Introduction
As the leading cause of death in the United States, cardiovascular disease (CVD) is expected to affect 44.1% of the population by 2035.Reference Khavjou, Phelps and Leib1 CVD has an extremely complex, multifactorial etiology, where neither genetic nor environmental influences act alone to contribute to disease onset.Reference Knopik, Maccani and Francazio2,Reference Sing, Stengard and Kardia3 There is growing body of literature linking maternal cardiometabolic health during pregnancy to adverse cardiovascular health outcomes in offspring later in life.Reference Christensen, Retterstol and Godang4,Reference Alsnes, Fraser and et al5 These developmental origins of long-term cardiovascular health outcomes are thought to be the consequence of changes to placental physiology.
The state of a mother’s cardiometabolic health during gestation and postpartum is related to the health and functionality of the placenta. As a central vascular organ overseeing fetal growth, development and the intrauterine environment, proper functionality of the placental remains central to successful gestational outcomes. Resting at the interface of the maternal and fetal environment, the placenta participates in a variety of molecular processes, such as nutrient transport, immunomodulation, and endocrine signaling, all of which are made possible through the development of a villi-based vasculature system which allows for communication between mother and offspring.Reference Cross, Baczyk and Dobric6 Maternal cardiovascular and metabolic conditions during pregnancy, including type II diabetes, hypertension and hypercholesterolemia have been implicated in dysfunction of placental micro and macro-vasculature. In addition, pathophysiologic characteristics of placental insufficiency, similar to those occurring in preeclampsia, manifests in cardiovascular effects including hypertension and kidney malfunction.Reference Leiva, de Medina and Salsoso7,Reference Sobrevia, Abarzua and Nien8 Additionally, such placental insufficiencies are associated with adverse gestational outcomes, such as fetal growth restriction, which itself serves as a significant risk indicator for the development of CVD later in life.Reference Audette and Kingdom9-Reference D.J.Barker, Margetts, Osmond and Simmonds11 Physiological and functional abnormalities of the placenta also serve as an indicator of maternal cardiometabolic health, as well as newborn health outcomes that potentially manifest as risk of chronic disease.Reference Ostling, Kruse and Helenius12-Reference Higashijima, Miura and Mishima17 Considering placental development occurs concurrently with fetal heart development, and each organ utilizes common humoral growth signals, such as transforming growth factor-beta (TGFβ) and vascular endothelial growth factor (VEGF), deficiencies in the organogenesis of either organ may alter the formation of the other, initiating physiological changes with potential lifelong cardiovascular consequences.Reference Cohen, Rychik and Savla18-Reference Azhar, Runyan and Gard20 While the associations between maternal cardiometabolic risk factors, placental insufficiency and offspring lifelong health outcomes are well defined,Reference Leiva, de Medina and Salsoso7,Reference Sobrevia, Abarzua and Nien8,Reference Odegard R.A., Nilsen, Salvesen and Austgulen21 the molecular mechanisms by which this developmental programming is established have yet to be robustly delineated. Additionally, these overt placental pathologies are relatively rare, and cannot account for the prevalence of CVD and deficiencies in maternal health, postpartum.
MicroRNAs (miRNA) are small noncoding RNA molecules (∼22 nucleotides) capable of post-transcriptional regulation of gene expression. These molecules utilize base-pairing to bind to the 3’-untranslated region of target mRNAs resulting in either translational repression or mRNA degradation, by which the exact mechanism largely depends on the degree of sequence complementarity between the miRNA and target mRNA. Dysregulation of placental miRNA have previously been implicated in both preeclampsia and fetal growth restriction, suggesting their potential role as modifiers of newborn and maternal health outcomes in response to various genetic and/or environmental conditions.Reference Ostling, Kruse and Helenius12-Reference Higashijima, Miura and Mishima17 However, many of these studies were conducted on small sample sizes (n < 100), and largely focused on newborn outcomes as they associate with placental miRNA expression as opposed to the relationship between gestational characteristics and expression of placental miRNA.Reference Ostling, Kruse and Helenius12-Reference Wang, Na, Song and Song15,Reference Higashijima, Miura and Mishima17
To explore the influence of maternal familial CVD on the placental miRNA landscape, we utilized placental miRNA sequencing data from the Rhode Island Child Health Study (RICHS; n = 230) and examined the relationship between maternal family history of CVD and placental miRNA expression. Through this clinical history variable we capture maternally inherited genetic risk of CVD, as well as shared familial behaviors and environmental risk factors that may associate with CVD, such as diet and exercise, that contribute to the overall health of the gestational environment.Reference Sing, Stengard and Kardia3,Reference Christakis and Fowler22 Bioinformatic target prediction was then used to identify potential mRNA targets of miRNAs significantly associated with maternal CVD risk, followed by overrepresentation analyses to characterize the biological pathways in which these mRNAs participate.
