Policy Significance Statement
Achieving effective accountability of artificial intelligence depends on a clear, concrete, and specific understanding of what is meant by concepts such as “transparency,” “interpretability,” “explainability,” and “accountability.” This article provides precisely such an understanding and outlines what each concept requires in order to move from being a high-level aspiration to a concrete and enforceable requirement. In particular, the article argues that it is “answerability”; the ability to call an entity to account which provides the impetus for each of the other concepts and renders them enforceable in a variety of different contexts.
1. Introduction
The dangers associated with autonomous or algorithmic decision-making systems (ADMs) are well known (O’Neill, Reference O’Neill2016). They can be opaque or invisible (Mittelstadt et al., Reference Mittelstadt, Allo, Taddeo, Wachter and Floridi2016; Pasquale, Reference Pasquale2015), they often contain no incentives to check accuracy and often rely on correlation rather than causation (Mayer-Schönberg and Cukier, Reference Mayer-Schönberg and Cukier2013), they “scale” any existing flaws in a decision-making process (AI Now Report, 2018); they can create pernicious feedback loops (Schermer, Reference Schermer2011; O’Neill, Reference O’Neill2016) in which the system causes precisely the negative effects it is designed to detect, and they can encourage deindividualization and rigidity of decision-making. All of this in combination can lead to an exacerbation and entrenchment of any existing disparities or injustices arising from the information asymmetry between the subject of the ADM system and those operating it (UKAI, 2018).
And yet conversely, the benefits of increased automation and connectivity, and in particular machine learning and other ADM techniques, are equally evident. From their use in medicine (Marchant, Reference Marchant2020; McKinney et al., Reference McKinney, Sieniek, Godbole, Godwin, Antropova, Ashrafian, Back, Chesus, Corrado, Darzi, Etemadi, Garcia-Vicente, Gilbert, Halling-Brown, Hassabis, Jansen, Karthikesalingam, Kelly, King, Ledsam, Melnick, Mostofi, Peng, Reicher, Romera-Paredes, Sidebottom, Suleyman, Tse, Young, De Fauw and Shetty2020), including the COVID-19 pandemic (Blumenstock, Reference Blumenstock2020; Weforum, 2020) to the Internet of Things (IoT) and autonomous vehicles (AVs), such systems are being deployed in ever wider contexts as a result of their greater efficiency and capacity to outperform human actors.
Inevitably, therefore, attention has turned to the optimisation of such systems and the ways in which their benefits can be harnessed at the same time as mitigating and minimizing their capacity for harm. As a result, a variety of governmental and nongovernmental organizations have written guidelines (HCSTC, 2018) to encourage a series of aspirational concepts such as transparency (HiLEG, 2019) interpretability (Royal Society, 2017; UKAI, 2018) explainability (EP, 2019; ICO and AT, 2020), and accountability (HCSTC, 2018; EP, 2019) Each of these concepts is also connected in different documents to the General Data Protection Regulation (GDPR 2016) requirement contained in Articles 13, 14, 15, and 22 to give data subjects “meaningful information about the logic involved” (EAD1e, 2019; EP, 2019; ICO and AT, 2020) in decisions taken about them.
The idea behind these concepts appears to be that if harm is caused by an automated system, compliance with these concepts will enable us to find out what has gone wrong with the system (or indeed the operation of it) and who should be responsible or answerable for any harms caused by such failures. If this is right, and we are able to do this successfully, it has the potential to bring a variety of benefits. If we know what has gone wrong in the past this will enable us to take steps to reduce the chances of the same problem arising again in the future. And indeed, the very existence of accountability may provide an incentive for any human agents involved with the system to reduce the chances of those problems arising in the first place. If, through these or other routes we are therefore able to reduce the harms caused by such systems and their designers and users, this in turn may mean the systems can be deployed with a greater degree of trust on the part of those who interact with them, enabling the systems to be used in more effective ways.
The difficulty at the moment, however, is that the concepts exist at a fairly abstract, or aspirational level, whereas in order for them to have the tangible effects described above they themselves also need to be more concrete, specific and enforceable (New and Castro, Reference New and Castro2018). For example, the Montreal Declaration states that its principles “are like points on a moral compass,” or an “ethical framework” (Montreal, 2018). They are phrased as imperatives (e.g., “AIS must be developed and used while respecting people’s autonomy”) (Montreal, 2018) but like many of the other documents and publications discussed here, the declaration (a) contains no more specific definition of terms such as “autonomy” and (b) contains no mechanism for rendering such principles enforceable. The aim of this article is therefore to undertake this process of concretisation and enforceability. We will begin by mapping how these, and other different concepts interrelate and what in particular they each require in order to enable them to move from being abstract, high-level aspirations to detailed and enforceable requirements applicable both to the automated systems themselves and to those who commission, design, build/implement, oversee, and operate them. We will then argue that if all the other concepts are to be useful or effective, the key concept which will help to achieve that, as well as other benefits, is accountability. Unless someone or something can be rendered accountable for failing to be transparent, interpretable, or reviewable, it is difficult to see how those concepts can really take effect. It is also the case, as Kacianka and Pretschner (Reference Kacianka and Pretschner2021) point out, that we cannot design systems to be accountable unless we know precisely what that means. Conversely, all other concepts may well in turn be shaped by what is ultimately required for and enforceable through accountability. In the second half of the article we will therefore examine the concept of accountability in more detail. The difficulty here is that either the term is so general and undifferentiated that it risks becoming useless in practical terms, or, conversely, there have been so many attempts to provide it with a more specific taxonomy that the range of choices is equally unhelpful in practice. We will therefore attempt to marry together these various taxonomies, building on the idea of Bovens et al. (Reference Bovens, Goodin and Schillemans2014) that we should answer a series of questions about accountability. We will therefore ask in turn who is accountable? To whom? For what? By which standards? Why (in the sense of the purpose fulfilled by the accountability)? And how (or in other words, by what mechanism)? Once we are able to answer these questions, this in turn will inform what precisely is required in terms of the other concepts such as transparency or explainability in a particular instance, and the supporting sociotechnical infrastructure needed to realize this.
2. Mapping the Concepts
In order to begin this process of concretisation and enforcement it is important that we have a more detailed, specific and practical understanding of what, precisely each of the high-level concepts entails and thus what they each require of the systems and people to which they apply, as well as their relationship to the relevant legal regulation of the area. Inevitably there is a high degree of overlap and interrelation between the principles, and very often the same ideas are covered in different policy documents by different terms (UKAI, 2018; Itechlaw 2019). Nuñez and Fernandez-Gago refer to this as synonymy. This, for them, is one aspect of ambiguity, the converse aspect of which is homonymy, where the same name is used to designate different properties. In addition to these problems, Nuñez and Fernandez-Gago (Reference Nuñez and Fernandez-Gago2013) also list “level of abstraction” and “subjectivity” (in the sense of context or discipline specificity) as further barriers to implementation and evaluation of the concepts which we need to overcome in order to render the concepts useful.
It is obviously not possible to consider every single document where these concepts are mentioned, but in the discussion below we have attempted to draw on as wide and as comprehensive a range as possible of declarations and policy statements issued by governmental and nongovernmental organizations both nationally and internationally.
