No CrossRef data available.
Article contents
THE ET INTERVIEW: BENEDIKT M. PÖTSCHER
Published online by Cambridge University Press: 18 September 2024
Abstract
An abstract is not available for this content so a preview has been provided. Please use the Get access link above for information on how to access this content.
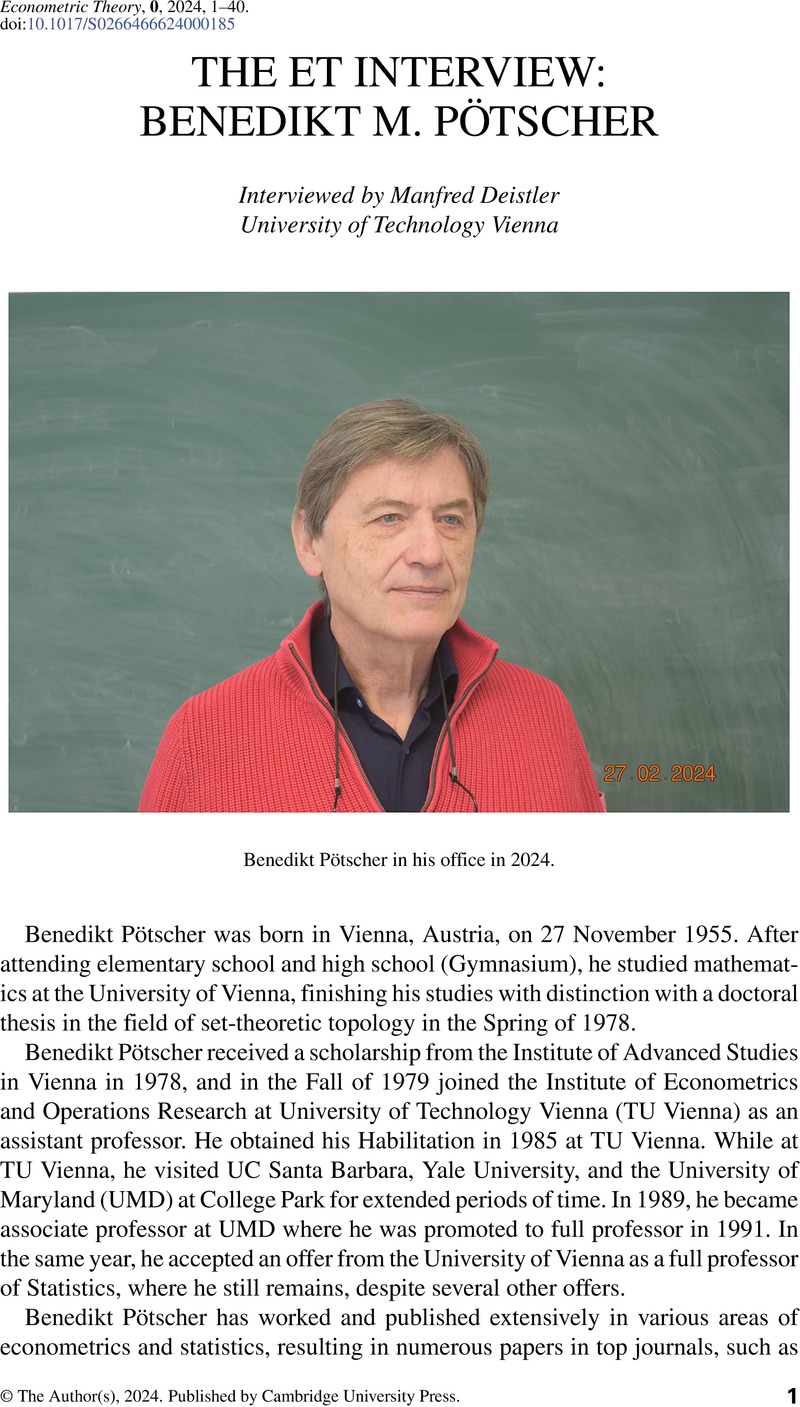
- Type
- ET INTERVIEW
- Information
- Copyright
- © The Author(s), 2024. Published by Cambridge University Press
References
REFERENCES
Andrews, D. W. K. (1987). Consistency in nonlinear econometric models: A generic uniform law of large numbers.
