Geographic access to healthy, affordable foods is a national priority for US public health policy, in part because it is hypothesized that lack of access is an underlying cause of obesity and diet-related chronic diseases( Reference Michimi and Wimberly 1 – Reference Giang, Karpyn and Laurison 7 ). Placing full-service supermarkets – a source of healthy, affordable foods – in food deserts, or in areas with limited access to healthy foods, has been proposed as a strategy to improve diet among residents. The Healthy Foods Financing Initiative, which passed as part of The Agricultural Act of 2014 (also known as the Farm Bill) in the USA, established a programme that provides federally funded incentives to locate full-service supermarkets in food deserts across the USA; states including Pennsylvania and California have state-initiated policies with similar incentivizing programmes( Reference Holzman 8 , Reference Karpyn, Manon and Treuhaft 9 ).
Focusing solely on the characteristics of food stores closest to residents’ homes limits the understanding of food ‘access’. Despite important empirical advances, relatively little is known about how individuals, and especially individuals who live in food deserts, interact with and may be affected by their food environment( Reference Hillier, Cannuscio and Karpyn 10 – Reference Kerr, Frank and Sallis 13 ). Unanswered questions include the following: What comprises the food environment for food desert residents in terms of types of foods sold and marketed both locally and where they actually shop? Do food desert residents shop at local stores without healthy options or go outside their neighbourhood? If they travel outside their neighbourhood, is it to outlets with more healthy food options? How are healthy and unhealthy foods marketed both in the neighbourhood and at the stores where they shop?
We also need to understand the roles of mode of transportation, the length of time that food shopping takes, the frequency of food shopping and the average dollar amounts spent on food in determining how food desert residents interact with and are affected by their food environments. Key questions in this vein include: Are only those with access to a car able to travel outside the neighbourhood? If food desert residents usually have access to a car, does the distance either to where they shop or to the nearest full-service supermarket matter? Is there a trade-off in terms of time and dollars spent in going further afield? Do residents who travel further shop less frequently and therefore purchase fewer fresh and healthy foods?
While scientists are beginning to understand that food purchasing venues closest to residents’ homes may not encompass the food environment with which they interact, no studies have provided a complete picture by taking food purchasing practices, characteristics of stores where residents shop and sociodemographic data of households into account. And no study, to the authors’ knowledge, has examined this in conjunction with detailed dietary data (i.e. dietary recalls or records) and BMI among food desert residents. Dietary data are difficult and expensive to collect and as such prior research has mostly utilized brief instruments (e.g. short dietary screeners) or FFQ( Reference Morland, Wing and Diez Roux 14 – Reference Zenk, Lachance and Schultz 18 ). However, because these instruments rely on pre-existing lists of foods to choose from, the instruments may not capture all foods that are part of participants’ diets and thus may result in biased outcomes. Unlike brief instruments and FFQ, 24 h dietary recalls capture the intake of all foods in a specified and recent time period and thus can be used to estimate absolute rather than relative intakes of energy and other food components( Reference Buzzard 19 ).
The present paper seeks to illuminate where, when and how residents of food deserts interact with the food environment (purchasing practices), describe the food environment in these neighbourhoods and where residents shop (food availability), and ultimately to test how indicators of food purchasing practices and food availability are associated with diet and weight. This is done by employing data from the Pittsburgh Hill/Homewood Research on Eating, Shopping and Health (PHRESH) study to gain insights that will ultimately inform further development of theory regarding the role of food access in obesity and policy.
Methods
Overview
PHRESH is a 5-year study of two urban neighbourhoods classified as food deserts (Hill District, Homewood) because they are comprised of low-income census tracts where a substantial number of residents have poor geographic access to a supermarket or large grocery store( 20 ). The Hill District neighbourhood is approximately 3·55 km2 (1·37 square miles) with a population of approximately 10 219, and Homewood is approximately 3·76 km2 (1·45 square miles) with a population of approximately 8300. Both neighbourhoods are predominantly African American and very similar in physical environment and sociodemographic features. However, a major difference is that a full-service supermarket has been constructed and is newly open (as of the end of 2013) in only one neighbourhood (Hill District). PHRESH is following a randomly sampled cohort of household primary food shoppers in these neighbourhoods and interviewing them at multiple points in time. The study is also auditing food availability and prices in all stores that sell food in both neighbourhoods and in stores outside both neighbourhoods where residents most frequently reported shopping. The present paper reports on data from the baseline interview and food store audits, both conducted prior to the opening of the full-service supermarket; later waves of data are not yet available. All study protocols were approved by the RAND Human Subjects Protection Committee.
