Residential segregation occurs when two or more social groups of people, categorized by a variety of scales (race, ethnicity, skin colour or socio-economic status (e.g. income)), live separately from one another, in different parts of the urban environment, and with different spatial distribution(Reference Massey and Denton1). Accordingly, racial residential segregation is a physical separation of the races by residence. Along these lines, economic residential segregation is a physical separation of two or more groups of people based on their income by residence (into homogeneous neighbourhoods)(Reference Massey and Denton1, Reference Williams and Collins2).
Segregated spaces, categorized by race or income, promote varied exposure to health issues, especially when comparing the less with the more affluent and integrated neighbourhoods(Reference Goodman, Lyons and Dean3–Reference Kershaw, Osypuk and Do7). As a result, segregation is one possible mechanism of racism, the spatial manifestation of inequalities and one of the fundamental causes of disparities in health(Reference Williams and Collins2).
Associations between racially segregated neighbourhoods and proximity to availability of ultra-processed foods (fast foods, soft drinks and frozen meals) have been reported in the literature. In the USA, poor and predominantly black neighbourhoods are geographically associated with fast-food restaurants(Reference Ball, Timperio and Crawford8) and are further from stores selling healthy foods(Reference Bower, Thorpe and Rohde9–Reference Zenk, Schulz and Israel11). In Brazil, scientific evidence has revealed the association between economic disadvantage and concentration of healthy food stores in affluent areas(Reference Lopes, Menezes and Araújo12, Reference Duran, Roux and Latorre13).
The characteristics of a neighbourhood regarding access to food may be a factor contributing to differences in food consumption among residents, probably linking racially segregated urban neighbourhoods to disparities in food consumption(Reference Morland and Filomena10). Some studies showed a relationship between racial/ethnic segregation and diets with lower amounts of fat and processed foods(Reference Park, Neckerman and Quinn14, Reference Osypuk, Diez Roux and Hadley15), high expenditure on fruits and vegetables(Reference Ryabov16) and higher sodium and potassium intakes(Reference Yi, Ruff and Jung17). However, the mechanisms explaining the associations between food consumption and economic residential segregation have not been fully understood.
The literature tends to focus on racial/ethnic segregation(Reference Yi, Ruff and Jung17, Reference Corral, Landrine and Hao18), and there are few recent studies on social class/economic residential segregation in Brazil(Reference Barber, Diez-Roux and Cardoso19). Studies on racial/ethnic segregation and food consumption have been conducted in the USA, but it is not clear if the mechanisms are similar in low- and middle-income country contexts. In Brazil, for instance, socio-economic classes are more commonly used in studies, because the Brazilian racial classification is very complex and makes it difficult to transfer common measures from the USA context to Brazil(Reference Barber, Diez-Roux and Cardoso19).
Calculating the economic residential segregation in an urban area, represented by the 6th most populous Brazilian city, testing whether residing in high-segregation neighbourhoods is associated with food consumption can contribute to the understanding of how residential segregation occurs in a city with lower-middle-income economy and how it is associated with disparities in food consumption. Besides, this may support evidence-based policies and interventions.
We hypothesized that individuals residing in highly segregated areas would report a lower prevalence of regular consumption of foods such as fruits, vegetables and fish, and higher or equal prevalence of regular consumption of foods such as soft drinks, artificial juice, sweet foods and/or other ultra-processed food products, in comparison to those residing in low-segregated areas. We also hypothesized the possible exception of beans, since different Brazilian studies indicate a higher prevalence of regular bean consumption among lower-income populations(Reference Jaime, Stopa and Oliveira20, Reference Malta, Iser and Santos21).
Regarding the replacement of lunch or dinner, although lacking a formal hypothesis, we aimed to better understand this very contemporary food behaviour in the Brazilian urban context. Thus, in order to expand our knowledge and understanding of the relationship between economic residential segregation and food consumption, the aim of the present study was to examine associations between the prevalence of healthy and unhealthy eating markers and economic residential segregation.
Methods
Study population
The current analysis examined data from the ‘MOVE-se Academias (2014–2015) study’, a cross-sectional study conducted by the Belo Horizonte Observatory for Urban Health of the Universidade Federal de Minas Gerais. The MOVE-se Academias study integrates the project ‘Health Academy: evaluation of physical activity promotion programs in Brazil’. It consists of a home-based health survey, for which the sample includes the resident population (users and non-users) in the geographical environment of the Health Academy Program (HAP; Programa Academia da Saúde (PAS) in Portuguese), distributed among the nine health districts of Belo Horizonte(Reference Fernandes, Andrade and Costa22).
The HAP are public spaces that provide – for free, under the Unified Health System – physical activity programmes, health promotion initiatives including eating and other community education activities to users over 18 years of age(23).