It should be noted that this is of course not the first attempt to map the different concepts (Floridi et al., Reference Floridi, Cowls, Beltrametti, Chatila, Chazerand, Dignum, Luetge, Madelin, Pagallo, Rossi, Schafer, Valcke and Vayena2018; EAD1e, 2019), but in building on prior work in this area we attempt to synthesize the multiple references to such concepts in order to present a core, more specific definition of each. We also propose the addition of two variables or dimensions through which the concepts might be viewed; chronology and activity. By the latter, we mean the distinction between concepts which tend to assume an obligation to provide information on the part of the system’s operatorsFootnote 1 (push), as opposed to information that those seeking to understand or even challenge the use of a system will require and thus seek actively (pull). By chronology we mean the point in the process at which the concept arises. Is it something which is inherent in the system from the start, or is it something that arises as an issue later on in the process such as, for example, when the use of the system is challenged (Bryson and Winfield, Reference Bryson and Winfield2017).Footnote 2 We do not suggest that these are the only potential dimensions or variables that might be considered, simply that they can assist in understanding how the different concepts fit or connect together and the extent to which they are concepts that must be sought (pulled) as opposed to provided (pushed).
2.1. Transparency
Perhaps the most basic concept with which to begin this process is that of transparency. This concept is of course not exclusive to the ADM context,Footnote 3 but rather is a central principle in all democratic polities (Birkinshaw, Reference Birkinshaw2005; Birkinshaw, Reference Birkinshaw2010; Curtin and Mendes, Reference Curtin and Mendes2011; Craig, Reference Craig2012), encompassing a variety of features such as the holding of meetings in public, the provision of information, and the right of access to documents. As a result, for example, it is referred to in several Articles of the EU’s Treaty of Lisbon (Lisbon, 2007).
It is therefore perhaps unsurprising that in the more specific context of ADM Recital 39 of the (GDPR) states that:
The principle of transparency requires that any information and communication relating to the processing of… personal data be easily accessible and easy to understand, and that clear and plain language be used. That principle concerns, in particular, information to the data subjects on the identity of the controller and the purposes of the processing and further information to ensure fair and transparent processing in respect of the natural persons concerned and their right to obtain confirmation and communication of personal data concerning them which are being processed.
This means that the information in question should be “easily accessible” (DPWP, 2017) “free of charge” (DPWP, 2017) and provided “in a timely manner (DPWP, 2017).” Under the Montreal Declaration (MDec) for a responsible development of artificial intelligence this includes access to the source code (Montreal, 2018) while for the European Parliament (EP, 2019) this means “not only transparency of code, but also of data and automated decision-making.” However, it may well be, as Kroll et al. (Reference Kroll, Barocas, Felten, Reidenberg, Robinson and Yu2017) point out, that “Disclosure of source code is often neither necessary (because of alternative techniques from computer science, such as reverse engineering) nor sufficient (given the difficulties in some instances of analyzing that code) to demonstrate the fairness of a process.” The A4Cloud project (Cattedu et al., Reference Cattedu, Felici, Hogben, Holcroft, Kosta, Llenes, Millard, Niezen and Nu2013; Felici and Pearson, Reference Felici and Pearson2013) similarly refers to the “visibility” of a system’s governing norms, behavior and compliance of behavior to the norms. For the US Department of Defence (DoD), transparency is about openness and collaboration “to reduce the chance of misperception, miscalculation or accidents” (DoD, 2020). Similarly for the Royal Society (2017), transparency is about openness and reproducibility of research, which chimes with the fourth of the “Asilomar Principles,” “a culture of cooperation, trust and transparency should be fostered among researchers and developers of AI” (Future of Life Institute, 2017; EP, 2019). For the House of Lords Select Committee on Artificial Intelligence, transparency is a technical concept, indeed one they refer to as “technical transparency” (UKAI, 2018) which can either arise ex ante, before the system is deployed, or ex post in the sense that the performance of the system can be tested. For that committee, transparency also represents the antithesis of trying to hide anything about the system, for example, trying to conceal from consumers the fact that they are interacting with a chatbot rather than a human being (however unlikely this might in fact be in practice, Guardian, 2018), or concealing the use of price discrimination. This in turn aligns with the EP’s definition, above, with the Information Commissioner’s Office and Alan Turing Institute’s guidance which refers to transparency as “being clear, open, and honest with people” (ICO and AT, 2020), as well as with ITECHLAW’s definition of transparency as:
an obligation for organisations that use AI in decision-making processes to provide information regarding (a) the fact that an organisation is using an AI system in a decision-making process; (b) the intended purpose(s) of the AI system and how the AI system will and can be used; (c) the types of data sets that are used by the AI system; and (d) meaningful information about the logic involved (Itechlaw, 2019).
Inclusion of this last requirement obviously derives directly from the text of Articles 13(2)(f), 14(2)(g), and 15(1)(h) of the GDPR, and its inclusion here is interesting since it suggests an interpretation of those articles which is in line with that suggested by Wachter et al. (Reference Wachter, Mittelstadt and Floridi2017), focusing specifically on information regarding the technical setup of the system, rather than any individualized explanation of the system’s behavior in any particular case. This seems likely also to be the meaning of transparency in its position as the IEEE’s fifth General Principle for Ethically Aligned Design of AI: “the basis of a particular Autonomous or Intelligent System decision should always be discoverable” (EAD1e, 2019).
Two other subsidiary components or facets of transparency are traceability and observability. Nuñez and Fernandez-Gago identify traceability as a term derived from logistics and supply chain management, used to “describe the ability to trace information related to goods during their production… the ability to track the complete set of operations that were performed” (Nuñez and Fernandez-Gago, Reference Nuñez and Fernandez-Gago2013). Similarly, the European Commission’s Independent High Level Expert Group on Artificial Intelligence define traceability as “the capability to keep track of the system’s data, development and deployment processes, typically by means of documented recorded identification” (HiLEG, 2019). Observability, on the other hand, is perhaps an example of the kind of synonymy Nuñez and Fernandez-Gago identify. They define it as “a property of an object, process or system that describes how well the internal actions of the system can be described by observing the external outputs of the system” (Nuñez and Fernandez-Gago, Reference Nuñez and Fernandez-Gago2013). And on that basis it would fit here within transparency on the basis that the sources listed above appear to conceive of transparency and its subsidiary facets as forms of openness. In other words, taking all these sources together, transparency appears to refer to the simple need for there to be readily available relevant information about the existence of ADM as well as further details about its operation such as its code and its use of data. Chronologically, therefore, in terms of fitting the concepts together, transparency is a basic starting point from which the other concepts below might derive, and where applicable,Footnote 4 it is a relatively neutral or objective requirement that the information be passively available ab initio (pushed) without the need for it to be sought specifically by those trying to understand or challenge the system (pulled).
