During recent decades dietary recommendations in most Western countries, including Europe and the USA, have been similar and aimed at improving longevity and overall health of their populations(1–3). Research on diet and health underlying dietary recommendations has traditionally focused on the effect of individual foods or nutrients, but in recent years dietary pattern analysis has emerged as a complementary approach(Reference Hu4). Studying dietary patterns in relation to mortality is one way of evaluating the overall health effects of adherence to general dietary recommendations. Hypothesis-oriented dietary patterns, or dietary indices, have been associated with overall mortality(Reference Huijbregts, Feskens and Räsänen5–Reference Kant, Schatzkin and Graubard8), CVD(Reference McCullough, Feskanich and Stampfer9, Reference Alberti, Fruttini and Fidanza10) and cancer(Reference Fung, Hu and Wu11–Reference Reedy, Mitrou and Krebs-Smith13). In a previous study, we developed a diet quality index (DQI-SNR) that assesses adherence to the 2005 Swedish Nutrition Recommendations (SNR) and the Swedish dietary guidelines (SDG) in the Malmö Diet and Cancer (MDC) cohort(Reference Drake, Gullberg and Ericson14). Results from that study suggested that the DQI-SNR is a useful tool for distinguishing individuals with diets similar to current dietary recommendations, reflected by a wide range of food and nutrient intakes. In accordance with previous studies on the dietary habits of Swedish men and women, participants in the MDC cohort showed low compliance with recommendations for SFA, dietary fibre, fruit and vegetables, vitamin D and folate(Reference Drake, Gullberg and Ericson14, Reference Becker15). There are several methodological issues that need to be addressed when constructing and evaluating a dietary index. Apart from selection of index components, utility is determined by how the scoring model used for calculating the total score is formulated. Little attention has been paid to this issue in previous studies using dietary indices although its importance has been highlighted in recent reviews(Reference Waijers, Feskens and Ocké16–Reference Kourlaba and Panagiotakos18). The objective of the present study was therefore to investigate if adherence to the current nutrition recommendations in Sweden is related to all-cause and cause-specific mortality in a population-based cohort of middle-aged Swedish men and women. Particularly, we aimed to investigate the effect of using different scoring models for the DQI-SNR.
Methods
Study population
The MDC study is a population-based prospective cohort. In 1991, the MDC source population was defined as all persons living in the city of Malmö and born between 1926 and 1945, with Swedish reading and writing skills. In May 1995, the cohort was extended to include all women born between 1923 and 1950 and all men born between 1923 and 1945. With this extension, 74 138 persons constituted the source population. Details of the recruitment procedures and the cohort are described elsewhere(Reference Berglund, Elmståhl and Janzon19, Reference Manjer, Carlsson and Elmståhl20). Collected data included medical history, socio-economics, lifestyle and dietary habits using questionnaires and interview. Direct measurements and blood samples were collected by nurses. In October 1996, when recruitment was closed, 28 449 participants had completed baseline examinations and of these 28 098 participants (11 063 men and 17 035 women) had complete dietary information. The MDC study was approved by the Ethical Committee at the Medical Faculty, Lund University (LU 51-90). In the index development study past food habit change, energy misreporting, having experienced a cardiovascular event and reporting diabetes diagnosis were significantly associated with high DQI-SNR scores(Reference Drake, Gullberg and Ericson14). The underlying assumption in prospective studies with long follow-up is that the exposure (i.e. dietary habits) is stable over time and free of systematic bias. Therefore, the present analysis excluded individuals reporting either to have changed their food habits substantially in the past (n 6849) and/or been previously classified as likely energy misreporters (n 5200)(Reference Mattisson, Wirfält and Aronsson21). Previous studies have indicated that excluding these individuals potentially reduces misclassification bias caused by unstable food habits and measurement errors associated with self-reported dietary data(Reference Mattisson, Wirfält and Aronsson21, Reference Sonestedt, Wirfält and Gullberg22). In addition, dietary guidelines are intended to promote good health among the healthy population. Therefore, individuals who reported a diabetes diagnosis in the baseline questionnaire (and/or use of antidiabetic drugs) and/or individuals with a history of cardiovascular event (myocardial infarction and stroke identified through national registers) were also excluded. In total, 10 964 individuals were excluded from the study and the final study population included 6940 men (aged 45–73 years) and 10 186 women (aged 44–73 years).