Econometrica
, 55, 1465–1471.Google Scholar
Andrews, D. W. K. (1992). Generic uniform convergence.
Econometric Theory
, 8, 241–257.Google Scholar
Andrews, D. W. K., & Guggenberger, P. (2009). Hybrid and size-corrected subsampling methods.
Econometrica
, 77, 721–762.Google Scholar
Andrews, D. W. K., & Ploberger, W. (1996). Testing for serial correlation against an ARMA
$\left(1,1\right)$
process. Journal of the American Statistical Association, 91, 1331–1342.Google Scholar

Bachoc, F., Leeb, H., & Pötscher, B. M. (2019). Valid confidence intervals for post-model-selection predictors.
Annals of Statistics
, 47, 1475–1504.Google Scholar
Bates, C. E., & White, H. (1985). A unified theory of consistent estimation for parametric models.
Econometric Theory
, 1, 151–178.Google Scholar
Bauer, P., Pötscher, B. M., & Hackl, P. (1988). Model selection by multiple test procedures.
Statistics
, 19, 39–44.Google Scholar
Berk, R., Brown, L., Buja, A., Zhang, K., & Zhao, L. (2013). Valid post-selection inference.
Annals of Statistics
, 41, 802–837.Google Scholar
Birman, M., & Solomjak, M. Z. (1967). Piecewise polynomial approximations of functions of classes
$W^{\alpha}_{p} $
.
Matematicheskii Sbornik
, 73(115), 331–355.Google Scholar

Blough, S. R. (1988). On the impossibility of testing for unit roots and cointegration in finite samples. Working Paper. Johns Hopkins University.Google Scholar
Bomze, I. M., & Pötscher, B. M. (1989).
Game theoretical foundations of evolutionary stability
. Lecture Notes in Economics and Mathematical Systems, 324. Springer-Verlag.Google Scholar
Campbell, J. Y., & Mankiw, N. G. (1987a). Are output fluctuations transitory?
Quarterly Journal of Economics
, 102, 857–880.Google Scholar
Campbell, J. Y., & Mankiw, N. G. (1987b). Permanent and transitory components in macroeconomic fluctuations.
American Economic Review Papers and Proceeedings
, 77, 111–117.Google Scholar
Christiano, L. J., & Eichenbaum, M. (1990). Unit roots in real GNP: Do we know and do we care?
Carnegie-Rochester Conference Series on Public Policy
, 32, 7–62.Google Scholar
Cochrane, J. H. (1988). How big is the random walk in GNP?
Journal of Political Economy
, 96, 893–920.Google Scholar
Dahlhaus, R., & Pötscher, B. M. (1989). Convergence results for maximum likelihood type estimators in multivariable ARMA models II.
Journal of Multivariate Analysis
, 30, 241–244.Google Scholar
Deistler, M., Dunsmuir, W., & Hannan, E. J. (1978). Vector linear time series models: Corrections and extensions.
Advances in Applied Probability
, 10, 360–372.Google Scholar
Deistler, M., & Pötscher, B. M. (1984). The behaviour of the likelihood function for ARMA models.
Advances in Applied Probability
, 16, 843–866.Google Scholar
Diebold, F., & Rudebusch, G. (1989). Long memory and persistence in aggregate output.
Journal of Monetary Economics
, 24, 189–209.Google Scholar
Domowitz, I., & White, H. (1982). Misspecified models with dependent observations.
Journal of Econometrics
, 20, 35–58.Google Scholar
Dunsmuir, W., & Hannan, E. J. (1976). Vector linear time series models.
Advances in Applied Probability
, 8, 339–364.Google Scholar
Ensor, K. B., & Newton, H. J. (1988). The effect of order estimation on estimating the peak frequency of an autoregressive spectral density.
Biometrika
, 75, 587–589.Google Scholar
Fan, J., & Li, R. (2001). Variable selection via nonconcave penalized likelihood and its oracle properties.
Journal of the American Statistical Association
, 96, 1348–1360.Google Scholar
Faust, J. (1996). Near observational equivalence and theoretical size problems with unit root tests.
Econometric Theory
, 12, 724–731.Google Scholar
Findley, D. F., Pötscher, B. M., & Wei, C.-Z. (2001). Uniform convergence of sample second moments of families of time series arrays.