The HAP was implemented in Belo Horizonte in 2006, through participatory mechanisms mostly, and therefore is located primarily in vulnerable areas. Currently, there are seventy-eight units with maximum capacity estimated at 400 users per unit(23, Reference Caiaffa, Friche, Galea, Ettman and Vlahov24).
Study sample
A probabilistic sample design was adopted to select the population using clusters in three stages: (i) ten HAP units were randomly selected from the list provided by the programme management, in which three of them (inherited from a previous study in 2008–2009) had probability equal to 1; (ii) census tracts, selected with distinct probabilities according to the proximity of the HAP (e.g. census tracts located at the HAP unit had probability of 1; census tracts located up to 500 m from any other unit had 2·4 greater chance of being selected in comparison to a unit 500 m away) and with sample size proportional to the total number of sectors surrounding each sampled HAP unit; and (iii) households, selected by means of systematic sampling based on the number of households per census tract of the Census 2010. In each household, one adult resident (aged 18 years or more) was selected according to the quota established by sex, age range and occupation.
HAP units were eligible if they met the following criteria: (i) located in the districts that participated in the baseline survey of 2008–2009; (ii) were implemented before the first semester of 2013; and (iii) were not directed to special groups or located in specific places, such as the university.
The addresses of the selected HAP units were georeferenced, and the Euclidean distances between the centroid of each census tract and the nearest HAP unit were calculated. The eligible census tracts were: (i) within a radius of 1000 m of the ten HAP units; and (ii) with more than fifty households. Out of the eligible census tracts, 246 were selected. Census tracts with only one interview were excluded (n 68), totalling seventy-five participants. Household interviewees – either included or excluded – did not differ in the analytic sample with regard to sex, age, income and education. A detailed description of the Move-se Academias study design and survey has been published elsewhere(Reference Fernandes, Andrade and Costa22).
For the purpose of the present study only non-users living in the geographical areas of the ten HAP units were included, since HAP users were dissimilar from non-users in several health aspects, including food consumption and physical activity(Reference Fernandes, Andrade and Costa22). The final sample totalled 1301 participants distributed in 178 census tracts.
Data collection
Household face-to-face interviews and anthropometric measurements (weight and height) were conducted by trained interviewers, with a questionnaire gathering sociodemographic, health habits and behaviour information.
Measures
Residential segregation
The unit of analysis adopted was the census tract. The 2010 Brazil Census data(25) were used to determine the proportion of heads of household in a census tract earning a monthly income of 0–3 minimum wages (approximately 0 to $US 900·00, in 2010), based on prior observational studies of economic segregation in Brazil(Reference Barber, Diez-Roux and Cardoso19, Reference Torres26). The cut-off point, 0–3 minimum wages, also corresponded to the average monthly income of Brazilians in 2015(27), the year of the survey, and the median income of the sample selected for the Move-se Academias study.
Economic residential segregation was calculated for all census tracts of the city using the Hot Spot Analysis Tool in ArcGIS version 10.3. Census tracts were excluded if the resident population was zero, or if data were omitted by the Census 2010 because of safety reasons, or if the total number of heads of household with a monthly income of 0–3 minimum wages was zero. A total of 3833 tracts of Belo Horizonte city were analysed; from these, 178 tracts were analysed in the Move-se Academias study in order to examine the association between economic residential segregation and individual food consumption.
The Getis–Ord local $G_i^{\rm{\ast}}$ statistic (‘
$G_i^{\rm{\ast}}$ statistic’ hereafter) was used to measure the spatial association and to evaluate the spatial clustering of high or low values among census tracts (i.e. census tract with higher proportion of households with 0–3 minimum-wage income surrounded by other census tracts with higher proportion of households with 0–3 minimum-wage income)(Reference Getis and Ord28, Reference Getis and Ord29). The
$G_i^{\rm{\ast}}$ statistic is a spatially weighted Z-score representing how much a neighbourhood’s (census tract) income composition (proportion of households with 0–3 minimum-wage income) deviates from the larger city (Belo Horizonte).
Census tracts were weighted using a first-order rook spatial weight matrix. Higher and positive Z-scores represent census tracts that are more segregated (i.e. census tract with higher proportion of households with 0–3 minimum-wage income surrounded by other census tracts with a higher proportion of households with 0–3 minimum-wage income or over-representation of the high proportion of heads of household in a neighbourhood earning a monthly income of 0–3 minimum wages), while lower and negative scores account for census tracts that are less segregated (i.e. census tract with a lower proportion of households with 0–3 minimum-wage income surrounded by other census tracts with lower proportion of households with 0–3 minimum-wage income or under-representation of the high proportion of heads of household in a neighbourhood earning a monthly income of 0–3 minimum wages).