Dietary assessment method
Dietary information was collected using a modified diet history method combining a 7 d menu book (collecting descriptions of prepared meals, nutrient supplements and cold beverages) and a 168-item quantitative diet questionnaire using both exact frequencies and a picture booklet to assess portion sizes of regularly eaten foods (other than prepared meals) during the past year. During a 1 h interview, the questionnaire and the menu book were checked so that they did not overlap, and detailed information was collected on cooking practices and recipes. Energy and nutrient intakes were computed from the reported food intake using the MDC Food and Nutrient Database, mainly originating from PC Kost2-93 of the Swedish National Food Administration in Uppsala(Reference Callmer, Riboli and Saracci23). Data on the validity(Reference Elmståhl, Riboli and Lindgärde24, Reference Riboli, Elmståhl and Saracci25) and reproducibility(Reference Elmståhl, Gullberg and Riboli26) of the method have been published. The relative validity coefficients (compared with 14 d of weighed food records) for the dietary variables investigated in the present study range from 0·35 to 0·77 (mean r = 0·59). In September 1994, the coding routines for the dietary assessment were changed slightly in order to reduce interview time. In order to reduce the potential bias caused by this change a categorical variable indicating method version (study entry before or after September 1994) was included in multivariate analysis(Reference Wirfält, Mattisson and Johansson27).
The DQI-SNR
The DQI-SNR has been described in detail previously(Reference Drake, Gullberg and Ericson14). In short, the construction of the index tried to minimize overlap (i.e. correlations) between components while assuring that the total score was positively associated with recommended intakes of both the components and several other foods and nutrients. The index consists of six components: energy percentage (E %) from SFA and PUFA, fish and shellfish (g/week), dietary fibre (g/MJ), fruit and vegetables (g/d) and E % from sucrose. All energy percentages were calculated using non-alcohol energy intake. The different cut-offs and scorings used for the DQI-SNR are presented in Table 1. DQI-SNR Model 1 used predefined cut-offs based on recommended intake levels in the 2005 SNR and the SDG. One standard deviation of the population mean was added to the SFA cut-off since only 2 % of the MDC cohort was below the recommended 10 E %. Since the recommendation for dietary fibre intake (approximately 3 g/MJ or 25–35 g/d) does not provide a cut-off for relative intakes, a similar adjustment was made for the dietary fibre cut-off (±1 sd)(Reference Drake, Gullberg and Ericson14). The cut-offs create dichotomous component variables expressing adherence (1 point) or non-adherence (0 points) to the recommendations. Total score ranged from 0 to 6. DQI-SNR Model 2 used the study population's median energy-adjusted intakes as cut-offs for the components to create dichotomous variables. One point was assigned to individuals above the median intake of PUFA, fish and shellfish, dietary fibre and fruit and vegetables, and one point to individuals below the median intake of SFA and sucrose. Total score ranged from 0 to 6. DQI-SNR Model 3 ranked the individuals into quintiles of energy-adjusted intake of the components to provide a larger score gradient. A proportional score ranging from 1 to 5 was assigned to the different quintile groups, with high scores to individuals with higher intakes of PUFA, fish and shellfish, dietary fibre and fruit and vegetables, and lower scores to individuals with higher intakes of SFA and sucrose. Total scores ranged from 6 to 30.
Table 1 Cut-off criteria and scoring used for the diet quality index (DQI-SNR) in the Malmö Diet and Cancer cohort (n 17126) using predefined cut-offs (DQI-SNR Model 1), median-based cut-offs (DQI-SNR Model 2) and quintile-based cut-offs (DQI-SNR Model 3)
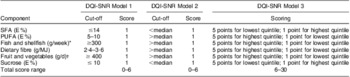
E %, energy percentage.
