Investigation of the relationship between dietary habits and the occurrence of cardiovascular and other conditions in population studies goes back several decades. Apart from pioneering findings produced in the early 1900s, most knowledge has accumulated in the second half of the 20th century.
Initially the main interest was focused on nutrients, while only later did the interest move to food groups and particularly dietary patterns, i.e. the overall eating habits as derived from the combination of different foods. A clear summary and hypothesis of this approach was published in 2002( Reference Hu 1 ).
In the a priori approach the dietary pattern is constructed by deciding which combination of food groups should be good or bad for health. These decisions are based on findings of studies that identify populations or subgroups of populations with different dietary habits and different amounts of disease or mortality, or on consensus statements( Reference Panagiotakos 2 ). Another way to identify a dietary pattern, one not conditioned by prejudice or nutritional knowledge, is based on mathematical approaches that do not need, at a starting point, any technical decision on the side of foods and nutrients. Examples of this a posteriori approach are cluster analysis, principal components analysis and factor analysis, where the procedure is based only on the inter-correlations among food groups or nutrients as defined by a matrix describing the population dietary habits( Reference Panagiotakos 2 ). Many studies have exploited this concept, still adopting different approaches for the definition of ‘dietary pattern’( Reference Trichopoulou, Kouris-Blazos and Wahlquist 3 – Reference Menotti, Alberti-Fidanza and Fidanza 31 ).
In the present work factor analysis on food groups was tested on individual participants of a population study with the purpose to identify dietary patterns and to explore their predictive role as possible risk factors for various morbid and fatal conditions. The null hypothesis was that factors identified by the factor analysis were not associated with the explored conditions.
Methods
Study population
The epidemiological material used for the present analysis derives from the two Italian rural cohorts of the Seven Countries Study, located at Crevalcore in northern Italy and Montegiorgio in central Italy, comprising men aged 40–59 years enrolled and first examined in 1960( Reference Keys, Blackburn and Menotti 32 ). At the start in 1960, chunk samples were defined in both areas and 1712 men were examined (98·8 % of the total roster). At the 5-year follow-up in 1965, ninety men had died and 1564 participants were re-examined.
Collection of dietary data
In 1965, food intake data were collected for all participants using the dietary history method administered by three experienced dietitians. The method is described in detail elsewhere( Reference Alberti Fidanza, Seccareccia and Torsello 33 ). Overall, thirty-nine food groups were identified but, for the purpose of the current analysis, the choice fell on seventeen of them. Those discarded were minor components of major groups (such as fresh fish, dried fish, frozen fish, canned fish and similar classifications), or minor food groups consumed in minimal quantities or used by very few individuals. The final choice roughly corresponded to a similar grouping already used in another analysis on the Seven Countries Study dietary data( Reference Menotti, Kromhout and Blackburn 7 ). They were: (i) bread; (ii) cereals (almost exclusively pasta); (iii) potatoes; (iv) vegetables (except potatoes and legumes); (v) legumes; (vi) fruit (all kinds); (vii) sugar; (viii) oils (almost exclusively olive oil); (ix) meat; (x) fish; (xi) eggs; (xii) fat (other than oils); (xiii) milk; (xiv) cheese; (xv) pastries; (xvi) alcoholic beverages (almost exclusively wine); and (xvii) sugar beverages. Average consumption levels of major nutrients were also available.
Collection of causes of death, CHD incidence and other variables
Collection of data on vital status and causes of death was complete for 40 years after the 1965 examination. Causes of death were allocated by reviewing and combining information from death certificates, hospital and medical records, interviews with physicians and relatives of the deceased and any other witness of fatal events. Causes of death were determined by a single reviewer following defined criteria, employing the WHO International Classification of Diseases and Causes of Death, 8th revision (ICD-8)( 34 ). In the presence of multiple causes, a hierarchical preference was adopted with violence, cancer in advanced stages, CHD and stroke, in that order. For the analysis the following endpoints were considered.