Three categories of residential segregation were created: high, $G_i^{\rm{\ast}}$statistic ≥1·96; medium,
$G_i^{\rm{\ast}}$ statistic between 0 and 1·96; and low,
$G_i^{\rm{\ast}}$ statistic <0. The high segregation category corresponds to statistically significant clustering of high values (α = 0·05) and the low segregation category corresponds to the absence of any clustering (integrated neighbourhoods) or areas in which the group of heads of household earning a monthly income of 0–3 minimum wages is significantly under-represented, clustering of low values (
$G_i^{\rm{\ast}}$ statistic <−1·96). These categories were combined since the frequency of occurrence of low category described above was very small(Reference Kershaw, Osypuk and Do7).
Individual-level outcome
Healthy eating markers were defined as regular consumption of fruits (five or more times per week), vegetables (five or more times per week), beans (five or more times per week) and fish (at least once per week). On the other hand, unhealthy eating markers were defined as consumption of meat or chicken with excess fat (individuals who reported consuming meat with excessive fat and/or chicken with skin); regular consumption of soft drinks or artificial juice (five or more times per week); regular consumption of sweet foods including cakes, chocolates, cookies and candy (five or more times per week); and having sandwiches, pizzas or other ultra-processed food products for lunch or dinner (seven days per week).
The ‘regular’ classification was based on the Surveillance System for Risk and Protection Factors for Chronic Diseases by Telephone Survey (VIGITEL)(30). The seven-day cut-off point for the variable ‘regular replacement of lunch or dinner with snacks’ was used as a marker of a more specific and selected habit in order to be a proxy for those individuals whose meal-replacement habit occurs every day, which is considered not healthy(31).
The food eating markers above, considering the risk or protection factors for the occurrence of chronic non-communicable diseases, were based on the following questions employed by the National Health Survey (NHS) conducted in Brazil in 2013(32):
1. ‘How many days a week do you usually eat fruit? (a) 1–2, (b) 3–4, (c) 5–6, (d) Every day, (e) Almost never, (f) Never’.
2. ‘How many days of the week do you usually eat vegetables, such as lettuce, tomato, cabbage, carrots, chayote (not including potato, cassava or yam)? (a) 1–2, (b) 3–4, (c) 5–6, (d) Every day, (e) Almost never, (f) Never’.
3. ‘How many days a week do you usually eat beans? (a) 1–2, (b) 3–4, (c) 5–6, (d) Every day, (e) Almost never, (f) Never’.
4. ‘How many days a week do you usually eat fish? (a) 1–2, (b) 3–4, (c) 5–6, (d) Every day, (e) Almost never, (f) Never’.
5. ‘When you eat red meat, do you usually: (a) Cut off excess of visible fat, (b) Eat it with fat?’; ‘When you eat chicken, do you usually: (a) Cut off the skin, (b) Eat it with skin?’.
6. ‘How many days a week do you usually drink soft drinks (or artificial juice)? (a) 1–2, (b) 3–4, (c) 5–6, (d) Every day, (e) Almost never, (f) Never’.
7. ‘How many days a week do you usually eat sweet foods, such as slices of cake or pie, chocolates, sweets, biscuits or sweet biscuits? (a) 1–2, (b) 3–4, (c) 5–6, (d) Every day, (e) Almost never, (f) Never’.
8. ‘How many days a week do you replace lunch or dinner meals by sandwiches, or pizza? (a) 1–2, (b) 3–4, (c) 5–6, (d) Every day, (e) Almost never, (f) Never’.
These indicators are shared by the VIGITEL and the NHS(Reference Malta, Iser and Santos21). Thus, although no study on the data collected by the NHS has been conducted yet, these indicators had their validity analysed by VIGITEL(Reference Mendes, Campos and Malta33–Reference Neves, Gonzaga and Martens35).
Individual-level covariates
Individual sociodemographic characteristics included age (in years), sex (male or female), race/skin colour based on the 2010 Brazil Census questions (white, brown (pardos in Portuguese) and black), monthly income in minimum wages (in Brazilian Reals) and schooling level (0–4, 5–8, 9–11 and >12 years). In addition, we included health behaviours and biological risk factors that could confound the association between segregation and food consumption, such as: length of residence in the neighbourhood (years); health status (self-rated health); practice of physical activities(32) (‘Has practiced some type of physical exercise or sports in the last three months? Yes or No’); and nutritional status based on BMI.
BMI was calculated by dividing the patients’ measured weight (in kilograms) by the square of their measured height (in metres). Excess weight was categorized as BMI ≥ 25 kg/m2 (overweight) and BMI ≥ 30 kg/m2 (obesity)(36).
