8.1 Introduction
The advance of GPS technologies and the swift adoption of smartphones has enabled the rapid growth of on-demand ride services. People, being connected to the network anywhere anytime, started to see the benefits of having on-demand access to a ride from multiple competing providers. It was a convenient system for all involved parties. Moreover, not adapting to the new network-based system meant missing out on benefits for the riders and losing the market share for the ride-hailing companies. As a result, as Figure 8.1 illustrates, even traditional street hailing services adopted e-hailing systems quite quickly. On-demand ride-hailing platforms, such as Uber and Lyft, have grown rapidly in the last decade in almost all countries; in some markets, they doubled the number of riders each year. It is fascinating to see a service that did not exist just ten years ago become so significant. It is now the fastest-growing transportation mode, has gained a larger market share than taxis in many cities, and has provided another transportation option and much more flexibility to passengers. It has altered the urban mobility landscape in a significant way. However, just like any other disruptive technology in its early years, it has brought some problems too. Municipal officials are concerned about congestion and pollution, drivers complain about their working terms (as evident in the forum [1]), taxi companies are enraged about unfair competition, and passengers are often agitated with sudden spikes in fares, all suggesting that this new experience is far from being stabilized.

Figure 8.1 The figure shows the categories of for-hire vehicles (FHV) in New York City and the fact that they all adopted e-hail/e-dispatch systems by 2015 [2].
One of the primary questions about ridesharing services is whether they decrease or increase traffic congestion (and relatedly carbon dioxide [CO2] emissions). Traffic congestion is a serious and growing concern in modern life in metropolitan areas. The 2019 Urban Mobility Report of the Texas Transportation Institute [Reference Schrank, Bill and Tim3] shows that the national congestion cost in the United States has risen from $75 billion in 2000 to $179 billion in 2017 (both in 2017 dollars), a whopping 139 percent increase in less than two decades that signifies the urgency of addressing this issue. Due to the rapid expansion of ridesharing services in urban areas in the last decade, the question of their impact on traffic congestion and carbon emissions has gained considerable interest among researchers and policymakers. Researchers are clearly split between advocates and critics of ridesharing services. On the one hand, these platform-enabled ride-hailing services may decrease traffic by increasing capacity utilization and reducing ownership/use of private cars. On the other hand, this type of ride-hailing may increase traffic congestion and pollution by replacing carpooling and nondriving modes such as mass transit, biking, and walking with separate and nonshared platform-initiated rides or motivating a new trip that would have not been made otherwise. These latter findings have surprised many observers since these services were expected to help mitigate traffic congestion.
This chapter reviews the literature and analyzes different perspectives on this debate,Footnote 1 describes key policy measures, and determines the future research opportunities that could help to settle this debate. The remainder of this chapter is organized as follows. Section 8.2 provides a historical overview of ridesharing services. Section 8.3 details the arguments on each side of the debate concerning ridesharing and traffic congestion. Section 8.4 reviews some policy measures for mitigating the negative impact of ridesharing services on congestion. Finally, Section 8.5 identifies the research gaps in this area for future research.
8.2 Ridesharing – A Historical Overview
The idea of ridesharing (people sharing a trip in the same vehicle) is as old as the history of transportation and human traveling. Additionally, the negative externalities and challenges that appear to be associated with ridesharing services are similar to the negative externalities brought with any other disruptive technology throughout history. In the field of urban mobility, when the first hackney coach/carriage, the ancestor of modern days taxis [Reference Gilbey4], was introduced in London in 1605, everybody was excited and pleased with the service. However, the number of hackney coaches grew very fast, brought great nuisances to the streets of London, and raised fierce competition and bitterness between hackney coaches, wherries, barges, and sedan chairs (the popular urban transportation modes of that time) [Reference Gilbey4]. By 1635 Charles I, the king of England, issued a proclamationFootnote 2 banning and limiting the usage of hackney coaches in specific regions [Reference Anderson7]. Hackney coaches had a bumpy road with many ups and downs until the 1660s, when finally the Hackney Carriages Act of 1662 recognized the hackney carriages as one of the modes of transportation and regulated their usage concerning fares, crawling, days and hours of operation, licensing, the permissible number of licensed coaches, and specifications of horses used [Reference Gilbey4]. The current questions and debates about ridesharing services and some of the policy responses are remarkably similar. As Mark Twain famously said: “History doesn’t repeat itself, but it often rhymes.”
By the end of the twentieth century, ridesharing became a necessary and inseparable part of modern life. Because of the rapid expansion of technologies and inventions in recent decades and the complexities of modern societies, current app-based ridesharing services are conglomerates of different features of older ridesharing services, each having a different history. It is useful to learn these histories to understand their impact on current transportation systems. The rest of this section briefly reviews the histories of dial-a-ride transit, carpooling, and carsharing as the predecessors of modern ridesharing options, and then it reviews the history of platform-based services.
8.2.1 Dial-a-Ride Transit
The paratransit system, commonly known as Dial-a-Ride, is a door-to-door or curb-to-curb transportation service for people with special needs, including seniors and people with disabilities, who cannot use the standard fixed-route transit systems. People can request this service for commuting to work or school, going to a hospital, visiting a friend, or just shopping or doing groceries, typically trips constrained within a geographic zone. The establishment of the service in the United States dates back to 1970 when it started in Mansfield, Ohio in a collaboration project with Ford Motors. Two years later it was followed by a similar service in the United Kingdom in the town of Abingdon [Reference Oxley8]. The service is technically an on-demand service, but in reality most often it must be prescheduled. There is a spectrum of services in different paratransit systems where on the one end the service could be an on-demand ridesharing service that would go through a fixed route and on the other end, it could be a fully responsive transport system providing door-to-door service from any origin to any destination within a city or a specified service area. The vehicles are usually specialized cars, minivans, vans, or minibuses well-equipped to handle passengers with wheelchairs or other special needs. The passengers are pooled together to share a ride based on their origin–destination (OD) pairs and requested time intervals.Footnote 3
8.2.2 Carpooling
The concept of carpooling (and vanpooling) goes back for decades and in the United States it became very common during World War II with efforts to save fuel for the war (see Figure 8.2). It again became popular during the oil crisis in the 1970s, due to the high cost of gasoline, before gradually declining in the years that followed [Reference Ferguson10, Reference Morency11]. Carpooling can occur in three different ways: 1) commute carpooling, where people arrange their commute on a long-term basis and commit to mutually agreed and predetermined pick-up and drop-off locations and times; 2) long-distance carpooling, where drivers and riders match for a potentially one-time long-distance trip with advance scheduling and typically with an abundance of flexibility; and 3) casual carpooling (also known as “slugging”) [Reference Kelly12], where drivers and riders start a one-time relatively short rideshare trip on the spot similar to hailing a taxi on the street.Footnote 4

Figure 8.2 World War II posters promoting carpooling for work commute.
8.2.3 Carsharing
Carsharing typically refers to short-time car rentals, often for less than an hour, for a single trip within the city. The fleet usually is comprised of small electric/hybrid cars and is geographically distributed in different parts of the city. The members of a carsharing club can see the location of the closest car on a map in the carshare provider’s mobile application. Depending on city regulations, sometimes they have specific parking places, and sometimes they can be parked at any free curbside parking spot. Notable providers of this service in the last fifty years include Witkar, Communauto, ZipCar, FlexCar, City Car Club, and City CarShare. In recent years peer-to-peer carsharing is also becoming popular through services such as RelayRides and Getaround.Footnote 5
8.2.4 Application-Based Ridesharing Services
It is difficult to pinpoint the first app-based or peer-to-peer ridesharing service. Neither Lyft nor Uber gets the credit, as many other platforms started offering ridesharing services ahead of them. These platforms include Craigslist Ride Board, CarPoolWorld, BlaBlaCar, GoLoco, PickupPal, Avego (currently Carma), ZimRide, Flinc, SideCar, and Tickengo (currently Wingz).
Among these platforms perhaps SideCar is the most notable. It was the first ridesharing service that started operation in San Francisco in 2011, and its purchase by General Motors in 2015 was very well-publicized [Reference Nagesh16, Reference Paul17], bringing more attention to app-based ridesharing services. SideCar could also be credited as the inventor of platform-based, on-demand, short-distance, intracity ridesourcing, as Chief Executive Officer (CEO) Sunil Paul’s Reference Paul2002 patent suggests [Reference Paul18]. Another notable platform is ZimRide. ZimRide is a long-distance, intercity ridesharing service initially targeting college students and was founded in 2007 after one of its founders observed strangers to each other sharing a ride in Zimbabwe. ZimRide connected drivers and riders through Facebook to ensure security.
The currently dominant ridesharing services were born through these early experiences. Lyft was started as a side project within Zimride to provide intracity ride services and initially launched in San Francisco in May 2012. Since the market was extremely asymmetric in favor of Lyft, the founders offloaded the intercity carpooling section of the company and sold ZimRide to the car-rental giant Enterprise in 2013 to focus more on developing Lyft. Uber was founded in 2009 and officially launched its service in San Francisco in 2011 as a limousine or luxury black car service for more affluent customers. Later, in the summer of 2012, it developed into the ridesharing service as we know it now by launching Uber X, which allows individuals to be Uber drivers with their own cars. This was about the time of Didi’s inception in China, which later became the second largest ridesharing service in the world, as Lyft and Uber also started to expand globally. Many other similar services were developed in other countries; see Table 8.1 for a short list.
Table 8.1 Some of the non-United States platform-based ridesharing companies
Company | Country of Origin | Operation Started in |
---|---|---|
BlaBlaCar | France | 2006 |
Carma (formerly Avego) | Ireland | 2007 |
Flinc | Germany | 2010 |
Ola | India | 2010 |
Gett | Israel | 2010 |
Cabify | Spain | 2011 |
Yandex | Russia | 2011 |
Didi | China | 2012 |
Grab | Singapore | 2012 |
Careem | UAE | 2012 |
Via | Israel | 2012 |
Bolt (formerly Taxify) | Estonia | 2013 |
Snapp | Iran | 2014 |
Gojek | Indonesia | 2015 |
FreeNow | Germany | 2019 |
Initially, neither Lyft nor Uber had a carpooling or ridesharing option leading many transportation experts to use the term “ridesourcing” for their service instead of any term that would include “pooling” or “sharing.” It could be the case that the adoption of the term “ridesharing” by many of these platforms in the early years of their operation when they were essentially operating as de facto taxi companies, was to avoid regulations surrounding taxis. To finally remove the ambiguity, in 2013, a new category of mobility service was defined in the United States as transportation network companies (TNCs) to distinguish the operation of these services from taxis, chauffeured car services, and other for-hire vehicles [Reference Geron19].
Eventually, in 2014 Lyft Line and Uber Pool were introduced, where multiple passengers could “share” a ride [Reference Rodriquez20]. Later in 2015 and 2016, new services such as Uber Commute, Uber Destinations, and Lyft Carpool were launched, where drivers were assumed to have a predetermined destination towards which they would pick up those passengers heading in that same direction. This was very similar to classical commuting carpooling and casual carpooling [Reference Byford21, Reference Hawkins22, Reference Hawkins23]. Uber also launched Uber HOP in 2015 [Reference Hawkins24, Reference Soper25], which appears to have morphed into Uber Express Pool in late 2017 and early 2018 [Reference Etherington26], in which riders have to walk to an efficient pickup location, in exchange for a cheaper fare, and share the ride with other passengers to drop off locations that are close to (but not exactly) their destinations. It was followed by a similar service by Lyft called Shared Saver in early 2019 [Reference Hawkins27].
It is important to keep in mind the above chronology of events in the development of ridesharing services when reviewing the literature in the next section, as some of the studies span a certain period of time.
8.3 Is Ridesharing a Solution or a Concern?
Early studies about ridesharing platforms mostly promoted ridesharing services as having the potential to improve the overall efficiency of the transportation system by reducing the idle capacity of vehicles. Data from the National Household Transport Survey (NHTS) [28] show that during the period April 2016 to March 2017 about 94 percent of personal vehicles in Massachusetts were idle (parked) 94 percent of the time (see Figure 8.3). Platform-based ridesharing held the promise of using some portion of this idle capacity, thereby contributing to the overall economy. These early studies also proposed the idea that app-based ridesharing services may reduce road congestion and carbon emissions in metropolitan areas, primarily by increasing capacity utilization in each trip [Reference Li, Hong and Zhang29, Reference Li, Hong and Zhang30]. These studies showed that a significant level of ridesharing adoption would have considerable benefits for reducing traffic and CO2 emissions.

