Introduction
Suboptimal maternal pre-pregnancy body mass index (BMI; underweight, overweight or obesity) is known to adversely influence the development of the fetus and child, shaping risk for poor cognitive outcomesReference Veena, Gale, Krishnaveni, Kehoe, Srinivasan and Fall1-Reference Casas, Chatzi and Carsin4 and disease trajectories throughout life.Reference Veena, Gale, Krishnaveni, Kehoe, Srinivasan and Fall1,Reference Bilbo and Tsang5 While the mechanisms driving these developmental outcomes are incompletely understood, low and high maternal pre-pregnancy BMI may dysregulate shared endocrine,Reference Johns, Denison and Reynolds6-Reference Lesage, Sebaai and Leonhardt8 inflammatoryReference Parisi, Milazzo, Savasi and Cetin9,Reference Stavropoulos-Kalinoglou, Metsios and Panoulas10 and nutritional pathways,Reference Gaccioli, Lager, Powell and Jansson11-Reference Farley, Choi and Dudley14 which in turn may impact on offspring (neuro)development.Reference Fitzgerald, Hor and Drake15,Reference Bordeleau, Fernández de Cossío, Chakravarty and Tremblay16 In Canada, approximately one in four women ages 20–39 have obesity.Reference Bushnik17 Higher maternal BMI during pregnancy in rats has been linked to a reduction in fetal neurotropic factors, poor neuronal differentiation, reduced brain plasticity and reduced overall cognitive function.Reference Bilbo and Tsang5 In humans, both pre-pregnancy maternal overweight and obesity have been associated with poorer neurodevelopmental outcomes.Reference Huang, Yu, Keim, Li, Zhang and Zhang3,Reference Sanchez, Barry and Sabhlok18 Similarly, infants born to mothers who are underweight may have poorer outcomes, including low birthweightReference Al-Hinai, Al-Muqbali, Al-Moqbali, Gowri and Al-Maniri19,Reference Murai, Nomura, Kido, Takeuchi, Sugimoto and Rahman20 and reduced verbal comprehension.Reference Li, Zhu and Zeng21
The postnatal environment also has a profound influence on child development, including brain development and function, and an enriched postnatal environment has the potential to correct developmental trajectories set in utero. Exposures to bioactive components in breast milkReference Ballard and Morrow22-Reference Innis24 and the duration of infant breastfeedingReference Bernardo and Cesar25 have also been shown to influence infant and child cognitive development, and exclusive breastfeeding for the first 6 months of life is associated with increased performance on intelligence tests and greater academic achievement.Reference Bernardo and Cesar25-Reference Kramer and Kakuma27
Beyond nutrition, parental education levels and the home and social environments are critical to shaping child cognitive development. Higher parental education levels, which may create a more stimulating home environment, better predict success on cognitive and academic tests in older children than the type of school attended by children.Reference Ardila, Rosselli, Matute and Guajardo28-Reference Bradley and Caldwell30 Home quality, including level of caregiver support and engagement with children and safety of household conditions, is also a significant predictor of child cognitive outcomes.Reference Bradley and Caldwell30 Importantly, studies that show a positive and moderating effect of social factors on child cognitive outcomes typically assess children during middle childhood and adolescence.Reference Ardila, Rosselli, Matute and Guajardo28-Reference Gluckman, Hanson, Spencer and Bateson32 Yet, assessing child cognitive outcomes during infancy and early childhood could allow for earlier identification of children on course for suboptimal development, and greater opportunity to intervene to correct paths established in utero and infancy. Further, we know that a mother’s social and environmental contexts can shape the home environment and also determine her metabolic health.Reference East33 Collectively, this highlights the importance of considering the complex, context-specific biopsychosocial determinants of child cognitive development when investigating how early life environments influence neurodevelopment.
To address this complexity, we first aimed to quantify associations between maternal pre-pregnancy BMI and child cognitive outcomes in a multi-site cohort in Canada. Next, we performed exploratory analyses to investigate relationships between maternal pre-pregnancy BMI, early nutritional environments (mother-reported breastfeeding behaviours), contextual and social factors (maternal education level, enrichment in the home environment) and child cognitive outcomes. We hypothesised that suboptimal maternal pre-pregnancy BMI (underweight, overweight or having obesity) would associate with poorer cognitive outcomes in children 3 years of age. We also hypothesised that higher maternal education levels, an enriched home environment, and increased breastfeeding practices would positively associate with child cognitive outcomes and may mitigate negative relationships between suboptimal maternal BMI and child cognitive development.
Methods
This study received ethics approval from the Carleton University Research Ethics Board (#106172) and the Health Canada Research Ethics Board (#2016-028H).
Study population
Data were collected as part of the Maternal-Infant Research on Environmental Chemicals (MIREC) study, a multi-site prospective cohort study of 2001 pregnant women who were attending prenatal clinics during the first trimester of pregnancy (6 to <14 weeks), recruited from 10 cities (11 hospital centres) across Canada between 2008 and 2011.Reference Arbuckle, Fraser and Fisher34 All study participants gave written informed consent. A follow-up study of the MIREC children (MIREC-CD Plus), recruited 610 families with children 2–5 years of age from six participating sites (Vancouver, Toronto, Kingston, Montreal and Halifax). Neurodevelopmental testing was performed on children 3–4 years of age. Women with a known history of medical complications (including heart, pulmonary, renal or liver disease, epilepsy, drug use, cancer, history of spontaneous abortion or haematological disorders) were excluded from the study. Additional inclusion criteria for the present study were singleton pregnancies, data collected on maternal pre-pregnancy and pregnancy weight (at 6–13 weeks, 16–21 weeks, 32–34 weeks and at delivery) and completion of the Weschler’s Preschool and Primary Scale of Intelligence, 3rd Edition (WPPSI-III) test by children at 3 years of age.Reference Arbuckle, Fraser and Fisher34 To be eligible for a neurological assessment, children had to be delivered at ≥28 weeks' gestation, with no major congenital birth defects, seizures or major neurological disorders during the perinatal period. No other exclusions were applied. Our final sample included 528 mother−child pairs (Fig. 1). Pairwise deletion was used for all adjusted models.