-
1. CHD deaths corresponding to codes 410–414 of the WHO ICD-8 plus cases of sudden death occurring within 2 h from onset of symptoms, accompanied by a secondary cause of CHD (410–414).
-
2. CVD deaths corresponding to codes 390–459 of the WHO ICD-8 (including also those defined CHD as above).
-
3. Cancer deaths corresponding to codes 140–239 of the WHO ICD-8.
-
4. All-cause mortality corresponding to any code of the WHO ICD-8.
Incidence of major CHD events was collected for the first 20 years of follow-up and was based on repeated field examinations, information obtained from local hospitals and physicians, review of clinical records and the availability of causes of death. Major CHD events, based on standard criteria( Reference Menotti, Alberti-Fidanza and Fidanza 31 ), were coronary deaths (both sudden and non-sudden) and fatal and non-fatal definite myocardial infarction.
The analysis was run on 1221 individuals with complete data on consumption of the seventeen food groups and on five other risk factors as possible confounders. The denominator for mortality endpoints was 1221, while the events were 187 for CHD, 513 for CVD, 324 for cancer and 1148 for all-cause mortality. After discarding cases with prevalent CHD, the denominator for CHD incidence in 20 years was 1153 and the events were 185.
For the purpose of the analysis, five risk factors measured at the same examination (1965) were considered as possible confounders.
-
1. Age, in years, rounded off to the nearest birthday.
-
2. Smoking habits expressed as dummy variables (0, 1) for ex-smokers and current smokers, with never smokers as reference.
-
3. Systolic blood pressure measured in mmHg at the right arm in supine position, at the end of a physical examination, by trained physicians using mercury sphygmomanometers and following the procedure later described in the manual on Cardiovascular Survey Methods (WHO Manual)( Reference Rose and Blackburn 35 ). Two readings approximated to the nearest 2 mmHg were made one minute apart and averaged.
-
4. Serum cholesterol, in mmol/l, measured on casual blood samples using the method described by Anderson and Keys and summarized in the WHO Manual( Reference Rose and Blackburn 35 ).
-
5. BMI (kg/m2) computed from height and weight measured following the procedure later reported in the WHO Manual( Reference Rose and Blackburn 35 ).
Baseline data were collected before the era of the Helsinki Declaration. Subsequently, oral informed consent was obtained in view of collecting follow-up data.
Statistical analysis
As a preliminary description of the study population, mean levels and standard deviations of risk factors were computed.
The main analysis was based on factor analysis, a statistical modelling technique used to describe variability among observed variables with the purpose to identify a lower number of unobserved variables called factors. In fact, a number of variables may potentially represent another unobserved variable. The observed variables are modelled as linear combinations of the potential factors and the information related to the interdependencies among the observed variables is used to reduce the set of variables( Reference Kim and Mueller 36 – Reference Bryant and Yamold 38 ). The meaning and definition of some terms employed in the present paper are reported in the Appendix.
Food group consumption (originally expressed in g/d) was adjusted by body weight (g food/kg body weight per d). Log transformation was discarded since results using various techniques of transformation (putting the 0 values equal to 1, 0·5, 0·1 or 0·01) produced similar findings to using untransformed data.
Factor analysis was performed, and then varimax rotation applied. The number of factors was not known and the choice was based on the use of the Kaiser criterion and the Cattel scree plot. For each factor, eigenvalues, factor loadings and factor score coefficients were produced by the computer program. Factors were identified by variables having a factor loading of 0·250 or more. Factor scores for each individual and for each factor were also produced by the program and then used as potential independent variables (risk factors) in Cox proportional hazards models for prediction of events. The events were CHD incidence in 20 years, and mortality from CHD, all CVD, cancer and all causes in 40 years. Factor scores from three factors were fed into the same models together with the covariates, producing one model for each endpoint.
Mean values of food groups, again adjusted by body weight, were computed in each quintile of Factor 2 score distribution. The overall quinquennial age distribution of the entire cohort was then used as reference population for re-computing the age-adjusted food group consumption in each quintile. Tests were made to compare food consumption in the extreme quintiles and across them (t test and test of trends).