Figure 8.3 Distribution of active time percentage of personal vehicles in Massachusetts from April 2016 to March 2017.
However, as the early fascination faded and people experienced the new system, some reports suggested that ridesharing services such as Uber may be actually contributing to congestion instead of decreasing it [Reference Rayle, Dai, Chan, Cervero and Shaheen31], while other reports were inconclusive [Reference Merrill and Coote32]. These studies, and highly publicized challenges such as the feud between Uber and New York City [Reference Flegenheimer33, Reference Flegenheimer34] and the lawsuits that Uber faced in recent years which resulted in bans on Uber’s operation in many cities and countries around the world [35, Reference Rhodes36], initiated the idea of regulating the industry. Policymakers around the world considered a broad range of regulations, including a cap on the number of operating vehicles, a limit on the growth rate of these platforms in a city, a ceiling on surge pricing,Footnote 6 as well as zoning restrictions and congestion pricing. These almost contradictory studies, along with the regulatory efforts and legal challenges backed by municipal and governmental administrations and taxi companies around the world, sparked a debate on the true impact of ridesharing services on urban congestion and the necessity of further regulations and policies.
This section summarizes the arguments and research findings that either claim ridesharing services have helped mitigate congestion and pollution problems or suggest that instead they are contributing to these problems.
8.3.1 Ridesharing as a Solution
The main argument claiming a positive impact from ridesharing services is based upon the notion that modern ridesharing at its core is a way of carpooling or carsharing. Indeed, before the rise of app-based ridesharing services, the concepts of carpooling and ridesharing were almost synonymous. Thus, consistent with the generally positive understanding of the impact of carpoolingFootnote 7 and carsharing,Footnote 8 it was expected that ridesharing services should also provide some savings in vehicle miles traveled (VMT),Footnote 9 CO2 emissions, travel time, and travel/fuel cost.
Not surprisingly, early studies of what we now recognize as app-based ridesharing services were a natural progression from carpooling studies. Most such studies assumed a dynamic ridesharing system in which trips could be coordinated between people with similar itineraries and scheduled on short notice (a few hours to a few minutes in advance) or even in the course of the trip [Reference Gruebele41–Reference Amey, Attanucci and Mishalani45]. For example, Agatz et al. [Reference Agatz, Erera, Martin, Savelsbergh and Wang43] propose an optimization approach for the problem of matching drivers and riders in real-time.Footnote 10 The appearance of the concept of dynamic ridesharing coincided with the rise of app-based services and created a new branch of research in the quest for designing more efficient transportation systems regarding different criteria including congestion.
Another classic body of research on platform-based ridesharing services is the well-known pickup and delivery problem (PDP). In PDP, a single vehicle, or a fleet of them, has to visit pickup locations to pick up goods or people and drop them off at delivery locations while minimizing the total distance or cost of all the routes. PDPs have many different varieties. The dial-a-ride transit service discussed earlier is a special example of this problem. Dynamic one-to-one multiple vehicle PDPs relate directly to ridesharing services. Here, the service is provided by a fleet of vehicles serving customers’ requests that are initiated on a dynamic basis, originated at one location, and destined for another.Footnote 11 Most studies of PDPs focus on logistics and cost minimization rather than on congestion-related aspects. Among the relevant studies, Wang et al. [Reference Wang, Dessouky and Ordonez50] show that in the presence of congestion and high occupancy vehicle (HOV) lanes and existing policies of discounted toll rates on HOVs, taking detours to pick up additional passengers, that is, sharing the ride, and using HOV lanes can reduce the cost of a ride as well as the travel time (thereby reducing congestion and emissions).
The true shared services such as Uber Pool and Lyft Line were built upon this rich literature of PDPs. Uber’s then CEO Travis Kalanick once described Uber Pool as a major evolution of Uber’s business model:
Two people taking a similar route are now taking one car instead of two. And when you chain enough of these rides together, you can imagine a perpetual trip — the driver picks up one customer, then picks up another, then drops one of them off, then picks up another. [Reference Anand51]
Because of this pooling and sharing of rides, initially, there was great hope and expectation for ridesharing services, as a revolution in urban mobility, to reduce congestion in addition to increasing consumer welfare. To analyze this, Li et al. [Reference Li, Hong and Zhang29, Reference Li, Hong and Zhang30] conduct a difference-in-difference (DID) analysisFootnote 12 with travel time index (TTI),Footnote 13 commuter stress index (CSI),Footnote 14 and delay as primary dependent variables to measure the impact of Uber on congestion in 101 urban areas. They conclude that Uber’s entry into these urban areas significantly reduced congestion. Hall et al. [Reference Hall, Palsson and Price52] also use a difference-in-difference analysis on a data set of transit ridership in U.S. metropolitan areas from 2004–2015 to show that Uber has had a complementary effect to public transit, suggesting a potential to reduce congestion, and that its effect on the increase in mass transit ridership grows over time. They also conclude that Uber’s biggest complementary effect is on the public transit system that had the smallest ridership before Uber’s entry. The article, while having a generally positive view on ridesharing services, does not directly study Uber’s impact on congestion but raises a speculative concern that an increase in ridership might lead to increased traffic and suggests that it needs further investigation. Furthermore, a study [2], conducted by the Office of the Mayor of New York City, shows that most of the increase in app-initiated rides in New York City during 2014 and 2015 were substitutions for rides in yellow cabs and thus did not contribute to the observed increase in total VMT (or VHT) and congestion during that period. Similar to the study in New York City, a study using data from Boston [Reference Alexander and González53] found that under moderate to high adoption rate scenarios, ridesharing is likely to result in a noticeable decline in urban traffic and congested travel times. These studies make the case for supporting policies that promote the use of ridesharing services to reduce congestion.
8.3.2 Ridesharing as a Concern
Despite the expected positive effects of ridesharing services on congestion, more recent studies have suggested that there might also be some rebound effects, that is, the reemergence of congestion in another form. These rebound effects include modal shift,Footnote 15 induced traffic,Footnote 16 deadheading,Footnote 17 encouraging car usage, and relocation of people further within metropolitan areas. While it is expected that ridesharing reduces the number of vehicles on the road, as well as total VMT and CO2 emissions, it may also make people more accustomed to car usage and result in mode switching from public transit or other nondriving modes to cars in the short-term. In the long-term, ridesharing could cause people to relocate further away from metropolitan centers, thereby adding to congestion and pollution. It was mentioned in the last section that the New York City’s report [2] exonerated the app-based ridesharing services from contributing to the observed increase in traffic congestion during 2014 and 2015. However, it warned against the potential of rapid growth in e-hailed rides in the future, leading to a decrease in public transportation trips, which in turn would increase total VMT (or VHT). A ride switched from a yellow cab to a ride-hailing service has a chance of being a shared ride; therefore, generally, this type of switch will decrease congestion and pollution. However, a switch from public transportation to ride-hailing would have a detrimental impact on congestion; the report suggests that a switch in less than 1 percent of public transit rides is enough to offset the congestion mitigation earned by an 11–13 percent switch in yellow cab trips.
The studies that suggest ridesharing services contribute to congestion usually focus on one or some of these rebound effects and try to analyze their offsetting or negating impact on the savings gained by shared rides. For example, Yin et al. [Reference Yin, Liu, Coulombel and Viguie54] use an integrated land-use transport model to investigate the environmental impact of carpooling in the Paris region taking into account the impact of rebound effects. They conclude that under long-term scenarios for 2030 the rebound effects of ridesharing decisions will cut the expected CO2 emission savings of carpooling substantially. For short-term scenarios, similar results are obtained by a study [Reference Rayle, Dai, Chan, Cervero and Shaheen31], which conducted an intercept surveyFootnote 18 in three spots in downtown San Francisco during May and June of 2014 mostly concentrated on nighttime and social trips. Among the results are observations about induced travels and transportation mode substitution where passengers were asked if they would make the trip and how would they do it had they not used the ridesharing services. The survey shows that if ridesharing services were not available, about 8 percent of the respondents would not have made the trip. Among the rest, about 39 percent would have taken a taxi, 33 percent would have used public transit, 8 percent would have walked, 2 percent would have used a bike, 8 percent would have used their own car, and 1 percent would have shared a ride with a friend or family member. This means about half of the respondents would have either not taken the trip or would have used a nondriving mode for their trip. This is also confirmed by a recent longitudinal analysisFootnote 19 using monthly transit ridership data from twenty-two transit agencies and four modes of transportation (commuter rail, heavy rail, light rail, and motorbus) across major U.S. cities that shows that in fact, ridesharing services after entering a market draw passenger from heavy rail services by 1.3 percent per year and bus services by 1.7 percent per year [Reference Graehler, Alexander Mucci and Erhardt55].
Similarly, a survey of nearly 1,000 ride-hailing passengers in the Boston metropolitan area in late 2017 [Reference Gehrke, Felix and Reardon56], conducted by Boston’s Metropolitan Area Planning Council (MAPC), finds some concerning facts. It indicates that if ride-hailing services had not been available, approximately 42 percent of passengers would have taken a train or a bus for their trip, 12 percent would have walked or biked, 5 percent would not have made the trip at all, and the remaining 41 percent would have used a personal vehicle or taken a taxi. This means 59 percent of all ride-hailing trips in this survey were contributing to the total VMT and thus overall congestion. The 42 percent substitution from public transit trips is particularly alarming! A similar study [Reference Clewlow and Mishra57] considers seven major U.S. cities from 2014 to 2016. The results show that if TNC services were not available, 49 percent to 61 percent of TNC trips either would not have been made at all or would have been made using a nondriving mode (transit, bike, walk). It also suggests that ridesharing services reduce the ridership from bus and light rail services but have a complementary effect on commuter rail services. Confirming these results, a 2018 study [Reference Schaller58] shows that ridesharing services have added 2.6 new vehicle miles on the road for each mile of personal driving removed, a 160 percent increase in driving on city streets of nine large, densely populated metropolitan areas (New York, Los Angeles, Chicago, Boston, San Francisco, Miami, Philadelphia, Seattle, and Washington DC). In these cities, about 60 percent of users of ridesharing services would have taken public transportation, walked, biked, or not made the trip if these services had not been available, while 40 percent would have used their own car or a taxi. The study suggests that in most cases, the ridesharing services are targeting the same customer base as public transportation, just as the New York City warned in 2015.
There are also other studies that suggest an increase in congestion without delving into the reasons behind it. For example, a report [59] prepared by the London Assembly, names ridesharing services as one of the factors contributing to congestion in London. The report shows that there has been a 70 percent increase in the number of registered ridesharing vehicles between 2012 and 2017. In 2017, private hire vehicles, with a sharp increase since 2013, accounted for 38 percent of total car traffic volume in London’s congestion charging zone; this is roughly double the proportion of taxis, which is a remarkable growth in less than five years for an already congested city. Likewise, Schaller [Reference Schaller60] presents the results of a study on TNC ridership data in New York City from June 2013 to June 2016. Most notably, he shows the following: 1) the introduction of pool options such as Uber Pool and Lyft Line in TNCs helped ridership to grow faster than the number of licensed vehicles (i.e., higher utilization for TNC cars); 2) the net increase in ridership of hire vehicles due to the growth of TNCs in three years was 52 million passengers, 31 million trips, and 600 million miles; and 3) the net mileage increase due to the ridesharing services was 3.5 percent of the city’s total VMT while this percentage of total VMT for Manhattan was more than two times larger. This is a significant increase in VMT in an already congested area.
A similar report about New York City, Shcaller [Reference Schaller61] illustrates a 59 percent increase in hire vehicle hours in the central business district (CBD) between 2013 and 2017. The increase in weekday mileage of these vehicles in the CBD in the same period was lower at 36 percent due to slower vehicle speed – the overall traffic speeds declined by 15 percent. As passenger trips with taxis declined, the trips with ridesharing platforms increased but at a higher pace; the net increase in passenger trips with hire vehicles was 15 percent during this period. It is also notable that the unoccupied hire vehicle hours increased by 81 percent versus a 48 percent increase in occupied hours during these four years. Similarly a study of Denver, Colorado [Reference Henao62] surveys 416 rides of 311 passengers of Uber and Lyft and performs a before-and-after analysis.Footnote 20 Among the various results, it demonstrates that with the introduction of ridesharing services the ratio PMT/VMT has dropped from 112.3 percent to 60.8 percent and the overall VMT increased by 84.6 percent, both suggesting an increase in congestion.
More recently, a 2019 study [Reference Erhardt, Roy, Cooper, Sana, Chen and Castiglione63] claims that TNCs are the biggest contributor to congestion in San Francisco. The study conducted a before-and-after analysis and was based on a data set scraped from the application interface of Uber and Lyft in 2016 and was combined with San Francisco’s travel demand model, SF-CHAMP, to control for background factors that may also impact congestion. The study shows that the average speed decreased from 25.6 miles per hour (mph) in 2010 to 22.2 mph in 2016 and that the vehicle hours of delay (VHD) increased by 63 percent over the same period. A 2020 study in Santiago, Chile [Reference Tirachini and Gomez-Lobo64] uses a Monte Carlo simulation method and shows that ride-hailing services increase the total vehicle kilometers traveled (VKT) unless the average occupancy rate is significantly increased by actually sharing/pooling the rides.
All these studies were conducted while the ridesharing services were present in the regions of study. Among them, some relied on a before-and-after comparison to obtain a better understanding of their impact on congestion after they entered those regions. However, even such before-and-after analyses may not be adequately conclusive as the comparison is done over a long period, usually several years. A very recent study [Reference Agarwal, Mani and Telang65] takes an interesting and different approach, and yet suggests similar conclusions. It focuses on the city-wide strikes by drivers for these services as exogenous shocks in three major Indian cities (Mumbai, New Delhi, and Bangalore). It implements a regression analysis to study the impact of ride-hailing services on traffic congestion. It suggests the conclusion that congestion was reduced during these strikes as much as 40–53 percent of the reduction observed during a typical holiday. It also reports a public transport substitution effect suggesting that ride-hailing services were drawing passengers from mass transit.
8.3.3 Analysis of the Discrepancies in the Results
As has been demonstrated in the previous sections, the existing studies on the question of whether TNCs contribute to congestion have conflicting results. Because these studies are mostly empirical, statistical, or simulation-based, they naturally inherit some degree of uncertainty due to the methodologies. However, as the remainder of this section discusses, several avoidable factors are contributing to the inconsistency of the results.
Missing Factors: The studies discussed earlier are not always comprehensive in terms of the factors they consider. For example, in Schaller’s report [Reference Schaller61], a fraction of the reported 15 percent net increase in passenger trips with hire vehicles could be associated with other factors such as population growth, replacement of personal car usage, and deadheading. Although it is clear from the metrics used in this report alongside those in his earlier report [Reference Schaller60] that ridesharing services had contributed to the overall traffic volume in the CBD during 2013–2017, the magnitude of this contribution could be different if one would include those factors in the study. The New York City’s report [2], on the other hand, accounts for the TNC rides that are replacements of the use of private vehicles, but this report also does not account for the population growth or deadheading. The exclusion of certain critical factors from these studies could be the source of some of the disparities between the results.
The Scale of Truly Shared Rides: Another factor that leads to inconsistent results is the misunderstanding of the true scale of sharing in different cities. An advocate of ridesharing services may argue that the studies that view ridesharing services as a source of congestion are not fully capturing the full effect of sharing rides, and if we properly account for all shared and pooled trips the conclusion might be different. However, according to a recently published data set [66], on average, only about 13 percent of Uber rides in New York City in 2019 were actually shared; the number for Lyft was 24.7 percent. Figure 8.4 shows the monthly percentage of shared trips in New York City for Uber and Lyft – as the two currently dominant ride-hailing companies there. The charts show that the maximum percentage has never exceeded 30 percent for either of them (28.6 percent for Uber and 28.5 percent for Lyft). They also show a divergence between the two service providers which could be due to their different ride options and pricing schemes. The fact that Uber’s percentage is declining is a matter of concern as Uber had more than three times as many rides as Lyft in the same period. The weighted average of their combined percentages of shared trips in 2019 was just 15.7 percent of all trips. Misunderstanding the real scale of shared rides in the ridesharing services, which may lead to wrong assumptions, or missing to control for this factor can explain some of the inconsistencies between the results in different studies.