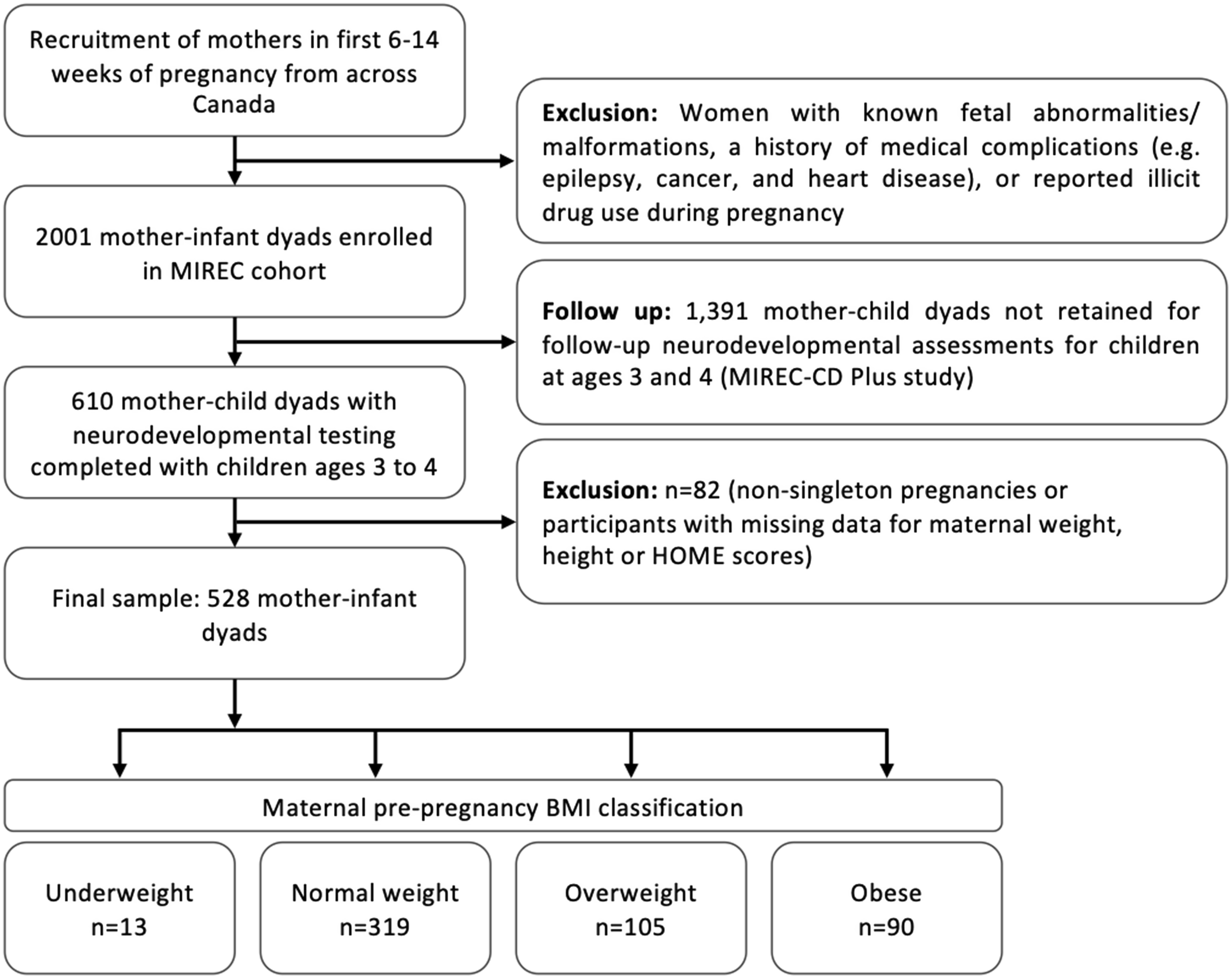
Figure 1. Participant and sample population flow chart.
Maternal BMI
Our primary potential risk factor was maternal pre-pregnancy BMI, derived from the self-reported pre-pregnancy weight and measured height between 6 and 14 weeks of pregnancy. BMI was classified according to definitions set by the World Health Organization (underweight: <18.5 kg/m2; normal weight: 18.5–24.9 kg/m2; overweight: 25–29.9 kg/m2; obese: ≥30 kg/m2).35 The majority of women in our cohort had pre-pregnancy BMI greater than 18.5 kg/m2; therefore, analyses for maternal underweight are exploratory and should be interpreted with caution. We explored associations across BMI classifications as well as the continuous associations of BMI with primary outcome measures. Additional covariates of interest included maternal age, maternal pre-pregnancy diabetes diagnosis (self-reported from questionnaire between 6 and 14 weeks of pregnancy) and infant outcomes (gestational age at delivery, sex and birthweight).35
Breastfeeding exposures
Breastfeeding practice and behaviours were self-reported when the infant was 6 months of age and were available for 260 of the mother-infant dyads in our cohort. Mothers were first asked: ‘Did you breastfeed your baby (including the milk that you express for bottles)?’. Follow-up questions asked included: ‘Does your baby drink any kind of milk other than breast milk (e.g. cow’s milk or commercial infant formula)?’, ‘Has your baby ever been fed with commercial infant formula?’, and ‘Are you still presently feeding him/her with commercial infant formula?’. For the current exploratory analyses, variables were derived from these responses to indicate whether, in the first 6 months, a mother had: ever breastfed, exclusively breastfed up to 6 months of age, intended to continue breastfeeding past 6 months of age, attempted to breastfeed once, stopped breastfeeding prior to 6 months of age, ever formula fed, and intended to continue formula feeding past 6 months of age. Due to the nature of the questionnaire responses, these categories are not mutually exclusive. Therefore, analyses can only evaluate these infant feeding behaviours as dichotomous responses and not independently of each other.