*Fish and shellfish in g/MJ per week for DQI-SNR Model 2 and DQI-SNR Model 3.
†Fruit and vegetables in g/MJ per d for DQI-SNR Model 2 and DQI-SNR Model 3.
Other variables
Information on socio-economic and lifestyle factors was collected from an extensive questionnaire administered at baseline. Educational level was defined according to the number of years of education completed or degree of educational level attained, i.e. primary (less than 9 years), secondary (9 years), upper secondary (high school degree) or university degree. Socio-economic status was categorized as blue-collar workers, white-collar workers or employers/self-employed according to the Swedish population census(28). Smoking was defined as never, former or current smokers. Leisure-time physical activity was defined by gender-specific tertiles of an activity score(Reference Drake, Gullberg and Ericson14). Cohabiting status was defined as living alone or cohabiting. Alcohol consumption was classified as zero, low, medium or high. Participants reporting no alcohol intake in the 7 d menu book and reporting no alcohol intake during the preceding year in the questionnaire were classified as zero alcohol consumers. For all other participants low, medium and high alcohol consumption level was set at alcohol intakes of <15, 15–30 and >30 g/d for women and <20, 20–40 and >40 g/d for men(29). Trained nurses measured weight (kg), height (m) and waist (cm). Waist was measured midway between the lowest rib margin and iliac crest. BMI was computed as the ratio between weight and height raised to the second power (kg/m2).
Outcome ascertainment
Information on vital status of the cohort was obtained from the Swedish National Death Registry and the National Tax Board. Information about causes of death was obtained from the Swedish Register of Death Causes at the National Board of Health and Welfare. The underlying causes of death (27 % based on autopsy and 69 % on physical examination/inspection) were classified according to the International Classification of Diseases (ICD), ninth and tenth revision (ICD-9 and ICD-10). Using individual civil registration numbers, which are linked to the Swedish Population Registry, the vital statistics were followed from study entry to emigration, death or 31 December 2008, whichever occurred first. The primary end-point for the present study was all-cause mortality and during the mean follow-up time of 14·2 years (in total 2 43 109 person-years of follow-up) 2450 deaths occurred in the study population, 1360 (55·5 %) among men and 1090 among women. The secondary end-points were cause-specific mortality from CVD (ICD9: 390–459; ICD10: I), cancer (ICD9: 140–239; ICD10: C, D00–D48) and other causes. During follow-up, 444 (32·6 %), 647 (47·6 %) and 269 (19·8 %) deaths occurred among men and 265 (24·3 %), 574 (52·7 %) and 251 (23·0 %) deaths occurred among women from CVD, cancer and other causes, respectively. Causes of death other than CVD and cancer were among men mainly injury/external causes (n 59), respiratory diseases (ICD10: J; n 56), digestive diseases (ICD10: K; n 38) and neurological diseases (ICD10: G; n 31) and among women mainly respiratory diseases (n 61), neurological diseases (n 47), injury/external causes (n 37) and digestive diseases (n 36).
Statistical analysis
All analyses were stratified by sex due to differences in eating patterns between men and women and a significant interaction between sex and index score with all end-points. We used Cox proportional hazards regression to examine the independent association of the DQI-SNR with all-cause and cause-specific mortality in the presence of covariates with follow-up time (days) as the underlying time metric. The DQI-SNR score was categorized into low, medium and high scores due to a small proportion of participants with low and high scores. The population distribution across total score using models 2 and 3 was normal and therefore the participants were ranked into gender-specific tertiles of score. The population distribution across total score using Model 1 was however right-skewed, and the participants were therefore divided into approximate tertiles. The risk of death in the two upper categories (medium and high score) was compared with the risk for the lowest category of score. To test the linear trend with mortality outcomes, we entered the DQI-SNR score in regression models as a continuous variable. The proportional hazards assumption was tested by plotting the log-minus-log plot. The covariates in the basic regression model were chosen a priori: method version, season of dietary data collection (spring, summer, autumn, winter), age and total energy intake (log-transformed to normalize distribution). Additional covariates considered for inclusion in the full multivariate model were identified from the literature and included: BMI, waist circumference, education, socio-economic status, smoking status, leisure-time physical activity, alcohol consumption and cohabiting status. Covariates had to modify the risk estimate by more than 10 % or be considered as important confounders by prior knowledge in order to be included in the full model. The full multivariate model thus included, in addition to the a priori selected covariates included in the basic model, smoking status, leisure-time physical activity and alcohol consumption. The statistical software package PASW Statistics 18 (SPSS Inc., Chicago, IL, USA) was used for all statistical analyses. P < 0·05 was considered statistically significant.