Finally, we included the perceived neighbourhood food environment, which could confound or partially mediate the association between segregation and food consumption. The perceived neighbourhood food environment was assessed using a three-item scale about variety, quality and price of fruits and vegetables: (i) ‘In your neighbourhood, do you find a great variety of fresh fruits, vegetables and vegetables for sale? (a) No, (b) Yes, (c) Do not know’; (ii) ‘Are the fresh fruits and vegetables in your neighbourhood of high quality? (a) No, (b) Yes, (c) Do not know’; and (iii) ‘Are the fresh fruits and vegetables in your neighbourhood on sale for an affordable price? (a) No, (b) Yes, (c) Do not know’. These questions were cross-culturally adapted from a prior work (the Multi-Ethnic Study of Atherosclerosis), with a moderatereliability(Reference Barber, Diez-Roux and Cardoso19, Reference Santos, Griep and Cardoso37). Perception of the food environment was used as a binary variable (low food environment perception: yes/no). Low perceived food environments were considered when the answers to all three questions were ‘no’.
Statistical analysis
A descriptive analysis of quantitative variables was performed using means and 95 % CI. Frequency distributions were calculated for categorical variables. Comparison of 95 % CI was used to identify any differences between selected sample characteristics by categories of economic residential segregation (high, medium and low).
To examine the association between segregation and prevalence of healthy and unhealthy food eating markers, we estimated the OR and its respective 95 % CI with binary logistic regression using generalized estimating equations, separately for each outcome. Generalized estimating equations and an exchangeable correlation structure were used because the observations were grouped in one specific structure (i.e. individuals were nested within census tracts)(Reference Hanley, Negassa and Edwardes38).
In our analyses, we adjusted for covariates associated with economic residential segregation and food consumption as potential confounders or mediators. Our first multivariate regression model (Model 1) was adjusted for demographic status (age, sex and race/skin colour). Model 2 was further adjusted for schooling level and family income. Model 3 was adjusted by self-rated health status and nutritional status (BMI, kg/m2), and Model 4 was further adjusted for perceived neighbourhood food environment.
All analyses were performed using the statistical software package Stata version 13 and accounted for the sampling complex design and unequal probabilities of selection. The manipulation of the geographic data was carried out using ArcMap version 10.3 (http://www.esri.com/software/arcgis/index.html). We used a significance level of 5 %.
The study was approved by the Research Ethics Committee of the Medical School, Universidade Federal de Minas Gerais (640.920 of 8 May 2014). All interviewees were informed about the objectives of the research and signed the informed consent form agreeing to participate in the study.
Results
Residential segregation in Belo Horizonte, a Brazilian city
We analysed 3833 census tracts in Belo Horizonte. Segregation scores ranged from −6·64 to 3·92, and approximately 18·9 % of the total sample were in high (Z-score ≥ 1·96 and P ≤ 0·05), 41·0 % in medium and 40·0 % in low areas of segregation (Fig. 1).
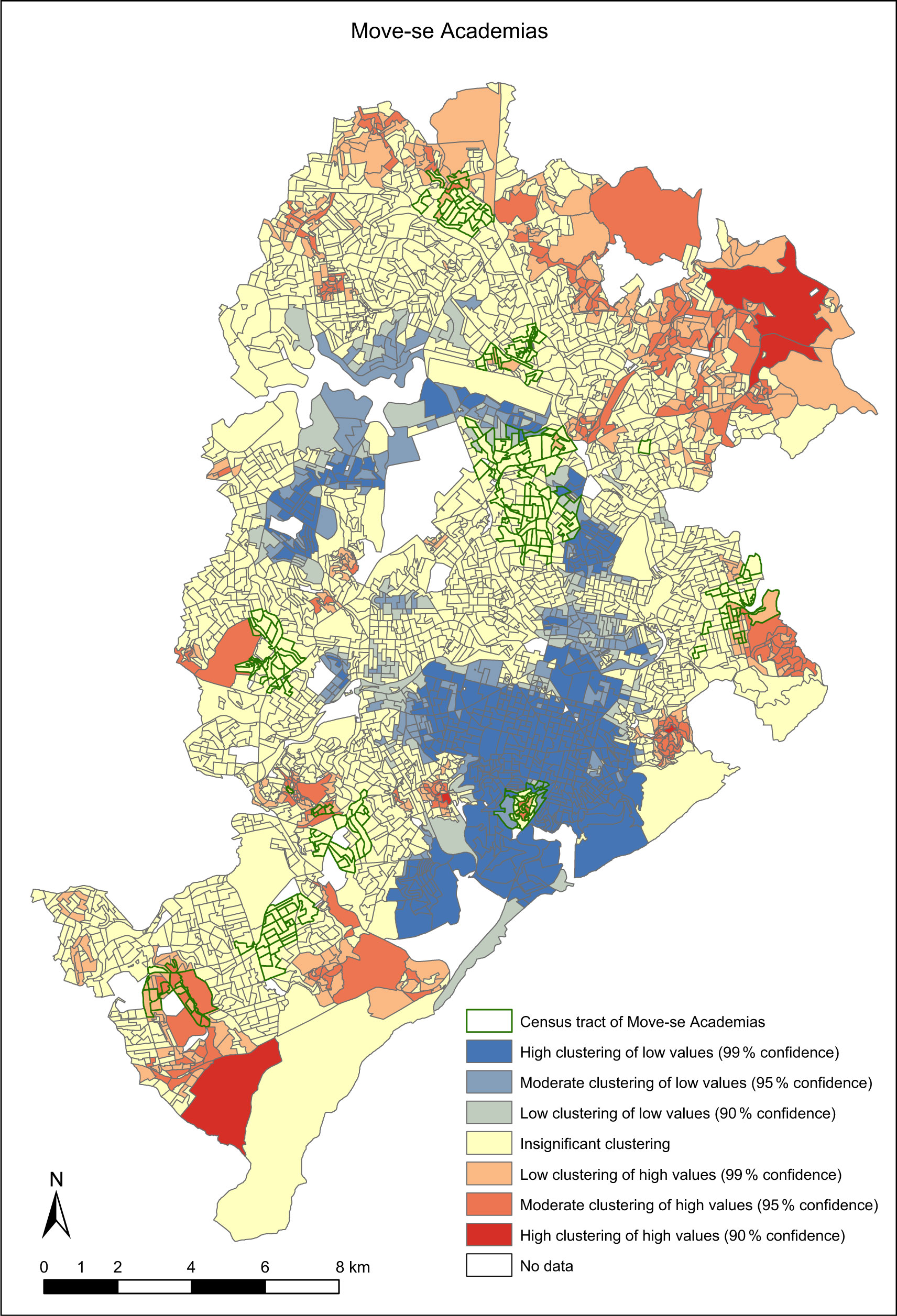