Methods
Cohort
The RICHS is a cohort of mother-infant pairs from the Women & Infants Hospital in Providence, Rhode Island, enrolled between September 2010 and February 2013. All mothers were at least 18 years of age, had no life-threatening conditions, and delivered singletons free of congenital/chromosomal abnormalities at or after 37 weeks of gestation. All participants provided written informed consent and all protocols were approved by the IRBs at the Women & Infants Hospital of Rhode Island and Emory University, respectively. Data provided by this study include placental microRNA transcript abundance (n = 230). Interviewer-administered questionnaires were utilized to collect sociodemographic and lifestyle data. Structured medical record review was used to collect anthropometric and medical history data. Maternal family history of CVD was reported and coded as a binary variable (yes/no). Follow-up questions regarding which primary relative had been afflicted, coded as “mother,” "father,” "brother,” and “sister.” Instances where the mother reported a family history of CVD but did not identify a specific primary relative were not included in the study in order to prevent improper classification of familial CVD incidence.
Tissue collection
Fetal placental samples from all subjects were collected as previously described.Reference Lesseur, Armstrong, Paquette, Koestler, Padbury and Marsit23 Briefly, placental samples were collected within 2 h of birth; fragments were obtained two centimeters (cm) from the umbilical cord and free of maternal decidua. Collected tissue was immediately placed in RNA later solution (Life Technologies, Grand Island, NY, USA) and stored at 4 °C for at least 72 h. Subsequently, tissue segments were blotted dry, snap frozen in liquid nitrogen, homogenized by pulverization using a stainless steel cup and piston unit (Cellcrusher, Cork, Ireland) and stored at −80 °C.
miRNA isolation and sequencing
Total RNA was extracted from placenta using the Qiagen miRNeasy Mini Kit and a TissueLyser LT (Qiagen, Germantown, MD, USA) following manufacturer’s protocol. Briefly, 25–-35 mg of frozen, powdered placental tissue was placed in a 2 ml round bottom tube with 700 ul of Qiazol Lysing Reagent and one 5 mm stainless steel bead. The tissue was homogenized in a pre-chilled tube holder on the TissueLyser LT for two, 5-min cycles at 30 Hz. The resulting homogenate was processed with the Qiagen miRNeasy Mini Kit with on-column DNAse digestion and eluted in 50 µl RNase-free water. The RNA was quantitated on a NanoDrop (Thermo Fisher, Waltham, MA, USA) and quality checked on Agilent Bioanalyzer using the Agilent RNA 6000 nano kit (Agilent, Santa Clara, CA, USA). Single end, 1 × 50 bp next generation sequencing of placental miRNA was performed by Omega Bioservices (Norcross, Georgia) as previously described.Reference Kennedy, Hermetz and Burt24
miRNA seq processing and QC
Raw FASTQ reads obtained from a total of 230 RICHS samples were subject to adaptor trimming with cutadapt v1.1634. The 3’ adaptor sequence were trimmed (TGGAATTCTCGGGTGCCAAGG) and then four bases were trimmed from each end of the read following vendor’s recommendation (BIOO Scientific, Austin, TX). We then used trimmed reads and miRDeep2 to quantify microRNA.Reference Friedländer, Mackowiak, Li, Chen and Rajewsky25 miRDeep2 was used to first perform alignment using bowtie1 with human genome hg38.Reference Langmead, Trapnell, Pop and Salzberg26 The ‘Quantifier’ module in miRDeep2 was used to obtain raw counts of microRNAs with miRbase version 22.Reference Griffiths-Jones, Saini and van Dongen27
Transcript filtering and normalization