Life expectancy was computed using the truncated length of survival within 40 years. The crude survival was estimated in quintile classes of Factor 2 score. Then the overall quinquennial age distribution of the entire cohort was used as reference population for re-computing the age-adjusted survival in each quintile.
A correlation matrix showed that only age had some moderate inverse correlation with the factor score, which was not the case for the other covariates.
The whole analysis was replicated employing principal components analysis. Analyses were run using the NCSS 2007 statistical and power analysis software (NCSS, Kaysville, UT, USA).
Results
Some general characteristics of the study population are reported in Table 1, with mean levels of the considered risk factors. The diet was characterized by a high average energy intake of 12 226 kJ (2921 kcal), with 11·4 % of energy from protein, 26·2 % from fat, 49·3 % from carbohydrates and 13·1 % from alcohol.
Table 1 Mean values of some risk factors in the study population: men aged 45–64 years (n 1221) from rural villages in northern and central Italy
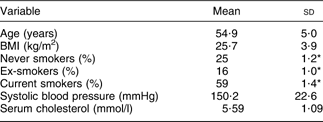
Variable | Mean | sd |
Age (years) | 54·9 | 5·0 |
BMI (kg/m2) | 25·7 | 3·9 |
Never smokers (%) | 25 | 1·2* |
Ex-smokers (%) | 16 | 1·0* |
Current smokers (%) | 59 | 1·4* |
Systolic blood pressure (mmHg) | 150·2 | 22·6 |
Serum cholesterol (mmol/l) | 5·59 | 1·09 |
*Standard error.
Seven models were tested with extraction of eight down to two factors. Following the Kaiser criterion, in the model with three factors, eigenvalues >1 were found for factors 1 and 2, while factor 3 had a value of 0·56. On the other hand, the Cattel scree plot, again for the model with three factors, showed a clear cliff of eigenvalues until factor 3, followed by an elbow and a flattening of the curve. At the end three factors were retained. The extraction of the first two factors covered 82 % of variability.
Table 2 reports the factor loadings and the factor score coefficients for the seventeen food groups within the three factors. The factor score coefficients were strongly correlated with factor loadings. The factor scores of the three factors were largely independent, with correlation coefficients of −0·072 between Factor 1 and Factor 2; 0·065 between Factor 1 and Factor 3; and 0·221 between Factor 2 and Factor 3. The structure of the three factors is summarized below Table 2. The ‘dominant’ food groups in Factor 1 were sugar, milk, meat, fruit, pastries and cheese, in opposition to Factor 2 where the dominant groups were bread, cereals, vegetables, fish, potatoes and oils. Factor 3 had a different not so typical structure including only eggs and alcoholic beverages. Factor 1 seemed rich in sugar and animal foods, while Factor 2 seemed rich in vegetable foods and fish.