Figure 8.4 Percentage of shared trips in New York City for (a) Uber, and (b) Lyft.
Adequacy of Data: Availability of data, the type of available data, and the sample size can have an enormous impact on the way these studies are conducted and on their results. For instance, the data used by Erhardt et al. [Reference Erhardt, Roy, Cooper, Sana, Chen and Castiglione63] does not capture shared trips, and in any sequence of pickups and drop-offs, it only takes into account the first pickup and the last drop-off and none of the stops in between. Moreover, the first pickup location is not exactly the passenger’s location but rather the point at which the driver accepts the new order. This is because the data were collected by a tracing system that would only track out-of-service TNC vehicles. The study’s dependence on this data set may skew the results towards the conclusion that ridesharing services contribute to congestion, as it leaves the main congestion reducing force of these services, namely shared rides, out of the analysis.
Choice of Congestion Metric: It appears that the choice of the congestion metric has a big impact on the result of the study. For example, TTI and CSI are used by Li et al. in [Reference Li, Hong and Zhang29, Reference Li, Hong and Zhang30]. Both TTI and CSI are very good at measuring commuting-related congestion but may not be accurate measures for the overall ridesharing-related congestion observed in a city as they are merely based on two snapshots of peak time and free-flow time. As another example, Clewlow et al. [Reference Clewlow and Mishra57] mention that 49 percent to 61 percent of “trips” would have been either avoided or made by a nondriving mode such as walking, biking, or transit. However, it does not study the translation of this increase in the number of car-based trips into actual additional VMT or VHT, thus making it hard to realize the significance of this change.Footnote 21
Availability of Services: Availability and the existing usage levels of public transit, carpooling, carsharing, and shared bikes in a city might have a considerable impact on the type of service that riders would receive from TNCs. For example, a high level of carpooling adoption in a city may shift TNC rides more towards nonshared ones. Consider two studies focusing on two cities, one with a high level and another with a low level of public transit and carpooling. Assuming that there are no modal shifts, the rides in the latter are expected to have a higher percentage of shared rides. This combined with a choice of congestion metric like the ratio PMT/VMT could give us inconsistent conclusions on the congestion contribution of ridesharing services in these two studies. This would also lead us to the next attribute, that is, the spatial differences of these studies.
Spatial Differences: Most existing studies are limited locally while making conclusions globally. A local study may not give us the overall picture of the impact on traffic congestion in a country. Further and more comprehensive studies would be required to find out the global impact of ridesharing services on congestion. However, when making policies, as will be discussed more in the next section, it is generally better to rely on local studies with local conclusions. The results from a dense and populous city may not translate to a small town or vice versa, as both supply and demand sides of a ridesharing market in a large city are vastly different from that of a small city. The number of active ridesharing services in a city and the number and percentage of people using them can have a tremendous impact on the number of shared rides. Moreover, many other factors such as accessibility of public transit, demographics, and geography also play a role and force policymakers to rely mostly on local data and analyses. Therefore, the locational differences in cities that were the subject of the existing studies could help explain the difference in their results.
Temporal Differences: Similarly, collecting data or surveying trips at different times of the day or different days of the week may skew the analysis. For instance, the intercept survey used by Rayle at al. [Reference Rayle, Dai, Chan, Cervero and Shaheen31] is heavily weighted towards Downtown San Francisco’s social hot spots, where parking for a personal vehicle is a big problem, and in the evening when public transit is less frequent and people are more inclined towards taking a TNC vehicle to avoid driving under the influence. Furthermore, the effect of TNCs on congestion could vastly differ from one year to another. Consider four studies that rely on data from the following periods (as some of the studies discussed above do): 2014,Footnote 22 2014–2015, 2015–2016, and 2016. One should not expect consistent results from these four studies as the industry evolved dramatically during this period. For example, New York City’s report [2] suggests that TNCs did not contribute to the observed increase in congestion in New York City during 2014 to 2015 but could contribute to congestion in a significant way in the future if they draw passengers from public transportation. One year later, Schaller [Reference Schaller60] suggests that the net mileage increase due to the ridesharing services from 2013 to 2016 is 3.5 percent of the city’s total VMT. The exponential growth of TNC services during 2015–2016 could explain these seemingly different results. When the underlying subject is doubling in size every year, it would not be surprising if these studies come to different conclusions.
8.4 Policy Measures to Reduce Congestion
As mentioned in the previous section, the answer to the question of whether ridesharing services have a positive or negative impact on congestion depends on the location and the time. If at the local level in a certain region and a certain period a negative impact is observed, it is necessary to devise policy measures for controlling or mitigating this impact. This section reviews some of these measures, focusing just on policy responses that can directly mitigate congestion caused by ridesharing services. Despite some overlaps, other sources of traffic congestion may require different measures.
Infrastructure Expansion: Adding more roads, tunnels, bridges, subway/bus lines, and bike lanes can provide a long-term solution for the congestion problems caused by ridesharing services, as can expansion of the city towards the outskirts. However, these options require an increase in taxation to finance infrastructure projects, which is not always politically popular. Also, in many cases cities may face geographic barriers for expansion, and even if the expansion is possible it may not resolve the congestion issue in downtown areas and business districts. This pushes the cities to rely on more short-term solutions such as designing restricted traffic zones and congestion pricing [Reference Santora67, 68].
Restricted Traffic Zones: Restricted/limited traffic zones (RTZs or LTZs) are areas within a city where entry to these zones requires permission and may be subject to a fee. Entry to such zones could also be limited to certain hours of the day or certain days of the week, or for certain types of vehicles or groups of people (such as residents, public workers, and disabled motorists). LTZs are very common in Italian cities such as Rome, Florence, and Milan. In many cases, LTZs are complemented with either pollution charges or congestion charges. Odd-Even Zones (OEZs) apply similar restrictions to odd or even license-plate numbers during the weekdays. Tehran and Beijing are notable examples of the implementation of this policy. Tehran is particularly interesting, as its use of this practice dates back to late 1979 and it implements concentric layers of OEZs and RTZs together [Reference Salarvandian, Dijst and Helbich69].
Zero-emission Vehicles Zones and Pollution Charges: To promote electric and environmentally clean vehicles in congested urban areas, cities can design zero-emission zones (ZEZs) or low-emission zones (LEZs) in which only electric vehicles or ultra-low emission vehicles could travel. An alternative for cities with medium congestion or pollution levels is to impose a pollution charge in specific areas of the city on vehicles that fail to meet certain standards. This would reduce pollution as well as the traffic volume in such areas. Milan’s Ecopass System [Reference Martino70] is a prime example of this policy.
Congestion Pricing: Congestion pricing is the practice of charging a flat or variable rate fee to vehicles that drive in a specified zone within a city to reduce traffic and pollution in that zone. There are multiple successful cases of this policy around the world. A report prepared by the Center for City Solutions [71] reviews the results of congestion pricing in Singapore, London, and Stockholm. With its Area Licensing Scheme (ALS) designed in 1973, and updated with an Electronic Road Pricing (ERP) system in 1998, Singapore is perhaps one of the leading cities in the world in designing a congestion pricing scheme to solve traffic issues caused by rapid economic development and geographic restrictions. Singapore’s congestion pricing scheme resulted in a 24 percent decrease in inner city traffic, 6 mph increase in average vehicle speeds, 15 percent increase in public transit ridership, and 10–15 percent reduction in greenhouse gas emissions in the inner city. In London, congestion pricing resulted in a 9.9 percent reduction in traffic between 2000 and 2015 despite a 20 percent increase in population; it also added 30 percent to average vehicle speeds and 8.5 percent to the city’s transportation revenue. In Stockholm, despite population growth, the traffic volume decreased by 22 percent, VMT declined by 16 percent and 5percent for the inner and outer city respectively, and the government had net earnings of $143 million per year. Milan’s Area C program is another successful example of congestion pricing that within two months of implementation delivered a 36 percent decline in commercial and private traffic, a 50 percent decrease in accidents, an 11 percent increase in average vehicle speed, and a 24–45 percent cut in greenhouse gas emissions [Reference Martino72]. Despite the lack of a successful case in the United States, the concept of congestion pricing or zone pricing is not new to U.S. cities, as there have been multiple attempts to establish such systems in cities such as New York and San Francisco [Reference Elinson73]. New York is set to be the first city in the United States to adopt a congestion pricing policy. In 2017, Governor Andrew Cuomo announced his plan to impose a fare on traffic in Manhattan’s CBD (south of 60th street) to both reduce traffic and raise funding to fix New York City’s failing public transit system [Reference Santora67]. A similar attempt in 2008 led by Mayor Michael Bloomberg to charge $8 on entries to the most congested parts of Manhattan failed to gain the support of other boroughs [Reference Confessore74]. The new three-phase plan was finally approved by the state in 2019 [Reference Durkin and Artauni75] and the first two phases are already implemented, including $2–5 per trip surcharges for for-hire vehicles in the congestion zone. The third phase, which involves congestion pricing for all entries to the CBD, was scheduled to be implemented in January 2021. It is now scheduled for late 2023. If the initial estimates of entry fees – $11.52 for cars and $25.34 for trucks [Reference Sankar76] – are implemented, it is expected that the annual revenue from the plan will exceed $1 billion, which could be spent for the revival of public transit infrastructure. The plan’s third phase is pending approval from the Federal Highway Administration (FHA) [Reference Berger77]. However, it is unclear how much this program would reduce the congestion caused by TNCs in Manhattan, as about half of their trips in the CBD zone start and end within that zone [Reference Schaller60].
Capping Number of Hire Vehicles and Limiting Zones of Operation: In some cities, limitations on the number of taxicabs and their operation areas are already in place. For example, in New York City, restrictions on the number of yellow and green (Boro) taxicabs and service zone limitations for green cabs have been in place for many years. Green cabs are only allowed to operate in upper Manhattan and the outer boroughs [Reference Mann78]. This helps cities in many ways, including by ensuring equity and access to reliable transportation in underserved areas of the city, facilitating fair competition and leveling playing fields, making the urban transportation sector economically sustainable while keeping fares affordable, and mitigating congestion in certain areas. Similar measures can be applied to ridesharing services by defining areas of operation for each TNC or putting a cap on the number of TNC vehicles in the entire city or certain areas within the city.
Learning from the Popularity of TNCs: It might be the easiest approach for policymakers to suppress TNCs with different measures or to impose outright bans on them to mitigate the congestion caused by them. However, policy choices ultimately boil down to satisfying customers’ needs one way or another. As mentioned in the historical review section, water wherries would have had a better outcome if they had focused on improving their service rather than fighting with hackney coaches. This is why the National Association of City Transportation Officials recommends encouraging taxi companies to adopt new technologies for staying competitive [79]. Focusing on the reasons that made platform-based ridesharing services very popular and mimicking them in other ride services such as taxis, buses, and trains can immensely improve the quality of rides passengers receive from those services. These reasons include, but are not limited to, providing additional information to the rider on the app and thus reducing uncertainties, enhancing the convenience of paying fares, providing broader spatial and temporal access to TNC vehicles, charging attractive prices relative to the convenience of the trip, reducing wait times, increasing the convenience of leaving a review for the ride experience, and more generally satisfying a younger and more technology-friendly generation by providing a more technology-friendly experience. For example, the additional information provided by a TNC app, such as GPS data, origin–destination route, estimated time of arrival, and travel time, can significantly reduce the uncertainties of a trip. One could imagine the stress and anxiety associated with making an appointment and relying on public transportation without too much buffer time. City transit authorities can incorporate many of these features into other modes of transportation, making them more popular and efficient. This can particularly reduce the number of riders that switch from public transport (the least convenient experience) to TNCs (the most convenient experience), thereby mitigating congestion.
8.5 Research Gaps & Future Directions
A complete answer about the impact of ridesharing services on traffic congestion requires more comprehensive, multifaceted, multidisciplinary research. This section discusses some of the research gaps, opportunities, and directions for further investigation of this question.Footnote 23
Traffic Zoning: One important research opportunity concerns the design of optimal RTZs, OEZs, ZEZs, and LEZs. For example, there is a lack of research on the use of geographic optimization methods to reduce congestion. Computational geometric approaches when combined with optimization methods could be very helpful to policymakers in designing such zones in a city and solving the related utility optimization problems. Geographic optimization methods can find the optimal boundaries of the zones and the optimal pricing for permit fees in each zone and can balance the traffic between the zones.Footnote 24
Congestion Pricing: If New York City’s congestion pricing plan goes into full implementation, it may soon be followed by San Francisco, Los Angeles, Chicago, and other big cities in the United States. This provides a research opportunity for helping especially urban policymakers to find the optimal entry fees and surcharges for ridesharing services as a mechanism to control traffic volumes generated by these services.Footnote 25
Micromobility Services: One of the policy measures discussed in the last section was the expansion of transportation infrastructure, which includes bike lanes and shared bike terminals. This could be generalized to almost all micromobility services. City bikes and shared electric scooters can be efficient and green alternatives to private cars and ridesharing services, despite shortcomings such as the seasonal nature of these options. Their relatively low costs (in both initial investment and usage fare), high accessibility, and ease of use makes them strong competitors to the currently dominant modes of urban mobility. The significance of these services can also be seen in the rapid growth of micromobility companies such as Lime and Bird in the last three years and the recent focus of ridesharing companies such as Uber and Lyft on offering these services. However, many cities are not ready for this new trend: Public bike-sharing stations and the allocation and reallocation of bikes between the terminals are not well-optimized; many cities do not have an adequate number of dedicated lanes for bikes and scooters; and scooter businesses are not regulated. A comprehensive and interdisciplinary study of micromobility systems is necessary and urgent. This necessity and urgency can be seen by, for example, the big surprise and chaos that communities, city officials, and transportation authorities faced by the sudden emergence of electric scooters in the technology-friendly cities of San Francisco and Los Angeles [Reference Matthews82, Reference Newberry83].
Elimination of Cruising for Parking: An aspect missing in current studies on the impact of ridesharing on congestion is the impact of cruising for parking by personal vehicles. As Shoup [Reference Shoup84] suggests, on average and over the long term, approximately 30 percent of traffic is due to such cruising. If a TNC trip replaces a trip that would have been made by a personal vehicle, it not only replaces the personal vehicle mileage for the distance between the origin and destination of the trip but also removes the potential need to cruise for parking and its additional VMT (VHT). This could reduce congestion and thus warrants further study.
Self-driving/Autonomous Vehicles: It is notoriously difficult to achieve an equilibrium in a dynamically changing two-sided market and even harder to maintain it. Any such equilibrium state will be short-lived, as the supply (drivers) and demand (riders) are steadily changing. It will also be very sensitive to any change in the decision-making parameters such as ride fare, waiting time, and travel distance. Moreover, the whole system is also prone to short- and long-term exogenous events such as sports events, gas prices, local mass layoffs, and new regulations. Therefore, the platforms inevitably have to move towards reducing the uncertainty on the side they have more control over, which is the supply side. This leaves TNCs with three options: 1) using significant incentives to make the supply side more predictable; 2) hiring a fraction of drivers as employees with a predetermined working schedule, adding a layer of certainty to the supply side; or 3) deploying a fleet of self-driving/autonomous cars to constitute a fraction of the supply. The first option is currently being implemented with much difficulty and very little success for a variety of reasons including competition with other platforms that may provide better incentives or the unpredictability of human behavior when faced with incentives. The second option is unlikely to be followed, as the currently active ridesharing platforms such as Uber and Lyft have gone through many legal challenges to avoid the costs of treating their drivers as employees. However, the third option appears to be promising. A simulation model by Fagnant and Kockelman [Reference Fagnant and Kockelman85] shows that each shared autonomous vehicle can remove up to 11 conventional vehicles from the streets while adding only up to 10 percent to the VMT due to more deadheading. This could simultaneously make the planning easier for TNCs and mitigate the congestion issue for cities,Footnote 26 More studies are required to better understand the magnitude of its mitigating impact on congestion.
Large Cities versus Small Cities: Due to network effects and the large population in major cities, it is expected that a significant number of people use ridesharing services just because people in their social network use them and not necessarily out of a need. This could have an immense impact on congestion. Moreover, due to the large market size in big cities, competitor companies have more time to enter the market and gain a share after the first TNC’s entry and to enjoy the network effect in their growth. Soon there will be several TNCs active in the city competing with each other in a race to the bottom by providing more and more incentives for the drivers. This may lead to an oversupply of drivers deadheading or offering very cheap fares to customers, thereby encouraging mode switches and causing induced traffic. Ultimately, these effects could contribute to congestion from both sides of the market. In contrast, small cities are more likely to have a monopoly or something close to that as the first TNC can grab the entire market quickly after its entry, making it extremely hard for any competitor to enter that market or efficiently compete there. Any new competitor would have to reach a critical mass or a significant number of drivers and riders to remain operationally sustainable. A monopoly in the ridesharing market in small cities could lower the congestion by reaching equilibrium more efficiently and increasing the number of shared trips. A game-theoretic approach might be fruitful in analyzing these two different situations.
Impact of E-commerce on Traffic Congestion: A meaningful portion of the recent increase in congestion observed in metropolitan areas could be due to the massive increase in the movement of commercial trucks after the boom in e-commerce. It is worth investigating the impact of e-commerce on traffic congestion. It appears to have a sizable impact (maybe bigger than that of ridesharing) on congestion, especially during the COVID-19 pandemic as people tended to do most of their shopping online. There has been very little attention in the literature to control for this significant factor. Studying the congestion impact of ridesharing services and e-commerce activities together could be very valuable, leading to more accurate conclusions.
Integrated Urban Mobility Systems: It is notable that the complementary effect that ridesharing services have on public transit, by improving first and last mile access especially for the longer distance services such as commuter rail [Reference Stiglic, Agatz, Savelsbergh and Gradisar86], has not gained much research attention. A similar statement can be made about the impact of ridesharing services on parking spaces. To prevent ridesharing services from taking public transit’s market share, we may find interesting solutions in measures such as integration of ridesharing databases with that of public transit, putting a quota for each ridesharing company or a limit for all of them combined, data-driven and geographic-based pricing, and taxation of rides originating in the vicinity of transit routes excluding those trips to or from a transit station. An interdisciplinary approach to develop a framework, along with models and methodological tools for analyzing problems arising in this field, could be a powerful approach to tackling this and other similar research questions, leading to more effective policies.
Comprehensive Study: A negative externality such as congestion (pollution) is just a small piece of a big puzzle. Policymaking in this area requires a holistic view. No matter how harmful ridesharing might be in one aspect, such as congestion, it may have greater benefits for society in other aspects. For example, a collaborative study between Uber, the University of Oxford, and the University of Chicago [Reference Cohen, Hahn, Hall, Levitt and Metcalfe87] shows that in 2015 Uber X in San Francisco, Los Angeles, New York, and Chicago rendered a total of $2.9 billion in consumer surplus. More independent research is needed to understand the overall socioeconomic impact of ridesharing services better.
It would be ideal if future research could address the root causes of traffic congestion by designing comprehensive studies that control for more factors, ensure the adequacy of data, compare the most suitable (and possibly multiple) congestion metrics, and take into account the spatial and temporal differences.
Acknowledgment
The author acknowledges that the charts in Figure 8.1 are used with permission of the City of New York.Footnote 27
9.1 Introduction
The last few years have seen a tremendous growth of mobility companies, referred to as Transportation Network Companies (TNCs), such as Uber, Lyft, and Via, that have introduced a variety of on-demand services. TNCs have grown exponentially. It took Uber six years to reach its first billion rides but only six months to reach the next billion [Reference Schaller1].
The popularity comes with concerns about the impact of these services on congestion and traffic conditions in general. In 2018, there were 42,201,375 TNC rides starting in the Boston municipality, with an average of 68.3 rides per habitant. According to the Massachusetts Department of Public Utilities (DPU), rides increased by 21 percent from 2017 [2]. The San Francisco County Transportation Authority (SFCTA) reports that TNCs were responsible for half of the increase in congestion in San Francisco from 2010 to 2016 (while employment and population growth contributed the other half). The report also finds that TNC trips account for an estimated 25 percent of the total congestion in the city and 36 percent of delays in the downtown area. On a typical day, they add 170,000 vehicle trips and more than 570,000 vehicle miles traveled (VMT) (20 percent of all local daily VMT). TNCs contribute to congestion at all times of the day, especially in the evenings [3, 4]. Furthermore, in general, the fraction of rides that are actually shared is small, meaning that TNC services are operating, in principle, as taxi services with a ride arranged through apps. This actually adds extra mileage rather than reducing traffic, considering the mileage driving to pick up passengers. Schaller [Reference Schaller1] reported that the non-shared ride TNC services (UberX, Lyft) put 2.8 new vehicle miles on the road for each mile of personal driving removed, for an overall 180 percent increase. The increased congestion brings other negative externalities as well, for example, reduced safety. According to a study [Reference Barrios, Hochberg and Yi5], the rise of TNC services has increased traffic deaths by 2–3 percent in the United States since 2011, equivalent to as many as 1,100 fatalities a year.
TNC services also compete with sustainable modes of travel such as public transport, walking, and biking, while they are, in general, less competitive with personal automobiles. The main factors impacting mode-choice, such as price, speed, convenience, and comfort, result in shifting passengers to TNCs from public transport and nonmotorized modes rather than cars. Many surveys show that if TNC services were to disappear, about 60 percent of current TNC users would switch to public transport, walking, and biking (or not make the trip), about 20 percent would use their own car, and 20 percent would use a taxi [Reference Schaller1]. Many traditional public transport services have been recently experiencing a reduction in ridership, especially buses. This decline is partially attributed to direct competition from TNCs [6]. The Chicago Transit Authority (CTA), for example, is reporting that the decline in ridership is partly caused by competition from TNCs, like Uber and Lyft. Equally alarming is the decline in student ridership. The Metropolitan Transportation Authority (MTA) in New York reported a 12.7 percent decline in student ridership in buses in 2018 [7].
Despite their popularity and large market, on-demand mobility services are far from profitable. Uber reported an operating loss of $8.5 billion in 2019 after losing more than $3 billion the previous year [8]. This lack of profitability seems to be a characteristic of the on-demand mobility service industry. Currie and Fournier [Reference Currie and Fournier9] compiled a database of 120 systems, including traditional dial-a-ride, demand-responsive transit (DRT), and Microtransit, from nineteen countries since the 1970s. They found that most of the systems eventually failed (for example, 67 percent in the United Kingdom), and 40 percent lasted fewer than three years. High operating costs are the main contributor to their failure. The use of new technologies, such as apps and the mobile internet that enabled the recent developments, has not helped, with profitability, at least not yet. Results also show that services with simpler operations (for example, “many-to-few” systems, where trips may have many [any] origin locations but all go to one or very few destinations and vice-versa providing economies of scale) have lower failure rates, compared to the complex all-to-all services (where requests can have any origin location and can go to any destination location). Enoch et al. [Reference Enoch, Potter, Parkhurst and Smith10] also concluded that systems are often not properly designed and there is a tendency to offer too much flexibility, which increases costs.
Ride-pooling is a strategy that can address all of these concerns, regarding societal impacts (on congestion, traffic, and competition with public transport) and TNC profitability (operating costs and overall efficiency). Using taxi-trip data from New York City, for example, Santi et al. [Reference Santi, Resta, Szell, Sobolevsky, Strogatz and Ratti11] concluded that even if trips are shared by only two passengers, a significant reduction in total VMT can be achieved in dense metropolitan areas, such as Manhattan. Alonso-Mora et al. [Reference Alonso-Mora, Samaranayake, Wallar, Frazzoli and Rus12] showed that, if all trips are shared, 25 percent of active taxis in NYC can satisfy 98 percent of the ride requests with an average waiting time of 2.8 minutes and mean trip delay of 3.5 minutes. This represents a significant reduction in required fleet size and hence, improvement in efficiency.
Because of the potential of ride-pooling to improve operating efficiency and, at the same time, reduce the impact of TNCs on congestion, experts have begun developing approaches to increase the number of shared trips. These approaches include alternative operating models on one hand, and long-term strategic partnerships with other service providers, such as public transport operators, on the other, which can result in simpler operations and economies of scale [Reference Enoch, Potter, Parkhurst and Smith10].
This chapter discusses opportunities for on-demand mobility services to improve sharing performance as a means to improve not only operating efficiency but also environmental sustainability. It explores, empirically through a large TNC dataset, the potential of these approaches to reduce on-demand mobility impacts, especially from a sustainability point of view using metrics such as VMT (a good surrogate of congestion and environmental impacts). It examines the impact of various factors (such as the fraction of requests known in advance, the percentage of shared requests, and the level of service expectations). It also reports on the experience with field tests and other experiments of coordinated public transport/TNC services and highlights lessons learned.
9.2 Background and Definitions
The concept of on-demand transportation services is not new. The first experiments were carried out in Atlantic City, New Jersey, in 1916 and some form of on-demand, shared-taxicab services have existed in US communities since at least the 1930s. By 1974 there were approximately twenty systems operating in North America, often referred to as dial-a-ride, demand-responsive, and paratransit systems [Reference Kirby, Bhatt, Kemp, McGillivray and Wohl13] used to complement regular transit services. Dial-a-ride was introduced as a form of shared transport where passengers make reservations (typically a day in advance). Requests are organized in itineraries satisfying a certain level of service constraints (for example, maximum wait time from desired departure time). Vehicles do not have a fixed route or timetable. Systematic research into dial-a-ride started in the 1960s by researchers at the General Motors Research Laboratories, Massachusetts Institute of Technology (MIT), and Northwestern University. The federally funded project Computer-Aided Routing System (CARS) at MIT focused on all (operating) aspects of dial-a-ride from all points of view, including the development of computerized algorithms for optimal routing and scheduling [Reference Strobel14]. Wilson et al. [Reference Wilson, Weissberg and Hauser15] were among the first to explore the potential of computers to plan and control dial-a-ride systems. Their efforts resulted in algorithms to assign requests to the most appropriate vehicles. They also investigated the problem of integrated dial-a-ride and fixed route public transport services and coordinated dial-a-ride systems. They aimed to design effective hybrid systems, in which dial-a-ride serves low volume and short trips, fixed-route public transport serves high volume trips, and a coordinated fixed-route/dial-a-ride system serves long but low-volume trips [Reference Wilson, Weissberg and Hauser15]. Today dial-a-ride, referred to as paratransit or demand responsive, services are mainly offered by public transport agencies in order to comply with the 1990 Americans with Disabilities Act (ADA). Qualified individuals can make reservations through a centralized system to use the service to access medical facilities as well as locations of other activities.
Today’s mobility on-demand services (for example, Uber, Lyft, Via) are fundamentally app-based dial-a-ride services with centralized dispatching and flexible driver arrangements [Reference Currie and Fournier9], with most of the requests placed in real-time. These new services appear under various names; however, the terminology used to define them is often inconsistent. For this discussion, we mainly use the terminology introduced by the Society of Automotive Engineers, SAE [16] as summarized by:
1. Shared mobility refers to the shared use of a vehicle, scooter, bicycle, or other travel modes. Users have short-term access to the travel mode on an as-needed basis [Reference Pangbourne, Meyer and Shaheen17, Reference Cohen and Shaheen18]
2. TNC services (also called ridesourcing or ridehailing services) are prearranged or on-demand transportation services for compensation, in which drivers and passengers connect via digital apps that support booking, electronic payment, and ratings of the services.
3. Ridesharing is the formal or informal sharing of rides between drivers and passengers with similar origin-destination pairs. Ridesharing may include carpooling and vanpooling, where several passengers share the cost of using a vehicle, and in some cases, driving responsibility.
4. Ride-pooling, also known as shared TNC services that are organized on-demand, enables people to share a vehicle ride with others. UberPool, UberExpressPool, and Lyft Line are examples of ride-pooling services [19].
5. Microtransit is a privately or publicly operated, technology-enabled public transport service, that typically uses vans to provide on-demand or fixed-schedule services with either dynamic or fixed routing.
6. Demand-responsive transit (DRT), also known as demand responsive transport, flexible transport, or Dial-a-Ride Transit (DART), is a form of public transport where vehicles can alter their routes based on demand rather than using a fixed route or timetable [Reference Brake, Nelson and Wright20].
9.3 Increasing Shareability
Recognizing the need for and potential of ride-pooling, TNCs are adjusting their technology and operating models to deliver more shared rides. They increasingly focus on ways to promote ride-pooling, with services such as UberPool, UberExpress, and Lyftline, offered at reduced prices. In the first two months of LyftLine’s service in San Francisco, one-third of all Lyft rides were LyftLines [Reference Nagy21]. Lyft recently redesigned its app and is developing strategies to improve ride pooling [22]. New operational models to increase ride-pooling opportunities have also been proposed in the literature, such as meeting points at origins and destinations [Reference Stiglic, Agatz, Savelsbergh and Gradisar23] and transfer points to switch vehicles [Reference Masoud and Jayakrishnan24].
Studies in the literature also show that coordination and integration of TNC operations and regular public transport services [25–28] has the potential to be beneficial to both parties involved. For example, Fan and Zhang [Reference Fan and Zhang28] concluded that there are financial benefits in the integrated operation of public transport and shared mobility services in Santa Clara, through increased economies of scale. As a result, not only does TNC operating efficiency improve, but also there is a positive impact on the demand for public transport. There is strong evidence that public transport agencies and TNCs are interested in exploring the coordination between public transport and TNCs. Uber and Lyft have recently added public transport directions and fares to their apps [Reference Blumenthal29, Reference Hawkins30]. In Denver, riders can purchase public transport tickets via the Uber app [Reference Bussewitz31]. Google has plans to show in Google map the multimodal trip options that combine public transport and TNCs [Reference Carrasqueira32]. The service already provides directions to walk or drive to public transport. By adding on-demand services, the app can also support the option of using a ride to a public transport station. At the same time, various public transport agencies, such as the Greater Richmond Transit Company, are pursuing an integrated design of public transport and TNC services that can be mutually beneficial [Reference Goffman33].
Although TNCs have been heavily investing in improving and promoting their shared services, such as UberPool, ExpressPool, and LyftLine, the majority of trips are actually singly served. For example, Uber reported that only 20 percent of Uber trips are shared/pooled trips in the major cities where UberPool service is provided. Lyft estimates that 37 percent of the users in cities with a LyftLine option request a LyftLine trip, but the actual rides being shared is substantially lower (22 percent in New York City in February 2018) [Reference Schaller1]. Even in a shared ride, some portion of the trip may involve just one passenger (for example, between the first and second pick-up).
Several studies explore the factors impacting the potential for shared trips using real-world data. Tachet et al. [Reference Tachet, Sagarra, Santi, Resta, Szell, Strogatz and Ratti34] present a simple index that measures the potential for ride-pooling as a function of trip generation rates, the amount of trip delay time that is acceptable to passengers, average traffic speed, and city size. They show that various metropolitan areas (New York, San Francisco, Singapore, and Vienna) exhibit similar behavior in terms of shareability potential, indicating that the same operating strategies to increase shared rides could work in different metropolitan areas.
In general, the factors impacting the ability of ride-pooling services to attract and match ride pooling requests are both demand and supply related. Demand-related factors include customers’ willingness to share (demand for ride-pooling) and level of service (LOS) expectations (extra ride time, waiting for a vehicle, etc.). They also include spatiotemporal distribution of requests, which determines the opportunity to match ride pooling requests. Supply-related factors include market fragmentation (various mobility service providers operating independently), which impacts the sharing of information and service resources (leading to inability to match requests across TNCs). They also include operating strategies and partnerships to facilitate ride-pooling and take advantage of the available opportunities for pooling requests to the full extent (request matching, vehicle dispatching and rebalancing, etc.). The remainder of this section reviews these factors.
9.3.1 Customer Willingness to Share and LOS Expectations
Passenger willingness to share and tolerance for increased waiting and trip detour times (additional time due to sharing a trip compared to a direct trip) affect ride-pooling opportunities [Reference Santi, Resta, Szell, Sobolevsky, Strogatz and Ratti11, Reference Alonso-Mora, Samaranayake, Wallar, Frazzoli and Rus12, Reference Tachet, Sagarra, Santi, Resta, Szell, Strogatz and Ratti34–Reference Alonso-Mora, Wallar and Rus35]. While several existing studies explore the factors affecting the adoption of TNC services and the frequency of use [36–39], studies on the types of TNC services customers use and the factors impacting these choices are rather limited. In a recent TNC survey [Reference Zhou, Ma, Hirschmann and Lao40], respondents were asked about their TNC use frequency, the characteristics of their most recent TNC trip, and their willingness to share rides with others, wait for service, walk to a pick-up/drop-off location, and place requests in advance. The results show that 54 percent of the respondents prefer using the pool/shared services (for example, UberPool, LyftLine). Of the responders, 83 percent indicated that they would choose to walk to/from a pick-up/drop-off location for a discount, with 57 percent willing to walk more than 5 minutes. Furthermore, 75 percent of the respondents would also place requests in advance (at least 15 minutes ahead of their desired departure time). Users with higher income, age, and bike and car ownership tend to use TNC services less frequently. They also use pool services less. Lower-income, age, and no bike/car ownership groups, as well as groups with membership in sharing services (car/bike sharing), use TNCs more and also preferred pool services more than other groups [Reference Zhou, Ma, Hirschmann and Lao40].
9.3.2 Spatiotemporal Distribution of Requests
Current ride-pooling systems aim at pooling passengers with similar origin-destinations (OD) and departure times. In addition to the complexities of assigning a vehicle to several requests and designing a route plan that serves them most efficiently, pooling of trips with similar OD pairs and time frames, at its core, suffers from the spatiotemporal sparseness of real-time requests that could actually be matched [Reference Schaller1]. The spatiotemporal distribution of requests for shared rides and their destinations determines the potential that two or more requests could be matched and served efficiently by a single vehicle, given their pickup/drop-off time windows and locations. Requests with similar paths and time windows can be assigned to the same vehicle. Trip origins and destinations are generally spread over numerous OD pairs which makes it difficult to find passengers who have compatible OD pairs. Moreover, potential ride-pooling passengers should also have compatible departure times. The likelihood of finding passengers who are willing to take a shared ride and are heading in the same direction at the same time is rather low and has hindered the effectiveness of real-time ride-pooling. To increase the likelihood of shared rides, the radius of matching passenger origins or destinations could be increased, or the time window of the departure time could be more flexible. In such cases the scheduling of the trips has to be sensitive to the level of service, as passengers may experience longer travel and waiting times. If the level of service deteriorates this may discourage passengers from choosing a shared ride in the future and hence, reduces the demand for shared services.
9.3.3 Market Fragmentation
Market fragmentation refers to multiple service providers/platforms, typically operating independently (except for some drivers working for multiple platforms). Multiple platforms fragment demand and myopically optimize their systems to serve their own customers. This may potentially result in a higher cost to society (pollution, fuel consumption, congestion), customers (fare, time), and their own services (fleet, crew). Séjourné et al. [Reference Séjourné, Samaranayake and Banerjee41] analyzed the impact of competition among on-demand mobility companies on operating costs. Their analysis sheds light into the benefits of aggregating pickup and drop-off locations, increasing the flexibility of pickup times, and allowing drivers work on multiple platforms (also known as multihoming). Pandey et al. [Reference Pandey, Monteil, Gambella and Simonetto42], using New York City taxicab data, conclude that competition among service providers degrades the level of service compared to a centralized model. Integrating all service providers is clearly not practical. However, mechanisms such as multihoming partially mitigate the efficiency loss and increase shared trips. Multihoming has the potential to provide a more flexible supply of labor, which not only increases ride pooling opportunities but also reduces costs, for example, by minimizing the need for rebalancing [Reference Bryan and Gans43].
9.3.4 Operating Strategies and Partnerships
Various studies have proposed and evaluated novel service models for the purpose of facilitating trip matching in order to increase its likelihood. These strategies have the potential to provide more opportunities to share rides by seeking broader consolidation opportunities (in terms of space and time) to efficiently group travelers, while maintaining a high level of service. Several examples follow.
Advanced requests [Reference Ma, Koutsopoulos and Zheng44]. In this scenario, requests are placed ahead of time with desired pickup and drop-off time windows. In general, the service can accommodate passengers with different advanced request intervals and different price sensitivities. Advanced requests are expected to increase the likelihood of sharing trips while reducing operating costs for TNCs. Pricing the service accordingly can be the means of incentivizing passengers to make requests in advance. Uber recently added a similar function to incentivize travelers to depart later than their desired departure times. For example, users can get a $1 Uber cash back if they are willing to depart in ten minutes and $2 if they depart within twenty minutes.
Dynamic waiting [Reference Korolko, Woodard, Yan and Zhu45]. In this scenario, requests are placed in real-time with desired pick-up times. As with advanced bookings, requests wait to be matched to a driver for a period of time (waiting time window). The waiting window is dynamic, depending on market conditions. For example, it increases when the supply of vehicles is constrained. The waiting window is used to increase the opportunities to pool together requests whose origin and destination locations are close to each other (within walkable distance).
Meeting points [Reference Stiglic, Agatz, Savelsbergh and Gradisar23]. In this scenario, requests are placed in real-time with desired pick-up times. Passengers walk to pick up locations and share a ride with other passengers. After alighting, passengers may have to walk from the drop-off spot to their destination. The meeting points are determined dynamically, given the request location and expected LOS of passengers already on-board.
Intermediate transfer points [Reference Masson, Lehuédé and Péton46, Reference Deleplanque and Quilliot47]. In this scenario, requests are placed in real-time with desired pick-up times. Users that are picked up may change vehicles during their trip. A transfer can occur at pre-specified locations in a static manner or can happen dynamically based on how trips are pooled together. Transfers increase the opportunity of matching rides as users from different origins can be pooled together at the transfer locations to a common destination.
Partnerships with other service providers [Reference Schwieterman and Livingston48, Reference Rao49]. In this scenario, on-demand TNCs and public transport agencies work in partnership. For example, hybrid on-demand and public transport services allow passengers to place requests with desired pickup times and origin/destination information. Passengers can be picked up at their origins by on-demand services and dropped off at an “optimal” public transport station. Alternatively, they can take a bus or metro, and then either walk or take another TNC to their destinations, or they can be directly served (door-to-door) by TNC services.
9.4 Assessing Opportunities
Successful outcomes with respect to increasing shared trips probably require a combination of approaches. We focus the discussion on two promising strategies for their potential to improve shared trips and reduce impacts such as VMT: a) advanced requests and b) partnerships with public transport agencies.
The advanced requests strategy is representative of the first three operating strategies discussed earlier. It relies solely on the TNCs to adopt alternative operating models by adding options to their services. Advanced requests can be viewed as a more formal way to extend some of the current offerings, for example, dynamic waiting. Therefore, from an implementation point of view, it represents incremental changes that are more likely to be adopted by companies and users. By contrast, strategies such as meeting points and intermediate transfer points may require significant changes to the digital infrastructure and deviate quite substantially from the core operating models currently deployed.
The second strategy we focus on, partnerships, is quite different from pure operating strategies, as it requires collaboration between TNCs and traditional public transport agencies. It represents an opportunity to take advantage of natural synergies that exist between TNCs and public transport services and has the potential to benefit both stakeholders. Public transport and TNCs both offer shared rides but with different degrees of flexibility. Partnerships between the two help address the weakness of one through the strength of the other.
9.4.1 An Empirical Evaluation of Advanced Requests
Given the on-demand nature of most requests, from a scheduling point of view, the short time between the placing of a request and the initiation of the service limits the ability of customer matching and vehicle scheduling algorithms to take full advantage of the shareability opportunities. A number of studies point out this inefficiency of the on-demand nature of TNC services. Alonso-Mora et al. [Reference Alonso-Mora, Wallar and Rus35] show that predicting future demand accurately could improve the efficiency of TNC operations. Using TNC ride data from three US cities (San Francisco, New York, and Los Angeles), Chen et al. [Reference Chen, Jauhri and Shen50] reported that ride-pooling with approximated future requests can yield significant benefits by reducing total fuel consumption (by 15 percent) and fleet size (by 30 percent). Various surveys indicate that TNC users report optimal waiting times for their ride to be five–eight minutes. In one study [Reference Zhou, Ma, Hirschmann and Lao40], it is reported that 68 percent of the TNC users in the survey actually waited more than four minutes for their service to arrive. Even more importantly, 75 percent of the users are willing to place requests more than fifteen minutes in advance, in return for a fare discount.
Given the inefficiency introduced by real-time requests and also the expressed willingness of users to place requests in advance, at the right fare, we examine in detail the potential of an advanced requests operating model for TNCs. Advance knowledge of future requests gives TNCs more flexibility to schedule trips and identify opportunities to match requests. We evaluate the performance of an advanced requests system relative to current practices using a large-scale dataset from the operations of a major on-demand ride-hailing company in China. In addition to the overall potential of such a strategy in reducing VMT (directly related to energy consumption and emissions) and operating efficiently, the impact of various design aspects of the advanced requests system (for example, advanced requests horizon, vehicle capacity) on its cost and performance is also of interest, as well as the sensitivity of the results to user preferences in terms of level of service (time to be served and excess trip time) and willingness to share.
The advanced requests system that we examine follows the one proposed by Ma et al. [Reference Ma, Koutsopoulos and Zheng44]. Requests are placed ahead of time with desired pick-up time windows. Let H be the advanced reservation horizon (how far ahead requests for service should be placed). Scheduling decisions are made every ∆t seconds (decision epoch), with ∆t ≤ H. That means every ∆t seconds, utilizing all available information on advanced requests and vehicle status, all requests are processed. At each decision epoch, all advanced requests within the advanced reservation horizon H are known. The decisions respect requests that have already been assigned to trips and, either assign pending requests to an existing trip, or start new trips.
The above model is quite general and can represent alternative operating models. For example, for H = 0 the model represents existing, on-demand, ride-pooling services, where passengers make requests expecting immediate service. Existing on-demand ride-pooling services are reactive with requests collected during a short time window, for example, thirty seconds, after which they are assigned to different vehicles and the service stops (pickup and drop-off locations) are scheduled accordingly.
We analyze performance metrics with respect to mobility (VMT) and level of service (LOS) that passengers experience (waiting time and trip delay time). We consider different operating characteristics of the ride-pooling service in terms of the advanced requests horizon and the decision epoch. We also consider different user preferences and traffic conditions. The overall experimental design is summarized in Table 9.1.
Table 9.1 Experimental design parameters.
Dimension | Parameter | Settings |
---|---|---|
Operating model | Advanced requests horizon Decision epoch Vehicle capacity | H∈ {0, 5, 15, 30} minutes Δt∈ {30, 60, 120} seconds {2, 4, 7, 10} passengers per vehicle |
User preferences | Willingness to share | % of customers requesting shared service ∈ {0, 25, 50, 75, 100} |
LOS | Strict users <=2 min, =5-min> Neutral users <=5 min, =10 min> Flexible users <=7 min, =15 min> | |
User groups | All customers are strict All customers are neutral All customers are flexible Mixed <20% strict, 60% neutral, 20% flexible> | |
Traffic conditions | Travel time | Traffic conditions ∈ {low congestion, normal congestion, heavy congestion} |
A large-scale on-demand trip request data set from Chengdu, China from November 01–30, 2016, provided by DiDi Chuxing, a TNC in China, is used in the analysis. To assess the impact of various factors on the performance of ride pooling services, we use requests with pickups and drop-offs within the third ring of the city (20 km × 20 km area, covering 85 percent of all requests). For each trip, the dataset contains a request ID, vehicle ID, and the time of the request and pickup and drop-off locations (approximately 7 million trips). Figure 9.1 shows the heatmap of the distribution of pickups and drop-offs for a morning period (7.30–8:.0 a.m.). The pick-up and drop-off locations are scattered throughout the network, with drop-offs relatively more concentrated in the city center.

