No CrossRef data available.
Article contents
Exploring ChatGPT's potential as an AI-powered writing assistant: A comparative analysis of second language learner essays
Published online by Cambridge University Press: 14 February 2025
Abstract
An abstract is not available for this content so a preview has been provided. Please use the Get access link above for information on how to access this content.
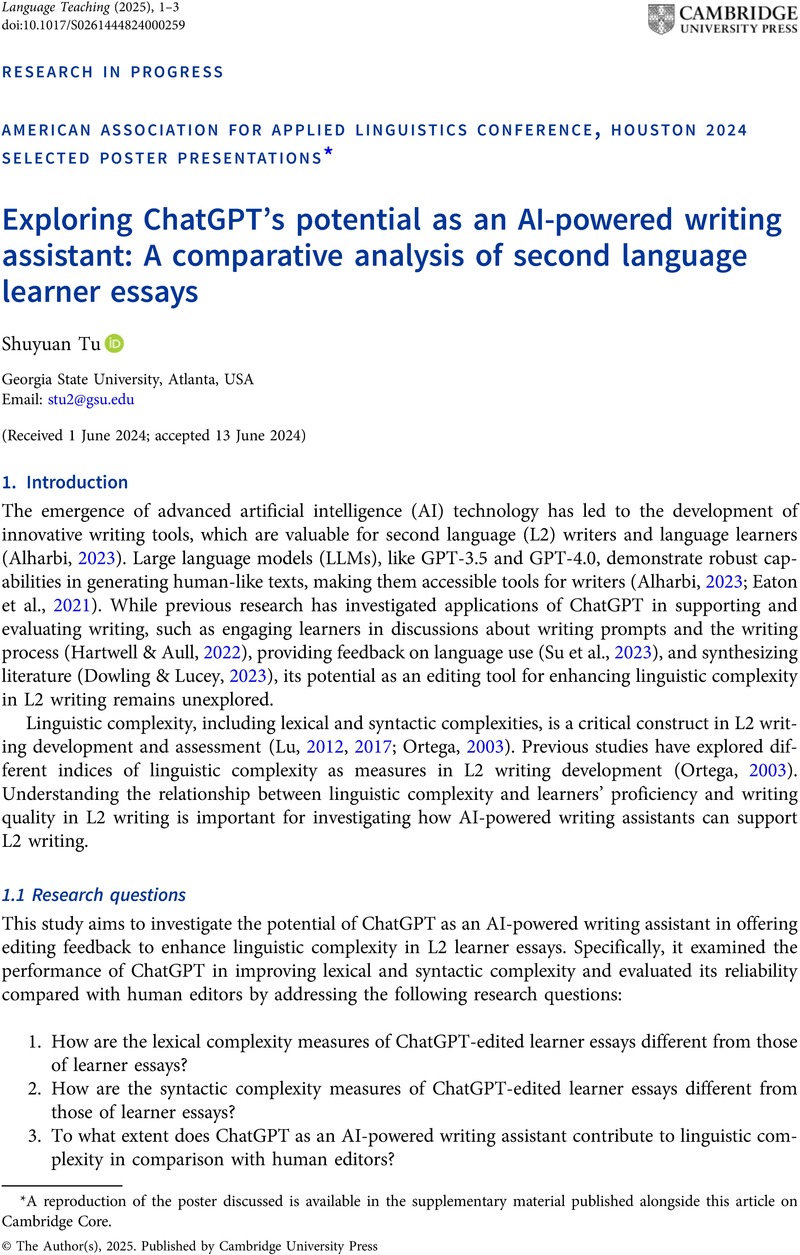
- Type
- Research in Progress
- Information
- Copyright
- Copyright © The Author(s), 2025. Published by Cambridge University Press
Footnotes
*
A reproduction of the poster discussed is available in the supplementary material published alongside this article on Cambridge Core.
References
Ai, H., & Lu, X. (2010). A web-based system for automatic measurement of lexical complexity. In 27th Annual Symposium of the Computer-Assisted Language Consortium (CALICO-10). Amherst, MA. June (pp. 8–12).Google Scholar
Ai, H., & Lu, X. (2013). A corpus-based comparison of syntactic complexity in NNS and NS university students’ writing. In Díaz-Negrillo, A., Ballier, N., & Thompson, P. (Eds.), Automatic treatment and analysis of learner corpus data (pp. 249–264). Amsterdam, the Netherlands.CrossRefGoogle Scholar
Alharbi, W. (2023). AI in the foreign language classroom: A pedagogical overview of automated writing assistance tools. Education Research International, 2023(1), 1–15. https://doi.org/10.1155/2023/4253331.CrossRefGoogle Scholar
Dowling, M., & Lucey, B. (2023). ChatGPT for (finance) research: The Bananarama conjecture. Finance Research Letters, 53, 103662. http://doi.org/10.1016/j.frl.2023.103662.CrossRefGoogle Scholar
Eaton, S. E., Mindzak, M., & Morrison, R. (2021). Artificial intelligence, algorithmic writing & educational ethics [Paper Presentation]. Canadian Society for the Study of Education Société canadienne pour l'étude de l'éducation, Edmonton, AB, Canada.Google Scholar
Hartwell, K., & Aull, L. (2022). Constructs of argumentative writing in assessment tools. Assessing Writing, 54. https://doi.org/10.1016/j.asw.2022.100675.CrossRefGoogle Scholar
Ishikawa, S. (2018). The ICNALE edited essays; A dataset for analysis of L2 English learner essays based on a new integrative viewpoint. English Corpus Studies, 25, 117–130.Google Scholar
Jacobs, H. L. (1981). Testing ESL composition: A practical approach. English composition program. Newbury House Publishers, Inc.Google Scholar
Lu, X. (2012). The relationship of lexical richness to the quality of ESL learners’ oral narratives. Modern Language Journal, 96(2), 190–208. https://doi.org/10.1111/j.1540-4781.2011.01232_1.x.CrossRefGoogle Scholar
Lu, X. (2017). Automated measurement of syntactic complexity in corpus-based L2 writing research and implications for writing assessment. Language Testing, 34(4), 493–511. https://doi.org/10.1177/0265532217710675.CrossRefGoogle Scholar
Ortega, L. (2003). Syntactic complexity measures and their relationship to L2 proficiency: A research synthesis of college-level L2 writing. Applied Linguistics, 24(4), 492–518. https://doi.org/10.1093/applin/24.4.492.CrossRefGoogle Scholar
Su, Y., Lin, Y., & Lai, C. (2023). Collaborating with ChatGPT in argumentative writing classrooms. Assessing Writing, 57, 100752. https://doi.org/10.1016/j.asw.2023.100752.CrossRefGoogle Scholar