Child cognitive outcomes
The WPPSI-III, which is a validated and accepted tool used to assess the intellectual functioning of children, was administered when the child was 3 years of age.Reference Weschler36 The WPPSI-III contains several subtests that assess children’s intellectual functioning in verbal and performance cognitive domains. The Full-Scale Intelligence Quotient (FSIQ) is an overall measure of the composite scores and measures the child’s overall cognitive functioning. Question composite scores were recorded for the following subtest categories: Full Scale, Verbal, Performance and General Language. Scale scores were recorded for all other subtest categories: Receptive vocabulary, Block design, Information, Object assembly and Picture naming. For the purposes of descriptive statistics and some analyses, the composite scores were divided into three previously defined categories based on WPPSI guidelines: low (scores <90), average (scores 90–109) and high (scores >90).Reference Weschler36 The scale scores were also divided into three categories: low (scores <7), average (scores 7–12) and high (scores >12).Reference Weschler36 By dividing the WPPSI-III scores into these categories, we could investigate the different impacts of the potential risk factors and covariates on overall intellectual functioning (including the likelihood of children being intellectually gifted or experiencing learning difficulties).
Contextual and social determinants
To assess whether contextual and social factors influenced the relationships between maternal BMI, early life breastfeeding practice and child cognitive outcomes, we considered key factors known to influence maternal-child health: maternal education and the home environment.Reference Schady29,Reference Bradley and Caldwell30,Reference Gluckman, Hanson, Spencer and Bateson32,Reference Lee, Park and Ha37-Reference Zhou, Baghurst, Gibson and Makrides39 The Home Observation Measurement of the Environment (HOME) Inventory was used to assess the quality and quantity of child support and stimulation available to the child in their home environment at 3−4 years of age.Reference Bradley and Caldwell30 The HOME scoring process was carried out by trained research staff and involved both observational and semi-structured components. During observation of the home environments, researchers rate the quality of stimulation and absence of hazards in the home, as well as mother−child interactions using a binary (yes or no) 45-item scale. In the semi-structured component, researchers interviewed the mother and collected a maternal account of the child’s experience at home on a given day of the week. In addition to analysing the HOME score as a continuous variable, we also report the HOME scores stratified by quartile cut-off points, provided by the HOME Administration manual,Reference Caldwell and Bradley40 to evaluate categorical variance. According to the manual, HOME scores in the lowest quartile, or those more than seven to eight points below the median, may be of concern for cognitive development.Reference Caldwell and Bradley40 The three categories derived using the HOME Administration manual quartile cut-off points were: below average (lowest quartile; score <45, n = 123), average (inter-quartile range; ≥45 to <50, n = 223) and above average (upper quartile; ≥50, n = 182) HOME score. Data collection centres, indicated as ‘centre’ in analyses, were included for descriptive purposes to evaluate population differences across Canadian data collection centres.
Statistical analysis
Analyses were conducted using SAS software (Version 9.4 of the SAS System for Windows, SAS Institute Inc., Cary, NC, USA). Descriptive statistics were used to evaluate differences in maternal and infant health and demographic factors across maternal BMI classifications and outcome variables (WPPSI-III full and sub-scores). Univariable analysis (ANOVA, and logistic regression for breastfeeding variables) were used to compare differences in outcome measures across maternal BMI classifications for outcome measures (p < 0.05).
Variables that displayed significant univariable relationships (p < 0.05) with our primary (FSIQ) and secondary outcomes (WPPSI sub-scores) were included in multivariable linear models. Variables significant at alpha 0.05 in stepwise backwards elimination were retained in the final multivariable models. The effect estimate (β) was used to indicate effect size (interpreted as Cohen’s f 2 : small = 0.02, medium = 0.15, large > 0.35). These models were also stratified by child sex and used to identify key factors which influence child cognitive outcomes (effect estimate [β] and variance [R 2]).
Lastly, we sought to understand whether key environmental variables moderated the relationships between maternal BMI and cognitive outcomes. We used unadjusted structural equation models described by Iacobucci et al. to test these effects.Reference Iacobucci41,Reference Iacobucci, Saldanha and Deng42 Data are presented as (mean ± SD) with significance set at p < 0.05.
Visualisation of candidate pathways
Directed acyclic graphs (DAGs) were used to display relationships between effect and outcome variables, as well as to display confounding, mediating and covariate relationships. Visual display of these relationships allows potential casual inferences to be drawn from observational data.Reference Shrier and Platt43 Using DAG techniques laid out by Shrier and PlattReference Shrier and Platt43 and regression analyses results, we developed diagrams to display pathways of potential relationships between variables observed from the data.
Results
Population description
In our study cohort, the breakdown of maternal BMI classification was: underweight (n = 13, 2.7%), normal weight (n = 319, 60.4%), overweight (n = 105, 19.9%) and obese (n = 90, 17.0%). Mothers with obesity had lower education levels than those of normal weight, with 42.2% having university-level education (vs. 74.0%, p = <0.0001; Table 1). HOME scores were lower for mothers who were underweight or who had obesity, but not those who were overweight, compared to mothers who were of normal weight (p = 0.0001, Table 1).
