We consider the following linear regression model:

where
are independent and identically distributed random variables, Yi, is real, Zi has values in Rm, Ui, is independent of Zi, and θ0 is an m-dimensional parameter to be estimated. The Lp estimator of θ0 is the value 6n such that

Here, we will give the exact Bahadur-Kiefer representation of θn, for each p ≥ 1. Explicitly, we will see that, under regularity conditions,
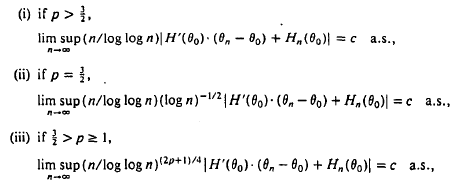
where 
and c is a positive constant, which depends on p and on the random variable X.