Figure 9.1 Distribution of TNC pickups and drop-offs for a morning peak period 7.30–8.30 a.m.
We evaluate the performance of the system for all possible combinations of the above experimental design resulting in 2,880 cases. Requests are scheduled with the objective of minimizing vehicle miles traveled (VMT). The scenario with advanced requests horizon H = 0 and decision epoch ∆t = 30 sec represents (as close as possible) current operations of various TNC services (for example, Uber, Lyft). For the model with advanced requests, the vehicle schedule is optimized every decision epoch, for example, thirty seconds, given vehicle states and real-time and advanced requests within the time horizon, for example, fifteen minutes. The scheduled requests are kept in the request pool until picked up and can be reassigned if a better schedule is found before pickup. The base case is the scenario where all requests are for single trips. If all requests are for single trips, 30,000 kilometers are required to serve all requests (7,500), for the period 7.30–8.30 a.m.
Figure 9.2 shows the impact on VMT of passengers’ willingness to share, for different user types and advanced request horizons. As expected, VMT decreases with the increase of the percentage of passengers willing to share trips. Compared to the base case, VMT is reduced by 14 percent, 25 percent, and 35 percent, when the sharing percentages are 50 percent, 75 percent and 100 percent respectively, for services with an advanced request horizon of fifteen minutes, vehicle capacity of four, and normal traffic conditions. Interestingly, no significant difference is found for the VMT to serve neutral, flexible, and mixed users (given the same settings on other variables in Table 9.1), while it consistently requires more VMT to serve strict users than the other user types. This suggests that, from the perspective of users’ preferences, most of the VMT reductions could be achieved if passengers are willing to wait for five minutes maximum or can tolerate a trip delay of ten minutes maximum.