Sample and household survey
Baseline household surveys were administered in May–December 2011. Households were randomly selected from a list of addresses obtained from the Pittsburgh Neighborhood and Community Information System, an aggregate of numerous data sets including parcel-level address data within Pittsburgh neighbourhoods, housed at the University Center for Social and Urban Research at the University of Pittsburgh. These data were merged with Allegheny County Office of Property Investment data to identify residential addresses and then cross-referenced with postal service data to remove vacant properties from the sample. Stratified random sampling was then applied to the remainder, with an oversampling of households in the Hill District. Pre-notification postcards and letters were mailed to all selected addresses (n 2900).
Eighteen trained data collectors who lived in the neighbourhoods went door-to-door to enrol households. Data collectors were able to identify the address as a residence and speak with an adult for 1956 households (67 % of all selected addresses). Of those households, 1649 were eligible to participate (agreed to the screening and the primary food shopper was aged 18 years or older) and 1434 (87 % of those eligible) agreed to do so. Of those households that participated, sixty-two (4 %) had unusable data, leaving a final sample of 1372 households.
Data collectors administered interviews that were approximately an hour in length to the main food shopper of each household, entering data on a laptop computer. Sociodemographic characteristics and other factors that might affect food access, diet and weight were measured. Respondents self-administered sensitive questions, such as those concerning household income and participation in federal assistance programmes. Interviewers measured respondent height and weight at the conclusion of the interview and guided respondents through a 24 h online dietary recall. Approximately one week later, respondents repeated this dietary recall a second time via a telephone interview.
Measures
Outcomes
Diet
Dietary intake was assessed with the Automated Self-Administered 24 h recall (ASA-24)( Reference Subar, Kirkpatrick and Mittl 21 ), which uses a modified version of the US Department of Agriculture’s Automated Multiple-Pass Method. Diet was based on the average of the two 24 h recalls unless respondents completed only one (7 % of respondents). Dietary quality was derived from the recall data using Healthy Eating Index-2005 (HEI-2005)( Reference Guenther, Reedy and Krebs-Smith 22 , Reference Guenther, Reedy and Krebs-Smith 23 ) scores. The HEI-2005 includes twelve components: fruit, vegetables, grains, milk, meat, beans, oils, saturated fat, sodium, solid fats, alcoholic beverages and added sugars. The index assesses conformance to federal dietary guidance based on dietary guidelines released in 2005. The food group standards are based on the recommendations found in MyPyramid( Reference Britten, Marcoe and Yamini 24 ) and are expressed as a percentage of energy or per 4184 kJ (or 1000 kcal). Thus HEI-2005 is a continuous measure, with 100 being the highest possible score and reflecting the best dietary quality.
BMI
Interviewers measured height to the nearest eighth of an inch (~3 mm) using a carpenter’s square (triangle) and an 8 ft (~2·4 m) folding wooden ruler marked in inches. Respondent weight was measured using the SECA Robusta 813 digital scale, which was capable of weighing respondents up to 181 kg. Interviewers recorded weight as it appeared on the scale’s display, to the nearest tenth of a pound (~0·05 kg). BMI (defined as weight in kilograms divided by the square of height in metres) was examined as a continuous outcome, but we also report standard categories: underweight (BMI<18·5 kg/m2), normal weight (BMI=18·5–24·9 kg/m2), overweight (BMI=25·0–29·9 kg/m2), class I obesity (BMI=30·0–34·9 kg/m2), class II obesity (BMI=35·0–39·9 kg/m2) and class III obesity (BMI≥40·0 kg/m2) in describing the sample.
Food access and purchasing practices
Distance to where respondents shopped
All respondents were asked where they did their major food shopping. Because 92 % of respondents reported leaving from home for their shopping, street network distances were derived from each respondent’s home to the reported retail venue. The street network distances (in kilometres) represented the shortest route respondents would likely drive along the existing road network from home to the identified retail venue, and were analysed as a continuous measure. Distance to where one shops was hypothesized to be negatively associated with dietary quality and positively associated with BMI by reducing the frequency with which fresh and healthier foods are replenished.