Fig. 1 Results of Getis–Ord local $G_i^{\rm{\ast}}$ statistical analysis based on the proportion of households with 0–3 minimum wages; MOVE-se Academias study, Belo Horizonte, Brazil, 2014–2015
Sample characteristics
We analysed 178 census tracts in Move-se. Segregation scores ranged from −3·25 to 3·03, and approximately 18·5 % of them were in high (Z-score ≥ 1·96 and P ≤ 0·05), 61.2 % in medium and 20.3 % in low segregation areas (Fig. 1). Of the 1301 participants of Move-se, 60·7 % were women. The mean age of the participants was 43·2 (95 % CI 41·8, 44·7) years and the mean segregation score and length of residence were 1·0 (95 % CI 0·9, 1·11) and 18·4 (95 % CI 17·3, 19·6) years, respectively (Table 1).
Table 1 Sample characteristics overall and by categories of economic residential segregation; MOVE-se Academias study, Belo Horizonte, Brazil, 2014–2015

Residential segregation was measured for study-defined neighbourhoods using the Getis–Ord local $G_i^{\rm{\ast}}$ statistic. Categories of segregation were defined as follows: high,
$G_i^ * \ge 1\cdot196$; medium,
$0{\rm{\gt }}G_i^ * {\rm{\lt }}1\cdot196$; and low,
$G_i^{\rm{\ast}} \lt 0$. Low perceived food environments were considered when the answers to all questions about fruit and vegetable availability (variety, quality and price) were ‘no’. In order to identify any differences, the comparison of 95 % CI was used: ahigh-segregated neighbourhood; bmedium-segregated neighbourhood (integrated); clow-segregated neighbourhood. Values within a row with unlike superscript letters were statistically different.
* Data includes twenty-three missing values.
† Data includes thirty-two missing values.
‡ Data includes ten missing values.
§ Data includes three missing values.
‖ Data includes twelve missing values.
Individuals residing in a highly segregated neighbourhood were less educated and tended to be younger. They were also more likely to self-rate their health status as poor/very poor. Furthermore, perceptions of the food environment were worse (low food environment perception) in these high-segregated areas in comparison to low-segregated, 27·5 (95 % CI 20·0, 36·7) % v. 8·8 (95 % CI 5·0, 14·9) %. No differences in BMI, race/skin colour categories, practise of physical activities and length of residence were observed across segregation categories (Table 1).
As presented in Table 2, the distribution of healthy and unhealthy eating markers was influenced by the economic residential segregation.
Table 2 Prevalence (95 % CI) of healthy and unhealthy eating markers among adults (≥18 years) by categories of economic residential segregation; MOVE-se Academias study, Belo Horizonte, Brazil, 2014–2015

Residential segregation was measured for study-defined neighbourhoods using the Getis–Ord local $G_i^{\rm{\ast}}$ statistic. Categories of segregation were defined as follows: high,
$G_i^ * \ge 1\cdot196$; medium,
$0{\rm{\gt }}G_i^ * {\rm{\lt }}1\cdot196$; and low,
$G_i^{\rm{\ast}} \lt 0$. Regular consumption defined as a consumption of any amount for five days or more per week, except for fish (one or more days per week) and replacement of lunch or dinner with snacks (seven days per week). In order to identify any differences, the comparison of 95 % CI was used: ahigh-segregated neighbourhood; bmedium-segregated neighbourhood (integrated); clow-segregated neighbourhood. Values within a row with unlike superscript letters were statistically different.
