nutrient information for individual foods was taken from the 2008 Tanzania Food Composition Tables( Reference Lukmanji, Hertzmark and Mlingi 35 ). These food composition tables were based primarily on data from WorldFood Dietary Assessment System, with some information also taken from the US Department of Agriculture’s food composition database and the South Africa food composition tables. Chemical analysis of the nutrient composition of a small number of foods (mainly maize and some leafy greens) was conducted at Sokoine University of Agriculture. The Tanzanian Food Composition Tables include nutrient composition of mixed dishes. The authors of the Tanzanian Food Composition Tables collected sample recipes from a variety of sources (e.g. street food vendors, student canteen, recipe books and websites). The nutrient composition of the recipe was then estimated from the nutrient composition of the individual ingredients. These tables provide information on the energy and nutrient contents and grams per serving size of over 400 foods and dishes commonly consumed in Tanzania. Nutrient intakes for each individual included contributions from foods but not supplements.
Statistical analysis
Descriptive statistics (means and sd) were calculated for energy, nutrient and food group intakes as estimated by the FFQ and the average of the two 24 h diet recalls. Foods were categorized into thirteen groups (see online supplementary material, Supplemental Table 1) for food group analyses. Nutrient intakes were adjusted for total energy intake using the residual method( Reference Willett and Stampfer 36 ). We also adjusted for total energy intake when analysing macronutrient intakes by calculating macronutrient intakes as a percentage of total energy intake (i.e. the nutrient density method). Fats, carbohydrates and protein were separately adjusted for total energy intake using the nutrient density method.
Table 1 Basic characteristics of Dar es Salaam Urban Cohort Hypertension Study (DUCS-HTN) participants, 2014 (n 317)

Rank correlation coefficients and 95 % CI were calculated to evaluate the strength of the associations between nutrient and food group intakes derived from the FFQ v. the average of the two 24 h diet recalls( Reference Rosner and Glynn 37 ). To compare nutrient intakes as measured by the two different dietary assessment methods, we calculated unadjusted, energy-adjusted and deattenuated energy-adjusted correlation coefficients. To compare food group intakes as measured by the two different dietary assessment methods, we calculated unadjusted and deattenuated correlation coefficients. Deattenuated correlations were used to adjust for random within-person variation across the 24 h diet recalls( Reference Rosner and Glynn 37 ). We also calculated intraclass correlations between nutrient and food group intakes as measured by the FFQ and average of the two 24 h diet recalls.
We examined the ability of the two dietary questionnaires to categorize participants into the same energy-adjusted nutrient intake quartiles. The proportions of individuals who were classified correctly within the same quartile, within the same or adjacent quartile, and in opposite quartiles (lowest quartile according to one dietary questionnaire and highest quartile according to the other) were determined. Kappa statistics with linear weights were calculated to further quantify the agreement between energy-adjusted nutrient intake quartiles as measured by the FFQ and average of two 24 h diet recalls( Reference Cohen 38 , Reference Cicchetti and Allison 39 ).
We examined systematic differences in macronutrient intakes as measured by the FFQ and 24 h diet recalls by creating Bland–Altman plots of energy intake, percentage of energy from fat, percentage of energy from protein and percentage of energy from carbohydrates( Reference Bland and Altman 40 ). The plots were created by plotting the difference in nutrient intake from the two dietary intake measurement methods against the mean of the nutrient intake from the two dietary intake measurement methods. A relationship between the difference and the mean values indicates systematic bias (e.g. the FFQ tends to overestimate nutrient intake more for those with higher total energy intakes). Limits of agreement (mean(nutrientFFQ – nutrient24hDR)±1·96×sd(nutrientFFQ – nutrient24hDR)) were calculated and plotted.
Analyses were performed using the statistical software package SAS version 9.3. Figures were created using R version 2.15.3.