Annals of Statistics
, 29, 815–838.Google Scholar
Findley, D. F., Pötscher, B. M., & Wei, C.-Z. (2004). Modeling of time series arrays by multistep prediction or likelihood methods.
Journal of Econometrics
, 118, 151–187.Google Scholar
Gach, F., & Pötscher, B. M. (2011). Nonparametric maximum likelihood density estimation and simulation-based minimum distance estimators.
Mathematical Methods of Statistics
, 20, 288–326.Google Scholar
Hannan, E. J. (1982). Testing for autocorrelation and Akaike’s criterion.
Journal of Applied Probability
, 19, 403–412.Google Scholar
Hannan, E. J., & Quinn, B. G. (1979). The determination of the order of an autoregression.
Journal of the Royal Statistical Society, Series B
, 41, 190–195.Google Scholar
Hauser, M., Pötscher, B. M., & Reschenhofer, E. (1999). Measuring persistence in aggregate output: ARMA models, fractionally integrated ARMA models and nonparametric procedures.
Empirical Economics
, 24, 243–269.Google Scholar
Hoadley, B. (1971). Asymptotic properties of maximum likelihood estimators for the independent not identically distributed case.
Annals of Mathematical Statistics
, 42, 1977–1991.Google Scholar
Kabaila, P. (1983). Parameter values of ARMA models minimising the one-step-ahead prediction error when the true system is not in the model set.
Journal of Applied Probability
, 20, 405–408.Google Scholar
Kimura, M. (1982).
Molecular evolution, protein polymorphism and the neutral theory
. Springer-Verlag.Google Scholar
Kolmogorov, A. N., & Tihomirov, V. M. (1961).
$\varepsilon$
-entropy and
$\varepsilon$
-capacity of sets in functional spaces.
American Mathematical Society Translations
, 17, 277–364.Google Scholar


Leeb, H., & Pötscher, B. M. (2003). The finite-sample distribution of post-model-selection estimators, and uniform versus non-uniform approximations.
Econometric Theory
, 19, 100–142.Google Scholar
Leeb, H., & Pötscher, B. M. (2005). Model selection and inference: Facts and fiction.
Econometric Theory
, 21, 21–59.Google Scholar
Leeb, H., & Pötscher, B. M. (2006a). Can one estimate the conditional distribution of post-model-selection estimators?
Annals of Statistics
, 34, 2554–2591.Google Scholar
Leeb, H., & Pötscher, B. M. (2006b). Performance limits for estimators of the risk or distribution of shrinkage-type estimators, and some general lower risk-bound results.
Econometric Theory
, 22, 69–97. (Correction, ibid., 24, 581–583).Google Scholar
Leeb, H., & Pötscher, B. M. (2008a). Can one estimate the unconditional distribution of post-model-selection estimators?
Econometric Theory
, 24, 338–376.Google Scholar
Leeb, H., & Pötscher, B. M. (2008b). Sparse estimators and the oracle property, or the return of Hodges’ estimator.
Journal of Econometrics
, 142, 201–211.Google Scholar
Leeb, H., & Pötscher, B. M. (2017). Testing in the presence of nuisance parameters: Some comments on tests post-model-selection and random critical values. In Ahmed, S. (Eds.),
Big and complex data analysis
(pp. 69–82). Contributions to Statistics. Springer.Google Scholar
Leeb, H., Pötscher, B. M., & Ewald, K. (2015). On various confidence intervals post-model-selection.
Statistical Science
, 30, 216–227.Google Scholar
Manski, C. F. (1984). Adaptive estimation of nonlinear regression models.
Econometric Reviews
, 3, 145–210.Google Scholar
Nelson, C. R., & Plosser, C. I. (1982). Trends and random walks in macroeconomic time series: Some evidence and implications.
Journal of Monetary Economics
, 10, 139–162.Google Scholar
Newey, W. K. (1991). Uniform convergence in probability and stochastic equicontinuity.
Econometrica
, 59, 1161–1167.Google Scholar
Nickl, R. (2003). Asymptotic distribution theory of post-model-selection maximum likelihood estimators. Master’s thesis, University of Vienna.Google Scholar
Nickl, R. (2007). Donsker-type theorems for nonparametric maximum likelihood estimators.
Probability Theory and Related Fields
, 138, 411–449. (Erratum, ibid., 141, 331–332).Google Scholar
Nickl, R., & Pötscher, B. M. (2007). Bracketing metric entropy rates and empirical central limit theorems for function classes of Besov- and Sobolev-type.