Overall, the regular consumption of fruits was reported by 36·7 (95 % CI 33·2, 40·3) % of participants, being lower among individuals residing in highly segregated areas (34·6 %; 95 % CI 25·5, 44·9 %). Likewise, regular consumption of vegetables was lower in high-segregated neighbourhoods compared with low-segregated neighbourhoods (70·1 %; 95 % CI 62·6, 76·7 % v. 87·6 %; 95 % CI 81·7, 91·7 %).
Similarly, the regular consumption of fish, reported to happen at least once per week, was lower among individuals residing in highly segregated areas (23·6 %; 95 % CI 17·2, 31·3 %). On the other hand, the regular consumption of beans was reported by 83·1 % (95 % CI 79·2, 86·5 %) of participants, being higher among individuals residing in highly segregated areas (91·0 %; 95 % CI 86·7, 93·9 %).
We verified that the regular replacement of lunch or dinner with snacks was significantly lower in highly segregated areas. The regular consumption of meat with excess fat was significantly higher among medium-segregation in comparison to low-segregation areas. There were no statistically significant differences comparing consumption of soft drinks, artificial juice and sweet foods among the segregation categories.
Multiple logistic regression results
In multiple logistic regression models using the generalized estimating equations (Tables 3 and 4), we found higher odds of regular consumption of beans (OR = 1·85; 95 % CI 1·07, 3·19) among those living in high-segregated compared with low-segregated neighbourhoods, after adjustment for individual-level factors and perceived food environment. By contrast, we found lower odds of regular consumption of vegetables (OR = 0·62; 95 % CI 0·39, 0·98).
Table 3 Adjusted OR and 95 % CI for healthy eating markers prevalence in high and medium categories of economic residential segregation; MOVE-se Academias study, Belo Horizonte, Brazil, 2014–2015

Ref., reference category.
Logistic regression models using the generalized estimating equations method were used to estimate adjusted OR and 95 % CI. Residential segregation was included in models as a categorical variable with ‘low’ residential segregation as the reference category (high, $G_i^ * \ge 1\cdot196$; medium:
$0{\rm{\gt }}G_i^ * {\rm{\lt }}1\cdot196$; and low,
$G_i^{\rm{\ast}} \lt 0$). Regular consumption defined as a consumption of any amount for five days or more per week, except for fish (one or more days per week). Low perceived food environments were considered when the answers to all questions about fruit and vegetable availability (variety, quality and price) were ‘no’.
Model 1: adjusted for age, gender and skin colour.
Model 2: Model 1 + family income and education.
Model 3: Model 2 + self-rated health and BMI (kg/m2).
Model 4: Model 3 + low perceived food environment.
Bold denotes statistical significance, P < 0·05.
Table 4 Adjusted OR and 95 % CI for unhealthy eating markers prevalence in high and medium categories of economic residential segregation; MOVE-se Academias study, Belo Horizonte, Brazil, 2014–2015

Ref., reference category.
Logistic regression models using the generalized estimating equations method were used to estimate adjusted OR and 95 % CI. Residential segregation was included in models as a categorical variable with ‘low’ residential segregation as the reference category (high, $G_i^ * \ge 1\cdot196$; medium,
$0{\rm{\gt }}G_i^ * {\rm{\lt }}1\cdot196$; and low,
$G_i^{\rm{\ast}} \lt 0$). Regular consumption defined as a consumption of any amount for five days or more per week, except for replacement of lunch or dinner with snacks (seven days per week). Low perceived food environments were considered when the answers to all questions about fruit and vegetable availability (variety, quality and price) were ‘no’.
Model 1: adjusted for age, gender and skin colour.
Model 2: Model 1 + family income and education.
Model 3: Model 2 + self-rated health and BMI (kg/m2).
Model 4: Model 3 + low perceived food environment.
Bold denotes statistical significance, P < 0·05.
Although high segregation was not associated with regular consumption of fruits, fish, or consumption of meat or chicken with excess fat, we found differences between medium-segregation and low-segregation areas after adjustment. We found lower odds of regular consumption of fish (OR = 0·65; 95 % CI 0·45, 0·94) in medium-segregated neighbourhoods compared with low-segregated neighbourhoods, after adjustments. In contrast, we observed high odds of regular consumption of meat or chicken with excess fat (OR = 1·46; 95 % CI 1·04, 2·06) in medium-segregation areas in comparison to more affluent neighbourhoods.
Discussion
Belo Horizonte seems to be an urban divided space, in which ‘invisible’ barriers limit equitable occupation of the city and probably contribute to either healthy or unhealthy food consumption of the people living in high-segregated neighbourhoods.