Raw miRNA counts were imported into DESeq2 for normalization and differential expression analysis. Only miRNA transcripts with more than one count per million in at least 10 percent of samples were included, leaving 802 miRNA transcripts to be analyzed of the initial 2656 sequenced transcripts. Dispersion estimates were then calculated, followed by generation of median ratio size factor estimates to normalize counts for analysis with in DESeq2.Reference Anders and Huber28 Normalized counts were then exported from DESeq2 for Surrogate Variable Analysis (SVA). The Variance Stabilization Transformation (VST) was applied to count matrices to yield approximately normalized and log2-transformed abundances, which were utilized in correlation analyses.Reference Kennedy, Hermetz and Burt24
Statistical Analyses
SVA
In an effort to adjust for unknown confounders, such as cell-type heterogeneity and unmeasured sources of technical variation, surrogate variables were estimated for normalized miRNA transcript reads using the sva package.Reference Leek29,Reference Love, Huber and Anders30 The full model used in the svaseq includes variation attributable to family history of CVD while the null model included only an intercept term. One surrogate variable was utilized as a covariate in our differential expression analysis.
DESeq2 differential expression analysis
miRNA transcript counts were modeled using a negative binomial generalized linear model to identify differentially expressed transcripts in DESeq2.Reference Love, Huber and Anders30 For each of the 802 individual miRNA transcripts which passed strict filtering and quality criteria, the variance stabilized transformed transcript abundance was regressed on the history of familial CVD (No = 0, Yes = 1), while adjusting for the first surrogate variable to control for unknown confounders.Reference Leek29,Reference Love, Huber and Anders30 We considered miRNAs with a false discovery rate (FDR) less than 10% to be considered a differentially expressed miRNA (DemiR) with respect to family history of CVD. Extensive sensitivity analyses were performed by including various parameters as covariates to the original model to assess the robustness DemiR effect sizes (Supplementary Fig. S1).
Target prediction and filtering
Potential DemiR targets were identified using miRDIP, an online database of miRNA target predictions.Reference Tokar, Pastrello and Rossos31 Only targets within the top 1% of confidence scores were returned. However, given that miRNA do not require perfect complementarity with a target mRNA and can only target mRNAs expressed in the examined tissue, bioinformatic target prediction is prone to generating false positives. To enhance our dataset with true miRNA:mRNA relationships we utilized pre-existing, parallel total RNA sequencing data in RICHS.Reference Kennedy, Hermetz and Burt24 We then calculated Pearson correlation coefficients for each miRNA:mRNA pair utilizing normalized miRNA and mRNA sequencing counts from RICHS.Reference Kennedy, Hermetz and Burt24 Only miRNA:mRNA target pairs with negative correlation coefficients (q-value < 0.05) were returned to be used in network and pathway analyses. Correlation coefficients ranged between –0.41 and –0.21.
Pathway analysis
Predicted DemiR targets were tested for pathway overrepresentation within ConsensusPathDB (CPDB),Reference Kamburov, Pentchev, Galicka, Wierling, Lehrach and Herwig32,Reference Kamburov, Wierling, Lehrach and Herwig33 against all genes that passed general QC filtering in RICHS whole transcriptome RNA-seq analysis.Reference Kennedy, Hermetz and Burt24,Reference Kamburov, Pentchev, Galicka, Wierling, Lehrach and Herwig32,Reference Kamburov, Wierling, Lehrach and Herwig33 CPDB utilizes 12 separate biological pathway databases, and calculates an enrichment p-value from the hypergeometric distribution of genes in the list of miRNA targets and the pathway gene set. Only mRNAs that were expressed > 1 cpm in at least 10% of the RICHS samples were included as the background for the pathway analysis.Reference Kennedy, Hermetz and Burt24 FDRs were calculated from the enrichment p-values, and a q-value less than 0.05 was considered a significant enrichment of miRNA targets in the tested pathway.