Table 2 Factor loadings and factor score coefficients estimated by factor analysis after extraction of three factors on seventeen food groups: men aged 45–64 years (n 1221) from rural villages in northern and central Italy
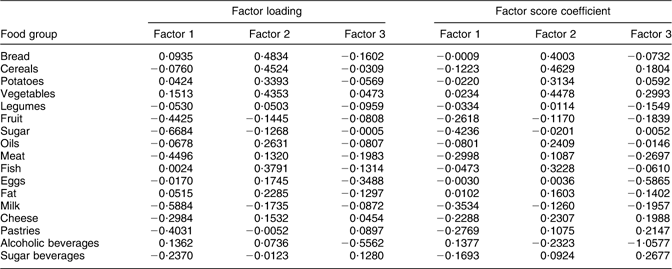
Factor loading | Factor score coefficient | |||||
Food group | Factor 1 | Factor 2 | Factor 3 | Factor 1 | Factor 2 | Factor 3 |
Bread | 0·0935 | 0·4834 | −0·1602 | −0·0009 | 0·4003 | −0·0732 |
Cereals | −0·0760 | 0·4524 | −0·0309 | −0·1223 | 0·4629 | 0·1804 |
Potatoes | 0·0424 | 0·3393 | −0·0569 | −0·0220 | 0·3134 | 0·0592 |
Vegetables | 0·1513 | 0·4353 | 0·0473 | 0·0234 | 0·4478 | 0·2993 |
Legumes | −0·0530 | 0·0503 | −0·0959 | −0·0334 | 0·0114 | −0·1549 |
Fruit | −0·4425 | −0·1445 | −0·0808 | −0·2618 | −0·1170 | −0·1839 |
Sugar | −0·6684 | −0·1268 | −0·0005 | −0·4236 | −0·0201 | 0·0052 |
Oils | −0·0678 | 0·2631 | −0·0807 | −0·0801 | 0·2409 | −0·0146 |
Meat | −0·4496 | 0·1320 | −0·1983 | −0·2998 | 0·1087 | −0·2697 |
Fish | 0·0024 | 0·3791 | −0·1314 | −0·0473 | 0·3228 | −0·0610 |
Eggs | −0·0170 | 0·1745 | −0·3488 | −0·0030 | 0·0036 | −0·5865 |
Fat | 0·0515 | 0·2285 | −0·1297 | 0·0102 | 0·1603 | −0·1402 |
Milk | −0·5884 | −0·1735 | −0·0872 | −0·3534 | −0·1260 | −0·1957 |
Cheese | −0·2984 | 0·1532 | 0·0454 | −0·2288 | 0·2307 | 0·1988 |
Pastries | −0·4031 | −0·0052 | 0·0897 | −0·2769 | 0·1075 | 0·2147 |
Alcoholic beverages | 0·1362 | 0·0736 | −0·5562 | 0·1377 | −0·2323 | −1·0577 |
Sugar beverages | −0·2370 | −0·0123 | 0·1280 | −0·1693 | 0·0924 | 0·2677 |
Structure of factors is based on a factor loading of 0·250 or more.
Factor 1: sugar, milk, meat, fruit, pastries, cheese.
Factor 2: bread, cereals, vegetables, fish, potatoes, oils.
Factor 3: eggs, alcoholic beverages.
Correlation coefficient between factor loading and factor score: Factor 1 = 0·978; Factor 2 = 0·873; Factor 3 = 0·964.
Table 3 provides a summary of models including the three factors together and the other covariates. The evident systematic finding was the inverse (protective) and significant role of Factor 2 score for all five endpoints. Factor 1 score was never significant, while Factor 3 score was so only for cancer deaths in 40 years. Factor 2 score was inversely related with all endpoints with significant hazard ratios ranging from 0·79 to 0·88. Among the covariates, age and current smokers were always statistically significant; ex-smokers only for CVD and all-cause mortality in 40 years; systolic blood pressure was significant for all endpoints except cancer deaths; serum cholesterol was significant only for CHD incidence and CHD deaths; in no case was BMI statistically significant.
Table 3 Solutions of Cox proportional hazards models including scores for the three factors and the covariates: men aged 45–64 years (n 1221) from rural villages in northern and central Italy
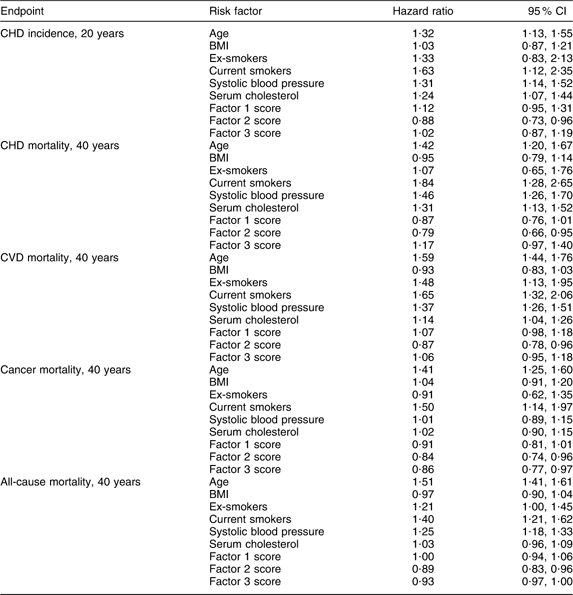
Endpoint | Risk factor | Hazard ratio | 95 % CI |
CHD incidence, 20 years | Age | 1·32 | 1·13, 1·55 |
BMI | 1·03 | 0·87, 1·21 | |