Table 1. Participant characteristics stratified by maternal pre-pregnancy BMI classification
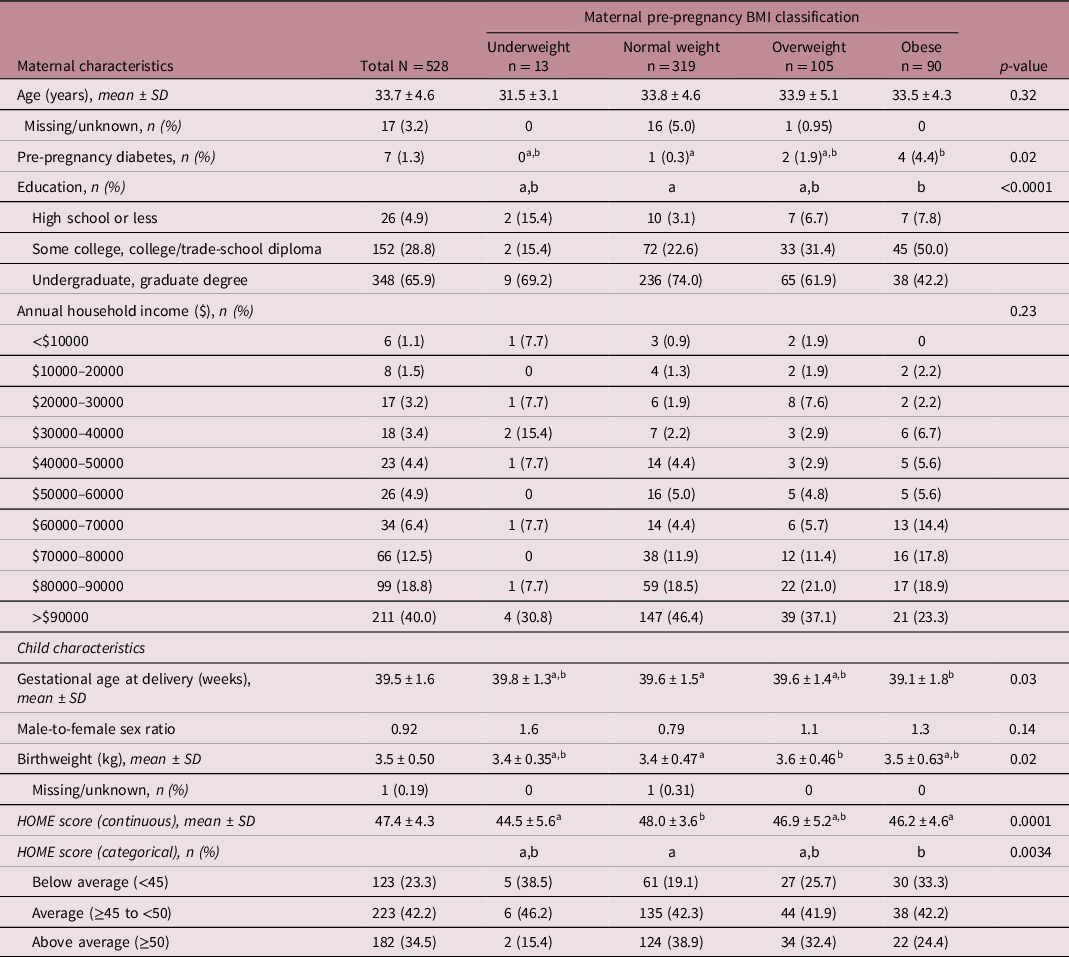
Statistically significant difference between BMI groups was determined at p < 0.05. Groups with different letters are statistically different (Tukey’s post hoc, p < 0.05). Maternal pre-pregnancy BMI was classified as underweight (<18.5 kg/m2), normal weight (18.5–24.9 kg/m2), overweight (25–29.9 kg/m2) or obese (≥30 kg/m2). HOME, Home Observation Measurement of the Environment.
The majority of mothers both initiated breastfeeding (n = 253, 97%), as well as formula fed their infant (n = 141, 99%) at some time in the first six postnatal months. Most mothers (n = 190, 73%) indicated intention to ‘continue breastfeeding’ past the 6-month postnatal period, while 45% (n = 116) of women exclusively breastfed their infant for the recommended 6-month period. Most also had intentions to continue formula feeding past the 6-month age of the infant (n = 124, 87%; Supplementary Table 1).
Relationships between maternal BMI and breastfeeding
In the subset of our sample with available data on breastfeeding practices, continuing to breastfeed infants at 6 months postpartum was more frequent in mothers of normal weight compared with mothers with suboptimal BMI (76.6% normal weight vs. 66.7% underweight, 71.2% overweight and 66.0% obesity). Mothers with normal pre-pregnancy BMI were less likely to stop breastfeeding by 6 months and less likely to never breastfeed their child within 6 months postpartum than mothers in all other BMI categories (Supplementary Table 1). However, there were no statistical differences in breastfeeding or formula feeding behaviours across maternal pre-pregnancy BMI categories (Supplementary Table 1).
Cognitive outcomes associated with maternal pre-pregnancy BMI
Most children in our cohort scored within the average (90–109; n = 232, 43.9%) or high (>109; n = 246, 46.6%) range for FSIQ. Children born to mothers who had obesity (but not overweight or underweight mothers) had lower FSIQ scores than children of mothers with normal pre-pregnancy BMI (104.2 ± 12.8 vs. 108.4 ± 13.7, p = 0.049, Table 2). Children of mothers with obesity also had lower verbal reasoning (105.8 ± 12.9 vs. 111.1 ± 13.5, p = 0.03) and information (11.1 ± 2.5 vs. 12.0 ± 2.6, p = 0.02) scores compared to those of normal weight mothers (Table 2). In univariable analyses, maternal pre-pregnancy BMI was negatively associated with child FSIQ (β = −2.0 [−3.4, −0.59], p = 0.006), verbal reasoning (β = −1.9 [−3.3, −0.53], p = 0.007) and information scores (β = −0.41 [−0.70, −0.14], p = 0.003; Table 3).