Results
Baseline characteristics
Anthropometric, lifestyle and socio-economic characteristics and median intakes of index components for men and women are shown in Table 2. Regardless of scoring model used, participants in the high score category were more likely to have higher educational level, higher socio-economic status, be cohabiting, be non-smokers, be physically active at leisure and have higher alcohol consumption compared with participants in the low score category (data not shown). All three scoring models were able to separate participants into groups with differing diet quality (data not shown). Participants with high scores had significantly lower intakes of SFA and sucrose, and higher intakes PUFA, fish and shellfish, dietary fibre and fruit and vegetables compared with participants with low scores (all P < 0·0001). In addition, a high score was associated with higher intakes of ascorbic acid, vitamin D, folic acid, Fe, Mg and Se (all P < 0·0001).
Table 2 Baseline characteristics of men (n 6940) and women (n 10186) from the Malmö Diet and Cancer cohort (1991–1996)
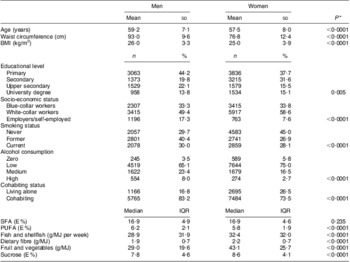
E %, energy percentage; IQR, interquartile range.
*The t test was used to compare means; the Mann–Whitney U test to compare ranking of educational level, socio-economic status, smoking status and alcohol consumption; the χ 2 test to compare cohabiting status; and the Mann–Whitney U test to compare median intakes of index components.
All-cause mortality and DQI-SNR
Estimates of all-cause mortality risk by category of index score are shown in Table 3. In the fully adjusted model, men with high scores (DQI-SNR Model 1) had a decreased risk of all-cause mortality (hazard ratio (HR)=0·79, 95 % CI 0·66, 0·95) compared with men with low scores. The trend of decreased risk across total score (continuous) was also significant (P for trend = 0·001). Similarly, high scores (DQI-SNR Model 3) were associated with lower risk of all-cause mortality (HR = 0·84, 95 % CI 0·73, 0·97; P for trend = 0·023) in men. After full multivariate adjustment, no associations were seen using DQI-SNR Model 2. Among women, no associations were seen in the fully adjusted model (all scoring models).
Table 3 Hazard ratios (HR) and 95 % confidence intervals for all-cause mortality among men (n 6940) and women (n 10186) by category (low, medium, high) of diet quality index (DQI-SNR) score using three scoring modelsFootnote *: the Malmö Diet and Cancer cohort (1991–1996)
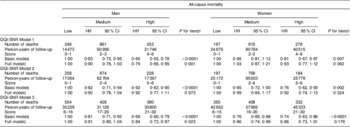
* DQI-SNR scoring models: DQI-SNR Model 1, predefined cut-offs; DQI-SNR Model 2, median-based cut-offs; DQI-SNR Model 3, quintile-based cut-offs.
† P for trend for continuous DQI-SNR score.
‡ Basic model adjusted for method version, season of data collection, age (continuous) and total energy intake (continuous).
§ Full model adjusted for method version, season of data collection, age (continuous), total energy intake (continuous), smoking status, leisure-time physical activity and alcohol consumption.