Results
Among the 1024 participants whom we attempted to contact for the DUCS-HTN dietary sub-study, 265 were not home after three contact attempts, 239 had out-migrated, forty-two had died, fifteen were mentally or physically incapable of participating, thirty-six refused to participate, two were pregnant and ten did not enroll for unknown reasons (Fig. 1), resulting in a sample of 415 participants. Of these individuals, 414 completed the FFQ and 357 also completed the two 24 h diet recalls. None of the FFQ had missing data on more than 10 % of FFQ food items. We excluded one participant who, according to the FFQ, consumed <2092 kJ/d (<500 kcal/d), as well as thirty-nine participants who consumed >20 920 kJ/d (>5000 kcal/d), which led to an analytical sample of 317 participants. Among these, the median age was 52 (interquartile range 45–60) years, 61 % of participants were female, 65 % were overweight or obese, and 75 % had less than secondary education (Table 1).

Fig. 1 Flowchart of study participation and data completeness in the Dar es Salaam Urban Cohort Hypertension Study (DUCS-HTN), 2014; which was a sub-sample of participants from the Dar es Salaam Health and Demographic Surveillance System (HDSS), 2011
The mean daily energy, nutrient and food intakes estimated by the FFQ were higher than those estimated by the average of the two 24 h diet recalls (Table 2). This was true for all nutrients and all foods, other than tea. Total energy intake as estimated by the FFQ was 1·68 times that estimated by the average of the two 24 h diet recalls: mean of 10 874 (sd 4310) kJ (2599 (sd 1030) kcal) according to the FFQ and 6468 (sd 1803) kJ (1546 (sd 431) kcal) according to the average of the two 24 h diet recalls. The ratio of nutrient intake comparing FFQ estimates with 24 h diet recall estimates ranged from 1·34 for SFA to 7·08 for vitamin A. Among food groups, the ratio of consumption comparing FFQ estimates with 24 h diet recall estimates ranged from 0·92 for tea to 9·00 for fruits.
Table 2 Daily food and nutrient intakes estimated by the FFQ and two 24 h diet recalls in the Dar es Salaam Urban Cohort Hypertension Study (DUCS-HTN), 2014 (n 317)

RAE, retinol activity equivalents.
The intraclass correlations between the FFQ and average of the two 24 h diet recalls for nutrients ranged from 0·09 for vitamin A to 0·38 for total energy and for food groups it ranged from 0·12 for vegetables (excluding roots) to 0·58 for tea. Energy adjustment tended to reduce correlations and deattenuation tended to increase correlations (Table 3). The median deattenuated energy-adjusted correlation for nutrients was 0·21 and ranged from −0·03 for riboflavin to 0·41 for percentage of energy from carbohydrates. Macronutrients when measured as a percentage of total energy intake (i.e. the nutrient density method) had higher correlations than did macronutrients adjusted for energy using the residual method: 0·41 v. 0·25 for carbohydrates, 0·40 v. 0·22 for protein and 0·36 v. 0·15 for fat. The median deattenuated correlation for food groups was 0·35 and ranged from 0·00 for root vegetables to 0·51 for alcohol.
Table 3 Correlations and intraclass correlations (ICC) of daily intakes of nutrients and food groups as assessed with the average of two 24 h diet recalls and the FFQ in the Dar es Salaam Urban Cohort Hypertension Study (DUCS-HTN), 2014 (n 317)
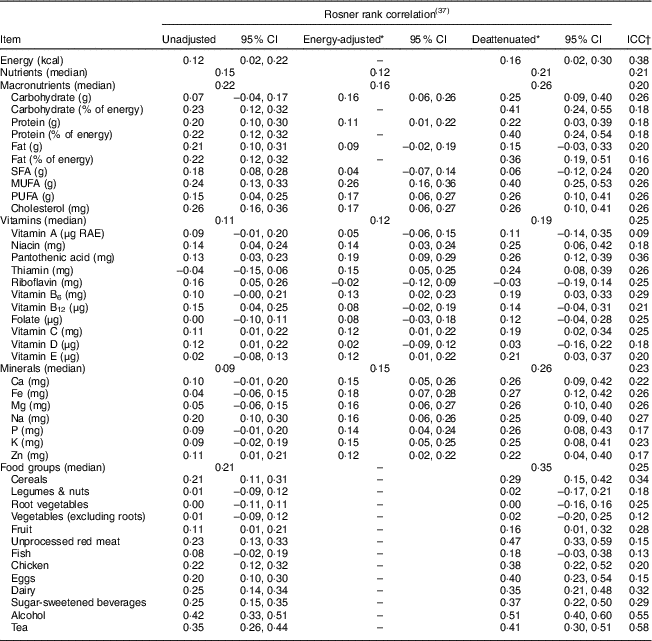
RAE, retinol activity equivalents.