Type of store where respondents shopped
Food stores can vary dramatically in what they sell and market; store type can capture some of these differences. Stores where respondents shopped were classified based on definitions from the Food Marketing Institute and the North American Industry Classification System, and classifications were confirmed with the study’s Community Advisory Boards, comprised of key resident stakeholders within each neighbourhood. Categories included: (i) full-service supermarkets (stores offering a full line of groceries and run by nationally or regionally recognized chains)( 25 ); (ii) discount food stores (stores that offer a limited assortment of low-priced and perishable items, such as Family Dollar or Save A Lot); (iii) supercentres (department stores that also offer full lines of groceries and general merchandise, such as Walmart or Target); (iv) wholesale clubs (stores that sell a large variety of goods in wholesale quantities, such as Sam’s Club and Costco); (v) ‘mom-and-pop’ or convenience stores (small individual/family-owned stores or chain stores including those with gas stations, such as Get Go, AM/PM or Shell Station); (vi) specialty grocery stores (such as Whole Foods or Trader Joe’s); and (vii) meat/seafood markets. Shopping at a full-service supermarket was hypothesized to be associated with healthier diet and lower BMI.
Shopping frequency
All respondents were asked how frequently they do their major grocery shopping, with five choices that ranged from less than once per month to more than eight times per month. Shopping frequency was modelled as an ordinal variable. It was expected that those who travelled further to shop would shop less frequently, take longer to complete each trip and use more convenient modes of transportation (i.e. car). More frequent major food shopping was also postulated to be associated with more purchases of perishable foods including produce and thus hypothesized to predict healthier dietary intakes and weight outcomes.
Transportation mode
All respondents were asked about their transportation mode for food purchasing. Responses were categorized into: (i) driving or borrowing a car; (ii) taking a jitney (unregulated taxi); (iii) public transportation; and (iv) getting a ride. Given the frequency of responses, we postulated that driving or getting a ride would provide the easiest transportation; thus, responses were condensed into a dichotomous variable of ‘drives or gets a ride’ v. all other modes of transport and modelled as such. Use of a car was postulated to make it easier to shop for food outside the neighbourhood and improve access to healthy options. For the same reason, it was also expected that use of a car would be associated with travelling further and possibly with more frequent and shorter shopping trips. Based on these assumptions, we hypothesized that use of a car would predict better diet and lower BMI.
Shopping duration
Respondents reported the amount of time they spent on a typical major food shopping trip, from the time they left home until the time they returned. Responses were open-ended, and we derived a continuous variable (in minutes) for use in analysis. As noted above, total time spent shopping for food was expected to affect and be affected by other food purchasing patterns, including transportation mode, distance travelled to shop and shopping frequency. It might also affect what is purchased and eaten. For example, a respondent who takes public transport to shop might take additional time to shop, which might translate into less time to prepare fresh or healthy foods. We did not hypothesize a relationship with BMI or diet but instead looked at this in an exploratory manner.
Food expenditures
Respondents were also asked: ‘Approximately how much money do you spend per week on food?’ and ‘How many people does this amount feed?’ We derived from these a continuous measure reflecting the amount (in dollars) spent per person per week. One reason people may travel further to purchase food, even in the face of longer trips and transportation issues, is to save money. Thus, analyses explored whether respondents who made longer shopping trips spent less per individual in the household. Because prior research has shown that lower-priced foods tend to be of lower quality and nutritional value( Reference Aggarwal, Monsivais and Drewnowski 26 – Reference Monsivais and Drewnowski 28 ), it was hypothesized that those who spent less money would have lower dietary quality and higher BMI.
Food environment
Distance to the nearest full-service supermarket and closest food store
Through secondary data listings and primary data collection combing neighbourhood streets and surroundings, all full-service supermarkets in the area were located and the street network distance from each respondent’s home address to the closest full-service supermarket was calculated, as was distance to the closest food store. Street network distances were calculated in ArcView 10 software using ESRI’s Network Analyst Tool and the North American Network Dataset. Variables are continuous and in kilometres. Greater distance was hypothesized to be positively associated with BMI and negatively associated with dietary quality.
In-store marketing and healthy food availability
Between November 2011 and January 2012, all stores that sold any type of food in both neighbourhoods – including, but not limited to, the stores where respondents reported shopping – were audited. The ten most-frequented food stores outside each of the two neighbourhoods where survey respondents reported shopping (sixteen stores in total across the two neighbourhoods due to overlap) were also audited.