Ex-smokers | 1·33 | 0·83, 2·13 | |
Current smokers | 1·63 | 1·12, 2·35 | |
Systolic blood pressure | 1·31 | 1·14, 1·52 | |
Serum cholesterol | 1·24 | 1·07, 1·44 | |
Factor 1 score | 1·12 | 0·95, 1·31 | |
Factor 2 score | 0·88 | 0·73, 0·96 | |
Factor 3 score | 1·02 | 0·87, 1·19 | |
CHD mortality, 40 years | Age | 1·42 | 1·20, 1·67 |
BMI | 0·95 | 0·79, 1·14 | |
Ex-smokers | 1·07 | 0·65, 1·76 | |
Current smokers | 1·84 | 1·28, 2·65 | |
Systolic blood pressure | 1·46 | 1·26, 1·70 | |
Serum cholesterol | 1·31 | 1·13, 1·52 | |
Factor 1 score | 0·87 | 0·76, 1·01 | |
Factor 2 score | 0·79 | 0·66, 0·95 | |
Factor 3 score | 1·17 | 0·97, 1·40 | |
CVD mortality, 40 years | Age | 1·59 | 1·44, 1·76 |
BMI | 0·93 | 0·83, 1·03 | |
Ex-smokers | 1·48 | 1·13, 1·95 | |
Current smokers | 1·65 | 1·32, 2·06 | |
Systolic blood pressure | 1·37 | 1·26, 1·51 | |
Serum cholesterol | 1·14 | 1·04, 1·26 | |
Factor 1 score | 1·07 | 0·98, 1·18 | |
Factor 2 score | 0·87 | 0·78, 0·96 | |
Factor 3 score | 1·06 | 0·95, 1·18 | |
Cancer mortality, 40 years | Age | 1·41 | 1·25, 1·60 |
BMI | 1·04 | 0·91, 1·20 | |
Ex-smokers | 0·91 | 0·62, 1·35 | |
Current smokers | 1·50 | 1·14, 1·97 | |
Systolic blood pressure | 1·01 | 0·89, 1·15 | |
Serum cholesterol | 1·02 | 0·90, 1·15 | |
Factor 1 score | 0·91 | 0·81, 1·01 | |
Factor 2 score | 0·84 | 0·74, 0·96 | |
Factor 3 score | 0·86 | 0·77, 0·97 | |
All-cause mortality, 40 years | Age | 1·51 | 1·41, 1·61 |
BMI | 0·97 | 0·90, 1·04 | |
Ex-smokers | 1·21 | 1·00, 1·45 | |
Current smokers | 1·40 | 1·21, 1·62 | |
Systolic blood pressure | 1·25 | 1·18, 1·33 | |
Serum cholesterol | 1·03 | 0·96, 1·09 | |
Factor 1 score | 1·00 | 0·94, 1·06 | |
Factor 2 score | 0·89 | 0·83, 0·96 | |
Factor 3 score | 0·93 | 0·97, 1·00 |
Hazards ratios are based on 1 sd, except for smoking habits (0, 1). Never smokers are the reference for ex-smokers and current smokers.
It became natural to concentrate on Factor 2 and try to explain its meaning. Factor scores do not have a unit of measurement. However, the average Factor 2 score was 0·00618, its standard deviation was 1·3750, with a minimum of −3·0421 and a maximum of 9·4738, and a reasonably normal distribution. High levels of Factor 2 (corresponding to its protective role) were characterized by high consumption of vegetables, potatoes, fish, bread, cereals and oils. This was partly true also for fat and cheese. Low levels of Factor 2 were characterized by an opposite (specular) pattern of food consumption. Examples of this are reported in Table 4, where several indicators point to the contrast between dietary habits of individuals in the highest v. the lowest quintile of the factor score distribution. For example, it is of interest to find that consumption of bread, cereals, potatoes, vegetables and fish is two to four times greater in men located in quintile 5 compared with men located in quintile 1. Consumption of oils was also much greater in quintile 5.