Figure 9.2 Impact on VMT of willingness to share (vehicle capacity four, normal traffic).
Operating models with advanced requests perform better than operating models with no advanced requests (advanced request horizon 0) from a VMT standpoint without deteriorating the level of service. Advanced requests facilitate a better matching of requests to vehicles as more information is available for decision making. For example, the operating model with an advanced requests horizon of fifteen minutes reduces the base case VMT (all requests are for single trips) by 35 percent, while the current practice model (with no advanced requests) saves 19 percent of the base case VMT if all requests are for shared trips. It is also interesting to note that even a short advanced requests horizon (for example, five to fifteen minutes) provides most of the benefits in terms of VMT reduction.
Figure 9.3 shows the impact on VMT of vehicle capacity for different user types and advanced request horizons. Higher capacity vehicles increase the sharing opportunities as more users can be assigned to the same vehicle and thus decrease the total VMT to serve all the requests. However, there are diminishing returns when the capacity exceeds seven. For example, if all passengers are willing to share, the operating model with advanced requests horizon fifteen minutes saves 35 percent, 40 percent, and 40 percent of the base case VMT for vehicle capacity of four, seven, and ten, respectively. The operating model with no advanced requests reduces the base case VMT by 19 percent, 23 percent, and 24 percent for vehicle capacity of four, seven, and ten, respectively.

Figure 9.3 Impact on VMT of vehicle capacity (all shared, normal traffic).
Figure 9.4 compares the performance of the operating model with advanced requests, assuming a fifteen minutes advanced request horizon and a thirty seconds decision epoch, to the performance of current services (for example, no advanced requests). It is assumed that all passengers are willing to share and have mixed preferences. Vehicle capacity is four and traffic conditions normal. The base case of only single trip requests is also shown. The results are based on the application of the model over the entire day.