2.2. Intelligibility/interpretability
However, as Kacianka and Pretschner (Reference Kacianka and Pretschner2021) point out, citing Ananny and Crawford (Reference Ananny and Crawford2018), while transparency has in the past been seen as a solution, transparency alone is not enough unless someone can understand the output of such a transparency mechanism. Very closely linked to the concept of transparency, therefore, is that of intelligibility or interpretability (EAD1e, 2019). Different terms are used by different sources, and some sources use them interchangeably, but both terms appear at their most basic level to refer to the ability to understand the information provided as a matter of transparency. For the UKAI report, for example, “intelligibility” refers to “the broader issue” of “making AI understandable” (UKAI, 2018), and similarly the IEEE refers to the need for humans to understand the system and the necessity for this to occur “at a level of ordinary human reasoning, not with incomprehensible technical detail” (Doshi-Velez and Kim, Reference Doshi-Velez and Kim2017; EAD1e, 2019). However, there is also a more technical dimension to the term “interpretability” which refers to the ability to understand cause and effect within the particular ADM model chosen, in the sense of understanding how the intrinsic logic of the system relates to the results it produces. On this basis the most interpretable systems are linear systems (in which any change in the value of the predictor variable results directly in a change in the value of the response variable at a constant rate), monotonic systems (in which the value of the response changes consistently in either the same or the opposite direction as the predictor value) and sparse or noncomplex systems in which the number of features (dimensionality) and interactions between them and the underlying distribution model is simple enough for them to be clearly understood (ICO and AT, 2020).
Chronologically, then, intelligibility or interpretability thus appears to build on the concept of transparency but again it is a relatively neutral or passive (push) requirement that the information provided through transparency must be understandable, both in a lay and more technical sense.
2.3. Explainability
This understandability, building on transparency then provides one of the key elements of explainability. Indeed, explainability is the concept which perhaps overlaps most with the other concepts. In the UKAI report, for example, explainability largely overlaps with the technical understanding of interpretability outlined above (Guidotti et al., Reference Guidotti, Monreale, Ruggieri, Turini, Pedreschi and Giannotti2018; UKAI, 2018; Rudin, Reference Rudin2019; Marcinkevičs and Vogt, Reference Marcinkevičs and Vogt2020), as it does in discussions of explainability from the technical literature (Bhatt et al., Reference Bhatt, Xiang, Sharma, Weller, Taly, Jia, Ghosh, Puri, JMF and Eckersley2020), and in the FATML Principles 2019 (Diakopoulos et al., Reference Diakopoulos, Friedler, Arenas, Barocas, Hay, Howe, Jagadish, Unsworth, Sahuguet, Venkatasubramanian, Wilson, Yu and Zevenbergen2019). But the UKAI report also recommended the establishment of the ICO and AT guidance on explaining decisions made with AI. And this guidance not only contains probably the most developed account of what might be meant by explainability, but also gives it a much wider reach (ICO and AT, 2020).
ICO&AT divide explanation into two subcategories; process-based, which explains how the system is designed, deployed and governed, and outcome-based, which deals with what happened in the case of a particular decision. These two subcategories then cut across the six explanation types they identify, which are as follows:
-
1. Rationale explanation: “the ‘why?’ of an AI decision. It helps people understand the reasons that led to a decision outcome, in an accessible way.”
-
2. Responsibility explanation which “helps people understand ‘who’ is involved in the development and management of the AI model, and ‘who’ to contact for a human review of a decision.”
-
3. Data explanation: “the ‘what’ of AI-assisted decisions. They help people understand what data about them, and what other sources of data were used in a particular AI decision.”
-
4. Fairness explanation: “helping people understand the steps you took (and continue to take) to ensure your AI decisions are generally unbiased and equitable.”
-
5. Safety and performance explanation, which “helps people understand the measures you have put in place and the steps you have taken (and continue to take) to maximize the accuracy, reliability, security and robustness of the decisions your AI model helps you to make.”
-
6. Impact explanation, which “helps people understand how you have considered the effects that your AI decision-support system may have on an individual, that is, what the outcome of the decision means for them. It is also about helping individuals to understand the broader societal effects that the use of your system may have” (ICO and AT, 2020).
The focus of these requirements may primarily be systems which do make decisions about people, whether these involve private sector decisions about recruitment or credit, or public sector decisions about benefits, immigration, and so forth. However, there is no reason in principle why the concept of “decision” should not also apply to the decision of an AV to apply the brake or take some other action, for example.
The guidance also stresses that each of these forms of explanation should be interpreted in a context-specific manner, so that the precise content of the explanation will depend on a series of factors including the domain of deployment, the impact of the ADM decision and the audience receiving the explanation. This fits with the similarly more holistic approach of HiLEG (2019) which states that explainability:
concerns the ability to explain both the technical processes of an AI system and the related human decisions (e.g. application areas of a system). Technical explainability requires that the decisions made by an AI system can be understood and traced by human beings… Such explanation should be timely and adapted to the expertise of the stakeholder concerned…In addition, explanations of the degree to which an AI system influences and shapes the organisational decision-making process, design choices of the system, and the rationale for deploying it, should be available.
This also fits perfectly with the view of HiLEG that “The degree to which explicability is needed is highly dependent on the context and the severity of the consequences if that output is erroneous or otherwise inaccurate” (HiLEG, 2019), and the view of Rudin (Reference Rudin2019) and Marcinkevičs and Vogt (Reference Marcinkevičs and Vogt2020) that while interpretability relates to an inherent ability, explainability refers to post hoc explanations, particularly for black box models.
Explainability, or perhaps more accurately explanation, therefore requires the system operator positively to select and make use of the transparent and understandable information discussed earlier in order to give an account or a report of the system’s use to someone in particular. Chronologically, therefore, explainability builds on transparency and intelligibility/interpretability, but it also entails the conveyance of that information by the system operator, in a meaningful form to a specific audience. As a concept it thus seems to sit exactly between passivity and activity, involving both push and pull. On the one hand it is clear from the ICO&AT work that the explanation is something that should be given, but it is also likely that sometimes more specific explanations will be actively sought and explainability requires that an adequate response be given. When this happens explainability entails and necessitates the concepts outlined in the next two sections.
2.4. Traceability
Closely connected to the idea of explainability is that of “traceability” (Kroll, Reference Kroll2021). This “refers broadly to the idea that the outputs of a computer system can be understood through the process by which that system was designed and developed,” and includes “ensuring the existence and legibility of records… and system documentation.” It is, as Kroll notes, a further concept adopted by national and international institutions (HiLEG, 2019; OECD 2019; DoD, 2020; EO PotUS, 2020), but again it tends to exist as an aspirational principle, rather than a concrete or specific requirement, though it comes closer to the latter in the proposed EU AI Regulation (COM, 2021) particularly Articles 12 and 20. Like explainability, it could potentially involve elements of both push and pull, on the basis that the information is likely to be sought (pull), and in that sense it is closely linked to reviewability or auditability, but it must have, in some way been captured or recorded so that it can be provided (pushed). It also shares with explainability the fact that it builds further on transparency and interpretability or intelligibility, but unlike the other concepts discussed here it is procedural, not substantive. Rather than detailing what must be pushed or pulled, as the other concepts do, traceability is concerned with the process by which this should happen, through the keeping of records which capture the transparent, intelligible material and the explanations built from that material. Traceability thus sits outside and spans the chronology of the other concepts, dealing instead with the documentation of, or as a lawyer might put it, the evidence required to fulfill the other concepts. While important it is, therefore, somewhat beyond the scope of the current article.
2.5. Reviewability or auditability
Moving fully to the perspective of “pull,” rather than allowing the explanation giver to choose and provide the relevant information, which may as a result be too narrow or omit certain things, Norval et al. (Reference Norval, Cobbe, Singh, Cobbe and Singh2021) propose the concept of “reviewability”; a targeted form of transparency whose “purpose is to expose the information necessary to review and assess the functioning and legal compliance of sociotechnical systems in a meaningful way.” In this it seems similar to the concept of “auditability” referred to by Hi-LEG (2019). Chronologically, reviewability thus also builds on transparency and interpretability, but in terms of perspective it does so in order to provide those interacting with the system the tools necessary to ascertain the information they need, rather than allowing the report of what happened to be determined by those giving it. As Norval et al. (Reference Norval, Cobbe, Singh, Cobbe and Singh2021) make clear in their later work, this does not focus reviewability exclusively on those who are subject to the relevant decisions; they see it rather as a means of facilitating “oversight more generally by designers, developers, deployers, users, and overseers.” And of course this in turn means that it can be used by such internal parties to provide better explainability to outside parties.