Economically segregated urban neighbourhoods
The city of Belo Horizonte was planned, built and founded to be a symbol of modernity; however, it has experienced an urban planning that probably made the socio-spatial segregation real.
Segregation apparently divides Belo Horizonte. Highly segregated neighbourhoods correspond to one of the most vulnerable areas of the city. On the other hand, low-segregated neighbourhoods are located predominantly in the south-central areas, one of the wealthier regions. The south-central area has the highest income per capita (R$ 3915·00) and Municipal Human Development Index (0·910) of the city. The per capita income in Belo Horizonte, in the same year, was R$ 1497·29 and the Municipal Human Development Index was 0·810(39).
Disparities in food consumption
The high economic residential segregation was independently associated with higher prevalence of regular consumption of beans and a lower prevalence of regular consumption of vegetables, after adjustments for individual-level characteristics and perceived food environment.
Eating behaviour of individuals may be influenced by existing norms and values around them and this could be correlated to access to, availability and price of these foods, which, in turn, can also modulate residents’ eating behaviour or shape their food preferences(Reference Menezes, Diez Roux and Costa40–Reference Story, Kaphingst and Robinson-O’Brien44).
Associations of segregation and diet were neither consistent nor significant. Research conducted in the USA described that neighbourhood-level racial/ethnic segregation and components of a healthy diet were not independently associated after adjustments(Reference Yi, Ruff and Jung17). However, more recent findings showed racial/ethnic segregation has a negative effect on fruit and vegetable expenditure and consumption(Reference Ryabov16, Reference Corral, Landrine and Hao18). Furthermore, regarding the availability of healthy foods, some prior research denotes a double risk in segregated areas, with an average of 30 % fewer supermarkets and more fast-food restaurants, convenience retails and other smaller stores offering an abundance of high-energy and low-nutrient foods(Reference Bower, Thorpe and Rohde9, Reference Walker, Keane and Burke45). In addition, racial/ethnically segregated neighbourhoods had less availability of fresh fruit and less promotion of unhealthy impulse buys relative to stores in more affluent areas(Reference Winkler, Lenk and Caspi46).
The high prevalence of beans consumption in the high-segregated neighbourhoods indicated that segregation may not just be a negative phenomenon. Beans are a significant component of the traditional Brazilian diet. They are considered to be a healthy food and are among the most consumed items in the country(Reference Jaime, Stopa and Oliveira20, Reference Souza, Pereira and Yokoo47). Regular consumption of beans can be associated not only with price, high satiation/satiety capacity of this food or a concrete way to survive starvation, but also with tradition, culture and preservation of cooking habits. Segregation is a relational phenomenon involving development and sustainability of culture and identity(Reference Williams and Collins2, Reference Torres26). Thus, consumption of beans in such neighbourhoods may reveal how preservation of some aspects of food culture can differently affect social groups.
Regarding vegetable consumption, it has been well documented that racial/ethnic segregation and socio-economic disadvantage affect the quantity and quality of fresh food stores(Reference Duran, Roux and Latorre13, Reference Ryabov16). An ecological study undertaken in Belo Horizonte revealed that the neighbourhood of a HAP had low quality of stores with limited access to healthy foods(Reference Costa, Oliveira and Lopes48). Besides, previous findings in Belo Horizonte and São Paulo showed that living in neighbourhoods with stores/markets selling fresh foods was associated with fruit and vegetable consumption(Reference Duran, Roux and Latorre13, Reference Menezes, Diez Roux and Costa40).
Differently from the results found for regular consumption of vegetables, the prevalence of regular consumption of fruits did not differ between high-segregated neighbourhoods, after adjustments. Like vegetables, fruits are fresh foods that are usually sold in the same section of the stores, but access to them can be different as demonstrated in a Brazilian study that audited 336 commercial establishments in Belo Horizonte. Auditing in 1600 m buffer zones of eighteen HAP sites (2013–2014), the authors reported that the quality of fruits was better and their availability was higher, compared with vegetables(Reference Menezes, Costa and Oliveira49). Thus, it is important to understand other variables that can be associated with segregation, including characteristics of consumers’ eating environment and monotonous consumption of fruits among the areas studied. It is possible that residents of high-segregated neighbourhoods consume a smaller variety of fruits compared with the more affluent ones.
Differences between medium-segregation and low-segregation neighbourhoods were observed; however, caution is needed when drawing generalities, since the low-segregated category corresponds to integrated areas (no segregated) or areas in which the group of heads of household earning a monthly income of 0–3 minimum wages is significantly under-represented (low-segregated areas). We may need to evaluate availability of food, other variables representing the community and the consumer nutrition environment in these areas to understand their differences and how the association between segregation and dietary behaviours is mediated by the objective food environment.