Figure 9.4 Comparison of VMT over the course of a day for different operating models: no advanced requests, advanced requests with, H = 15 min 100 percent willingness to share, mixed user preferences, decision epoch thirty sec, vehicle capacity four, and normal traffic.
The advanced requests operating model performs consistently better than a system with no advanced requests across different time periods of the day. Compared to the base case when all requests are for single trips, on average, if all passengers are willing to share, a system with no advanced requests will reduce VMT by 20 percent, while the operating model with fifteen minutes advanced requests will save 35 percent of the total VMT. The 35 percent VMT reduction represents not only a reduction in congestion but also in environmental impacts. In terms of the LOS for the passengers, the no advanced requests system yields an average waiting time of 1.8 minutes and trip delay time of 3.3 minutes, while the advanced requests model has an average waiting time of 2.3 minutes and trip delay time of 5.8 minutes.
In conclusion, operating strategies based on requests in advance have the potential to improve shared rides and reduce the environmental footprint of TNC services. Even short advance request horizons offer substantial benefits at the cost of a small increase in waiting and travel delays. Furthermore, survey data indicate that users are actually interested in using such services.
9.4.2 Partnerships with Public Transport
Recently, there has been a growing interest in exploring partnerships between public transport agencies and TNCs. Such partnerships have the potential to increase shareability opportunities. Many commuting trips for example, take place at the same time and could use the same rail station. This increases the chances of pooling requests that go to the same public transport station (many-to-few case), providing economies of scale for the TNC and also feeding potentially new users to public transport services. In general, public transport agencies may partner with TNCs for various reasons, such as improving mobility where public transport is scarce or cutting down on costs and attracting new customers. TNCs on the other hand, view such partnerships as a means to increase revenue by serving markets with favorable spatio-temporal characteristics. Various studies indeed suggest that TNC and public transport services can be used collaboratively [Reference Schwieterman and Livingston48]. Uber data, for example show a strong relationship between the origin and destination of the rides and the location of public transport stops. Almost 40 percent of Uber rides in London either start or end near a Tube stop [Reference Rao49]. Uber reported a 22 percent increase in the number of its trips that began within 200 meters of a Tube stop after London launched a limited nighttime Tube service in 2016 [Reference Rao49]. In Portland, Oregon, 25 percent of Uber trips occur within a quarter-mile of a public transport station [51]. Lyft rides follow the same pattern, with 25 percent of Lyft riders using the service to connect to public transport. In Boston, 33 percent of Lyft rides start or end near a public transport (MBTA) stop [Reference Kaufman52].
The initial partnerships emerged due to safety concerns. The goal of the partnerships was to motivate people to use public transport to attend social and entertainment activities and, later in the night, when public transport services are not available, help them get back home safely with subsidized TNC rides. The Dallas Area Rapid Transit (DART) partnered with Uber on St. Patrick’s Day to reduce drunk driving casualties during one of the deadliest holidays [Reference Lyons53]. In 2014, the University of Florida proposed a pilot to complement late-night circulatory bus services with subsidized Uber trips to discourage late night-driving [54]. The success of these trials triggered a wider interest in partnerships between TNCs and public transport agencies. The experience with such emerging partnerships however, varies among agencies, ranging from failures such as Bridje in Kansas City, which attracted only a total of 1,452 rides in a year (2016) and was terminated, to the successful Uber partnership with the city of Innisfil, Ontario, with 8,000 rides per month.
In general, three main types of partnerships are observed between public transport and TNCs: First/Last Mile connections, paratransit, and bus route replacement.
First/Last Mile connections: Providing on-demand rides that connect riders to public transport options is the most common partnership. Schwieterman and Livingston [Reference Schwieterman and Livingston55] reviewed twenty-nine agencies in North America and found that since 2016, at least six offered discounted TNC rides to/from public transport rail stations [Reference Schwieterman and Livingston55]. The Pinellas Suncoast Transit Authority (PSTA), for example, became the first public transport agency in the United States to partner with a TNC to provide first/last mile services. “Direct connect” was a pilot service that used public funds to subsidize Uber rides that would allow riders to get to and from a bus stop. “Direct Connect” replaced underperforming, low-frequency bus routes that were carrying less than five passengers per stop per day [54]. The pilot showed promise to reduce costs and the city allocated additional funds to expand the service to ten times the number of bus stops originally served [54]. European cities are also forming similar partnerships, such as BerlKonig in Berlin, Germany [56], and ViaVan in Espoo, Finland [57].
Some partnerships in this group however, experienced poor results. Centennial, Colorado, terminated a partnership with Lyft that offered free trips to light rail stations (Go Centennial). The city had to spend more (compared to the traditional paratransit service they offered, known as Call-n-Ride service), while serving fewer rides [Reference Arellano58]. A report on the “Go Centennial” program blames lack of systematic integration between regional public transport services and the Lyft service for the poor results. Trips were not synchronized with the light rail schedule and alighting riders had to wait for Lyft to arrive. The same report also acknowledges the presence of parallel services (from other demand responsive services) that caused inefficiencies and limited the benefits that come from economies of scale [59].
Paratransit (dial-a-ride): It is typically very expensive to operate traditional paratransit services, which are often mandated by the Americans with Disabilities Act. The Brookings Institute estimates that in 2013, 12.2 percent of the operating costs of public transport agencies (approximately $5.2 billion) went to paratransit. Contracts with TNCs to provide paratransit services are particularly interesting, as agencies are under pressure to find innovative solutions to deal with the ever-growing paratransit costs. The experience from partnerships between public transport and TNCs to offer paratransit services is promising. Agencies, such as in Boston (MBTA) and Las Vegas (RTC), use TNCs as platforms to provide paratransit services that are easier for passengers to use and at a lower cost for the agency [Reference Marshall60, Reference Marroquin61]. In Boston, the MBTA recently renewed a three-year contract with Uber and Lyft to supplement their paratransit services [62], even though the TNC-based service may have problems serving some wheelchair users who have to be accommodated through other means [Reference Daniel and Alulema63]. In Las Vegas, RTC aimed to reduce the $32 per ride cost for traditional paratransit services by outsourcing the trips to Lyft. The public transport agency estimates that each Lyft ride costs about $15 [64].
Bus route replacement: Another type of collaboration is using TNC services to replace bus routes. The town of Innisfil, Ontario, decided to partially subsidize TNC trips in place of the traditional public transport system operating in the city. The average subsidy of $5.62 per passenger is lower than the subsidy for a typical bus ride [Reference Schwieterman and Livingston55]. Uber reports that the partnership is saving Innisfil $8 million a year [65]. In Arlington, Texas, the town’s entire bus service was replaced by services provided by Via, making Arlington the largest US city without a typical public transport system [Reference Etherington66]. The Via rides cost between $3 and $5, depending on distance and origin/destination of the trip. The customer is notified of the expected pickup time but, in general, waiting time is less than ten to twelve minutes, which makes the service particularly attractive. The program has been successful, and the contract was renewed with expanded service from 30 percent coverage to 100 percent coverage [Reference Hanna67].
In summary, partnering with TNCs can have benefits for public transport authorities. Connecting riders to public transport, replacing inefficient bus routes, and providing cost-effective paratransit services are the most promising areas of collaboration. Collaboration can also be beneficial to TNCs. As mentioned earlier, TNCs are more cost-effective when trips are concentrated (for example, many-to-few). Partnerships with public transport favor and greatly facilitate such operations. The lessons learned from the current efforts suggest that service design, business models, demand spatio-temporal characteristics, marketing, and demographics are important for the success of public transport-TNC partnerships. The degree of complementarity (TNCs working together with public transport agencies) and substitution (TNC trips replacing public transport trips) between TNCs and public transport varies. The substitution effect is expected to be larger for example, in cities or areas where public transportation LOS is low (for example, high travel times, low frequency of service) compared to places with a strong public transport system.
9.5 Conclusion
TNC services have seen significant growth in recent years. While they play an important role in supporting urban mobility, they may also introduce negative externalities. Increasing ride pooling has the potential both to reduce the negative societal impacts and to improve operating efficiency (which is of great concern to TNCs). Although increasing the number of shared trips is a desirable goal, experience suggests that shared trips are currently only a small fraction of all trips. The chapter summarized the factors impacting ride-pooling and synthesized the main approaches that can be deployed to increase ride pooling. Two representative approaches to increase the number of shared trips discussed in detail, operations where requests are required to be known in advance (with short time horizons), and partnerships with public transport agencies for providing multimodal services, have shown promising results.
A case study with data from a large TNC showed that significant benefits (VMT savings) can be realized when advanced requests are combined with an increased willingness to share. Even short, advanced request intervals (five to fifteen minutes) can capture the majority of the benefits of advanced requests. The VMT savings are realized at the expense of a small reduction in LOS.
Partnerships between public transport and TNCs can be beneficial to both sides, in terms of reducing costs, improving level of service, and potentially increasing demand. Three main types of partnerships are observed: offering first/last mile connections, providing paratransit services, and substituting for unproductive bus routes. The discussion suggests that service design, business models, consumer attitudes, and demographics, are important for achieving increased ride pooling.
However, the success of these strategies depends on many factors that can play a key role in shaping the future role of TNCs as an integral part of a sustainable urban mobility system, especially considering that public transport should and will always be the backbone of urban transportation.
Pricing is an important lever that TNCs can use to drive demand for various services. Pricing, properly differentiated by service type, impacts consumer choices (along with level of service). Currently, prices for the typical TNC service (ride alone) are rather low, set aggressively to increase market share. Fares are subsidized by the venture capital the companies have attracted and do not always reflect the true cost of the trips. As a result, given this low ceiling (price for the single trips), there is currently limited room to set prices appropriately for the other products. Price differentiation is not strong enough to incentivize users to switch to the more sustainable options, such as ride-pooling.
Policy can play an important role in guiding TNCs to offer more sustainable services. A recent study proposes a number of financial instruments, including surcharges to help cities recover TNC-associated costs and externalities (management, curb utilization, congestion, and pollution), fees designed to penalize inefficient routes, and rewards for shared trips and trips that are complementary with public transport [68].
Integrated fare platforms, providing fare bundles for trips involving public transport/TNC connections is a logical next step in the development of partnerships between public transport and TNCs. Such integrated fare platforms eliminate the obstacle and inconvenience of separate payment means for customers, facilitate revenue allocation among stakeholders, and promote multimodal trip searching and recommendations.
Finally, it should be pointed out that, currently, data sharing between TNCs and public transportation agencies and city authorities is rather limited. Data sharing and greater transparency are important to further develop integrated, inclusive, multimodal services that promote sustainable mobility and accessibility in urban areas.
9.6 Acknowledgments
The authors would like to thank DiDi Chuxing, China for providing the data for this research, and the Massachusetts Green High-Performance Computing Center (MGHPCC) for the computational resources.
10.1 Introduction
The “sharing economy” has been lauded for decentralizing commercial transactions, allowing individuals to directly transfer products and services. Less often discussed is how it has precipitated a parallel geographic decentralization. Whereas historically the industries being disrupted by these platforms have been concentrated in downtown centers and business districts, the same transactions are now spreading throughout cities and towns. Uber and Lyft drivers make many more pick-ups and drop-offs in residential areas than traditional taxis(Lam & Liu, Reference Lam and Liu2017). Airbnb listings offer short-term lodging in residential neighborhoods that have never had hotels. When this is discussed, it is often done in terms of supply and demand. First, some describe it as a boon to both the seller and the buyer as it expands the opportunity for each to deliver and receive services in locations that are more convenient to them. Second, there is sometimes concern, especially in the case of Airbnb, that these new transactions are removing products (for example, housing) from existing markets, shifting their balance. This market-oriented perspective, however, fails to engage the more fundamental implication of sharing economy platforms for neighborhoods: By shifting the geographic distribution of commercial transactions, they have the potential to alter who is in a neighborhood at a given time and what they do – whether residents or visitors – while they are there. This could lead to pervasive impacts to the social and behavioral dynamics, going beyond questions of supply and demand.
Here we present a generalized theory of how sharing economy platforms can and do impact neighborhoods. Our rhetorical focus is on urban neighborhoods, and we draw heavily from literature on cities, but the argument is relevant to suburban and even rural areas as well. After articulating this model more fully, we apply it to Airbnb, treating its incursion into the neighborhoods of Boston, MA as a test case. Along the way, we discuss some of the conceptual challenges that must be taken into account in such analyses.
10.2 A Framework for How Sharing Economy Platforms Can Impact Neighborhoods
The sharing economy has enabled private citizens to directly exchange services and products. This “peer- to-peer” construction has also changed the places where such transactions are likely or even possible. We propose a three-step framework for how these shifts in the commercial landscape of the city can affect the social and behavioral dynamics of neighborhoods (see Figure 10.1). Throughout, we use Airbnb and ride-sharing services (Uber and Lyft) as illustrative examples as they are the most common sharing economy platforms. That said, we go deeper into the implications of the model for Airbnb in the next section.
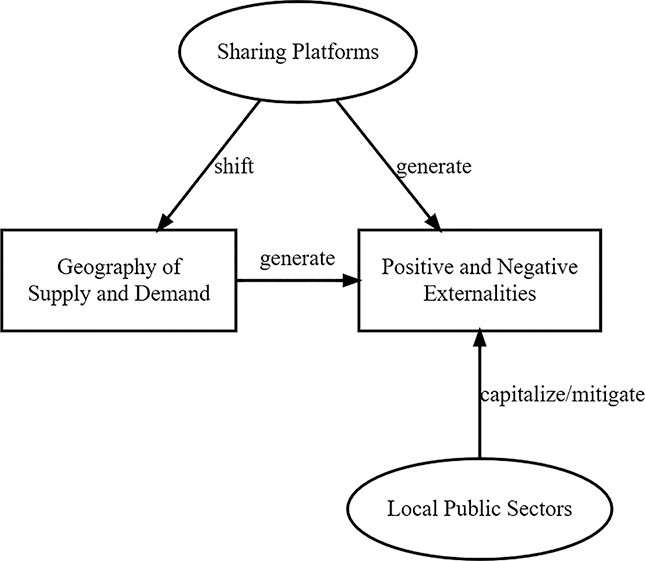
Figure 10.1 Three-step model of the sharing economy.
First, the sharing economy alters the traditional geographic distribution of service supply. Many services have historically been concentrated in business districts because of the incentives of agglomeration and access to consumers and the relatively high cost of serving areas with low expected demand. Sharing economy platforms have disrupted these mechanisms by lowering the transaction cost of offering services, thus enabling producers and consumers to find each other in less densely populated areas. This can be seen in both Airbnb and ride-hailing services. In terms of the former, researchers examining New York City, Boston, San Francisco, and Barcelona have found that Airbnb listings are common in neighborhoods that did not previously have hotels (Benítez-Aurioles, Reference Benítez-Aurioles2017; Horn & Merante, Reference Horn and Merante2017; Koster, van Ommeren, & Volkhausen, Reference Koster, van Ommeren and Volkhausen2021). Likewise, much has been written about how ride-sharing has increased access to taxis in residential neighborhoods, especially in New York City, where taxis notoriously concentrate in Manhattan and rarely venture out to the other four boroughs (Correa Diego, 2017).
Second, these geographic shifts in supply and demand can generate unexpected positive and negative externalities for communities. Oftentimes the impacts of the sharing economy are articulated in terms of city- or region-wide industry patterns, like drops in overall hotel revenue (Dogru, Mody, & Suess, Reference Dogru, Mody and Suess2017; Zervas, Proserpio, & Byers, Reference Zervas, Proserpio and Byers2017). We are concerned here with more local impacts at the neighborhood level as the introduction of services brings in people and activities that were not there previously. These impacts might be economic in nature, but they also could arise from the social interplay between these visitors, the services themselves, and the local community. Such effects might be positive. For example, a sharing economy platform can stimulate economic development by increasing access to flexible jobs and foot traffic by outsiders who will frequent local establishments. For instance, one study in New York City found that neighborhoods with more Airbnb listings saw an increase in business, as reflected by expanded staff hiring (Alyakoob & Rahman, Reference Alyakoob and Rahman2019). On the other hand, the increased activity might bring nuisances, like unpleasant guests or burdensome traffic, or, as has been seen, lower the supply and in turn raise prices for rental units in the community. We find the example of Airbnb leading to additional crime intriguing, in fact, as it might implicate either of two mechanisms instigated by a density of short-term rentals. As tourists begin to spend more time in the neighborhood, they might commit crimes, create disorder, or be targets for those who might do so themselves (Biagi & Detotto, Reference Biagi and Detotto2014; Brunt, Mawby, & Hambly, Reference Brunt, Mawby and Hambly2000; De Albuquerque & McElroy, Reference De Albuquerque and McElroy1999; Harper, Reference Harper2001; Ryan, Reference Ryan1993; Schiebler, Crofts, & Hollinger, Reference Schiebler, Crotts, Hollinger, Pizam and Mansfield1996; Stults & Hasbrouck, Reference Stults and Hasbrouck2015). Alternatively, the replacement of stable (or at least semi-stable) renter households with short-term rental units can create gaps in local social networks, potentially undermining a community’s natural ability to prevent crime (Kawachi, Kennedy, & Wilkinson, Reference Kawachi, Kennedy and Wilkinson1999; Sampson, Reference Sampson2012; Sampson & Groves, Reference Sampson and Groves1989).
Third, local governments are tasked with designing policies and regulations that capitalize on the positive externalities of the sharing economy while minimizing the negative externalities. This third component of the model is a response to the first two factors we have described, they also will create a feedback loop, reshaping the distribution and consequences of both the sharing economy activity and its consequences. For instance, regulatory and legislative approaches to Airbnb and ridesharing have often focused on specific (and, at times, anecdotal) concerns about the impacts of these platforms on neighborhoods. In the case of New York City’s negotiations with Uber and Lyft, the emphasis was primarily on increased traffic. For Airbnb in Boston, it was gentrification and displacement of the incumbent community. It is not to say that these considerations were incorrect, but that a more extensive science of the kinds of externalities we suggest here will be critical to developing fully informed policy. In the work we present here, our goal is not necessarily to critique current policy strategies for regulating the sharing economy, but to recommend a scientifically informed process for designing such regulations. That said, it is likely that in many cases the latter will give rise to the former.
Our three-part framework lays the groundwork for various research questions and practical implications for the sharing economy. In terms of the first component of the model, to what extent do sharing economy platforms shift the geographic distribution of a given service? Which neighborhoods see the greatest change? Are these changes correlated with demographic factors? As we progress to the second part of the model, what are the externalities arising from the geographic shifts resulting from a given sharing economy platform? To what extent are they consequential? Do they affect certain communities more than others? Critically, answers to these questions make it possible for policymakers to take action at the third stage of the model. They will be better equipped to determine which policy tools are most effective in managing the externalities surrounding the sharing economy. How do we design them to best effect and which neighborhoods most need attention? We thus encourage researchers and policymakers to partner under the perspective that these three components – the geography of supply and demand, localized social and behavioral dynamics, and public policy – are deeply intertwined in determining how the sharing economy contributes to the ongoing evolution of neighborhoods. With luck, such partnerships will provide the depth of knowledge necessary to design and advance “next-generation” regulatory approaches that move past traditional policy tools.
10.3 Airbnb and Urban Neighborhoods
Airbnb and other peer-to-peer rental platforms make an ideal test case for our framework on how sharing economy platforms can impact neighborhoods. Per the first component of our model, travelers are no longer limited to districts with hotels, which are often concentrated around downtown neighborhoods with commercial and industrial zoning. Instead, they are now able to stay in apartments, houses, and condominiums in residential neighborhoods. This newly introduced commercial activity and the visitors it brings with it can have a variety of externalities, as stated in component two of our model. These can be both positive and negative, thereby impacting the character and trajectory of a neighborhood. An understanding of these externalities should in turn influence the regulation of short-term rentals, which have been the subject of much public discourse and policy debates in cities around the world. This is the culmination and third piece of our model.
To date, most research on the impact of Airbnb rentals on communities has centered on two main outcomes, each related to patterns of supply and demand – the revenue of the hotel industry and the price of housing. The former line of inquiry has found that Airbnb draws potential customers away from hotels and thus lowers their revenue, especially for middle- and low-end hotels (Dogru et al., Reference Dogru, Mody and Suess2017; Zervas et al., Reference Zervas, Proserpio and Byers2017). This work, however, has been more about the way that Airbnb disrupts the hotel industry in a city or broader region, and not about how Airbnb impacts neighborhoods. Meanwhile, research on the relationship between Airbnb and housing values works off the assumption that many Airbnb listings are for units that would otherwise be owner-occupied or rental properties. By lowering this supply, Airbnb in turn leads to increases in housing value and rental prices, a result that has been found in multiple cities in North America and Europe (Ayouba et al., Reference Ayouba, Breuillé, Grivault and Le Gallo2019; Barron, Kung, & Proserpio, Reference Barron, Kung and Proserpio2018; Horn & Merante, Reference Horn and Merante2017; Garcia-López et al., Reference Garcia-López, Jofre-Monseny, Martínez-Mazza and Segú2020; Sheppard & Udell, Reference Sheppard and Udell2016).
Less attention has been paid, however, to the ways that the prevalence of Airbnb in a neighborhood might impact other aspects of a neighborhood. This is important scientifically and practically, as it allows us to better understand how short-term rentals interact with local social dynamics and can provide guidance for policies and regulations that balance their costs and benefits for local residents and businesses. In order to enumerate these possibilities, we must first consider what it means to have tourists in a residential neighborhood. First, tourists tend to frequent local establishments, especially for food and drink, which could increase economic activity in “main street” districts that serve local communities. For example, Alyakoob & Rahman (Reference Alyakoob and Rahman2019) found that New York City neighborhoods with a greater presence of Airbnb listings experienced more growth in restaurant employment. They attributed this effect to visitors using Yelp reviews data. Likewise, Schild (Reference Schild2019) identified a positive impact of Airbnb usage on the number of firms in the entertainment sector.
Although they might contribute to the local economy, Airbnb users might not have an exclusively positive effect on neighborhoods. Tourists can potentially be disruptive by keeping different hours than locals and possibly partaking more avidly in alcohol or other disruptive activities. They also are more vulnerable to property crime than locals as they lack protective relationships and have money and valuables with them. Criminologists have regularly found that neighborhoods with more tourists tend to have greater crime (Boakye, Reference Boakye2010; Harper, Reference Harper2001; Schiebler et al., Reference Schiebler, Crotts, Hollinger, Pizam and Mansfield1996). No one has yet directly tested, however, whether the presence of Airbnb in a neighborhood leads to rises in crime and disorder via this mechanism.
Additionally, as we stated earlier, there is also the possibility that the tourists themselves are not responsible for rises in crime, but that the diminished number of stable households in the neighborhood interrupts the function of local social networks, which are known to be crucial in the management of crime and disorder (Sampson & Groves, Reference Sampson and Groves1989). To illustrate, if a sufficient number of units throughout a community have been converted to short-term rentals – the most transient form of occupancy possible – fewer neighbors are present to sustain a strong social network, also referred to as the social organization (Sampson, Reference Sampson2012), and establish the “social capital,” including trust, reciprocity, and social cooperation, known to be crucial to communities (Coleman, Reference Coleman1988). Regardless of the specific terminologies used, researchers have found these processes to be active in diminishing crime (Kennedy et al., Reference Kennedy, Kawachi, Prothrow-Stith, Lochner and Gupta1998; Sampson, Reference Sampson2012). There is also evidence in the network science literature that demonstrates the positive effect of enhancing the community structure of social networks on their collective prosocial behavior. The strength of the community structure in network science is often measured by network modularity, which measures the ratio of intensity of ties within and across a set of nodes that are identified as a community. Emergence of prosocial norms can then be studied by allowing nodes on a network to play some strategic games with their network neighbors and update their behaviors by occasionally mimicking their successful neighbors. The final status of the collective behavior is then related to the structural features of the network, such as modularity, degree distribution, and other standard network measures. The games are chosen in such a way to model various social dilemmas (for example, prisoner’s dilemma or ultimatum game), in which players can either act selfishly for short-term gains, or take prosocial actions that have long-term benefits for them and the network as a whole. Several studies using this framework have demonstrated that how players resolve such dilemmas depends on the structure of the social network and have shown a strong impact of network modularity – that measures the intensity of community structure based on ratio of link density within and across communities – on population-level attributes such as cooperation, fairness, and stability (Gianetto & Heydari, Reference Gianetto and Heydari2015, Reference Gianetto and Heydari2016; Heydari, Heydari, & Mosleh, Reference Heydari, Heydari and Mosleh2019; Mosleh & Heydari, Reference Mosleh and Heydari2017).
A corollary question is how the presence of Airbnb and tourists might impact the behaviors of local property owners. As listings increase in a neighborhood, they act as a tangible representation of the attractiveness and marketability of housing there. This might then inspire property owners to invest in their buildings, potentially accelerating increases in property values, rental prices, and more generalized gentrification.
Here we leverage our three-component model of sharing economy and neighborhoods to examine outcomes in Boston, MA, which has been the site for early work on how Airbnb impacts property value. It has also been one of the cities that has led the way in developing policy interventions that seek to limit the negative impacts of Airbnb on neighborhoods. Specifically, we analyze how the longitudinal growth of Airbnb across the neighborhoods of Boston, MA is associated with: (1) the increase in food establishments; (2) levels of crime and disorder; and (3) the tendency of property owners to invest in parcels across the city.
10.4 Airbnb’s Presence in Boston
To examine the impact of Airbnb on Boston neighborhoods, we downloaded listings and reviews from InsideAirbnb.com, an independent, noncommercial website that scrapes and publishes data sets of Airbnb listings for cities across the world. InsideAribnb.com has published these data annually since 2015, but Airbnb entered Boston in 2009. In order to overcome this limitation, we leveraged the “host since” field, which indicates the date a property became an Airbnb listing, to estimate which Airbnb listings were present in each year 2010–2014. Koster et al. (Reference Koster, van Ommeren and Volkhausen2021) took a similar approach using the date of a listing’s first review, but we found that the “host since” variable more consistently had a value and would be more precise in any case.
As shown in Figure 10.2, Airbnb had limited presence in Boston in the early years, with a negligible number of listings and reviews. There was rapid growth, however, between 2014 and 2018, over which time the number of listings more than doubled from 2,558 to 6,014. There were also nearly 80,000 total reviews by 2018. Our focus here, though, is not the city as whole, but how the increased prevalence of Airbnb was distributed across neighborhoods. We examine this by joining each listing to the containing census tract, which we use to approximate neighborhood (avg. population = 4,000; 168 with meaningful population in Boston). A major challenge, though, is determining how best to measure Airbnb’s presence in a neighborhood, particularly in terms of how we would expect that presence to have impact on local behavioral and social dynamics.