Journal of Theoretical Probability
, 20, 177–199.Google Scholar
Nickl, R., & Pötscher, B. M. (2010). Efficient simulation-based minimum distance estimation and indirect inference.
Mathematical Methods of Statistics
, 19, 327–364.Google Scholar
Perron, P., & Ren, L. (2011). On the irrelevance of impossibility theorems: The case of the long-run variance. Journal of Time Series Econometrics, 3, 3(3).Google Scholar
Pötscher, B. M. (1982). Some results on
${\omega}_{\mu }$
-metric spaces. Annales Universitatis Scientiarum Budapestinensis de Rolando Eötvös Nominatae. Sectio Mathematica, 25, 3–18. (Correction, ibid., 28, 283).Google Scholar

Pötscher, B. M. (1983). Order estimation in ARMA-models by Lagrangian multiplier tests.
Annals of Statistics
, 11, 872–885. (Correction, ibid., 12, 785).Google Scholar
Pötscher, B. M. (1985a). The behaviour of the Lagrangian multiplier test in testing the orders of an ARMA-model.
Metrika
, 32, 129–150.Google Scholar
Pötscher, B. M. (1985b). Moments and order statistics of extinction times in multitype branching processes and their relation to random selection models.
Bulletin of Mathematical Biology
, 47, 263–272.Google Scholar
Pötscher, B. M. (1987a). Convergence results for maximum likelihood type estimators in multivariable ARMA models.
Journal of Multivariate Analysis
, 21, 29–52.Google Scholar
Pötscher, B. M. (1987b). A generalization of Urysohn’s metrization theorem and its set-theoretic consequences.
Studia Scientiarum Mathematicarum Hungarica
, 22, 457–461.Google Scholar
Pötscher, B. M. (1989). Model selection under nonstationarity: Autoregressive models and stochastic linear regression models.
Annals of Statistics
, 17, 1257–1274.Google Scholar
Pötscher, B. M. (1990). Estimation of autoregressive moving-average order given an infinite number of models and approximation of spectral densities.
Journal of Time Series Analysis
, 11, 165–179.Google Scholar
Pötscher, B. M. (1991a). Effects of model selection on inference.
Econometric Theory
, 7, 163–185.Google Scholar
Pötscher, B. M. (1991b). Noninvertibility and pseudo-maximum likelihood estimation of misspecified ARMA models.
Econometric Theory
, 7, 435–449. (Corrigendum, ibid., 10, 811).Google Scholar
Pötscher, B. M. (2002). Lower risk bounds and properties of confidence sets for ill-posed estimation problems with applications to spectral density and persistence estimation, unit roots, and estimation of long memory parameters.
Econometrica
, 70, 1035–1065.Google Scholar
Pötscher, B. M. (2006). The distribution of model averaging estimators and an impossibility result regarding its estimation.
IMS Lecture Notes - Monograph Series
, 52, 113–129.Google Scholar
Pötscher, B. M. (2009). Confidence sets based on sparse estimators are necessarily large. Sankhya, 71-A, 1–18.Google Scholar
Pötscher, B. M., & Leeb, H. (2009). On the distribution of penalized maximum likelihood estimators: The LASSO, SCAD, and thresholding.
Journal of Multivariate Analysis
, 100, 2065–2082.Google Scholar
Pötscher, B. M., & Preinerstorfer, D. (2018). Controlling the size of autocorrelation robust tests.
Journal of Econometrics
, 207, 406–431.Google Scholar
Pötscher, B. M., & Preinerstorfer, D. (2019). Further results on size and power of heteroskedasticity and autocorrelation robust tests, with an application to trend testing.
Electronic Journal of Statistics
, 13, 3893–3942.Google Scholar
Pötscher, B. M., & Preinerstorfer, D. (2021). Valid heteroskedasticity robust testing. Econometric Theory. Published online by Cambridge University Press: 11 September 2023, 1–53.Google Scholar
Pötscher, B. M., & Preinerstorfer, D. (2023). How reliable are bootstrap-based heteroskedasticity robust tests?
Econometric Theory
, 39, 789–847.Google Scholar
Pötscher, B. M., & Prucha, I. R. (1986a). A class of partially adaptive one-step
$M$
-estimators for the nonlinear regression model with dependent observations.