Store audits were completed by trained observers using an adapted version of the Bridging the Gap–Community Obesity Measures Project Food Store Observation Form (FSOF)( Reference Rimkus, Powell and Zenk 29 ). Observers chose the dominant product in view from the following list: (a) fruits; (b) vegetables; (c) sugar-sweetened beverages; (d) candy or sweetened baked goods; (e) salty snacks; or (f) other. We derived two dichotomous variables indicating (i) whether fruits or vegetables dominated the view (a or b was chosen) and (ii) whether snack foods dominated (c, d or e was chosen). Observers also counted the number of fresh fruit and vegetable varieties up to a maximum of twenty in each category, resulting in continuous indicators of (i) fresh fruit and (ii) fresh vegetable availability.
Analysis
We began with a series of analyses that illuminated food purchasing practices and access and their relationships in our sample, to lay the groundwork for interpreting our main results. To provide an overview of the sample’s food shopping environment, statistics (counts) were calculated describing the number of stores of each type, stratifying by store location (inside the two study neighbourhoods v. outside but where residents most frequently reported shopping). To provide a richer understanding of these store types, we also calculated the percentage of each type (inside and outside the neighbourhood) predominantly displaying snacks and the percentage predominantly displaying fruits and vegetables, as well as the mean number of varieties of fruits and vegetables sold. To describe shoppers and their purchasing practices, sociodemographic characteristics of respondents (i.e. primary food shoppers) as well as their standing on the outcome variables (BMI and dietary quality) and the measures of food access and purchasing practices were estimated (percentage in various categories of the variables’ distributions). Pearson correlations among the food access and purchasing practice variables were calculated for all continuous measures, while Spearman correlations were estimated between shopping frequency (an ordinal variable) and other measures. In the case of the binary variable (drives or gets a ride), t tests were computed to test associations with continuous measures, while a χ 2 test was used to test for an association with shopping frequency. ANOVA was used to compare the mean distances to the nearest full-service supermarket of respondents and the type of store where residents chose to do their major shopping.
We followed these analyses with tests of our primary hypotheses relating to dietary quality and BMI. For each outcome (BMI and dietary quality) and each predictor (measures of food access and purchasing practices), we estimated a pair of linear regression models. First, we tested each model with a single variable predicting each outcome (resulting in bivariate tests). Then, we repeated each test adjusting for sociodemographic characteristics that are known correlates of either BMI or dietary quality, to rule out possible confounding of these factors with the predictors of interest. Variables in these adjusted models were age, gender, income, educational attainment, marital status and presence of children in the household (presence of children was a dichotomous variable, i.e. if one or more child was present in the household or not). We applied a conservative P value of 0·01 to all of the coefficients generated by our regression models to adjust for the number of tests conducted.
Results
Food environment where respondents live and shop
In the two study neighbourhoods, there were twenty-four food retail venues. Almost all of these were ‘mom-and-pop’ or convenience stores. Just two sold produce, one was a fruit and vegetable stand and another was a neighbourhood convenience store with a few fresh produce choices. Snacks were the item that almost always dominated the view from the main entrance of the neighbourhood-based food retail venues.
Outside the neighbourhoods, there were sixteen stores where residents of either neighbourhood reported doing their major food shopping. Half of the stores where respondents shopped (n 8) were full-service supermarkets. There were also two supercentres, two discount grocery stores, two meat/seafood markets, one wholesale club and one specialty grocery store. Almost all of these food stores had a substantial selection of fresh fruit and vegetables. However, only two of the full-service supermarkets and one of the discount grocery stores had a dominant view of fruit or vegetables from the main entrance. Snacks dominated the view from the main entrance in both of the supercentres, the specialty grocery store and one of the meat/seafood markets (Table 1).
Table 1 Characteristics of food stores in two Pittsburgh food deserts and food stores where residents of these areas shop; baseline data from the Pittsburgh Hill/Homewood Research on Eating, Shopping and Health (PHRESH) study, May–December 2011
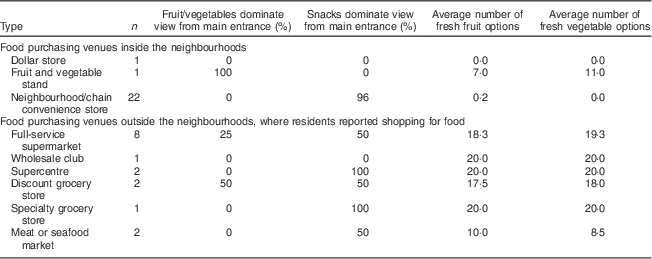
Respondent characteristics
Most respondents were between 45 and 75 years of age, reported a per capita household income between $US 5000 and $US 20 000 per annum, identified as Black (90·5 %) and were high-school graduates and/or had some college or technical school (Table 2). The mean BMI was 30·9 (sd 7·5) kg/m2 and more than three-quarters (76·6 %) were overweight or obese. Mean HEI-2005 score was 48·9 (sd 10·9; out of a total possible score of 100). Nationally, HEI-2005 mean scores are 57·2, and among non-Hispanic Blacks, 55·0( Reference Ervin 30 ).