Cause-specific mortality and DQI-SNR
Estimates of cause-specific mortality risk by category of index score are shown in Table 4 for men and Table 5 for women. In the fully adjusted model, a high score among men using DQI-SNR Model 1 was associated with decreased risk of CVD mortality (HR = 0·59, 95 % CI 0·44, 0·81; P for trend < 0·0001) compared with men with low scores. Similar associations were seen using the other two scoring models, but they were attenuated and not significant in the fully adjusted model. Men with high scores (DQI-SNR Model 1) had a lower risk of cancer mortality (HR = 0·82, 95 % CI 0·68, 0·97); however, there was no significant linear trend across total score. In the fully adjusted models, no associations with cancer mortality were seen using scoring models 2 and 3. There was a significant linear trend (P = 0·030) of decreased risk associated with other-cause mortality among men when using the DQI-SNR Model 3. Among women, no associations between DQI-SNR score (all scoring models) were seen with CVD and cancer mortality. High scores using DQI-SNR Model 2 were associated with decreased risk of other-cause mortality (HR = 0·59, 95 % CI 0·37, 0·94; P for trend = 0·035). The association with other-cause mortality using DQI-SNR Model 3 was similar (HR = 0·65, 95 % CI 0·46, 0·91; P for trend = 0·022).
Table 4 Hazard ratios (HR) and 95 % confidence intervals for cause-specific mortality among men (n 6940) by category (low, medium, high) of diet quality index (DQI-SNR) score using three scoring modelsFootnote *: the Malmö Diet and Cancer cohort (1991–1996)
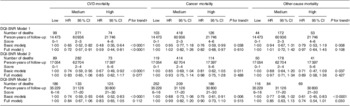
* DQI-SNR scoring models: DQI-SNR Model 1, predefined cut-offs; DQI-SNR Model 2, median-based cut-offs; DQI-SNR Model 3, quintile-based cut-offs.
† P for trend for continuous DQI-SNR score.
‡ Basic model adjusted for method version, season of data collection, age (continuous) and total energy intake (continuous).
§ Full model adjusted for method version, season of data collection, age (continuous), total energy intake (continuous), smoking status, leisure-time physical activity and alcohol consumption.
Table 5 Hazard ratios (HR) and 95 % confidence intervals for cause-specific mortality among women (n 10 186) by category (low, medium, high) of diet quality index (DQI-SNR) score using three scoring modelsFootnote *: the Malmö Diet and Cancer cohort (1991–1996)
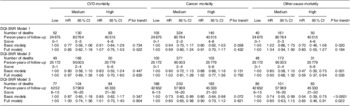
* DQI-SNR scoring models: DQI-SNR Model 1, predefined cut-offs; DQI-SNR Model 2, median-based cut-offs; DQI-SNR Model 3, quintile-based cut-offs.
† P for trend for continuous DQI-SNR score.
‡ Basic model adjusted for method version, season of data collection, age (continuous) and total energy intake (continuous).
§ Full model adjusted for method version, season of data collection, age (continuous), total energy intake (continuous), smoking status, leisure-time physical activity and alcohol consumption.
Discussion
Overall findings
The main finding of the present study is that different scoring methods for dietary indices, even when characterizing identical dietary components, can seriously influence the magnitude of the observed diet–mortality associations. Results suggest however that a dietary pattern similar to what is currently recommended is associated with decreased risk of all-cause mortality and cause-specific mortality, particularly CVD mortality among men.