Table 2. Continuous and categorical distribution of WPPSI-III scores by maternal pre-pregnancy BMI classification
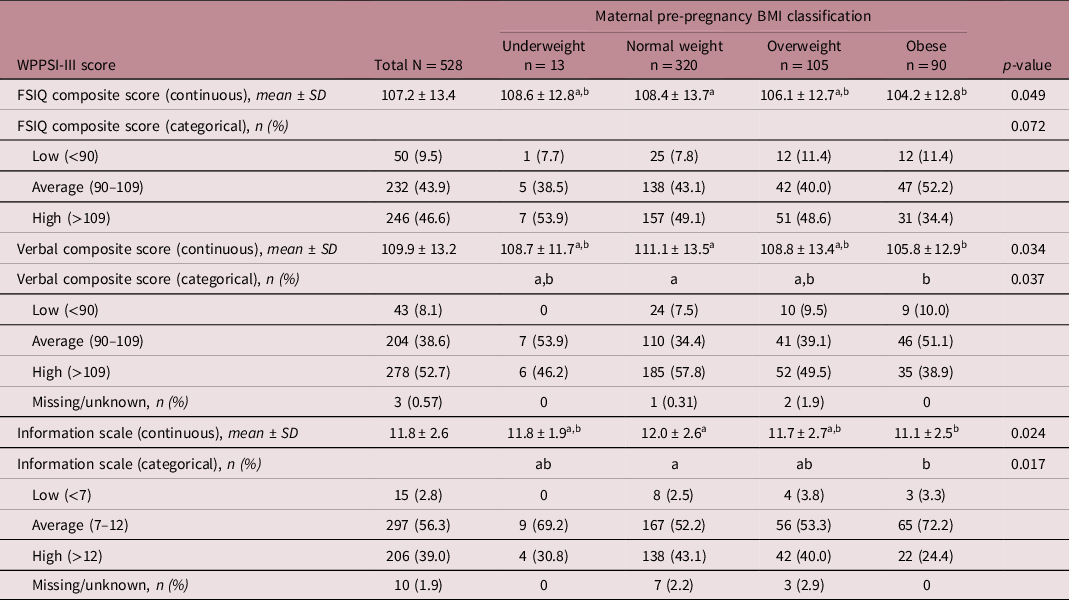
The distribution of WPPSI-III scores across BMI categories were analysed as continuous variables and in previously defined categories.Reference Kramer, Aboud and Mironova26 Statistically significant differences between BMI groups were determined at p < 0.05. Groups with different letters are statistically different (Tukey’s post hoc, p < 0.05). Average WPPSI composite scores are 110–120 (below 90 may indicated cognitive disability), and average WPPSI scale scores are 7–12. FSIQ, Full-Scale Intelligence Quotient; WPPSI, Weschler’s Preschool and Primary Scale of Intelligence, 3rd Edition.
Table 3. Moderating influence of social contextual factors on the relationship between maternal pre-pregnancy BMI and child cognitive development
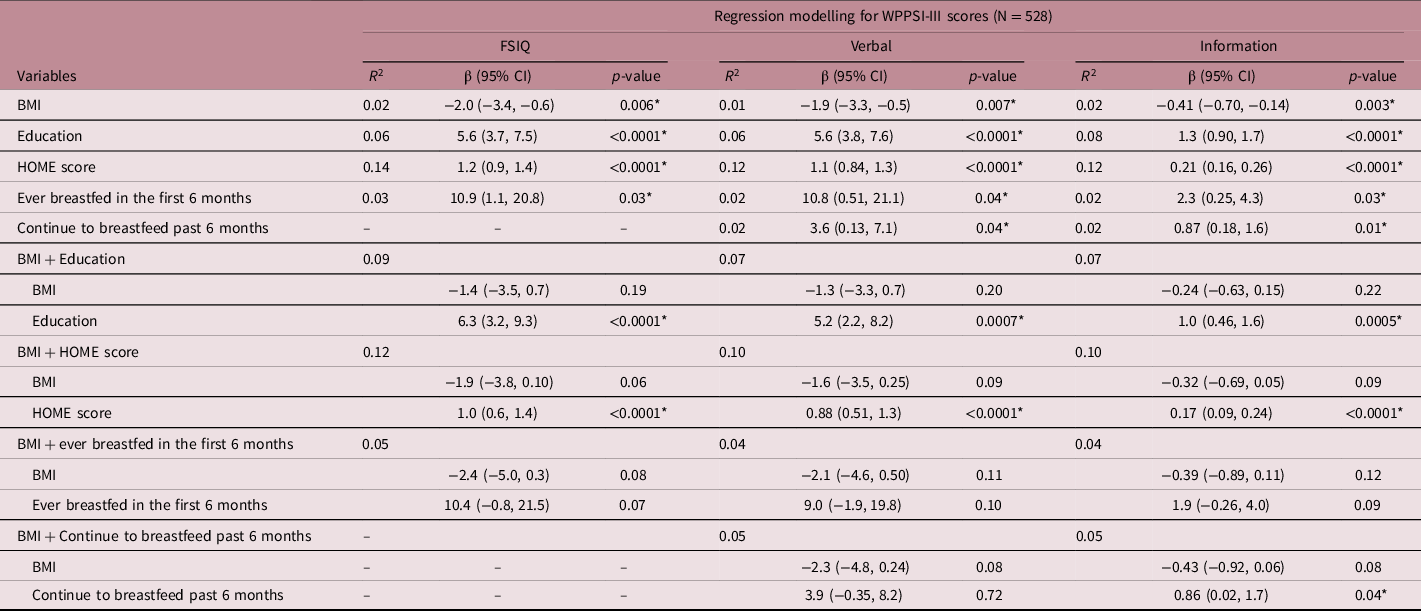
Moderating variables are identified by the significant influence of both main effect (X) and moderating (M) variables independently on the WPPSI-III score (Y), and an identifiable change in effect size when both X and M are included in the model. Statistically significant variables at α < 0.05 are included in following model step.
Contextual factors influenced cognitive outcome
Maternal education level was positively associated with child FSIQ (β = 5.6 [3.7, 7.5], p < 0.0001), verbal (β = 5.6 [3.8, 7.6], p < 0.0001) and information (β = 1.3 [0.90, 1.7], p < 0.0001) scores; as were HOME scores (FSIQ: β = 1.2 [0.92, 1.4], p < 0.0001; verbal: β = 1.1 [0.84, 1.3], p < 0.0001; information: β = 0.21 [0.16, 0.26], p < 0.0001), and having ever been breastfed (FSIQ: β = 10.9 [1.1, 20.8], p = 0.03; verbal: β = 10.8 [0.51, 21.1], p = 0.04; information: β = 2.3 [0.25, 4.3], p = 0.03; Table 3).