Figure 10.2 Airbnb’s expansion in Boston: 2009–2018.
Airbnb listings are a “naturally occurring” data set, meaning they were not originally constructed for research purposes. The upshot is that it is incumbent upon researchers to determine how to operationalize the measures of interest (O’Brien, Reference O’Brien2018). As such, previous studies have proposed several ways of measuring Airbnb’s presence in a community. Some have focused on the number of listings, either as the percentage of total housing units in a census tract (Wegmann & Jiao, Reference Wegmann and Jiao2017) or relative to the number of buildings, being that many buildings contain multiple apartments of condos (Koster et al., Reference Koster, van Ommeren and Volkhausen2021). Others have focused on the number of reviews, either as a pure count (Schild, Reference Schild2019) or relative to the number of households in the neighborhood (Alyakoob & Rahman, Reference Alyakoob and Rahman2019), arguing that reviews are a better reflection of the number of tourists brought to the neighborhood by the platform. There is no consensus as to whether one of these is superior to others, however, for which reason we use three measures of Airbnb presence here. First, we measured the density of Airbnb as the number of Airbnb listings in a neighborhood divided by the number of housing units (as accessed from American Community Survey 2011–2017 estimates). Second, we measured the penetration of Airbnb as the number of unique addresses with listings divided by the number of parcels (lots that contain one or more units, per the City of Boston’s Assessing Department) in the census tract, thereby approximating the share of buildings with at least one Airbnb listing. Third, we measured usage of Airbnb as the number of reviews divided by housing units in a census tract. For the sake of brevity, we use these three terms – density, penetration, and usage – throughout.
The reason for adopting three measures of Airbnb’s presence is because none of them captures the whole picture of Airbnb’s impacts on neighborhood on its own. For example, if most listings in a neighborhood are concentrated in a few condo buildings, the potential positive or negative effects generated by Airbnb-related activities might be limited to the immediately surrounding areas, leaving the rest of the community unaffected. On the other hand, if the census tract is a sprawled suburban neighborhood filled with single family houses, the same number of Airbnb listings would be spread more evenly, having a wider impact across the neighborhood. In addition, per the advice of Schild (Reference Schild2019), Airbnb reviews more directly reflect the volume of usage by tourists as a higher density or penetration of Airbnb listings is not always accompanied by more visitors. There is of course, though, the potential for substantial measurement error in this last measure as leaving reviews for hosts is not a mandatory policy of Airbnb, hence many visitors choose not to do so. Also, a party with multiple visitors can only leave one review for a listing, inevitably fewer than the people who were present. As a consequence, this measure underestimates the total volume of tourists produced by Airbnb, and analyses using it must assume that these undercounts are consistent across neighborhoods; that is, the tendency to undercount is not exaggerated in some neighborhoods more than others. Another benefit of using these three different measures is the ability to potentially differentiate between those impacts caused by the sheer number of tourists (quantified with usage), versus those created by listings (quantified with penetration and density). As we noted earlier, this is specifically relevant to our discussion of Airbnb might lead to elevated crime in a neighborhood: Tourists could attract or perpetrate crime, or the conversion of units to short-term rentals could lead to weaker ties and relationships within the community.
Our first task is to determine whether we are justified in treating each of these three measures as a separate indication of the presence of Airbnb in a neighborhood. While they are semantically and computationally distinct, it is completely possible that they are too strongly correlated to be analyzed independently. Figure 10.3 presents the correlations among the three measurements for census tracts, showing that they are substantially correlated (r = .47 – .76), but not so much to suggest that they cannot be analyzed separately. The strongest correlation is between density and usage, which is unsurprising being that they are calculated using the same denominator. Meanwhile, the weakest correlation is between penetration and usage. As one can see in the scatter plot at the bottom left corner of Figure 10.3, there are numerous neighborhoods with a high level of Airbnb usage (that is, many reviews per housing unit) but a low level of Airbnb penetration (that is, number of parcels with at least one listing); meanwhile there are others with low Airbnb usage but high penetration. Given this, we move forward analyzing all three measures.

Figure 10.3 Correlations between three measures of Airbnb’s presence in Boston.
In Figure 10.4 we can see that Airbnb density, penetration, and usage all increased over time and across census tracts in Boston. That is not to say that this growth was uniform. Certain census tracts were the first to have a measurable presence of Airbnb and then proceeded to have high levels of Airbnb by all three measures. The highest were tracts with as many as 8 % of units and 40 % of buildings featuring at least one listing. Even more striking, our measure of Airbnb usage is as high as 1.5 reviews per housing unit in the tract. In contrast, in many other tracts the presence of Airbnb was limited and even absent throughout the study period. Meanwhile a handful of tracts started with very low Airbnb presence and then witnessed rapid growth of Airbnb-related activities.

Figure 10.4 Airbnb’s presence in Boston.
Note: Each row represents a census tract from 2010 to 2018. The darker the color, the higher the Airbnb presence. Tracts are in the same position in each panel, meaning we can compare panels to confirm that most tracts with high level of presence on one measure scored similarly on the other measures.
Before proceeding, it is worth noting which neighborhoods have high and low presence of Airbnb. Figures 10.5–10.7 map the spatial distributions of the three measures over time, demonstrating that the measures indeed tell different stories about Airbnb’s expansion in Boston. In Figure 10.5, we notice that as the number of listings increases, Airbnb penetrations were most significant in census tracts that are located in the downtown urban center (in the northeast of the city). However, in Figure 10.6, although census tracts in urban centers still show relatively high Airbnb presence measured by Airbnb density, the tracts with highest level of Airbnb density are scattered surrounding the downtown areas. This divergence can be explained by population density in different urban neighborhoods. Urban centers usually have more people and less land while surrounding neighborhoods are somewhat less dense. Hence, Airbnb penetration might be high in an urban center, but its density is dwarfed by the immense number of housing units there. Owing to their strong correlation, Airbnb usage displayed a similar pattern to density (see Figure 10.7). Whereas the downtown and the first ring of neighborhoods around it share the distinction of highest presence of Airbnb depending on the measure used, neighborhoods located further out are consistently low in all three measurements. In the next section, we estimate Airbnb’s impacts on neighborhood’s investment and quality of life using the three measurements.

Figure 10.5 Airbnb penetration.

Figure 10.6 Airbnb density.

Figure 10.7 Airbnb usage.
10.5 Evaluating Airbnb’s Impacts on Boston Neighborhood
10.5.1 Methods
To estimate Airbnb’s impacts on Boston neighborhoods, we return to the three hypotheses from above: (1) Airbnb might encourage property owners to invest in their buildings; (2) the presence of tourists will support expansion of the local restaurant industry; and (3) the increased prevalence of tourists will lead to elevated disorder and crime. In order to test each of these hypotheses, we draw from indicators collected or developed by the Boston Area Research Initiative (BARI). First, BARI releases measures of investment and growth annually based on building permits approved by the City of Boston (O’Brien & Montgomery, Reference O’Brien and Montgomery2015; O’Brien et al., Reference O’Brien, Montgomery, de Benedictis-Kessner and Sheini2019). The most relevant such indicator is alteration to existing buildings, calculated as the percentage of parcels in a census tract undergoing an addition or renovation in a year (excluding newly constructed buildings).Footnote 1 Second, we calculated the number of new food establishments from the records of new food establishment licenses approved by the Health Division of the Department of Inspectional Services (ISD).Footnote 2 Third, we obtained three indicators of social disorder and crime based on 911 dispatches (Ciomek & O’Brien, Reference Ciomek and O’Brien2019): Public social disorder, such as panhandlers, drunks, and loud disturbances; private conflict arising from personal relationships (for example, domestic violence); and public violence that did not involve a gun (for example, fight). Each of these measures was based on a combination of case types in the 911 system, divided by the population of the census tract (to form a rate). We also access demographic measures from the American Community Survey as control variables, particularly measures of socioeconomic status (for example, income, poverty rate), as they are closely related to many of the variables here. Table 10.1 provides a summary of the variables.
Table 10.1 Description of variables
mean | sd | min | max | Observations | |
---|---|---|---|---|---|
Permits for Property Alteration (%) | 0.19 | 0.09 | 0.04 | 0.61 | 1,176 |
Number of Licenses for Food Establishment | 1.03 | 2.32 | 0.00 | 24.00 | 1,344 |
Airbnb Density (%) | 0.01 | 0.01 | 0.00 | 0.08 | 1,344 |
Airbnb Penetration (%) | 0.04 | 0.06 | 0.00 | 0.41 | 1,344 |
Airbnb Usage (%) | 0.12 | 0.20 | 0.00 | 1.44 | 1,344 |
Events of Private Conflict (per 1,000) | 11.13 | 6.18 | 0.00 | 32.97 | 1,344 |
Events of Guns (per 1,000) | 4.21 | 4.52 | 0.00 | 25.79 | 1,344 |
Events of Violence (per 1,000) | 28.10 | 21.84 | 1.33 | 172.54 | 1,344 |
Events of Social Disorder (per 1,000) | 7.51 | 8.67 | 0.00 | 75.82 | 1,344 |
Each of the variables was measured annually throughout the study period, making for a panel design. Thus, we analyze the impacts of Airbnb on a neighborhood using a set of generalized difference-in-difference (DID) fixed-effects models with multiple periods and continuous treatments (Lechner, Reference Lechner2011):