Journal of Econometrics
, 32, 219–251.Google Scholar

Pötscher, B. M., & Prucha, I. R. (1986b). Consistency in nonlinear econometrics: A generic uniform law of large numbers and some comments on recent results. Working Paper No 86-9. Department of Economics, University of Maryland.Google Scholar
Pötscher, B. M., & Prucha, I. R. (1989). A uniform law of large numbers for dependent and heterogeneous data processes.
Econometrica
, 57, 675–683.Google Scholar
Pötscher, B. M., & Prucha, I. R. (1991a). Basic structure of the asymptotic theory in dynamic nonlinear econometric models. I. Consistency and approximation concepts.
Econometric Reviews
, 10, 125–216.Google Scholar
Pötscher, B. M., & Prucha, I. R. (1991b). Basic structure of the asymptotic theory in dynamic nonlinear econometric models II. Asymptotic normality..
Econometric Reviews
, 10, 253–357.Google Scholar
Pötscher, B. M., & Prucha, I. R. (1994a). Generic uniform convergence and equicontinuity concepts for random functions: An exploration of the basic structure.
Journal of Econometrics
, 60, 23–63.Google Scholar
Pötscher, B. M., & Prucha, I. R. (1994b). On the formulation of uniform laws of large numbers: A truncation approach.
Statistics
, 25, 343–360.Google Scholar
Pötscher, B. M., & Prucha, I. R. (1997).
Dynamic nonlinear econometric models: Asymptotic theory
. Springer-Verlag.Google Scholar
Pötscher, B. M., & Schneider, U. (2009). On the distribution of the adaptive LASSO estimator.
Journal of Statistical Planning and Inference
, 139, 2775–2790.Google Scholar
Pötscher, B. M., & Schneider, U. (2010). Confidence sets based on penalized maximum likelihood estimators in Gaussian regression.
Electronic Journal of Statistics
, 4, 334–360.Google Scholar
Pötscher, B. M., & Schneider, U. (2011). Distributional results for thresholding estimators in high-dimensional Gaussian regression models.
Electronic Journal of Statistics
, 5, 1876–1934.Google Scholar
Pötscher, B. M., & Srinivasan, S. (1994). A comparison of order estimation procedures for ARMA models.
Statistica Sinica
, 4, 29–50.Google Scholar
Preinerstorfer, D. (2017). Finite sample properties of tests based on prewhitened nonparametric covariance estimators.
Electronic Journal of Statistics
, 11, 2097–2167.Google Scholar
Preinerstorfer, D., & Pötscher, B. M. (2016). On size and power of heteroskedasticity and autocorrelation robust tests.
Econometric Theory
, 32, 261–358.Google Scholar
Preinerstorfer, D., & Pötscher, B. M. (2017). On the power of invariant tests for hypotheses on a covariance matrix.
Econometric Theory
, 33, 1–68.Google Scholar
Prucha, I. R., & Kelejian, H. H. (1984). The structure of simultaneous equation estimators: A generalization towcards nonnormal disturbances.
Econometrica
, 52, 721–736.Google Scholar
Schuster, P., & Sigmund, K. (1984). Random selection – a simple model based on linear birth and death processes.
Bulletin of Mathematical Biology
, 46, 11–17.Google Scholar
Sen, P. K. (1979). Asymptotic properties of maximum likelihood estimators based on conditional specification.
Annals of Statistics
, 7, 1019–1033.Google Scholar
Sewastjanow, B. A. (1974). Verzweigungsprozesse
, vol. 34 of
Mathematische Lehrbücher und Monographien, II. Abteilung: Mathematische Monographien
. Akademie-Verlag,
Berlin.Google Scholar
Tanaka, K., & Satchell, S. E. (1989). Asymptotic properties of the maximum-likelihood and nonlinear least-squares estimators for noninvertible moving average models.
Econometric Theory
, 5, 333–353.Google Scholar
White, H. (1980). Nonlinear regression on cross-section data.
Econometrica
, 48, 721–746.Google Scholar
White, H., & Domowitz, I. (1984). Nonlinear regression with dependent observations.
Econometrica
, 52, 143–161.Google Scholar
Willard, S. (1970). General topology. Addison-Wesley Publishing Co., Reading, Mass.-London-Don Mills, Ont.Google Scholar