Contextual factors moderated associations between maternal pre-pregnancy BMI and cognitive outcomes
Higher maternal education and HOME score attenuated the negative relationship between suboptimal maternal pre-pregnancy BMI and poor child cognitive outcomes (Table 3). The strength of the association between maternal pre-pregnancy BMI and FSIQ, verbal reasoning and information scores was reduced by 30%–41% and 7%–22% when maternal education or HOME scores were accounted for in the moderating analyses, respectively (Table 3). The intention to continue breastfeeding beyond 6 months displayed a very small moderation of the BMI estimate for information score, which was negligible in size (change in β < 0.01). No other variables had a significant moderating impact on the relationship between BMI and child cognitive outcomes (Table 3).
Sex-based differences in relationships between maternal pre-pregnancy BMI, contextual factors and cognitive outcomes
When stratified by child sex, there was a negative association between maternal pre-pregnancy BMI and verbal reasoning scores in female (−3.0 [−5.8, −0.12], p = 0.04, R 2 = 0.10), but not male, children (Supplementary Tables 2 and 3). Education, HOME score and centre accounted for 21% and 11.6% of the variation in FSIQ scores for males and females, and education and HOME score accounted for 17.8% and 12.9% of the variation in information scores for males and females, respectively (Supplementary Table 3).
Candidate pathways between contextual factors and child WPPSI scores
We used DAGs to qualitatively represent relationships between maternal pre-pregnancy BMI and FSIQ, verbal and information scores. Possible pathways (unbroken sequence of variables)Reference Sauer, VanderWeele, Velentgas, Dreyer and Nourjah44 between maternal BMI and child cognitive outcomes, with independent kinships (relationships within a path)Reference Sauer, VanderWeele, Velentgas, Dreyer and Nourjah44 through HOME score, breastfeeding (e.g. ever breastfed in the first 6 months or intention to continue breastfeeding past 6 months), and education are presented in Fig. 2a–2c. Potential relationships existed between maternal pre-pregnancy BMI and breastfeeding practices (Fig. 2a and 2c), and maternal education and the home environment (Fig. 2a–2c). Thus, our results suggest that a mother’s education level may influence a variety of contextual factors not included in our research variables, which through other (un)measured variables, can influence child cognitive outcomes. For example, maternal education level has a complex relationship with contextual factors of economic, food and housing security, as well as the HOME score (home environment). Through the HOME score, maternal education may ultimately influence child cognitive outcomes. Breastfeeding practices (ever having breastfed in the first 6 months, or continuing to breastfeed past 6 months) are influenced by contextual factors related to maternal BMI, which in turn influenced cognitive outcomes measured by the information scale.
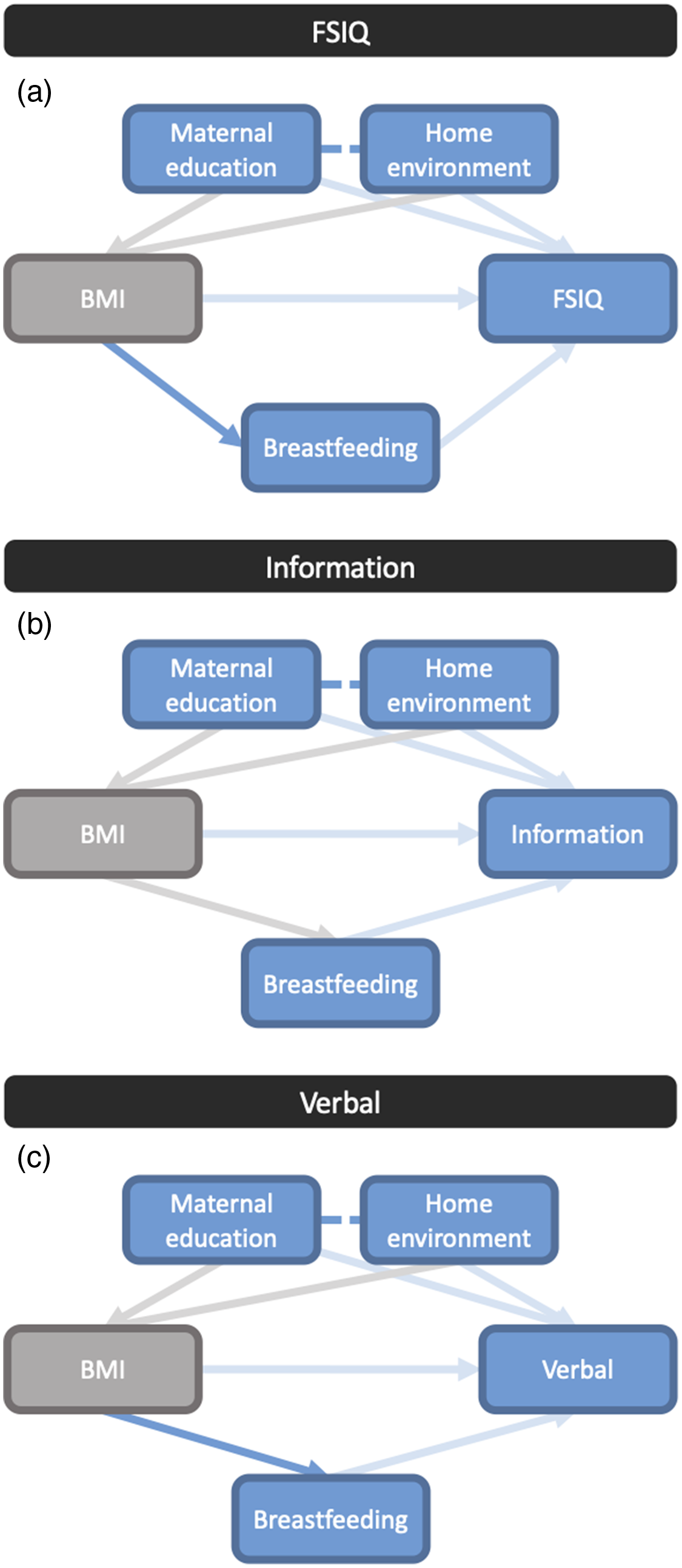
Figure 2. Directed acyclic graph (DAG) displaying pathways in the relationship between maternal pre-pregnancy BMI and infant. (a) Full-Scale Intelligence Quotient (FSIQ), (b) verbal, and (c) information scale scores. Each line represents a pathway connecting variables, with arrow heads pointing in the direction of the association. Solid blue lines indicate the direct pathways identified in DAG analyses. Faded (light blue or grey) lines represent relationship pathways that can be identified but cannot be confirmed as direct when other variables are accounted for, which highlights the complex and multitude of contextual variables in the relationships. Blue lines represent the pathways from exposures to primary outcomes, while grey lines represent connections between exposure variables. Dashed lines connect variables which have an effect on the same outcome variable.