* Nutrient intakes, but not food group intakes, were adjusted for total energy intake by the residual method.
† ICC for residual method energy-adjusted nutrients except for energy and ‘percentage of energy’ variables.
Cross-classification analyses of estimated nutrient intakes found low to moderate agreement (Table 4). The median proportion classified within the same quartile was 29 %, which is higher than the 25 % that is expected due to chance alone. The proportion correctly classified within the same quartile ranged from 23 % for SFA to 32 % for niacin and pantothenic acid. The proportion classified within the same or adjacent quartiles ranged from 60 % for riboflavin to 72 % for MUFA. The proportion classified into opposite quartiles (first quartile according to one questionnaire and fourth quartile according the other) ranged from 7 % for percentage of energy from protein to 13 % for total energy, cholesterol, vitamin A and riboflavin. The median proportion classified into opposite quartiles was 10 %, which is the same as what would be expected by chance. Weighted kappa values ranged from −0·03 for riboflavin to 0·14 for niacin and pantothenic acid.
Table 4 Cross-classification of energy-adjusted daily intakes of nutrients and food groups in quartiles as assessed with the average of two 24-h diet recall and the FFQ in the Dar es Salaam Urban Cohort Hypertension Study (DUCS-HTN), 2014 (n 317)

RAE, retinol activity equivalents.
Bland–Altman plots of energy and percentage of energy from the three major macronutrients show moderate agreement (Fig. 2). The plots also show potential systemic bias in energy intake because the difference in energy intake between the FFQ and 24 h diet recalls is larger among participants with higher mean reported energy intake. However, systematic bias was not seen in percentage energy from fat, protein or carbohydrates. Ranges for limits of agreement were relatively wide, which indicates that there was wide variability in how the FFQ measured macronutrient intake relative to the average of the two 24 h diet recalls.

Fig. 2 Bland–Altman plots to assess agreement between the FFQ and the average of two 24 h diet recalls (24 hDR) for (a) energy (to convert to kJ, multiply kcal values by 4·184), (b) fat (percentage of energy), (c) carbohydrates (percentage of energy) and (d) protein (percentage of energy) in the Dar es Salaam Urban Cohort Hypertension Study (DUCS-HTN), 2014 (n 317)
Discussion
Our results indicate moderate validity of the Tanzanian FFQ when compared with two 24 h diet recalls in an urban adult population in Dar es Salaam. As has been observed in previous validation studies, we found that the FFQ generally overestimated intakes relative to the 24 h diet recalls( Reference Parr, Barikmo and Torheim 25 , Reference Jackson, Motswagole and Kwape 29 , Reference Bohlscheid-Thomas, Hoting and Boeing 41 – Reference Rodríguez, Méndez and Torún 44 ) and deattenuation tended to increase estimated correlation coefficients( Reference Longnecker, Lissner and Holden 45 ). Coefficients comparing estimated nutrient intakes from the FFQ and two 24 h diet recalls were low to moderate. We found that the FFQ performed best for macronutrients when quantified as a percentage of energy intake, but less well for nutrients adjusted for energy intake using the residual method. The FFQ appeared to be a better measure of macronutrients and minerals than of vitamins. This may be because vitamin intake tends to vary greatly from day to day (as many vitamins are found in only a small selection of foods), whereas macronutrient intake remains relatively constant.