Results
This study analyzed miRNA sequencing data from 230 placentae from the RICHS. The demographics of the participants are displayed in Table 1. In general, placentae collected in this study were from full term pregnancies (≥37 weeks), all from relatively healthy mothers who did not experience serious pregnancy complications. Forty-nine percent (n = 113 of 230) of maternal participants reported a family history of CVD, and forty-nine percent (n = 112 of 230) were either overweight or obese, n = 57 of those also reporting a family history of CVD.
Table 1. Demographic characteristics of RICHS participants included in the miRNA sequencing analysis (n = 230)

To analyze the association between maternal family history of CVD and placental miRNA expression, we performed differential expression analysis using negative binomial generalized linear models constructed in DESeq2 on placental small RNA sequencing data. We identified nine Differentially Expressed miRNAs (DEmiRs) associated with familial CVD history (FDR < 0.1), four of which (miR-1246, miR-324-5p, miR-1307-3p, and miR-520a-3p) met a strict Bonferroni threshold (p-value < 6.23e-05) (Fig. 1). Sensitivity analyses were performed to adjust for various biological and technical covariates (RNA integrity, flow cell lane, pregnancy smoking, maternal race, infant sex and infant birth weight percentile) in order to characterize the robustness of DEmiR effect sizes, and the DEmiR log2 fold changes were strikingly consistent even with these additional adjustments to the original model (Supplementary Figs S1 and S2).

Fig. 1. Placental miRNA associates with maternal family history of CVD. (a) Volcano plot representing the results of the differential expression analysis. The y-axis shows the-log10(p-values) in the association of each miRNA with family history of CVD. The x-axis displays the effect estimates in units of log2 fold change in each miRNA’s transcript abundance in individuals with familial incidence of CVD. 9 miRNAs are significantly (FDR <0.1) associated with familial CVD history and 4 of those (square shaped) reach are significant after Bonferroni correction (p-value < 6.23e-05). (b) Estimates of log2 fold change of miRNA transcript abundance of miRNAs significantly associated with familial CVD history. Squares represent the effect size estimate, while error bars represent standard error of the effect size estimate.
Bioinformatic targets of all nine DEmiRs were predicted using the miRNA Data Integration Portal (miRDIP).Reference Tokar, Pastrello and Rossos31 miRDIP predicted 4516 targets across all nine DEmiRs. To enhance our dataset with true miRNA:mRNA relationships, existing mRNA sequencing data from RICHS samples were used to calculate Pearson correlation coefficients were calculated between normalized miRNA and mRNA sequencing counts. Only miRNA:mRNA pairs where the transcript abundance of a DEmiR and its miRDIP predicted target were negatively correlated (p-value < 0.05) were considered in downstream pathway analyses. This correlation-based filtering revealed 7 DEmiRs and 66 predicted mRNA targets (Supplementary Table S1).Reference Kennedy, Hermetz and Burt24
The 66 predicted DEmiR targets were tested for pathway overrepresentation within ConsensusPathDB (CPDB).Reference Kennedy, Hermetz and Burt24,Reference Kamburov, Pentchev, Galicka, Wierling, Lehrach and Herwig32,Reference Kamburov, Wierling, Lehrach and Herwig33 Pathways related to TGFβ signaling, cellular metabolism, and immunomodulation were overrepresented among DEmiR targets (q-value < 0.05). These enrichment results are largely driven by targets of miR-574-5p, miR-324-5p, miR-326 and miR-520a-3p (Supplementary Table S1).
Discussion
Maternal mortality rates in the United States are higher than any other industrialized country and continue to rise. More than 15% of these deaths are caused by CVD-related health complications, and over half of these deaths have been deemed preventable as they occur postpartum, increasing the opportunity for intervention.Reference Wong and Kitsantas34 Physiological and functional abnormalities of the placenta serve as an indicator of maternal cardiometabolic health, as well as newborn health outcomes that potentially manifest as risk of chronic disease.Reference Ostling, Kruse and Helenius12-Reference Higashijima, Miura and Mishima17 While our study does not investigate placentae from mothers diagnosed with CVD, the chronic nature of the disease provides a unique opportunity to study how the accumulation of genetic and environmental insults, such as those suggested by familial CVD, influences placental functionality and the molecular mechanisms governing adverse gestational outcomes and the developmental programming of chronic disease.