2.6. Accountability
It is clear, however, that in many cases the vital ultimate step in the chronology is that of accountability. This is not, of course, to deny the intrinsic value of the other stages in the process listed above. Administrative lawyers considering the benefits of procedural fairness (or in the US terminology, due process), are used to dividing its justifications into those which are “instrumental” and those which are “dignitarian.” For “instrumentalists,” the value of a fair process in making decisions is that the decisions themselves are likely to be better as a result (Galligan, Reference Galligan1996; Steyn, 2003; Phillips, 2010). “Dignitarians,” on the other hand, emphasize the intrinsic value of procedures. According to Tribe, for example, the right to a fair hearing expresses the “elementary idea that to be a person, rather than a thing, is at least to be consulted about what is done with one” (Tribe, Reference Tribe1988), whether or not that in fact makes any difference to the ultimate outcome. To these is often added a secondary instrumental perspective that if justice is not only done but is seen to be done (Hewart, Reference Hewart1924), this can also enhance public trust in the AI system enabling it to function and be governed more effectively. These dignitarian justifications, and the wider instrumentalist point about public trust apply equally to the principles of transparency, interpretability, explainability and reviewability examined here, and indeed are often reflected in the policy documents advocating those concepts (EGESNT, 2018; Montreal, 2018; EAD1e, 2019; EP, 2019; ICO and AT, 2020), reinforcing the point that those concepts are indeed valuable in themselves.
But in particular, as Sedley J identified in R v Higher Education Funding Council, ex parte Institute of Dental Surgery [1994] 1 WLR 242:
[t]he giving of reasons may among other things concentrate the decision-maker’s mind on the right questions, demonstrate to the recipient that this is so; show that the issues have been conscientiously addressed and how the result has been reached; or alternatively alert the recipient to a justiciable flaw in the process (emphasis added).
It is thus clear that the key instrumental benefit of these other concepts is their ability to support the final concept of accountability (New and Castro, Reference New and Castro2018), and here too they are often connected to that concept in the relevant policy documents (Royal Society, 2017; EGESNT, 2018; UKAI, 2018; EAD1e, 2019; EP, 2019; HiLEG, 2019; ICO and AT, 2020). In that sense, accountability represents the final step in the chronological map of the concepts, and it is the key concept linking the perspectives of those giving and those receiving the relevant information (see also Floridi et al., Reference Floridi, Cowls, Beltrametti, Chatila, Chazerand, Dignum, Luetge, Madelin, Pagallo, Rossi, Schafer, Valcke and Vayena2018). In a narrower sense, accountability also has the potential to render all the other concepts examined above enforceable in some way, as is clear from many of the policy documents considered so far in particular that of the EP which “considers accountability… to be integral to achieving trustworthy artificial intelligence” (EP, 2019). This works in two ways. First, and more narrowly, unless an entity can be rendered accountable for failing to be transparent, interpretable or reviewable, it is difficult to see how those concepts can really have any practical impact (see also New and Castro, Reference New and Castro2018). But more generally, those concepts can support broader forms of accountability. This may well mean that the other concepts will be shaped by what is ultimately required for full accountability of the system in a wider sense, as the following diagram demonstrates:
Defining accountability is therefore crucial, and given that it is often the point at which ADM will begin to interface with other sociological systems, it is necessary to examine its definition from a fully interdisciplinary perspective. In English “accountability” is capable of covering a range of different meanings, which perhaps explains why the European Union’s Article 29 Working Party felt that it could not be easily translated out of its Anglo-Norman origins into other languages (DPWP, 2010), and why Dubnik writes that “the lack of any inherent definable characteristic that could act as an anchor for the notion of accountability rendered it vulnerable to assuming a broadened meaning tied to the modifying contexts and/or the synonyms with which the word is often associated” (Dubnik, Reference Dubnik2014). Bovens et al. (Reference Bovens, Goodin and Schillemans2014) note that its etymological roots stem from the Middle Ages when it was first used in the Domesday books by William 1 in 1085 as a translation from the French expression “comptes a rendre” (Dubnick, Reference Dubnick2007).
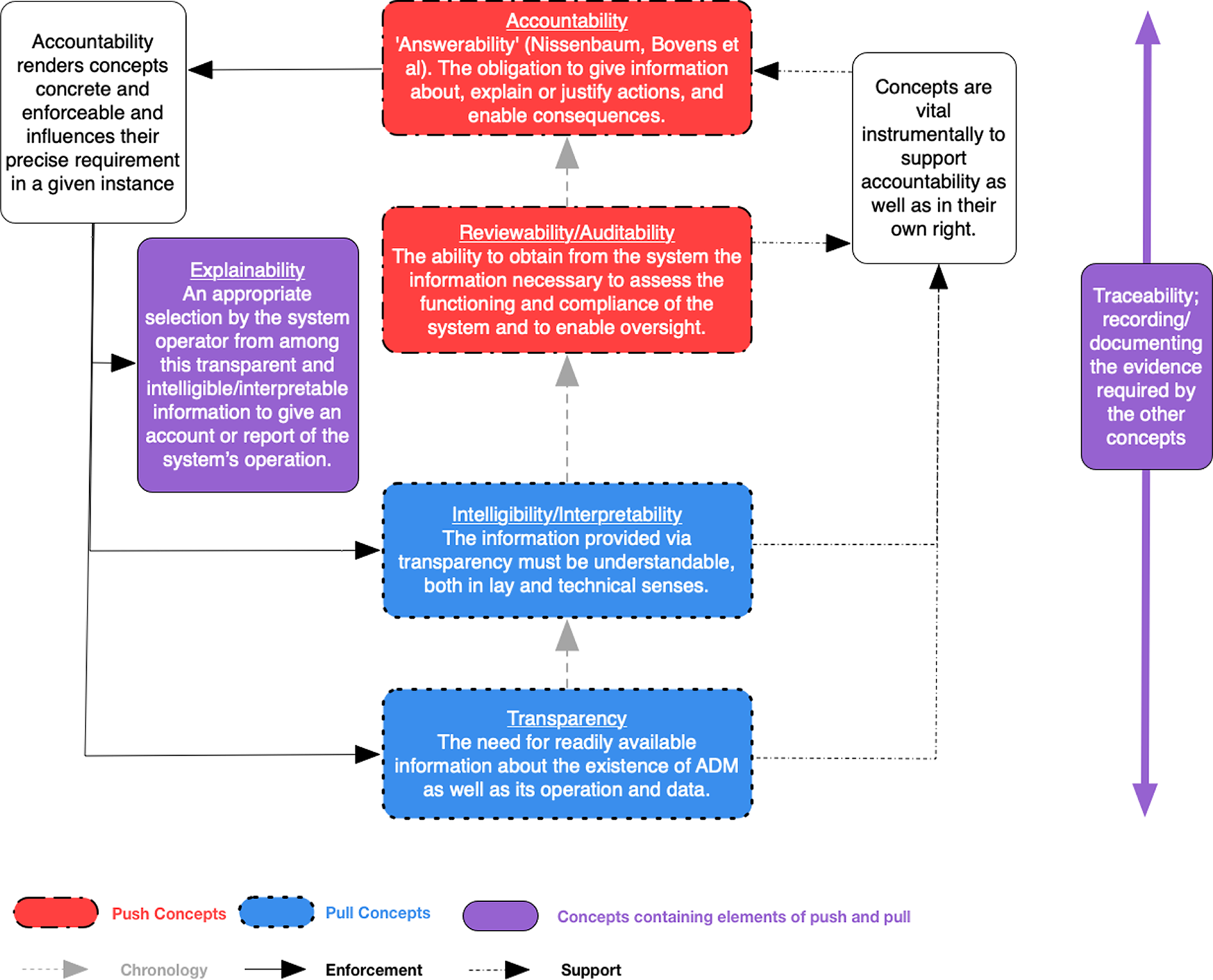
At its most straightforward the concept of accountability entails what Nissenbaum refers to as “answerability”: the obligation to give information about an action taken, explain or justify the taking of that action, and the obligation to make some kind of consequent action, including punishment, rectification, and so forth (Nissenbaum, Reference Nissenbaum1996). Thus, for example, Schedler writes that “A is accountable to B when A is obliged to inform B about A’s (past or future) actions and decisions or justify them and to be punished in the case of misconduct” (Schedler, Reference Schedler1999). Similarly, Binns writes that “A is accountable to B with respect to conduct C if A has an obligation to provide B with some justification for C and may face sanction if B finds the justification inadequate” (Binns, Reference Binns2018) and Bovens et al. (Reference Bovens, Goodin and Schillemans2014) note that accountability is therefore a “relational” concept, linking those who owe an account and those to whom it is owed, as well as being retrospective and consequential (in the sense that it entails consequences, often punishment) (see also New and Castro, Reference New and Castro2018).