The DQI-SNR and mortality outcomes
The DQI-SNR was associated with mortality among both men and women. However, the reduction in CVD mortality among men with high DQI-SNR scores was not seen among women. Several plausible reasons could be behind these null associations including that the women in this study population constitute a more homogeneous group (i.e. health-conscious with narrow ranges of nutrient and food intakes) and that associations with mortality may be affected by other uncontrolled factors (e.g. treatment at hospital, timing of hospitalization). In addition, CVD develops approximately 10 years later in women and the female study population is slightly younger than the male. Excluding women below the age of 50 years at baseline strengthened the associations seen with all-cause and cancer mortality; however, the number of CVD deaths was reduced even further and no associations could be seen (data not shown). The strong risk reduction in mortality from non-CVD, non-cancer causes among women has not been observed previously to our knowledge. However, these results are in line with a meta-analysis investigating adherence to the Mediterranean diet which found a significant reduction (13 %) in incidence of Parkinson's and Alzheimer's disease(Reference Sofi, Cesari and Abbate30) and a study by Park et al.(Reference Park, Subar and Hollenbeck31) that found an inverse association between dietary fibre and mortality from respiratory diseases. Although the health effects from dietary patterns similar to the SNR vary between populations, the magnitude of the beneficial effect detected in the current study population is high particularly among men(Reference Huijbregts, Feskens and Räsänen5–Reference Knoops, Groot de and Fidanza7). This may be a population-specific observation or could be explained by the high relative validity of the dietary assessment method used in the current study(Reference Thiébaut, Kipnis and Schatzkin32).
Selection of scoring model
The present results highlight the importance of choosing appropriate scoring models for dietary indices. Overall, there were large differences in the predictive capability of the models used and the associations seen also differed between men and women. The present study considered three scoring models for the DQI-SNR. Although similar scoring models have previously been applied in other studies using different dietary indices, to the best of our knowledge no study has investigated the effect of different scoring models on the predictive capability of a single index. Predefined cut-offs based on recommended intakes have been used for several dietary indices(Reference Seymour, Calle and Flagg6, Reference Patterson, Haines and Popkin33–Reference Fondell, Christensen and Bälter35). This scoring model is particularly useful when investigating the level of adherence to dietary recommendations and the possible effect a particular a priori determined dietary pattern may have on health outcomes. In contrast, population-based cut-offs are often used for dietary indices investigating the combined effect of several foods and/or nutrients when there are no predefined levels of recommended intake (e.g. the Mediterranean Diet Score)(Reference Trichopoulou, Costacou and Bamia36). These scoring models, in contrast to predefined cut-offs, are not dependent on a certain level of intake of the individual components in order for the components to provide sufficient discriminative power(Reference Waijers, Feskens and Ocké16). However, when these indices are applied in populations with very different eating patterns (e.g. applying the Mediterranean Diet Score in a Scandinavian population) it is questionable what type of dietary pattern is investigated. A combination of both predefined and population-based scoring models has also been applied previously(Reference McCullough, Feskanich and Stampfer9, Reference Kennedy, Ohls and Carlson37). Weighting of components could also be considered(Reference Waijers, Feskens and Ocké16–Reference Kourlaba and Panagiotakos18). However, current knowledge does not provide the evidence needed on the individual components and their relative impact on mortality outcomes. For investigation of the association between diet quality and specific diseases it could be advised to include analysis on the individual components to determine which aspects of overall diet quality are more important in relation to the health outcome.
Because of differences in reported food and nutrient intakes and as well as possible confounding variables, it was necessary in the present study to conduct analyses separately for men and women. Particularly, the differences in eating pattern would affect the DQI-SNR models 2 and 3, which used median and quintile-based cut-offs. The DQI-SNR Model 1 cut-off values were predefined according to the Swedish dietary recommendations; however, some adjustments were made in order to provide sufficient discriminative power for all components (i.e. SFA). In men, the DQI-SNR Model 1 was strongly associated with all-cause and CVD mortality. These results suggest that in order to increase longevity, men could adhere to dietary recommendations similar to the 2005 SNR and SDG. The major advantage with this model is its simplicity and easy interpretation. Use of median-based cut-offs (DQI-SNR Model 2) showed the lowest predictability of mortality among both men and women. The few associations seen with mortality suggest that, for this population and the included components, this type of scoring model is not suitable. The advantage of this model is that each component distinguishes equally. However, it is not certain that the median reflects a level of intake associated with health benefits as indicated by the poor predictability. A quintile-based approach (or similar approaches) may be the preferred model when the study population is fairly homogeneous (i.e. in terms of health behaviours and dietary intakes), as in the case of women of the MDC cohort. The female population had higher intakes of dietary fibre, fruit and vegetables and was to a greater extent non-smoking and low alcohol consumers. The fact that the quintile-based scoring model (DQI-SNR Model 3), which enables greater separation of women based on nutrient intakes, showed greater predictability thus suggests that this may be due to a narrow range with generally high intakes of fibre and fruit and vegetables among women. However, although this model showed greater predictability of mortality among women, both the DQI-SNR Model 3 and DQI-SNR Model 2 are highly dependent on the intake distribution of the selected components and could be poor estimates of adherence to specific recommendations, especially since some recommendations involve both and upper and lower intake level (e.g. PUFA). The conclusion that can be drawn from these results is that the scoring model severely influences the predictive capability of the DQI-SNR. Results suggest that when appropriately selected, predefined dichotomous cut-offs provide sufficient discriminative power. In addition, predefined cut-offs may increase reproducibility when they are based on recommended intake levels. However, if the cut-offs are inappropriately selected in regard to the population intake levels (i.e. when very few or all individuals adhere) this may attenuate possible associations.