Table 4 Age-adjusted consumption of some food groups in quintile classes of factor score derived from Factor 2: men aged 45–64 years (n 1221) from rural villages in northern and central Italy
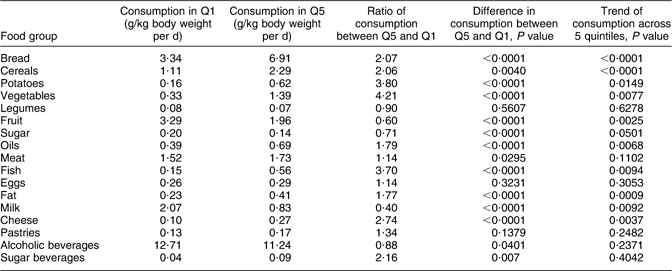
Food group | Consumption in Q1 (g/kg body weight per d) | Consumption in Q5 (g/kg body weight per d) | Ratio of consumption between Q5 and Q1 | Difference in consumption between Q5 and Q1, P value | Trend of consumption across 5 quintiles, P value |
Bread | 3·34 | 6·91 | 2·07 | <0·0001 | <0·0001 |
Cereals | 1·11 | 2·29 | 2·06 | 0·0040 | <0·0001 |
Potatoes | 0·16 | 0·62 | 3·80 | <0·0001 | 0·0149 |
Vegetables | 0·33 | 1·39 | 4·21 | <0·0001 | 0·0077 |
Legumes | 0·08 | 0·07 | 0·90 | 0·5607 | 0·6278 |
Fruit | 3·29 | 1·96 | 0·60 | <0·0001 | 0·0025 |
Sugar | 0·20 | 0·14 | 0·71 | <0·0001 | 0·0501 |
Oils | 0·39 | 0·69 | 1·79 | <0·0001 | 0·0068 |
Meat | 1·52 | 1·73 | 1·14 | 0·0295 | 0·1102 |
Fish | 0·15 | 0·56 | 3·70 | <0·0001 | 0·0094 |
Eggs | 0·26 | 0·29 | 1·14 | 0·3231 | 0·3053 |
Fat | 0·23 | 0·41 | 1·77 | <0·0001 | 0·0009 |
Milk | 2·07 | 0·83 | 0·40 | <0·0001 | 0·0092 |
Cheese | 0·10 | 0·27 | 2·74 | <0·0001 | 0·0037 |
Pastries | 0·13 | 0·17 | 1·34 | 0·1379 | 0·2482 |
Alcoholic beverages | 12·71 | 11·24 | 0·88 | 0·0401 | 0·2371 |
Sugar beverages | 0·04 | 0·09 | 2·16 | 0·007 | 0·4042 |
Q1, lowest quintile; Q5, highest quintile.
In the case of the higher consumption of fat in quintile 5 we could not explore the situation in more detail, but in general the fat consumption was moderate being only a little more than half compared with the consumption of oils. On the other hand, the situation of cheese could be investigated more deeply considering the role of fat v. lean cheese. We found that moving from quintile 1 to quintile 5 of Factor 2 score the proportion of lean cheese in the overall cheese consumption increased more than linearly, probably offering a partial counteraction v. fat cheese. For example, the contribution of lean cheese to the overall cheese consumption in the lowest quintile of Factor Score 2 was negligible, while it became 6 % in quintile 5.
The age-adjusted life expectancy within 40 years was 17·7 years in quintile 1 of Factor 2 score and increased up to 21·8 years in quintile 5. The difference of 4·1 years between quintile 5 and quintile 1 was statistically significant.
When the analysis was replicated using principal components analysis adopting the same data and criteria, the results were substantially identical, which means that the error terms in the factor analysis model (the variability not explained by common factors) can be assumed to have all the same variance. The correlation coefficients between each pair of factor scores (in factor analysis and in principal components analysis) were 0·99, with intercepts close to zero.