However, as Bovens et al. (Reference Bovens, Goodin and Schillemans2014) note, while all disciplines begin with this core of meaning, it is then often “thickened” out in different directions by research in different disciplines, leading to what they criticize as being “fragmented and noncumulative scholarship,” which their Handbook aims to reverse. As Bovens (Reference Bovens2007) further notes, accountability often serves as a conceptual umbrella that covers various distinct concepts such as those identified above, as well as equity, democracy, efficiency, responsiveness, responsibility and integrity, or is interchangeable with “good governance” or virtuous behavior. “Discussions,” writes Bovens (Reference Bovens2010), “seem to go in circles, as every volume and author tries to redefine accountability in his or her own way.” To paraphrase Aaron Wildavsky: “If accountability is everything, it may be nothing.” Similarly, Koppell criticizes a phenomenon he refers to as “Multiple Accountabilities Disorder” (MAD): “the lack of specificity regarding the meaning of accountability, or failure to articulate a choice—can undermine an organization’s performance” (Koppell, Reference Koppell2005). Using the organization ICANN (the Internet Corporation for Assigned Names and Numbers) as a case study he argues that MAD can have two problematic effects. “First, the organization may attempt to be accountable in the wrong sense (such as a judge taking orders). Second, and perhaps worse, an organization may try to be accountable in every sense… pleasing no one while trying to please everyone” (Koppell, Reference Koppell2005).
3. Taxonomies of Accountability
3.1. Accountability as a virtue and as a mechanism
In order to address this problem, Koppell suggests that we should distinguish between five different dimensions of accountability: transparency, liability, controllability, responsibility, and responsiveness, though of course we have suggested above that the first of these, transparency, is a separate concept.
Each of Koppell’s conceptions of accountability is then assessed by asking a critical question of the accountable organization (though there is no reason that these questions could not be extended to any other kind of entity to be held to account):

a Which presumably includes any external, regulatory rules.
However, Bovens argues that a further distinction should be made between this approach, which he suggests regards accountability as a “virtue,” and an approach which examines accountability as a mechanism (Bovens, Reference Bovens2010). Building on this, the A4Cloud project defines a “three-layer” model of accountability in the context of data governance, distinguishing between accountability attributes, practices, and mechanisms.
The A4Cloud project has identified five core Accountability Attributes (Cattedu et al., Reference Cattedu, Felici, Hogben, Holcroft, Kosta, Llenes, Millard, Niezen and Nu2013), which clearly draw on those listed by Koppell. The first of these is transparency which we have identified as a separate concept, the others being as follows:
-
1. Responsiveness: the property of a system, organization or individual to take into account input from external stakeholders and respond to queries of these stakeholders.
-
2. Responsibility: the property of an organization or individual in relation to an object process or system of being assigned to take action to be in compliance with the norms. This fits with the ICO&AT’s first facet of accountability which is “taking responsibility for complying with the other data protection principles” (though of course other regulatory principles may be relevant too) as well as the assignment of responsibility within an entity.
-
3. Remediability: the property of a system, organization or individual to take corrective action and/or provide a remedy for any party harmed in case of failure to comply with its governing norms.
-
4. Verifiability: the extent to which it is possible to assess compliance with accountability norms. Again, this fits with the ICO&AT’s second facet of accountability which is “being able to demonstrate that compliance” referred to in the first facet.
To which the A4Cloud project later adds assurance, obligations, liability, sanctions, and remediation.
However, in keeping with Bovens’ distinction between accountability as a virtue and as a mechanism, these five attributes are part of a broader taxonomy which divides the accountability model into three layers; attributes, practices, and mechanisms. Accountability attributes, “are the concepts from which accountability is built” (Cattedu et al., Reference Cattedu, Felici, Hogben, Holcroft, Kosta, Llenes, Millard, Niezen and Nu2013). “Accountability practices are sets of behaviors that an organization should have in order to be accountable.” These in turn they distinguish into four broad categories:
-
1. Defining governance to comply in a responsible manner with internal and external criteria,
-
2. Ensuring the implementation of appropriate actions to actualise such governance,
-
3. Explaining and justifying those actions, namely, demonstrating regulatory compliance, and
-
4. Remedying any failure to act properly.
Accountability mechanisms, on the other hand, “are procedures and tools—often technical tools, including software, but also organizational and/or legal procedures and other mechanisms—by which accountability practices are supported and implemented.”
3.2. Accountability as a series of key questions
But cutting across these taxonomies is another, which divides accountability into a series of key questions (Bovens et al., Reference Bovens, Goodin and Schillemans2014):
-
- Who is accountable?
-
- To whom?
-
- For what?
-
- By which standards?
-
- And why?
To which we add the further question of “how” this accountability might be brought about. It might at first be thought that this cross-cutting taxonomy is simply yet another definition of accountability which further increases the fragmentation of the concept and resulting “Multiple Accountabilities Disorder.” However, in our view it is this taxonomy which helps us both to understand why those multiplicities exist and to resolve them in a way that enables the concept of “accountability” to play a useful role across a series of different contexts. The multiple definitions of accountability and its fragmentation into different disciplines occur precisely because accountability does mean different things in different sociolegal contexts. If we therefore address those differences directly and establish the purpose for which we want to establish accountability and thus the precise form of accountability we wish to consider in a given instance, we can avoid disorder while still recognizing the multifaceted nature of accountability in different contexts. This approach allows us to choose and tailor the appropriate version of accountability for the relevant circumstance.