The validity of the FFQ to measure food group intakes was also moderate. However, we found that the FFQ has poor validity for measuring legume and nut intake, and vegetable (both root vegetables and other vegetables) intake. The poor validity of the FFQ for these food groups may be due to the difficulty of quantifying the intake of foods that tend to be a component of a dish, such as vegetables added to stews. This can be particularly challenging in Tanzania, where vegetables are often consumed in mixed dishes along with many other vegetables, and possibly meat or fish, rather than independently. Tanzanian meals are often composed of a starch (i.e. rice or stiff porridge (called ugali in Swahili)) and a stew, making it difficult for individuals to assess the quantity of each component of the food. An additional challenge in Tanzania and elsewhere in SSA is that food is often consumed communally, from a shared household dish rather than from individual plates, which complicates estimating portion size, as well as portion content. To account for this, we included ‘handful’ (ujazo wa kiganja cha mkono in Swahili) as a serving size option in the FFQ.
Previous FFQ validation studies have similarly found that correlations tend to be lower for vegetables than other food groups( Reference Cade, Thompson and Burley 22 , Reference Parr, Barikmo and Torheim 25 , Reference Rodríguez, Méndez and Torún 44 , Reference Cade, Burley and Warm 46 – Reference Haftenberger, Heuer and Heidemann 48 ). A cross-check question on the daily number of servings of vegetables could be added to future versions of the FFQ to help correct for over-reporting of vegetable intake( Reference Cade, Thompson and Burley 22 ).
Our results are similar to those from previous FFQ validations that have been conducted in SSA. We identified four full-length FFQ that have been validated for use in SSA populations: (i) a 164-item quantitative FFQ with a recall period of 7 d was validated against 2 d weighed food records using data from seventy participants in Mali; (ii) a 122-item FFQ was validated against four 24 h diet recalls using data from seventy-nine participants in Botswana; (iii) a 145-item FFQ was validated against a 7 d weighed food record using data from seventy-four participants in South Africa; and (iv) a quantitative FFQ was validated against two 24 h diet recalls using data from fifty women in Tanzania (information was not provided on number of food items)( Reference Parr, Barikmo and Torheim 25 , Reference MacIntyre, Venter and Vorster 27 , Reference Jackson, Motswagole and Kwape 29 , Reference Jordan, Hebestreit and Swai 30 ). We found a median correlation of 0·35 for food groups, which is comparable to median correlations of 0·28, 0·8 and 0·37 that were found in Mali, Botswana and South Africa, respectively. Our food group correlations ranged from 0·00 to 0·51, compared with correlations ranging from −0·04 to 0·56, 0·18 to 0·58 and 0·14 to 0·56 in Mali, Botswana and South Africa, respectively.
For most nutrients and food groups that we examined, we found relatively similar population-level intakes to what has been found by other researchers in Tanzania. Lukmanji et al. found a similar distribution of macronutrient intakes in a study of pregnant women with HIV in Dar es Salaam( Reference Lukmanji, Hertzmark and Spiegelman 33 ). A global study estimated that sugar-sweetened beverage intake in Tanzanians aged 40 years or older ranged from 0·16 to 0·32 servings/d, depending on age and sex( Reference Singh, Micha and Khatibzadeh 49 ). This is similar in range to our findings of 0·2 servings/d, according to the FFQ, and 0·1 servings/d, according to the 24 h diet recalls. However, we also observed some intake values that differed considerably from previous studies. For example, a global study estimated that, on average, Tanzanians consume 2·75 (95 % CI 2·45, 3·08) g Na/d( Reference Powles, Fahimi and Micha 50 ), which is more than double the intake of 0·96 g/d estimated from our FFQ and 0·38 g/d estimated from our 24 h diet recalls.