Discussion
Using data from a pan-Canadian prospective pregnancy cohort, we found that cognitive outcomes for children at 3–4 years of age were negatively associated with high maternal pre-pregnancy BMI, particularly in mothers who had obesity. Cognitive scores were lower among children born to mothers with obesity than normal weight mothers. However, social environment factors such as maternal education and the home environment can predict positive cognitive outcomes, and we showed that these can explain the negative influence of maternal BMI on cognitive outcomes.
Our observed associations between elevated maternal pre-pregnancy BMI and classification of having obesity with poorer child cognitive performance at age three, specifically for FSIQ, verbal, and information score subsets of the WPPSI, is consistent with previous literature findings where higher maternal BMI is associated with poorer child cognitive outcomes.Reference Bilbo and Tsang5,Reference Nichols, Rundle and Factor-Litvak45 Pregnancy is associated with changes in the maternal inflammatory milieu, and obesity also contributes to chronic, sub-clinical inflammation, which has been linked to adverse health outcomes for the infant and mother.Reference Schmatz, Madan, Marino and Davis46 This pro-inflammatory intrauterine environment may have impacts on congenital structural defects and overall childhood cognitive functioning.Reference Veena, Gale, Krishnaveni, Kehoe, Srinivasan and Fall1,Reference Craig, Palomaki, Neveux and Haddow2,Reference Schmatz, Madan, Marino and Davis46,Reference Rivera, Christiansen and Sullivan47 Further, dysregulated signalling of metabolic hormones, including insulin and leptin, or exposure to excess nutrient levels during critical periods of neurodevelopment may lead to suboptimal cognitive outcomes in offspring.Reference Rivera, Christiansen and Sullivan47 Whether our observed subtle differences in WPPSI in early childhood persist throughout childhood/adolescence and influence factors like school readiness and later cognitive capacity remains to be determined. However, WPPSI assessments and scores are considered to be a good predictor of cognitive success in later life.Reference Weschler36
While there were differences in WPPSI cognitive scores between BMI groups in our study, once other variables such as maternal education level and home environment were considered, the effect of maternal pre-pregnancy BMI on cognitive outcomes was either minimised or fully explained by other social variables. This finding aligns with our hypothesis, as well as results from a similar study that investigated the effects of maternal hyperglycaemia and obesity on child neurodevelopmental problems at ages three to four.Reference Krzeczkowski, Boylan and Arbuckle48 Here, the authors found associations between maternal adiposity and poorer cognitive outcomes, but as in our analysis, relationships did not remain significant after adjusting for confounding factors.Reference Krzeczkowski, Boylan and Arbuckle48 That higher parental education level is associated with a more resource-rich environment, helping children to perform better on cognitive tests,Reference Ardila, Rosselli, Matute and Guajardo28,Reference Schady29,Reference Eriksen, Kesmodel, Underbjerg, Kilburn, Bertr and Mortensen49 and HOME scores can reflect the cognitive stimulation a child has received at home,Reference Leventhal, Selner-O'Hagan, Brooks-Gunn, Bingenheimer and Earls50 is consistent with our finding of a positive association between maternal education and home environments with child cognitive outcomes.
We also found that both maternal education and home environment accounted for approximately 5%–10% greater variation in male cognitive scores compared to female infants once the negative impact of maternal BMI was buffered. Male infants are known to be more vulnerable to adverse exposures in early lifeReference Andersen51 and to the development of neurodevelopmental disorders.Reference DiPietro and Voegtline52 Thus, it is plausible that the protective effects of a positive home environment are greater for males than females. It is also possible that sex or gender-influenced rearing practices in the home environment may account for some of this variation,Reference Endendijk, Groeneveld, Bakermans-Kranenburg and Mesman53 however, the extent that this is true in the Canadian context is unclear. Whether sex-based differences in the degree that social environments moderate the relationship between maternal pre-pregnancy BMI and cognitive development persist throughout childhood must be better understood, as improved knowledge on these complex relationships could inform targeted strategies that promote positive cognitive outcomes in children, even for those who have been exposed to suboptimal environments during critical windows in early development.
Maternal BMI can influence breastfeeding practices and early nutritional environments by influencing the composition of breastmilk as well as the frequency and duration of breastfeeding.Reference Mäkelä, Linderborg, Niinikoski, Yang and Lagström54 Among women with overweight and obesity, psychological barriers related to breastfeeding, such as low confidence in one’s ability to breastfeed, have been described and associate with lower rates of breastfeeding initiation and duration.Reference Chang, Glaria, Davie, Beake and Bick55 In the MIREC cohort, higher maternal education level is associated with likelihood of breastfeeding past 6 months of age,Reference Till56 and in our sample population, mothers with obesity had lower education levels than normal weight mothers, suggesting that maternal education level may influence relationships between maternal BMI and breastfeeding practices. In our exploratory analysis, we did not find any strong relationships between breastfeeding practices and child cognitive outcomes, likely due to limited infant feeding data. Even so, in the DAG analysis we did identify a potential direct pathway between maternal pre-pregnancy BMI category and breastfeeding practices, such as initiating and continuing breastfeeding. Our finding that having ever been breastfed associated with higher cognitive scores, and the known relationship between breast milk bioactive molecules, including nutrients, and infant and child development suggest that supporting new mothers in their breastfeeding practices may be a potential intervention pathway to promote child cognitive health.Reference Ballard and Morrow22-Reference Innis24
Strengths and Limitations
Strengths of our study include the nation-wide collection of biometrics and early life exposures throughout the perinatal period and early childhood, as well as data on the home environment, which few pregnancy studies capture. The evaluation of early life exposures in this study and use of data and child health outcomes from early childhood (rather than from middle childhood or adolescence) allows us to identify relationships earlier when studying the perinatal period and cognitive development. Future studies that consider longitudinal measurements of child cognitive outcomes from the perinatal period through adolescence will provide a more comprehensive picture of the potential mechanisms that underlie child cognitive development and function.