Our small RNA-seq analysis identified fetoplacental miRNAs whose expression associate with maternal family history of CVD (Fig. 1). The expression of microRNAs is known to be sensitive to various gestational environments, including maternal obesity and hypoxia.Reference Olejniczak, Kotowska-Zimmer and Krzyzosiak35,Reference Carreras-Badosa, Bonmatí and Ortega36 Oxidative stress resulting from the potential comorbidities of familial CVD throughout gestation may contribute to the dysregulation of placental miRNAs. There is also a known relationship between genetic variation specific to CVD, and various facets of miRNA biogenesis, activity and function,Reference Hogg and Harries37,Reference Huan, Rong and Tanriverdi38 where genetic risk of disease may ultimately be influencing the expression of placental miRNAs and further disrupting the biological pathways they regulate. Fetal inheritance of maternal CVD genetic risk alleles may be relevant to the transcript abundance of specific miRNAs if a quantitative relationship exists between specific genetic loci and microRNA expression levels within the placenta, similar to those previously identified within RICHS placentae on the mRNA level.Reference Peng, Deyssenroth and Di Narzo39
The functional relevance of miRNAs is largely dictated by the mRNAs available for them to interact with. These interactions are heavily influenced by the tissue of origin of both miRNAs and their target mRNAs. While many of the DEmiRs we identified (miR-324-5p, miR-520a-3p, miR-574-5p, miR-326) are implicated in cardiometabolic conditions during pregnancy, including preeclampsia and diabetes,Reference Gunel, Hosseini, Gumusoglu, Dolekcap and Aydinli40-Reference Jayabalan, Nair and Nuzhat43 their functional role remains largely understudied in the context of placental physiology.
Pathway overrepresentation analyses of DEmiR predicted targets overwhelmingly suggested TGFβ signaling to be influenced by dysregulation of placental miRNAs whose expression associates with maternal family history of CVD (Table 2). TGFβ signaling encompasses a large family of genes that participate in a myriad of cellular processes, but these genes are particularly important to vascular tissues for neovascularization as well as remodeling and repair of existing tissue.Reference Goumans, Liu and ten Dijke44
Table 2. DEmiR target pathway enrichment analysis results

Among our predicted DEmiR targets, specifically those of miR-574-5p, are SMAD2 and SMAD4; two activators and nuclear translocators of the SMAD-dependent TGFβ pathway, whose dysregulation are implicated in preeclampsia pathogenesis in human umbilical vein cells (HUVEC) in vitro.Reference Jones, Stoikos, Findlay and Salamonsen45-Reference Feng, Wang, Xu, Zou, Liang, Liu and Chen47 SMAD2/4 also appear frequently throughout the various biological pathways reported as overrepresented, many of which contribute toward TGFβ signaling (including activin and nodal signaling pathways), highlighting their importance to a diverse set of endothelial cellular processes and placental physiology as a whole (Table 2).Reference Watabe and Miyazono48
TGFβ signaling in the placenta is known to play critical roles in preimplantation and initial decidualization, and is also active throughout gestation in endometrial remodeling.Reference Jones, Stoikos, Findlay and Salamonsen45,Reference Ni and Li46 While neovascularization is required for successful fetal development, aberrant regulation of the signaling pathways overseeing this process may lead to improper vessel network development in the form of hypervascularization or vasculature malformation.Reference Arroyo and Winn49,Reference Kiba, Sagara and Hara50 MiR-574-5p was found to increase in expression with respect to maternal family history of CVD, and was also negatively correlated with two TGFβ pathway activators SMAD2 and SMAD4. The upregulation of miR-574-5p may lead to deficient SMAD2/4 levels, therefore preventing downstream activation of the TGFβ pathway (Fig. 2). This inhibition may result in broad changes to endothelial function in the placenta, leading to less optimal placental vasculature networks, or to the construction of immature vessels, ultimately manifesting as placental insufficiencies, and consequently, adverse gestational outcomes.