Discussion
In the present work, the classification of food consumption and pattern provided by Factor 2 in the process of factor analysis was highly and inversely associated with incidence of CHD and mortality from various causes and all causes. The outcome was bound only to the findings offered by the factor analysis, without any prejudice about the role of the different foods and their combinations.
All this means that the inter-correlations among consumption of food groups hide food patterns that are chosen by free-living populations, or subgroups of populations. The factor score derived from Factor 2 does not provide an immediate indication of the food pattern, unless we proceed to identify a portion of the distribution and through it the average food group consumption for that specific subgroup. There is no way, at this stage, to know the reasons for those choices, which were – anyhow – good or bad for health and survival. The pattern of the diet in individuals with high values of Factor 2 score was similar to the pattern considered a typical Mediterranean diet, rich in vegetables foods, oil and fish.
In the present analysis the predictive power of multivariate coefficients for Factor 2 score was statistically significant in the presence of other important covariates, such as age, smoking habits, blood pressure, serum cholesterol and BMI.
The literature provides many reports on dietary patterns and their relationships with disease or mortality. In some of them, the pattern was constructed a priori( Reference Trichopoulou, Kouris-Blazos and Wahlquist 3 – Reference Ocke, Bueno-de-Mesquita and Feskens 6 , Reference Trichopoulou, Costacou and Bamia 9 – Reference Lagiou, Trichopoulos and Sandin 15 , Reference Mitrou, Kipnis and Thiébaut 17 , Reference Bamia, Trichopoulos and Ferrari 19 , Reference Panagiotakos, Pitsavos and Stefanadis 26 , Reference Martínez-González, García-López and Bes-Rastrollo 29 , Reference Menotti, Alberti-Fidanza and Fidanza 31 ). More recently the definition of the dietary pattern was made a posteriori by mathematical procedures such as cluster analysis, principal components analysis, factor analysis and reduced rank regression( Reference Menotti, Kromhout and Blackburn 7 , Reference Osler, Helm Andreasen and Heitmann 8 , Reference Waijers, Ocké and van Rossum 16 , Reference Masala, Ceroti and Pala 18 , Reference Shimazu, Kuriyama and Hozawa 20 , Reference DiBello, Kraft and McGarvey 21 – Reference Héroux, Janssen and Lam 28 , Reference Guallar-Castillón, Rodríguez-Artalejo and Tormo 30 ). Both approaches provided similar findings when the pattern was tested against morbidity and/or mortality, with benefits bound to characteristics defined as ‘Mediterranean diets’ or to the prevalence of plant foods over animal foods. Similar findings were identified in population samples of different countries, such as Belgium( Reference Bazelmans, De Henauw and Matthys 13 ), Canada( Reference Héroux, Janssen and Lam 28 ), Costa Rica( Reference DiBello, Kraft and McGarvey 21 ), Denmark( Reference Osler, Helm Andreasen and Heitmann 8 ), Great Britain( Reference Brunner, Mosdøl and Witte 23 , Reference McNaughton, Mishra and Brunner 27 ), Greece( Reference Trichopoulou, Kouris-Blazos and Wahlquist 3 , Reference Trichopoulou, Costacou and Bamia 9 , Reference Panagiotakos, Pitsavos and Chrysohoou 24 , Reference Panagiotakos, Pitsavos and Stefanadis 26 ), Italy( Reference Farchi, Fidanza and Grossi 4 , Reference Massari, Freeman and Seccareccia 10 , Reference Masala, Ceroti and Pala 18 , Reference Menotti, Alberti-Fidanza and Fidanza 31 ), Japan( Reference Shimazu, Kuriyama and Hozawa 20 ), the Netherlands( Reference Waijers, Ocké and van Rossum 16 ), Spain( Reference Martínez-González, García-López and Bes-Rastrollo 29 , Reference Guallar-Castillón, Rodríguez-Artalejo and Tormo 30 ), Sweden( Reference Lagiou, Trichopoulos and Sandin 15 ) and the USA( Reference Mitrou, Kipnis and Thiébaut 17 , Reference Heidemann, Schulze and Franco 22 ), and pooling or comparing different countries( Reference Huijbregts, Feskens and Rasanen 5 – Reference Menotti, Kromhout and Blackburn 7 , Reference Fidanza, Alberti and Lanti 11 , Reference Knoops, deGroot and Fidanza 14 , Reference Bamia, Trichopoulos and Ferrari 19 , Reference da Silva, Bach-Faig and Quintana 25 ). This suggests that some dietary characteristics may have protective effects in rather different populations and cultures and be related to CHD, CVD and all-cause mortality.