3.2.1. Why? Accountability for what purpose?
From this point of view it perhaps makes sense to begin with the last of the questions listed by Bovens et al. above, and ask what it is that we are trying to achieve through accountability. Nissenbaum points to a number of purposes of accountability, which as before are closely related to the other questions examined so far. For some, she notes, in line with our dignitarian perspective, a developed sense of responsibility is a good in its own right, and so within this taxonomy it is here that accountability may be regarded as a virtue to be encouraged (Nissenbaum, Reference Nissenbaum1996). For others, to take our more instrumental perspective, it is valued because of its consequences for social welfare; holding people accountable for the harms or risks they bring about provides strong motivation for trying to prevent or minimize those risks. Accountability can thus be “a powerful tool for motivating better practices and consequently more reliable and trustworthy systems.” For this reason, she argues, accountability should be encouraged not only in relation to “life-critical systems,” but even for more minor malfunctions causing individual losses of time, convenience and contentment (Nissenbaum, Reference Nissenbaum1996). Similarly, Reed et al. (Reference Reed, Kennedy and Nogueira Silva2016) note that accountability requirements may not be aimed at resolving legal liability questions but rather at reassuring the public, for example, that self-driving technology has been developed with public safety in mind, and in a way which allows problems to be identified and rectified, though of course as they and Nissenbaum discuss, accountability can provide a reasonable starting point for punishment or compensation. It is this aspect of accountability which makes it so crucial in the scheme developed here. It is accountability which gives impetus and traction to the other concepts and those other concepts will in turn be shaped by what is necessary to achieve accountability in a particular instance. Thus, as the table below will illustrate further, which of these aims we want to achieve in a given instance (compensation, punishment, public reassurance, and better incentivization) will influence our chosen form of accountability and thus the answers to all the other questions listed above. We do not propose any particular hierarchy between these purposes; they can all be found in different contexts and the choice to pursue, for example compensation as opposed to punishment, or rehabilitation as opposed to retribution, must be made on broader moral, economic, political, or other grounds beyond the scope of this investigation. Our point is simply that once one of these purposes has been chosen, this purpose will, as we go on to demonstrate, have an inevitable impact on the mechanism, target, subject matter, recipient, and standards of the accountability at issue.
3.2.2. How is accountability achieved?
Once we know our purpose in establishing accountability the next obvious question is how this might be achieved. Romzek and Dubnick (Reference Romzek, Dubnick and Shafritz1998) plot four different mechanisms of accountability that might be used; bureaucratic accountability, legal accountability, professional and political accountability in the following matrix:

And summarize the principal features of the four different types of accountability systems as follows:

Later, Dubnick develops this scheme by regarding accountability as a “genus” of which there are various species which can be distinguished not just by the settings within which they are likely to appear (and it is evident that the forum in question is relevant), but also by whether they are related to accountability through the process of moral push or moral pull (Dubnick, Reference Dubnick, Sampford, Preston and Boise1998):

Dubnick also examines the various narratives which have shaped the definition of accountability by reference to the ways in which it should be brought about, developing yet another matrix as follows:

The “how” of accountability is also the focus of Mansbridge’s work. She argues for a contingent approach which asks when accountability systems should rely most heavily on sanctions and when they can mix in more elements of up-front selection and justifiable trust so that instead of arguing for “more accountability” we should think more carefully about how best to achieve it (Mansbridge, Reference Mansbridge2014).
Once we have established the purpose and method of accountability, this will start to influence the answer to a third question.
3.2.3. Who is accountable?
Beginning with the key questions that are the focus of much accountability scholarship, Coeckelbergh (Reference Coeckelbergh2012) notes that there is often a difference between different disciplines regarding the target of accountability. Thus, while “the conditions for attributing moral responsibility prescribed by traditional theories make demands on agency, control and knowledge” these are “seldom met in engineering and—more generally speaking—technological action.” Thus, while in line with Aristotle’s Nichomachean Ethics responsibility is individual and is based on having control and knowledge (Aristotle, n.d.), technological action is often distributed and collective rather than individual and should therefore be understood as distributed between various actors at various levels and times (Coeckelbergh and Wackers, Reference Coeckelbergh and Wackers2012). This is so even with more straightforward rules-based systems, but applies to an even greater degree when those systems are themselves autonomous to some extent. This, of course, provides further evidence for the interdisciplinary distinctions identified by Bovens et al. (Reference Bovens, Goodin and Schillemans2014), who equally note that law, international relationship, public administration, accounting, and politics may focus on entities such as government agencies, legal bodies, transnational actors, political parties, NGO’s, public contractors, semiindependent public bodies, and private enterprises as well as individuals.
These differences of focus also demonstrate the interdependence of the different key questions within this taxonomy. Identifying the target of accountability by reference to responsibility inevitably connects the “who” with the “why” as well as the “how.” Bovens et al. distinguish on this front between hierarchical accountability within an organization, collective accountability to the organization as a whole and individual accountability. This question is obviously also significant because while up until now there have been difficulties enough in attributing individual versus collective responsibility (Williams, Reference Williamsforthcoming), technology provides further challenges in the sense that it can exacerbate this problem (HCSTC, 2018), and because, as noted above, the “who” that at least initially fulfills the control and knowledge conditions may in fact be a machine.
3.2.4. Accountability to whom?
This question again demonstrates the interlinked nature of the different questions, since “to whom” is again inherently connected to the “why” and “by which standards.” Lawyers, inevitably, will thus focus either on the potential victims of the activity to which the accountability relates (the rules on standing), or on the state as representing accountability to society generally (Marshall and Duff, Reference Marshall and Duff1998), and will look to regulatory standards. However, Diakopoulos (Reference Diakopoulos2015) writes about the role of journalists in achieving accountability to the user and to the wider public directly. Thus again, different disciplines will focus on different answers to this question. Bovens et al. (Reference Bovens, Goodin and Schillemans2014), for example, point to a variety of accountability relationships based on a variety of different fora, distinguishing in particular between political, managerial, administrative, legal, and professional forms of accountability (Mulgan, Reference Mulgan2003; Politt, Reference Politt2003; Romzek, Reference Romzek and Perry1996).
3.2.5. Accountability for what?
Again, this question is highly interlinked with those considered so far. For example, if the purpose is to establish criminal liability, the accountable entity can only be liable for effects it has caused, while financial accountability might be of most relevance to accountants. Bovens et al. (Reference Bovens, Goodin and Schillemans2014), thus cite Day and Klein (Reference Day and Klein1987), Sinclair (Reference Sinclair1995), and Behn (Reference Behn2001) as suggesting that accountability relationships may center on different types of “content”; financial, procedural, communicative, and so forth. Thus Behn, for example, sorts accountability into four categories: accountability for finances, for fairness, for abuse of power, and for performance, arguing that accountability for finances and fairness are more common because of the relative ease of holding an entity to account on these two grounds as distinct from the others. In other contexts, the “for what” presupposes a framework of established principles with which the actor must comply and must demonstrate compliance (Behn, Reference Behn2001).