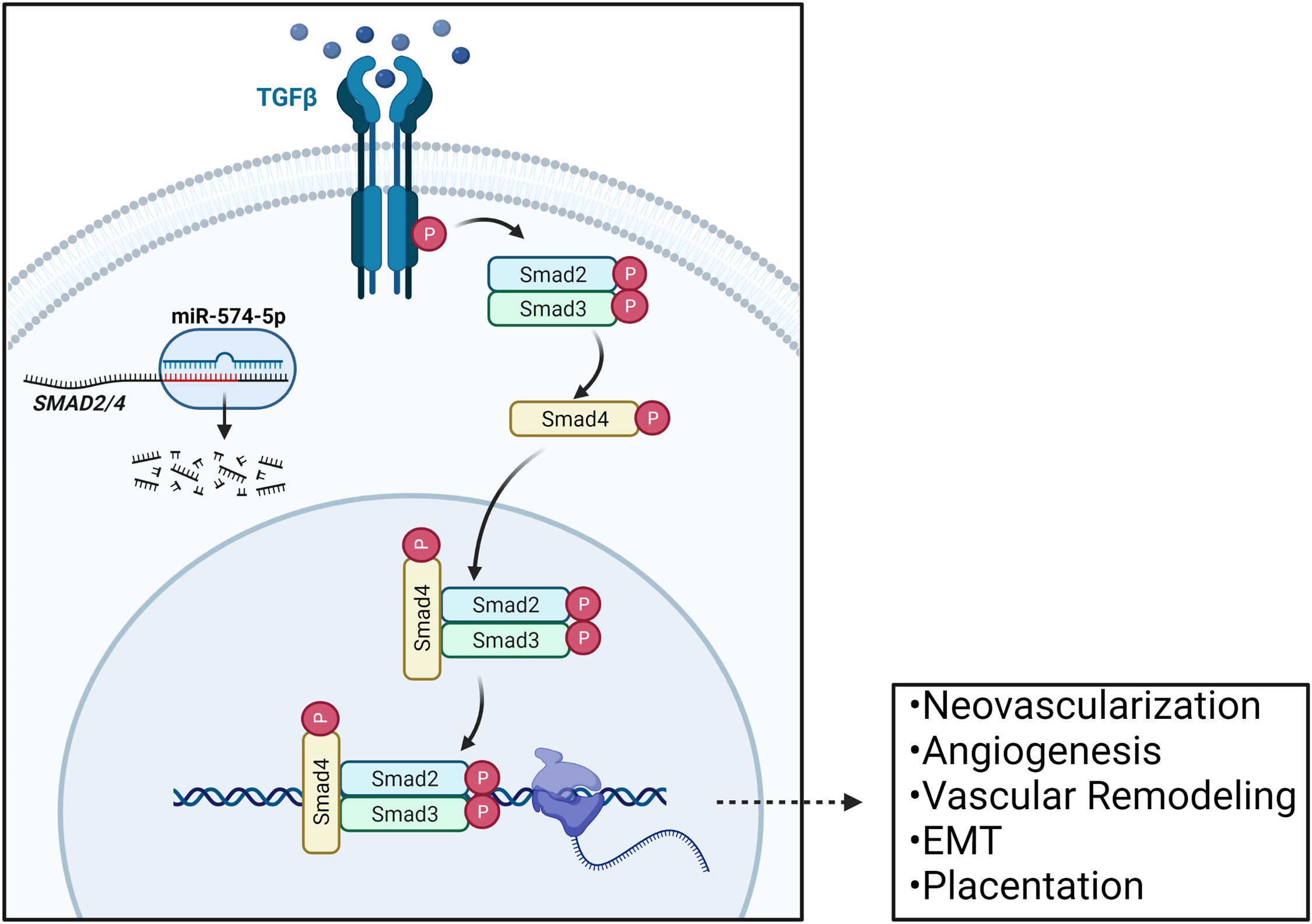
Fig. 2. Regulation of TGFβ signaling in the placenta via miR-574-5p mediated SMAD2/4 regulation. SMAD2 serves as an activator of the TGFβ pathway, and SMAD4 serves as a nuclear translocator. The mRNA transcripts of these genes are both predicted targets of miR-574-5p, and their dysregulation within the placenta may impact various TGFβ-controlled processes in endothelial cells throughout gestation, including: neovascularization, angiogenesis, vascular remodeling, epithelial to mesenchymal transitions, and overall placentation. Adapted from “TGFb Signaling Pathway”, by BioRender.com (2019). Retrieved from https://app.biorender.com/biorender-templates.