Table 2 Characteristics of study respondents (primary household shoppers in two Pittsburgh food deserts); baseline data from the Pittsburgh Hill/Homewood Research on Eating, Shopping and Health (PHRESH) study, May–December 2011
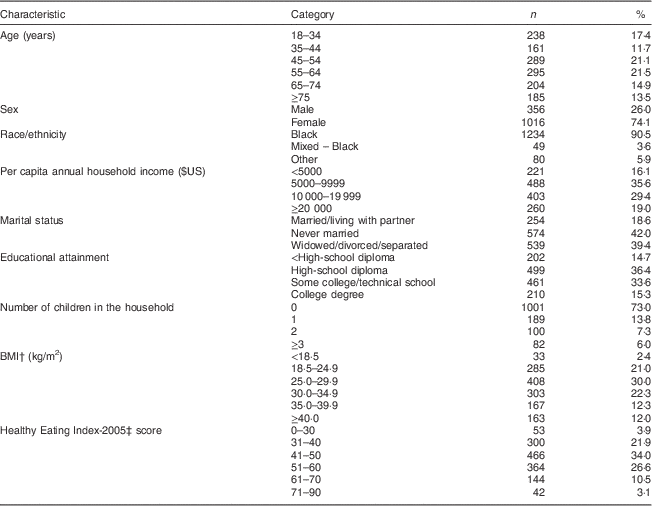
† BMI: mean=30·9 (sd 7·5) kg/m2.
‡ HEI-2005 score: mean=48·9 (sd 10·9).
Respondents’ food access and purchasing practices
The nearest store that sold any type of food products (mostly ‘mom-and-pop’ or convenience stores) averaged 0·4 km from home. The nearest full-service supermarket was, on average, 2·4 km away from respondents’ homes. There was just one respondent who reported doing his/her major food shopping at a store in his/her neighbourhood; all other respondents did their main food shopping outside the neighbourhood. Most respondents (75·5 %) did their major shopping at a full-service supermarket, and two of every three people who shopped at a full-service supermarket (51·2 % of total residents) frequented one that was not the nearest one to their home. Full-service supermarkets where respondents shopped averaged a distance of 4·3 km from their residences. An additional 11 % reported shopping at a supercentre.
Associations between food access and purchasing practices
On average, respondents travelled 6·0 km for their major shopping and spent about $US 37 per person per week. Almost half shopped two to four times per month, and the most common modes of transportation for shopping were driving or taking a jitney (Table 3). Examining correlations among food access and purchasing practice variables showed that distance to the nearest full-service supermarket was not associated with how far respondents travelled to do their major food shopping, or with how frequently they shopped, shopping duration or food expenditures (Table 4). There was also little difference between distance to the nearest full-service supermarket and the type of store where residents chose to do their major shopping (F=0·87; P=0·504). The associations that were observed were fairly small. Greater distance to where respondents actually did their major food shopping was associated with lower spending, less frequent shopping and increased time to do shopping (Table 4). Those respondents who reported driving or getting a ride to where they shopped reported shopping more frequently and spent less time per trip. In addition to its association with distance travelled, amount spent per person per week was higher among those who shopped more frequently.
Table 3 Where and how residents of two Pittsburgh food deserts shop for food (major shopping); baseline data from the Pittsburgh Hill/Homewood Research on Eating, Shopping and Health (PHRESH) study, May–December 2011

Table 4 CorrelationsFootnote † between food access and purchasing practice indicators; baseline data from the Pittsburgh Hill/Homewood Research on Eating, Shopping and Health (PHRESH) study, May–December 2011

*P<0·01, **P<0·001, ***P<0·0001.
† Correlations with shopping frequency were computed as Spearman correlations; all others were computed as Pearson correlations.