3.2.6. Accountability by what measure?
Romzek and Dubnick argue that this too depends on the particular discipline and forum at issue, as well as the particular “thicker” definition of accountability chosen. In other words, the measure of accountability will again be directly connected to the method used to achieve it, its target and so on. Romzek and Dubnick’s particular definition of public accountability is “the means by which public agencies and their workers manage the diverse expectations generated within and without the organization,” and their proposed framework divides this public sector accountability into bureaucratic, legal, professional and political measures (Romzek and Dubnick, Reference Romzek and Dubnick1987). It seems likely, however, that their framework could have a broader application than this, though in the private sector an additional form of accountability to the market, either shareholders or consumers, might usefully be added.
Binns, on the other hand, attempts to produce a more general measure of accountability based on the concept of public reason. Noting the “ambiguity” in what he sees as the final step in accountability, in which the recipient of the account either accepts or rejects the account given, he asks what kinds of justifications a decision-maker can legitimately expect will satisfy the decision-subject. Binns’ analysis is that, whether the decision is an algorithmic one or not, the problem is the same and is typical of a more general, long-debated problem in moral and political philosophy relating to “the tension between… the need for universal political and moral rules which treat everyone equally” and the contrary possibility that “reasonable people can disagree about the very matters of knowledge, value and morality on which those rules might be decided.” His solution is to turn to public reason which proposes that universal rules must be justifiable on grounds that are suitably public and shared by all reasonable people in society, without appeal to controversial beliefs. The precise content of these principles should emerge from a “process of reflective equilibrium between equal citizens” (Binns, Reference Binns2018).
4. Resolving the Multiplicities of Accountability
To summarize, then, the most important consideration to address first is why we are concerned about accountability in a particular context and what we are trying to achieve by discussing it. That question is then inherently connected to the other key questions previously outlined; once we have identified why we want to discuss or enhance accountability in a particular context that will help us to determine how best we might achieve that. This will in turn, as explained above, tend to dictate who should be accountable, to whom, for what, and by what measure. Thus, for example, if we want to provide particular incentives to an entity, backed up by sanctions and possibly with compensation for those who are injured by failures in the system, we might look to a system of tort or criminal law, which in turn would suggest that those causally responsible for the damage should be liable to those with standing to pursue the claim (i.e., those damaged by it) and that this would follow the negligence standard developed by that area of law (EP, 2019). If, on the other hand, we wanted to achieve a change in the law as well as a change in incentive we might turn to the political system which would hold elected representatives accountable to those who had elected them for any promises they may previously have made, according to the standards of public opinion.
These different systems of accountability within the “key questions” taxonomy then merge with the “mechanisms” and “practices” section of the alternate taxonomy adopted by Bovens, Koppell and the A4Cloud approach, as well as with their “remediability” attribute or values. Thus, for example, a court’s mechanism of holding someone legally liable will result in certain practices such as insurance, behavioral changes, regulatory compliance and so on. In the case of some systems such as law, the mechanism also absorbs their additional attributes of obligations, liability, sanctions, and remediation, since the law will define the specific obligations to which entities are subject, as well as the sanctions for their breach, the rules for allocating liability and the necessary remedy in the form of compensation, specific performance, and so forth. The “Responsiveness” attribute from AI4Cloud’s attributes, mechanisms and practices scheme also merges with the question “to whom” the entity should be made accountable, while the “responsibility” attribute merges with the question of who should be responsible. It is therefore possible to combine the various proposed schemes into an overall map, and to illustrate how this might work by reference to specific examples. In other words, the solution to multiple accountability disorder and fragmentation of the concept is to see the inherently multifaceted nature of accountability as its strength. Disorder is to be avoided not by seeking to unify accountability under one heading, but by having a clear chart of the different purposes of accountability (why?), the different mechanisms by which these purposes might be achieved (how?), and the inherent links between this purpose, mechanism and other questions such as the target (who?), subject matter (for what?), recipient (to whom?), and the standards of accountability in each instance (by what measure?). This clarity will then enable us to choose the most appropriate form of accountability in each context, as the following table demonstrates.

* Practices listed as: (a) Defining governance to comply in a responsible manner with internal and external criteria; (b) Ensuring the implementation of appropriate actions to actualise such governance; (c) Explaining and justifying those actions, namely, demonstrating regulatory compliance, and (d) Remedying any failure to act properly (p. 2).
** The details in the adjacent cells are inferred from and build on the scheme devised by Romzek and Dubnick, p. 37.
5. Linking the Two Maps: Viewing the Other Concepts Through the Lens of Accountability
Not only does this more structured understanding of accountability help us to avoid disorder in that context, it also allows us understand more specifically what might be entailed in each of the other concepts outlined above. Thus, for example, if a company uses a system which causes harm, this may well result in some form of bureaucratic or professional accountability within the organization. Those responsible for designing or deploying the system will need to make readily available (push) information about what the system was supposed to do; as far as possible what has happened; who was involved in any relevant processes or choices and who has been affected by it. This information must be interpretable or intelligible to those further up the organization. In other words those lower down the chain (the “who?”) must provide (push) sufficient transparency and interpretability/intelligibility for those higher up the chain (the “to whom?”) to be able to establish the answers to “what” has happened and what should be done about it. They should also be able to supply some kind of account or explanation of the “what” (explainability). Those higher up the chain must also be able to obtain (pull) any further information they find to be necessary (reviewability) in order to assess the functioning and compliance of the system and to enable satisfactory oversight of it (the standard of accountability in this context).