Despite our geographically diverse cohort, our population was less diverse in other measures: mothers had a higher-than-average household income than the Canadian population average, higher educational levels, and were generally healthy and predominantly White/Caucasian, with little ethnic diversity. This lack of demographic diversity means that populations which are at higher risk of poor health due to contextual socio-economic factors are not well represented in our population. Infant feeding data were also based on unvalidated self-reports and only available for about half our cohort, and there were 82 participants who were missing data on our exposure variables of interest, or who had a non-singleton pregnancy, and were subsequently excluded from our analyses. It is possible that these factors introduced further selection and reporting bias, and may have reduced the representativeness of our sample. More generally, one must also acknowledge the limitations to the HOME instrument, including the use of a binary (yes or no) response scale, limiting the assessor’s ability to capture nuance in the quality and quantity of child support and stimulation. Further, the HOME score lacks a standardised procedure for administration, and has not proven to be sensitive to some high-risk home environments, including those with parental illicit substance use.Reference Totsika and Sylva57 Despite these limitations, the HOME score remains widely used to measure the quality of a child’s home environment and is recognised to be a strong predictor of child cognitive development.Reference Totsika and Sylva57-Reference Lett, Stingone and Claudio60 Maternal pre-pregnancy BMI data were also calculated from self-reported weight, which is known to be an underestimation of true weight, particularly among women and those with overweight or obesity.Reference Elgar and Stewart61 This may have contributed to some confounding in our results, underestimating the BMI distribution within our subcohort, and the small sample of mothers who were underweight means that findings for this subset should be interpreted with caution. It should also be noted that the combined prevalence of overweight and obesity in this subset of the MIREC cohort was 36.9%. While this is below the Canadian prevalence of overweight and obesity among adult women (ages 20–34: 43.6%, ages 35–49: 59.2%),62 we believe that the cohort size and scope of the data collected in the MIREC study make this cohort well-positioned to study these exposure-outcome relationships. Lastly, beyond the covariates considered in our models (maternal age and pre-pregnancy diabetes diagnosis, and infant gestational age at delivery, sex and birthweight), there are also additional variables that may confound relationships between maternal BMI, the home environment, and child neurodevelopment, including maternal mental health status, pregnancy history, unmeasured factors contributing to maternal obesity or suboptimal neurodevelopment and child cognition, and unmeasured contextual factors. BMI itself may also be a proxy for social gradients.Reference Lundberg, Ryd, Adiels, Rosengren and Björck63,Reference Hoebel, Kuntz and Kroll64 However, limitations in available data and restrictions on further analyses due to the SARS-CoV-2 pandemic prevent us from exploring these relationships further at this time. Even so, our results highlight the importance of these social and environmental variables even within a relatively homogenous population, and should be thoroughly explored in populations with greater ethnic and socio-economic diversity in the future.
Conclusion
Using data from a prospective Canadian cohort, we found that negative associations between maternal pre-pregnancy BMI and child cognitive outcomes were either minimised or fully explained by other social variables, including maternal education and the home environment. Understanding the various social, environmental, and biological factors operating preconception and perinatally that may place an infant at a cognitive disadvantage later in life, including how risk factors cluster and compound one another (e.g. lower maternal education level, resource-limited home environment and lower likelihood of breastfeeding), and which factors are associated with positive cognitive outcomes (e.g. breastfeeding, being read to at home,Reference Logan, Justice, Yumuş and Chaparro-Moreno65,Reference Kalb and van Ours66 a sense of security and belongingReference Sciaraffa, Zeanah and Zeanah67) is critical to uncovering causal mechanisms. It is also essential for informing strategies to predict and promote child cognitive health, which may guide health practice and policy, the development of context-specific supports to promote breastfeedingReference Bever Babendure, Reifsnider, Mendias, Moramarco and Davila68-Reference Leeming, Marshall and Locke70 or improve parental education or the home environment,Reference McNally, McCrory, Quigley and Murray71 and ultimately child developmental outcomes.Reference Gilmer, Buchan and Letourneau72
Supplementary materials
For supplementary material for this article, please visit https://doi.org/10.1017/S2040174422000228
Acknowledgements
This study was funded by the Faculty of Science, Carleton University (to KLC). The MIREC Study was funded by the Chemicals Management Plan of Health Canada, with funding from the Canadian Institutes of Health Research (grant # MOP–81285) and the Ontario Ministry of the Environment. We thank the mothers and children of the MIREC study, the health care teams, Nicole Lupien and Romy-Leigh McMaster, Centre de Recherche, CHU Sainte-Justine, for their administrative assistance associated with this study, the MIREC study group of investigators and site investigators for their contributions to this important cohort and data, and Dr Paul Villeneuve for his contributions to this manuscript.
Contributions
Conceptualisation: KLC, TEA, ZW; Data analysis and interpretation: ZW, ID, LC, MW, KLC, AJM, PV, TEA; Drafting of manuscript: ZW, ID, LC, KLC; Revision of manuscript: KLC, TEA WDF, ZW, MW, AJM.
Conflicts of interest
The authors have no conflicts of interest and nothing to disclose.
Ethical standards
The authors assert that all procedures contributing to this work comply with the ethical standards of the Tri-Council Policy Statement: Ethical Conduct for Research Involving Humans – TCPS 2 (2018) and with the Helsinki Declaration of 1975, as revised in 2008. This study received ethics approval from the Carleton University Research Ethics Board (#106172) and the Health Canada Research Ethics Board (#2016-028H).