Associations with BMI and dietary quality
As shown in Table 5, bivariate tests of each food access and purchasing practice variable and BMI showed some statistically significant (P<0·01) positive associations. Not adjusting for any additional factors, each mile (or 1·6 km) to where respondents reported doing their major shopping was associated with an increase in BMI of 0·18 units (kg/m2). Similarly, unadjusted bivariate associations showed that shopping at a supercentre was associated with a 1·72-unit increase in BMI. So, for a 5 ft 5 in (165 cm) tall female who weighs 168 lb (76·4 kg; BMI of 28·0 kg/m2), doing major food shopping at a supercentre was associated with a 2·08-unit increase in BMI (equivalent to weighing 180 lb (81·8 kg) or having a BMI of 30·0 kg/m2). Respondents who spent more time shopping had a statistically significantly higher BMI, with each 10-min increase associated with a 0·10-unit increase in BMI. Spending more money per capita on food had a marginally significant negative association with BMI, with each additional $US 10 per person per week associated with a 0·10-unit decrease in BMI.
Table 5 Bivariate and adjusted associations between food access and purchasing practice indicators, diet and BMI; baseline data from the Pittsburgh Hill/Homewood Research on Eating, Shopping and Health (PHRESH) study, May–December 2011
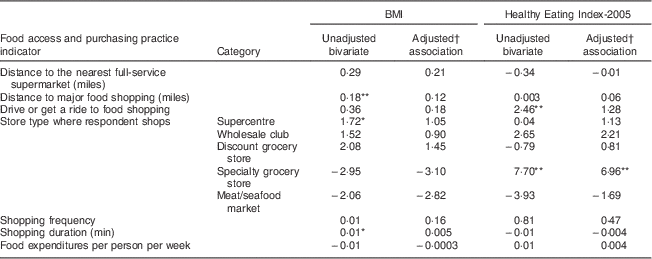
*P<0·01, **P<0·001.
† Adjusted for age, gender, household income, educational attainment, marital status and number of children in the household.
When estimated relationships controlled for sociodemographic characteristics (age, gender, income, educational attainment, marital status and presence of children in the household), the statistical significance of these associations was eliminated. Distance to where respondents shopped was a marginally significant predictor (P<0·05, so significance not shown in Table 5) of higher BMI (each additional mile (1·6 km) was associated with 0·12-unit increase in BMI); shopping at a specialty grocery store and meat/seafood market also held marginal significance (P<0·05, so significance not shown in Table 5) in their association with lower BMI (3·10 and 2·82 units, respectively). For the same 5 ft 5 in (165 cm) tall female, such adjusted associations translate into approximately 2 lb (0·9 kg) per additional mile (1·6 km), and a weight of 19 lb (8·6 kg) less (i.e. 149 lb (67·7 kg)) for the same respondent who reported shopping at a specialty grocery store, and about 16·5 lb (7·5 kg) less for shopping at a meat/seafood market.
In bivariate analyses for dietary quality, driving or getting a ride to where one shops and shopping at a specialty grocery store were associated with higher dietary quality (P<0·001), higher dietary quality was marginally associated with more frequent shopping and lower dietary quality was marginally associated with shopping at a meat/seafood market (P<0·05, so significance not shown in Table 5). Respondents who drove or got a ride to where they did their major food shopping had a HEI-2005 score that was 2·46 points higher (P<0·001); respondents who shopped at specialty grocery stores had a 7·70 points higher HEI-2005 score (P<0·001); and those who shopped more than four times per month had a 0·81 points higher HEI-2005 score (P<0·05, so significance not shown in Table 5). While most of this cohort had a HE-2005I score somewhere between 31 and 60 (of a possible 100), eating an additional two servings of fruit daily could increase one’s HEI-2005 score by 2·5 points.
Once adjusted for demographics, the only statistically significant association (P<0·005) that remained was between higher HEI-2005 score and shopping at a specialty grocery store. Driving or getting a ride to where respondents shopped was a marginally significant (P<0·05) predictor of increased dietary quality (respondents who drove or got a ride to where they shopped reported an average HEI-2005 score 1·28 units higher than others; comparable to about one additional serving of fruit per day). There was also a statistically significant association (P<0·001) between shopping at a specialty grocery store and higher HEI-2005 score (by 6·96 points).
Discussion
By nearly any definition, the neighbourhoods that were part of the present study were food deserts, areas with extremely limited or no access to healthy food options within their boundaries: twenty-two of the twenty-four food retail venues were ‘mom-and-pop’ or convenience stores with no availability of fresh produce. Perhaps for this reason, most residents reported doing their main food shopping at one of sixteen food stores outside the neighbourhoods, half of which were full-service supermarkets. Of the food stores where residents shopped, most sold a variety of fresh fruits and vegetables.