Our study had several limitations. We used two 24 h diet recalls as our reference method instead of several weighed food records. Our 24 h diet recalls may have underestimated dietary intake due to multiple factors including under-reporting due to recall bias (e.g. forgot to report snacks or small food items) and under-representativeness of weekend diet (which is often larger than weekday diet). Other studies have found that participants incorrectly report portion sizes on 24 h diet recalls( Reference Korkalo, Erkkola and Fidalgo 51 ) and a meta-analysis of FFQ validation studies found that correlations of nutrient intakes were lower for FFQ validated against 24 h diet recalls rather than food records( Reference Molag, de Vries and Ocké 52 ). However, weighed food records may affect participants’ behaviour and it is not possible to conduct food records in populations that have low literacy levels such as ours, unless an interviewer observes and records every meal, as was done for the FFQ validation in Mali( Reference Parr, Barikmo and Torheim 25 ). The meta-analysis of FFQ validation studies also found that nutrient correlations were lower when the reference method of dietary questionnaire was conducted fewer than eight times( Reference Molag, de Vries and Ocké 52 ). We conducted two 24 h diet recalls per participant due to financial and logistic constraints. Further factors that may have lowered the agreement and correlation between our FFQ and the reference method were the limited training received by the study interviewers in how to conduct dietary questionnaires (i.e. one full-day session and two days of field tests) and questionnaire fatigue, for both participants and interviewers, due to the lengthy questionnaires conducted prior to the dietary questionnaires. The non-dietary DUCS-HTN questionnaire and measurements took approximately 1 h to complete and each dietary questionnaire took 30–45 min to complete.
Our FFQ queried participants about many individual foods. A future study could include questions on frequency of consumption of food groups in addition to questions on individual food items. This may help quantify whether asking about individual foods overestimates the food group consumption. It is possible that an FFQ that focused on the most common mixed dishes and amount and types of sauces added to dishes, rather than asking mainly about individual food items, might improve dietary assessment in this population.
In summary, our results indicate moderate agreement between the FFQ and two 24 h diet recalls for use in urban Tanzania. Our results could be used to inform the creation and implementation of improved dietary questionnaires for use in SSA. Future FFQ validation studies may wish to examine the effect of intensity of interviewer training on FFQ validation quality. In addition, future studies might conduct FFQ validations using more than two 24 h diet recalls spaced out over a longer period of time as the reference method.
Acknowledgements
Acknowledgements: The authors would like to thank Dr Ramadhani Abdallah Noor for his feedback on the FFQ and Stella Lawrence for her help in training interviewers on conducting dietary questionnaires. They would also like to acknowledge participants who generously gave their time and invited interviewers to their homes. This project would not have been possible without the hard work of many interviewers, data entry clerks, administrative staff and drivers. Their enthusiasm and hospitality made this project possible and a pleasure to work on. Financial support: This work was supported by a pilot grant through the Center for the Global Demography of Ageing (grant number AG024409). R.M.Z. was supported by a National Institutes of Health T32 training grant (grant number CA 09001). The funders had no role in the design, analysis or writing of this article. Conflict of interest: None. Authorship: R.M.Z., G.D. and J.K. designed the study; K.I., R.M.Z., G.D., P.K. and J.K. led the fieldwork and data collection; R.M.Z. conducted the analysis with input from all co-authors; R.M.Z. wrote the first draft of the manuscript and all co-authors provided comments and input; G.D. had primary responsibility for final content. All authors read and approved the final manuscript. Ethics of human subject participation: The study protocol was approved by the Institutional Review Board of the Harvard T.H. Chan School of Public Health and the Muhimbili University of Health and Allied Sciences Ethical Committee. All participants provided written informed consent; if the participant was unable to sign, a witness signed on behalf of the participant.
Supplementary material
To view supplementary material for this article, please visit https://doi.org/10.1017/S1368980018000848