While miR-1246 was also shown to increase in expression with respect to maternal family history of CVD, its predicted targets did not pass our strict filtering criteria to be included in the pathway analysis. However, increased expression of miR-1246 has been shown to also promote angiogenesis through activation of TGFβ signaling via VEGF-mediated angiogenic pathways in HUVEC in vitro, but the exact mechanism by which this activation is achieved is not well understood.Reference Bai, Wang, Zhang, Zhang and Zhang51 The diverse regulatory potential of miR-574-5p and miR-1246 in overseeing multiple facets of the TGFβ pathway, and their dysregulation with respect to maternal family history of CVD, suggests an intricate balance of both positive and negative regulatory phases of endothelial processes that may be sensitive to both genetic and environmental risk factors associated with CVD.
These endothelial abnormalities are associated with placental insufficiencies, which, in turn, could result in adverse newborn health outcomes, acute maternal cardiometabolic complications and lifelong risk of chronic disease in offspring. This relationship between placental physiology and gestational outcomes in both mother and offspring may allow placental miRNAs to serve as biomarkers for risk of postpartum maternal cardiometabolic health complications as well as for early life chronic disease risk indicators in offspring, providing an opportunity for clinical implementation of early intervention and preventative measures to potentially alleviate the societal burden of CVD.Reference Cohen, Rychik and Savla18,Reference Camm, Botting and Sferruzzi-Perri19,Reference Ghulmiyyah and Sibai52
While the data presented here are incapable of concluding the direct impact of placental miRNA dysregulation, there is a growing body of literature, as well as the associations outlined here, that suggest dysregulation of placental miRNAs may be contributing to the developmental programming and propagation of chronic diseases, such as CVD. However, these findings should be interpreted within the context of this study’s limitations. Further work validating the pathophysiological significance of the dysregulated placental miRNAs identified here should be conducted prior to application in public health and/or clinical settings, including both replication of these findings, as well as experimental studies to better demonstrate underlying mechanisms.
This study only includes term placentae from live births, where premature births and other birth defects were excluded. The cross-sectional design of this study limits the interpretation of miRNA associations temporally, and may not be representative of miRNA associations throughout gestational development. This study relies on self-reported family history of CVD, and which may ultimately lead to variation in results attributable to recall bias. While we tested for various confounders to the best of our ability, there is still a possibility of unmeasured confounding which remains in our analysis. Lastly, the RICHS cohort consists predominantly of healthy, white mothers and their offspring from the New England region of the United States, potentially limiting the ability of these results to be generalized to those with acute cardiometabolic complications, and to more racially diverse populations.
Conclusion
MiRNAs serve as an important form of post-transcriptional gene regulation during early development, and are sensitive to both genetic and environmental conditions. Here we have shown that the expression of 9 placental miRNAs are associated with maternal family history of CVD, and that the mRNA targets of these miRNAs largely play a role in TGFβ signaling, indicating their involvement in endothelial cell functionality and placental physiology as a whole. Dysregulation of miRNA expression in the placenta may contribute to adverse newborn and maternal health outcomes, ultimately playing a role in the developmental programming of chronic diseases, including CVD.
Supplementary materials
For supplementary material for this article, please visit https://doi.org/10.1017/S2040174422000319
Acknowledgements
We acknowledge the cooperation of the participants enrolled in the RICHS, and the contributions of the research staff who also worked on RICHS.
Financial support
This work was supported by the National Institutes of Health (NIH-NIGMS T32GM008490, NIH-NIEHS R24ES028507, NIH-NIEHS R01ES025145, NIH-NIEHS P30 ES019776; NIH-NIGMS P20GM104416).
Conflicts of interest
The authors declare they have no competing interests or personal relationships that would potentially influence the work presented in this paper.
Ethical standards
All participants provided written, informed consent and all protocols were approved by the IRBs at the Women & Infants Hospital of Rhode Island and Emory University, respectively.