Many studies have shown that poor access to full-service supermarkets is associated with poor diet and obesity( Reference Bodor, Rice and Farley 6 , Reference Morland, Wing and Diez Roux 14 , Reference Laraia, Siega-Riz and Kaufman 17 , Reference Powell, Han and Chaloupka 31 – Reference Sharkey, Johnson and Dean 34 ). But other data suggest that full-service supermarket access has little to do with diet or obesity( Reference Block, Christakis and O’Malley 35 – Reference An and Sturm 40 ) and too much access to fast-food outlets and convenience stores, which predominantly sell energy-dense, nutrient-poor foods and few healthy alternatives, is what matters for obesity( Reference Laska, Hearst and Forsyth 41 , Reference Sallis, Floyd and Rodriguez 42 ). One study even showed that residing closer to a full-service supermarket was associated with higher BMI( Reference Wang, Kim and Gonzalez 37 ). Most of this research has focused solely on the neighbourhood food environment and has assumed that residents shop for food near their homes. Although this assumption has been questioned by a growing number of studies( Reference Hillier, Cannuscio and Karpyn 10 , Reference Rose and Richards 43 – Reference Drewnowski, Aggarwal and Hurvitz 47 ), our study is the first to our knowledge that does this among residents of urban, low-income, primarily African-American food deserts. In addition, our analysis was able to examine a range of food access and purchasing practices, how they relate to one another and their associations with dietary or weight outcomes.
Eighty-one per cent of our respondents reported an annual household income of less than $US 20 000 per capita. Residents were also at high risk for chronic disease related to overweight and obesity based on their BMI. The mean BMI was 30·9 kg/m2; 46·6 % of the cohort was categorized as obese. It was found that residents did not rely on food stores near their homes for their major food shopping; in fact, residents travelled an average distance of 4·3 km to shop at a full-service supermarket of choice, despite the closest full-service supermarket being located more than 1·6 km closer to them. Many travelled much further to access stores of other types. This is similar to what Hillier et al. found: that residents of two low-income Philadelphia neighbourhoods, on average, travelled 1·0 km further than their closest food store for their general food shopping( Reference Hillier, Cannuscio and Karpyn 10 ).
The present study also found that the vast majority of residents in these food deserts already shop at a full-service supermarket, even though there are none in their neighbourhood (i.e. within a radius of 1·6 km of their homes). Our estimate that 76 % of residents shopped at a full-service supermarket is lower than that for a multi-ethnic sample of recipients of the Special Supplemental Nutrition Program for Women, Infants, and Children in two low-income neighbourhoods in Philadelphia (98 %)( Reference Hillier, Cannuscio and Karpyn 10 ) and much higher than estimates for two other food desert samples – a predominantly African-American sample and a multi-ethnic sample, both in low-income neighbourhoods in Detroit (38 % and 35 %, respectively)( Reference Zenk, Schulz and Hollis-Neely 44 , Reference Zenk, Schultz and Israel 46 ). One possible explanation for these differences is variation in the study samples. All of the studies, however, indicate that substantial percentages of low-income individuals shop at full-service supermarkets. The likely impact of improving access to such venues in food deserts should be considered in light of these data.
Indeed, distance to the nearest full-service supermarket had no significant relationship with most other access measures, including the distance travelled to do major food shopping, the frequency of shopping or the type of store where residents did their main shopping. This finding raises additional concerns. If current distance from full-service supermarkets is not related to indicators of purchasing practices and food access, it is questionable whether reducing this distance will change behaviour. Similarly, bivariate associations between food access and purchasing practices and BMI and dietary quality (HEI-2005), as well as adjusted tests, cast doubt on such interventions. Distance to the nearest full-service supermarket was associated with neither BMI nor dietary quality.
Nevertheless, our results highlight that understanding food access is complex. Prior to adjusting for sociodemographic factors, distance to where residents actually shopped was related to BMI (greater distance to where one shops was associated with higher BMI); yet it was not associated with dietary quality. In unadjusted associations, higher dietary quality was associated with driving or getting a ride to where one shops. Adjusted results showed a different story: the only association that remained statistically significant (P<0·005) was between shopping at a specialty grocery store and higher dietary quality.