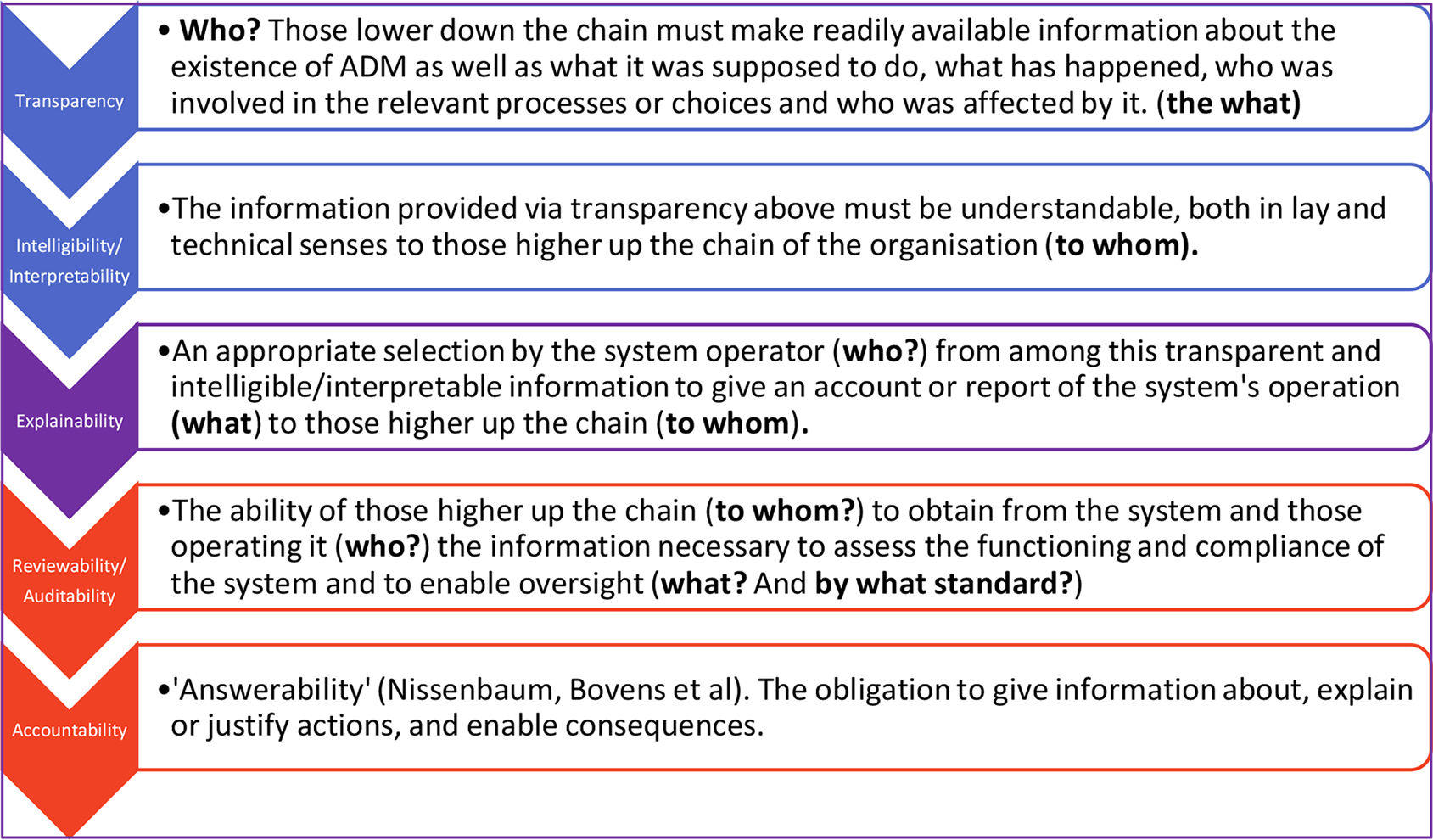
If such harm has occurred there may also be a desire for some form of punishment or compensation (the why) which would suggest legal liability of some kind (the how). Those responsible (the who) may well be those with a causal input into the chain of events leading up to the harm, who would be accountable to the victims of such harm or to the state (to whom) for failure to comply with the standards of tort law or criminal law respectively. In such instances the court will need transparency of information, which must be intelligible to or interpretable by those involved in the legal system (including by a lay jury in the context of criminal law), those defending the system will need to be able to explain its operation, while claimants, prosecutors and the court in general will need to be able to ascertain the information necessary to allow them to assess the functioning and compliance of the system with any applicable rules. In this context the “push” and “pull” aspects of these concepts will be governed by specific legal duties of disclosure as well as burdens of proof which rest to varying degrees on prosecutors and claimants.
Indeed in some instances, such as the GDPR for example, the form of accountability will affect not only the quality of the information available when required (reviewability, transparency and interpretability) but also enhance the onus on the system operator to provide (push) that information (explainability, or indeed explanation). There is thus a direct and symbiotic relationship between these other concepts and accountability, in that their content and requirements are directly informed by what is necessary for the particular form of accountability at issue, but it is also that accountability which provides the vehicle which renders them enforceable and practically useful. Accountability is thus the key lens through which the concepts as a whole should be viewed.
6. Challenges to Accountability
This does not mean, of course, that accountability will always be straightforward. Nissenbaum, for example, lists four main barriers to accountability in “a computerized society” as being the problem of many hands; a complacent tendency to accept software flaws as inevitable; a tendency to use “the computer” as a scapegoat and the tendency of software producers to deny accountability while leaving it to their software licensees who are least well placed to be accountable (Nissenbaum, Reference Nissenbaum1996). Diakopoulos adds that problems also arise from lack of enforcement of accountability mechanisms that might be in place and from a tendency to game and manipulate any standards used, to which we might add the difficulty of specifying sufficiently precisely the level of compliance necessary in any given case. Diakopoulos also notes the lack of accountability that can arise from trade secrets (Diakopoulos, Reference Diakopoulos2015) (a clash also noted by Bennett, Reference Bennett2013), the use of legacy code which cannot easily be reconstituted, or by the pure complexity of the scheme used. Elish suggests that in turn the resulting gaps in accountability tend to be filled by a “moral crumple zone” in which “the human in a highly complex and automated system may become simply a component… that bears the brunt of the moral and legal responsibilities when the overall system malfunctions” (Elish, Reference Elish2019) But even here it is evident that a better understanding of the concepts defined above can help. The problem of many hands, for example, or those of scapegoating and buck-passing are essentially problems of attribution of causal liability which can be addressed at least to some extent by greater transparency and explanation. A tendency to accept software flaws as inevitable suggests a failure in accountability that could be addressed directly, while trade secrets and scheme complexity go directly to requirements of transparency and interpretability respectively. And Elish’s moral crumple zone arises precisely because of an inaccurate placement of liability resulting from the failure of those earlier concepts; a situation that a more accurate form of accountability, supported by the other integral concepts, could prevent. If the correct form of accountability is thus identified, and the related concepts of transparency, interpretability, and accountability are deployed as necessary to support it they can as a whole provide a structure which renders those deploying a system “answerable” to those affected by it in a manner which provides concrete remedies and incentives.
It is clear, however, from the context-specific nature of accountability that achieving it will require the input of the relevant discipline for each context. But it is also clear that when it is accountability for an autonomous system that is at stake this process must be fully interdisciplinary, involving both the relevant discipline and technologists or computer scientists on both substantive and procedural fronts. This is imperative both because the accountability discipline (law, politics, etc.) must fully understand the relevant technology in order to provide an optimal form of accountability and because, conversely, technology can in fact underpin and help to realize that accountability (Naja et al., Reference Naja, Markovic, Edwards, Cottrill, Verborgh, Hose, Paulheim, Champin, Maleshkova, Corcho, Ristoski and Alam2021).
7. Conclusion
In conclusion, as intelligent systems are deployed in an ever wider variety of contexts, those responsible for their governance have responded by developing a series of overlapping abstract concepts which aspire to regulate its operation. However, if those concepts are to do the work expected of them in regulating and governing such systems, they must become specific and enforceable. We have in this article taken two steps toward achieving that aim. First, we have identified more precisely what each concept requires and in particular we have examined how the concepts fit together in terms of chronology and the extent to which they require the provision (push) or active seeking (pull) of information. And second, we have argued that the key concept in rendering them enforceable is that of accountability. There is a variety of taxonomies of accountability in the literature. However, at the core of each account appears to be a sense of “answerability”; a need to explain or to give an account. It is this ability to call an entity to account which provides the impetus for and ability to enforce each of the other concepts. Conversely, if we divide accountability more specifically, as suggested above, into questions of who is accountable, to whom, for what, by what measure and why, this in turn will inform what precisely is required in terms of transparency, interpretability, explainability, or reviewability in a particular instance. This understanding will then enable us to develop the supporting sociotechnical infrastructure needed to realize these more concrete concepts and enable them to fulfill their intended roles.
Funding Statement
This work was supported by the Engineering and Physical Sciences Research Council (EPSRC) under research grants EP/R033846/1, EP/R033501/1 and EP/R03379X/1. The funder had no role in study design, data collection and analysis, decision to publish, or preparation of the manuscript.
Competing Interests
The authors declare no competing interests exist.
Author Contributions
The authors have all contributed to the work in accordance with the Publishing Ethics Guidelines.
Data Availability Statement
This study does not contain empirical data. The other resources on which the article draws are listed in the references section.
Comments
No Comments have been published for this article.