Altogether, the performance of our score is not too different from those of other food pattern scores reported in the literature, either defined arbitrarily or by mathematical procedures, and this despite the differences in statistical approaches, criteria for the definition of dietary pattern, endpoints and length of follow-up.
This conceptual variety and the use of different units of measure make it impossible or difficult to compare in a standardized way the various indices and the strength of their association with disease or mortality outcome across different studies. Despite the uncertainties mentioned above, there has been an attempt to produce a meta-analysis of studies centred on the Mediterranean diet, that apparently confirmed the protective power of those eating habits( Reference Sofi, Cesari and Abbate 39 ).
A limitation of our analysis was bound to the small denominator, only partially compensated by the long follow-up period that reached 40 years, a term that is unusual for all other contributions. For the same reason it was impossible to produce a separate analysis for the two rural communities. Moreover, the analysis could not take into account the possible changes of dietary habits along the years. On the other hand, possible changes should have been limited considering the initial age of the individuals and the rural environment which might be more resistant to lifestyle changes, at least starting from the 1960s.
Conclusions
A dietary pattern index derived a posteriori from a mathematical procedure was inversely and significantly associated with CHD incidence in 20 years and mortality from CHD, CVD, cancer and all causes in 40 years of follow-up. This association was shown to be independent of the possible confounding effect of age, cigarette smoking, systolic blood pressure, serum cholesterol and BMI, confirming the healthiness of some eating habits.
Acknowledgements
This research received no specific grant from any funding agency in the public, commercial or not-for-profit sectors. The authors do not have conflicts of interest. The authors’ contributions were as follows: A.M., study concept and design, part of statistical analysis, most of text writing; A.A.-F., collection of dietary data and their classification, critical review of manuscript; F.F., collection of dietary data and their classification, part of study concept and design, critical review of manuscript; M.L., part of statistical analysis, part of text writing; D.F., data handling, organization of files, classification of dietary data, critical review of manuscript.
Appendix
Meaning and definition of some terms employed in the present paper
Since factor analysis is not so widely known and its concepts and terminology are sometimes contradictory, some terms employed in the present analysis are defined below.
Factor. A factor is made by a linear combination of the original variables and represents the underlying dimension that summarizes or accounts for the original observed variables.
Extraction of a factor. This is the stage of factor analysis in which the covariance matrix is resolved into a smaller number of underlying factors or components.
Varimax rotation. This is an optional (but strongly suggested) orthogonal rotation of the factor axes that maximizes the variance of the squared loading of a factor on all of the variables in a factor matrix. It has the effect of differentiating the original variables by extracted factor and therefore each factor tends to have either a large or a small loading of any particular variable.
Eigenvalue. The eigenvalue is the column sum of the squared loadings for a factor and represents the amount of variance accounted for by a factor.
Factor loadings. These are the correlation coefficients between the standardized variables and the factors. A high loading suggests that independent variables are represented by a particular factor.
Factor score coefficients. These are the coefficients – regression weights – of the variables used in the construction of the factor, that allow also to estimate the selected factor for each observation.
Factor scores. These are the scores, for each case and for each factor, computed by exploiting the factor score coefficients, i.e. the estimate for a case of an underlying factor formed from the linear combination of the observed variables. This means that each factor becomes a numerical characteristic of each individual that can be used as a variable in subsequent modeling.