These results imply that weight status (or BMI) and dietary quality may not, in fact, be affected by the same food access variables. In fact, at least one prior study with an urban African-American sample also found that shopping at specialty stores was associated with better dietary quality( Reference Zenk, Schulz and Hollis-Neely 44 ). It may be that these stores emphasize health and well-being broadly, and thus their shoppers eat more nutritiously and consume less energy. Certainly, these stores carry non-nutritious foods, and the one audited for the present study predominantly displayed snacks near the entrance. However, specialty grocery stores may ‘brand’ themselves as places where the health conscious shop, carrying a higher proportion of items like lower-sugar cereal, gluten-free products, and almond- or soya-based milk than traditional grocery stores.
This latter point, that marketing may be critically important to understanding the food environment, perhaps more so than access, may be particularly true for residents of food deserts, who are largely low-income individuals. Research by Spears has shown that when ‘poor’ shoppers have to make difficult decisions, it depletes self-control more so than it does among ‘rich’ shoppers and may leave low-income individuals more vulnerable to marketing( Reference Spears 48 ). Yet, a qualitative study found limited differences between residents of food deserts and food ‘oases’ in terms of budget considerations, convenience and the importance of the freshness and appearance of food in determining shopping patterns( Reference Walker, Block and Kawachi 11 ). And food marketing is clearly directed at all income groups. If new supermarkets are introduced into food deserts, it seems important to focus on how they market and display healthy and unhealthy foods, not just on their presence in the community.
Although our results show little reason to believe that introducing full-service supermarkets to food deserts will improve diet, they do not preclude engineering food environments with other goals in mind. Introduction of full-service supermarkets to urban communities may provide employment for residents and a safe and attractive gathering place that builds sense of community and neighbourhood pride. However, our finding that most people shop further than their nearest full-service supermarket suggests the importance of involving residents in the planning phase to increase the likelihood that new markets fulfil their needs and preferences and will be used by residents.
Our findings question the supposition that increased physical access to full-service supermarkets will be associated with better diet and that better diet will lead to lower BMI – assumptions that underlie current food policy initiatives placing grocery stores in food deserts. However, our study has limitations. While our collection of data from individuals and their food environment is extensive, our analysis is based on cross-sectional data. There is no way to discern causality. Moreover, a food desert has been defined as absence of a supermarket within 1·6 km of home. Thus, it is possible that the closest full-service supermarket for our respondents, averaging 2·4 km from their homes, was not close enough to facilitate healthful eating. Furthermore, although the neighbourhoods where our study took place are typical urban food deserts in many ways, the city of Pittsburgh has features (including hills and bridges) that may affect the utility of distance measures for understanding access. Scientists and policy makers will only be able to discern the true effect of changing the food environment in these neighbourhoods when our longitudinal data are collected and analysed. Nevertheless, the current analysis suggests that increased geographic access may not be enough to change food purchasing practices, diet or weight, and underscores the need for continued investigation into other programmes and policies that might complement access efforts if they prove effective or replace them if they prove ineffective.
Acknowledgements
Acknowledgements: The authors wish to sincerely thank Stephanie Lonsinger who provided administrative assistance for all aspects of the study, from its implementation to analyses. These analyses would not have been possible without La’Vette Wagner, Field Coordinator, PHRESH, and our eighteen resident data collectors. Thanks also go to Scott Beach, Rob Keene, Lynda Connelly and Eric Wolfe at the University Center for Social and Urban Research, University of Pittsburgh, who assisted with the training of our data collection team and management of our survey data. Financial support: This research was supported by the National Institutes of Health (NIH)/National Cancer Institute (NCI) (grant number NCI R01CA149105: ‘Does a New Supermarket Improve Dietary Behaviors of Low-income African Americans?’). The NIH/NCI had no role in the design, analysis or writing of this article. Conflict of interest: None. Authorship: T.D. led all aspects of the study including formulating the research questions, designing and implementing the study, analysing the data and writing the article. S.N.Z. assisted with framing the research questions, interpretation of results and writing the article. B.G.-D. assisted in formulating the research questions, designing the study, leading statistical analyses and design of the sampling frame, and writing the article. D.A.C. assisted with formulating research questions, analysing the data and writing the article. R.B. and G.H. assisted with analyses and interpretation of results. E.D.S. coordinated all field research and assisted with interpretation of results. R.L.C. served as a senior advisor to formulating the research questions, interpreting results and writing the paper. Ethics of human subject participation: All study protocols were approved by the RAND Human Subjects Protection Committee.