Strengths and limitations
The prospective design, large sample size and the long follow-up period are major strengths of the present study. The MDC cohort consists of middle-aged to elderly men and women and thus generalizability of our findings to other age groups is uncertain. To reduce misclassification and recall bias our analytical sample excluded individuals with self-reported food habit change in the past (indicting potentially unstable food habits over time), potential energy misreporters and clinical conditions thought to affect food choices (i.e. diabetes diagnosis and previous cardiovascular event). The study sample was after these exclusions similar to the MDC cohort in regard to lifestyle characteristics and dietary intakes. Also, the frequency of deaths and distribution of causes of death among men and women in the study sample were similar to those in the MDC cohort, indicating that no major selection bias occurred based on the exclusions made. The associations seen were however slightly strengthened after the exclusion of these individuals, highlighting the importance of this type of sensitivity analysis when dealing with self-reported dietary data. Further advantages of the present study are the high relative validity of the modified diet history method used in the MDC study in comparison to other methods used in similar population-based studies(Reference Thiébaut, Kipnis and Schatzkin32). Also, another major strength is the use of high-quality national registry data on vital status and underlying cause of death with high reliability and virtually no loss to follow-up. The DQI-SNR has not been previously applied in studies relating this dietary pattern with disease outcomes. Although the DQI-SNR is a relatively crude measure of adherence to the Swedish dietary recommendations, the development study(Reference Drake, Gullberg and Ericson14) suggests that qualitative differences in diet are associated with DQI-SNR score. These results are further confirmed here. It would however be premature to conclude that the observed inverse association between DQI-SNR and mortality is causal. Given the observational nature of our study, the possibility that the DQI-SNR is a surrogate for some unknown, poorly measured or inadequately controlled determinant of mortality cannot be ruled out. Although we controlled for the most important risk factors for mortality, we cannot dismiss the possibility of residual confounding.
Conclusions
The scoring model used for dietary indices may have a significant impact on observed associations with disease outcomes. The rationale for choice of scoring model should be included in studies investigating the association between dietary indices and disease. Our observations also indicate that a dietary pattern similar to the current dietary recommendations is effective in increasing longevity among middle-aged to elderly men and women of a population-based cohort. However, since compliance with some recommendations was poor in this cohort, the health benefit might potentially be substantial if complete adherence was achieved.
Acknowledgements
The study was funded by the Swedish Heart–Lung Foundation, the Ernhold Lundström Foundation, Region Skåne and Skåne University Hospital. None of the authors has any personal or financial conflict of interest. I.D. and E.W. designed the study. I.D. performed all statistical analyses, wrote the paper and had primary responsibility for the final content. B.G., B.H. and E.W. provided suggestions and feedback on statistical analysis and helped interpret results and review the manuscript. E.S., P.W., M.P., J.H. and J.N. participated in interpretation of results and reviewing of the manuscript. All authors have read and approved the final manuscript.