Strengths and limitations
These findings may be influenced by some limitations, as follows. First, the design of the Move-se Academias study includes units of a health promotion programme located predominantly in vulnerable areas of the city. Thus, the proportion of heads of household earning a monthly income greater than three minimum wages in these areas was very small. As a result, the low-segregation group corresponds to the absence of any clustering or areas in which the group of heads of household earning 0–3 minimum wages is significantly under-represented. Nevertheless, with the sample of the present study, we were able to reproduce approximately 18 % of high segregation evidenced in the larger area studied. Furthermore, we can speculate that there is a possible influence of HAP and their distinct periods of existence on the eating practices of the study participants. However, we cannot determine the influence of the programme’s existence on eating practices of non-users because there is insufficient evidence in the literature. Second, possible measurement biases exist, as expected from the usual dietary risk factor information related to the interviewee’s memory failure. Third, the census tracts might not correspond to socially defined neighbourhoods. However, there are different approaches to define a geographic context; several studies have used census tracts to define neighbourhood boundaries and associated them with health outcomes(Reference Almeida, Friche and Jennings50, Reference Arcaya, Tucker-Seeley and Kim51).
Despite this, the present study has many strengths. To our knowledge, there is no study in the literature assessing the relationship of food consumption with economic residential segregation in Brazil. There are several consistent and significant studies examining the disparities in availability of fresh fruits, vegetables, low-fat milk and high-fibre foods among segregated urban neighbourhoods(Reference Ball, Timperio and Crawford8, Reference Morland and Filomena10). But this is the first research conducted in Brazil aiming to describe food consumption using the $G_i^{\rm{\ast}}$ statistic, a novel spatial approach to measure segregation. In addition, the present study uses an urban population sample from a large Brazilian city and 2010 Brazil Census data to understand the relationship between segregation territories and food consumption.
Conclusions
The present study provides empirical evidence that segregation is an important determinant of diet in low-medium-income countries, confirms the different profiles of food consumption in segregated areas and contributes to a growing body of research seeking to understand how segregation is associated with health and diet.
Our results suggest living in a high-segregation neighbourhood was associated with healthy food consumption in two different ways: (i) negatively, as shown by the lowest regular consumption of fresh foods; and (ii) positively, favouring the consumption of minimally processed foods, preserving traditions and culture. A better understanding of residential segregation in Brazil could potentially inform policies and/or programming by reflecting the unequal distribution of deprivation and privilege, especially at a time when progress in reducing inequality is under threat.
Segregation is a symptom of the structures of governance, work and iniquities, and Brazil is at a challenging moment. Our social welfare net, that until recently has been supporting innumerable achievements in the food and nutrition agenda (including reducing hunger and poverty, promoting food and nutrition security in the country), as well as the different assistance actions (by securing the rights and protection of vulnerable populations) are weakening. The establishment of spending cuts in education and health, associated with revisions to labour and pension laws, probably will impact the population’s income and intensify social inequalities(Reference Vasconcelos, Machado and Medeiros52–Reference Paiva, Mesquita and Jaccoud54), consequently deepening the residential segregation and its negative repercussions on the health of Brazilians.
Furthermore, considering different access to food in this area, a better understanding of neighbourhood characteristics and food environment aspects could clarify the links among eating behaviours, socio-economic disadvantages and segregation.
Acknowledgements
Financial support: This work was supported by the Conselho Nacional de Desenvolvimento Científico e Tecnológico (CNPq; grant number 552752/2011-8 and Research Productivity Scholarships to A.A.L.F., D.C.M. and W.T.C.) and the Coordenação de Aperfeiçoamento de Pessoal de Nível Superior – Brasil (CAPES; Finance Code 001). CNPq and CAPES had no role in the design, analysis or writing of this article. Conflict of interest: None. Authorship: Authors contributed as follows. M.S.L.: analysis and interpretation of data; statistical analysis; drafting of manuscript; reviewed the final version of this manuscript and approved its publication. W.T.C.: conception and design; critical revision of manuscript; obtaining funding; administrative, technical and material support; reviewed the final version of this manuscript and approved its publication. A.C.S.A.: analysis and interpretation of data; statistical analysis; reviewed the final version of this manuscript and approved its publication. D.C.M.: conception and design; critical revision of manuscript; obtaining funding; administrative, technical and material support; reviewed the final version of this manuscript and approved its publication. S.B.: critical revision of manuscript; technical support; reviewed the final version of this manuscript and approved its publication. A.A.L.F.: conception and design; critical revision of manuscript; obtaining funding; administrative, technical and material support; reviewed the final version of this manuscript and approved its publication. Ethics of human subject participation: This study was conducted according to the guidelines laid down in the Declaration of Helsinki and all procedures involving research study participants were approved by the Research Ethics Committee of the Medical School, Universidade Federal de Minas Gerais (640.920 of 8 May 2014). Written informed consent was obtained from all subjects.