where i represents the census tract, t represents the year. Yi,t are the dependent variables: alteration to existing structures, growth in food establishment licenses, and indicators of social disorder and crime. g is the estimated causal effect of Airbnb presence.Footnote 3 The key independent variables are lagged for one year to avoid reverse causal effects. h and b are the tract and year fixed effects, respectively, capturing both time-invariant characteristics of tracts and spatially invariant characteristics of years (for example, a city-wide increase in Airbnb prevalence or property investment). Ci,t is the vector of control variables including log of median home values, median household income, and population density, among others.Footnote 4 Note that we run the models three times for each outcome variable, independently testing the impact of each aspect of Airbnb prevalence.
10.5.2 Results
The results from the DID models supported one of our three hypotheses of how Airbnb can impact neighborhoods. As shown in Table 10.2, increases in the prevalence of Airbnb predicted increased property investment in the following year. This was true whether we measured Airbnb in terms of density (b = 0.004, p < 0.01), penetration (b = 0.001, p < 0.001), or usage (b = 0.000, p < 0.01). The coefficients indicate that a one percentage-point increase in Airbnb density was associated with a 0.4 percent increase in property alteration and that a 10 percentage-point increase in Airbnb penetration was associated with 1 percent increase in building permits qualified as housing investments. This effect seems practically small but presents a reasonable ratio of 10:1 between properties listing on Airbnb and properties pulling building permits for improvements. It is worth noting that these are not necessarily the same properties, but it would seem reasonable to assume there is at least some overlap. Further, a 1 percent increase in property alteration is nontrivial, considering that the average census tract has just below 10 percent of properties apply for permits in a given year. In summary, Table 10.2 provides evidence that the prevalence of an Airbnb market in a neighborhood contributes to a greater percent of property investment. This joins existing evidence that Airbnb presence leads to higher housing property value, but we found no evidence to suggest that residential property investment is the intermediate factor through which Airbnb density affects housing values.
Table 10.2 Fixed-effects models on property alterations
(1) | (2) | (3) | |
---|---|---|---|
Airbnb Density (%) | 0.386∗∗ | ||
Airbnb Penetration (%) | (0.162) | 0.108∗∗∗ | |
Airbnb Usage (%) | (0.039) | 0.017∗∗ | |
(0.008) | |||
Tract FE | Yes | Yes | Yes |
Year FE | Yes | Yes | Yes |
Controls | Yes | Yes | Yes |
Observations | 1,126 | 1,126 | 1,126 |
F | 6.742 | 6.417 | 6.841 |
R2 | 0.940 | 0.941 | 0.940 |
Note: clustered standard errors are displayed in parenthesis. All independent variables are lagged for one year. Control variables include median home value (log) and events of violence, social disorder, guns, and private conflicts
Significance levels: * p<0.00; ** p<0.01; *** p<0.001.
Table 10.3 shows no clear relationship between Airbnb prevalence in Boston neighborhoods and the expansion of food establishment licenses, regardless of which measure of Airbnb prevalence we used. Table 10.4, however, shows that neighborhoods with a higher level of Airbnb penetration saw rises in violent crime in the following year (b = 0.546, p < 0.001), and notably to a greater extent than the concurrent measure of penetration. There was still no corresponding effect on public social disorder or private conflict, however. Airbnb density in the previous year was also associated with higher levels of violent crime, albeit at a lower significance, and thus magnitude, relative to penetration (b = 1.407, p < 0.05). Airbnb usage had no effect on any of the three measures in the following year. We also performed a two-year lagged analysis, and the results are in general agreement with those with one-year lag in terms of the impact of Airbnb penetration on events of violence. There, Airbnb penetration not only predicted increased violence at this time scale, but also showed a moderate impact on events of private conflict, an effect that was not present in the one-year lagged analysis. The effects of Airbnb usage and density also concurred with the one-year lagged analysis (Ke et al., Reference Ke, O’Brien and Heydari2021).
Table 10.3 Fixed-effects Poisson regressions on new food establishment licenses
(1) | (2) | (3) | |
---|---|---|---|
Airbnb Density (%) | –0.040 | ||
(0.047) | |||
Airbnb Penetration (%) | –0.012 | ||
(0.008) | |||
Airbnb Usage (%) | –0.003 | ||
(0.003) | |||
Tract FE | Yes | Yes | Yes |
Year FE | Yes | Yes | Yes |
Controls | Yes | Yes | Yes |
Observations | 1,196 | 1,196 | 1,196 |
Chi2 | 63.089 | 64.386 | 63.863 |
Note: clustered standard errors are displayed in parenthesis. All independent variables are lagged for one year. Control variables include median home value (log) and events of violence, social disorder, guns, and private conflicts.
Significance levels: * p<0.00; ** p<0.01; *** p<0.001.
Table 10.4 One-year lagged independent variables
Events of Private Conflict | Events of Social Disorder | Events of Violence | |||||||
---|---|---|---|---|---|---|---|---|---|
(1) | (2) | (3) | (4) | (5) | (6) | (7) | (8) | (9) | |
Airbnb Penetration (lag 1) | 0.041 | –0.115 | 0.546*** | ||||||
(0.039) | (0.118) | (0.133) | |||||||
Airbnb Density (lag 1) | –0.112 | –0.426 | 1.407* | ||||||
(0.227) | (0.293) | (0.614) | |||||||
Airbnb Usage (lag 1) | 0.001 | –0.011 | 0.037 | ||||||
(0.009) | (0.016) | (0.021) | |||||||
Tract FE | Yes | Yes | Yes | Yes | Yes | Yes | Yes | Yes | Yes |
Year FE | Yes | Yes | Yes | Yes | Yes | Yes | Yes | Yes | Yes |
Controls | Yes | Yes | Yes | Yes | Yes | Yes | Yes | Yes | Yes |
Observations | 1,004 | 1,004 | 1,004 | 1,004 | 1,004 | 1,004 | 1,004 | 1,004 | 1,004 |
F | 0.62 | 0.16 | 0.04 | 0.8 | 1.32 | 0.79 | 8.7 | 2.69 | 1.56 |
Note: clustered standard errors are displayed in parenthesis. Control variable is median household income. Significance levels: * p<0.05; ** p<0.01; *** p<0.001.
10.6 Discussion
We have used the expansion of Airbnb across the neighborhoods of Boston, MA to illustrate an overarching framework for considering how sharing economy platforms can impact neighborhoods. Airbnb has epitomized the way that such platforms have changed the geographic locus of certain commercial transactions, often by expanding them from business districts into more residential communities. In turn, cities like Boston have seen Airbnb listings direct more and more tourists to residential areas. Many have expressed concerns at the externalities that this unexpected influx of visitors might create. Here we articulated and tested three specific hypotheses for what these might be: (1) encouraging greater investment in property; (2) expanding the local hospitality industry; and (3) increasing crime and social disorder.
The empirical test provided support for our first hypothesis as well as some suggestive evidence for our third. Neighborhoods with increasing prevalence of Airbnb listings saw more investment in property, as measured through building permit applications approved by the City of Boston. Meanwhile, we saw mixed evidence for the other two hypotheses. Part of this might be limitations from the outcome measures we used. In particular, counting new restaurants is a constrained approach that requires that the hospitality industry expanded sufficiently to support entire new businesses. Researchers using the more nuanced measure of restaurant employment in New York City did find that Airbnb led to localized growth in the industry (Alyakoob & Rahman, Reference Alyakoob and Rahman2019). Meanwhile, we see some initial evidence that Airbnb listings in a neighborhood are associated with increases in public violence in a neighborhood.
The suggestion that Airbnb might be associated with increases in public violence is intriguing, if preliminary. Admittedly, we sought here to illustrate a generalized framework for pursuing hypotheses on the externalities of sharing economy platforms. As such, this analysis and finding does not fully engage the more nuanced set of relationships between Airbnb and crime that we proposed earlier. To reiterate, Airbnb might impact crime through either of two pathways. First, tourists could directly raise crime, either by perpetrating crimes or acting as attractive targets for criminals. Alternatively, a density of Airbnb listings may undermine local social dynamics that mitigate and prevent crime. A seminal concept in criminology known as social disorganization theory posits that communities with high residential turnover will have difficulty creating and sustaining the relationships and norms necessary for limiting crime (Sampson, Reference Sampson2012). Consistent with this model, Airbnb is the most transient type of household possible, literally “turning over” every few days. To this end, the listings may leave gaps in the social fabric off the neighborhood that could permit crime to increase. Adjudicating between these two hypotheses requires a targeted conceptual approach and methods that attend to the nuanced differences between them. We conduct such a test in a recently published study in a separate venue (Ke et al., Reference Ke, O’Brien and Heydari2021). We do indeed find evidence that Airbnb listings lead to increased crime in neighborhoods by undermining the local social organization – not because they attract tourists, as one might assume. By undermining a neighborhood’s social organization, higher Airbnb penetration diminishes the natural ability of a neighborhood to counteract and discourage crime, specifically violent crime.
Our goal in presenting this study is to highlight three important considerations, one conceptual, one methodological, and one practical. Taking the conceptual first, the framework here provides a guide for examining how other sharing economy platforms might be impacting neighborhoods. The study of each will first need to determine if and how the platform in question is in fact shifting the geographic distribution of commerce. It then will need to proceed to reasoning how those transactions might be altering existing behavioral or economic dynamics. In the current case, we reasoned that Airbnb highlights the value of local housing stock, thereby stimulating investment by property owners. We also discussed how the introduction of tourists might lead to more patrons at local restaurants, but also more disorder and crime. Similar logics would need to be developed for each sharing economy platform and might reveal that certain platforms would be more likely than others to have tangible impacts on communities.
Working through the first two steps of our conceptual process raises a crucial methodological consideration. How does one quantify the presence of a sharing economy platform in a neighborhood? In order to maintain brevity and avoid ambiguity, most scientific studies present and justify a single measure for a quantity of interest. This is a truism for science in general, and has become pronounced in recent years with the use of naturally occurring data sets, including but not limited to the data generated by sharing economy platforms (O’Brien, Sampson, & Winship, Reference O’Brien, Sampson and Winship2015). In contrast, we have taken the opportunity here to detail our own struggle to identify a single measure for the presence of Airbnb in a neighborhood, revealing that multiple measures are possible and that they are neither statistically nor conceptually equivalent. This highlights the challenge faced by researchers in this space and makes clear that the measures themselves need to be developed with care. Further, researchers need to be clear on the interpretation of the measure or measures they select. To illustrate this latter point, there are clear differences between the meaning of our three measures. Whereas high density could be created by a few large buildings with many units listed, penetration reflects a more general distribution of listings throughout a neighborhood. The latter might better reflect the exposure of many parts of a neighborhood and their residents to Airbnb. Meanwhile, the usage measure, which estimates the total number of visitors to the neighborhood, might be most relevant for hypotheses regarding the volume of impact of individual tourists.
Last, it is important to consider how this framework might be a useful tool as local governments look to manage and regulate the sharing economy. Presumably, the goal of such regulations is to maximize the benefits generated by the sharing economy and minimize the negative impacts. Research of the sort presented here will inform policymakers to the specific positive and negative externalities that should be taken into account when designing such regulations. Here we see that Airbnb is leading to more investment in neighborhood properties, which might be argued as a positive outcome. It joins, however, a wealth of evidence that the expansion of Airbnb listings can lead to increases in housing and rental costs and gentrification more generally. Municipal leaders need to weigh these costs and benefits alongside each other when determining how a regulation will impact neighborhoods.
Meanwhile, combining the results here with our further analysis elsewhere (Ke et al., Reference Ke, O’Brien and Heydari2021), we see that Airbnb can also lead to elevated levels crime and disorder in a neighborhood. But the crucial question of measurement returns, this time highlighting how intervention requires not only an understanding of the externalities of the sharing economy, but also of the mechanisms by which these externalities occur. Comparing different ways of measuring the presence of Airbnb in a neighborhood, the increase in crime appears to have been instigated not by the arrival of tourists, as many have assumed. Instead, the listings themselves create a transience that can undermine local social networks and the community’s ability to prevent crime. There have been arguments that these sorts of effects are nonlinear and are only visible as they reach certain thresholds (Clear et al., Reference Clear, Rose, Waring and Scully2003). Fittingly, the same has been demonstrated for gentrification (Hwang & Sampson, Reference Hwang and Sampson2014), which is the potential concern arising from increases in building permits.
With information like that provided by our analysis, cities might be able to develop more informed regulations. Complete bans of Airbnb listings are a blunt tool that foregoes any of the possible benefits they might offer, like stimulating local businesses. Cities like Boston, MA, should be applauded for pursuing a middle ground, but the question is whether they were designed to best support communities. Boston, similar to other locales, has placed the focus on limiting property owners to renting out only one unit in an owner-occupied building at a time. This does limit the overall number of listings, but it has its weaknesses. At the extreme, it could lower density of short-term rentals in the neighborhood while permitting maximal penetration across its parcels. The results in studies based on the suggested framework here (Ke et al., Reference Ke, O’Brien and Heydari2021), would suggest that the negative externalities of the listings could still take hold in such a situation. Policymakers might instead consider quotas of parcels within a neighborhood that can have one or more short-term rentals. In conclusion, this framework can support well-crafted empirical research that then can be translated into well-designed policies. This is critical as it becomes increasingly clear that sharing economy platforms will be a major component of the commercial landscape for the foreseeable future.
11.1 Introduction
Engineering advances have been opening new possibilities for sharing electric energy. Technological and social innovations in the electric energy sector may allow consumers to become more actively engaged in producing and managing the generation, distribution, and use of their electricity, which could shift the locus of organizational decision making and control away from traditional utilities. These innovations also have potential to diversify and restructure who is included and excluded from energy sector benefits (Stephens, Reference Stephens2020).
The transition toward a “sharing economy” in the transportation and lodging sectors, and in other emerging sharing economy systems, can be understood as a process of separating rights of use from the other rights of ownership for goods that had previously not been as easily divisible in this manner. For example, app-based short-term lease platforms have disrupted the lodging sector by enabling owners to create new value by more easily assigning the rights of use of their dwellings, and consumers now have new options of affordable unique rentals as an alternative to owning a vacation home. In the current traditional deregulated energy system, ownership of energy generation infrastructure is centralized along with allocation of rights of use. Generally, consumers did not own energy assets and had little power to consume the type (for example, traditional vs clean and renewable) or price (for example, fixed rate, time-of-use) of energy that aligned best with their economic and social motives. Sharing economy ideas combined with new technology promise to decentralize energy generation thus increasing ownership and opening broader markets (for example, demand response, community generation, and resilient microgrids).
Sharing economy innovations in the electric grid, including community solar and energy blockchain systems, are expanding the role of assets owned by consumers. These innovations are transitioning the role of generation and management from large corporations and utilities to consumers. This restructuring has potential to democratize energy systems depending on how policy and regulations guide the development of a more distributed renewable-based society (Stephens, Reference Stephens2019). As households and businesses become prosumers, they are producing and sharing surplus energy with the grid and other users, creating what some are referring to as “locavolts” linked in microgrid systems to enhance electric energy resilience and to leverage local financing. Prosumers who can “share” their electricity may also be empowered to change the rules that have governed their relationships with utilities for the past century.
At the same time, technological innovations related to renewable electric energy generation, distribution, and demand-side management may enable new types of energy sharing with the potential to disrupt and transform the current electrical energy industry. In the same way that Uber and Airbnb have disrupted existing transport and hospitality industries, we suggest that the electricity energy sector may also be trending toward large-scale system reconstruction by way of innovations for energy sharing. In recent years, one outcome of these energy sharing innovations has been the rise of a new group of energy platform operators (for example, thermostat companies and other third parties) acting as “energy services providers” that position themselves as the electricity sharing economy platforms facing consumers. Many have identified the “death spiral of the utilities’ business model” in which the traditional fee for energy delivery that utilities charge to customers fails to provide adequate revenue from prosumers who use the grid only for short periods of peak power (Felder and Athawale, Reference Felder and Athawale2014). These periods of peak power, for example, in the early evening when the solar resource diminishes and residential demand increases, drive infrastructure costs. Energy platform operators may enter the power sector as new market players that aggregate small amounts of distributed energy resources (DERs) provided by each user into megawatts that can be traded in the traditional regional energy, capacity, and ancillary services markets.
Future energy systems are likely to integrate a regionally appropriate mix of renewable electricity generation that is dispatched, stored, and distributed through platforms that enable sharing at multiple scales, from milliseconds to decades (Stephens, Reference Stephens2019). The potential for sharing in electricity systems is expanding as energy systems around the world are transitioning from centralized large fossil fuel power plants to distributed renewable-based power generation at multiple locations (Stephens, Reference Stephens2020). Such flexibility in time scale and locations is critical, as the success or failure of any organization or individual operating in the sharing economy is determined by its ability to effectively manage an elastic match between resource supply and demand. Sharing in future electric systems has the potential to disrupt relationships governing utilities, energy consumers, and distributed electricity generation at the individual and household levels, at the community and organizational levels, and at the regional, state, national and even international levels.
This chapter reviews the range of institutional models for electricity generation and distribution; considers how electrical energy systems are undergoing a transformation toward more distributed, renewable-based configurations; and explores the multiple evolving mechanisms for energy to be shared among generators, distributors, and consumers. We present a typology of sharing economies in electric energy systems and identify the technological and sociopolitical potential for sharing electrical energy as systems evolve. The chapter also explores policy considerations that flow from such large-scale transformations in the energy sector. The chapter concludes with opportunities for future research and questions about the implications of energy sharing for energy justice.
11.2 Background on the Electric System Structure, Business Models, and Regulatory Frameworks
Over time, a wide range of institutional models, shaped by both technological and sociopolitical factors, have emerged for provisioning energy for households and industry (Stephens et al., Reference Stephens, Wilson and Peterson2015). Regulatory frameworks have evolved in parallel with transformations of technology and business models. It is important to understand how these transformations evolved over the last century, and the sociotechnical factors that led to them, to understand the disruptive potential of sharing economy innovations in the electric power sector.
Traditional utilities are structured as regulated monopoly providers of electrical power and grid services for a specific geographic area (Kiesling, Reference Kiesling2014). The role of utilities is to deliver power to satisfy consumer demand, which may require the utility to generate, transmit, store, and/or purchase power and regulate its frequency and/or voltage. Early on, utilities faced steep barriers to market entry, because power plants and transmission lines were very expensive. Consequently, when household electricity was introduced in the United States in the early 1880s, it was available only in larger cities for wealthy homeowners and almost 90 percent of rural homes were without electricity in the first decades of the twentieth century (Velaga et al., Reference Velaga, Prabakar, Singh, Sen and Kroposki2019). Over time, growth in household energy demand drove down the average cost of delivered power. This combination of economies of scale and high barriers to entry led to the three dominant utility business models that operate in the United States today: (1) state-operated not-for-profit consumer-owned electric cooperatives, (2) local publicly run or managed utilities (POUs), and (3) investor-owned utilities (IOUs). Although there are relatively few investor-owned utilities, they tend to be very large, serving over 75 percent of utility customers nationwide.
Across the world, electric power markets operate in a variety of forms that lie on a spectrum from monopolization to democratization. The evolution of energy systems and markets around the world has been very heterogeneous. In some countries, deregulated markets were established through the restructuring of some of the existing utilities, with the intent to disrupt energy generation monopolies by increasing competition. In deregulated electric grids, electric energy, capacity, and reserves are each traded on a wholesale market, which must meet customer demands while satisfying any constraints of the transmission system (for example, line limits). Finally, in energy choice systems, energy users have the opportunity to select among multiple energy providers based on rates and energy generation options (see Figure 11.1).
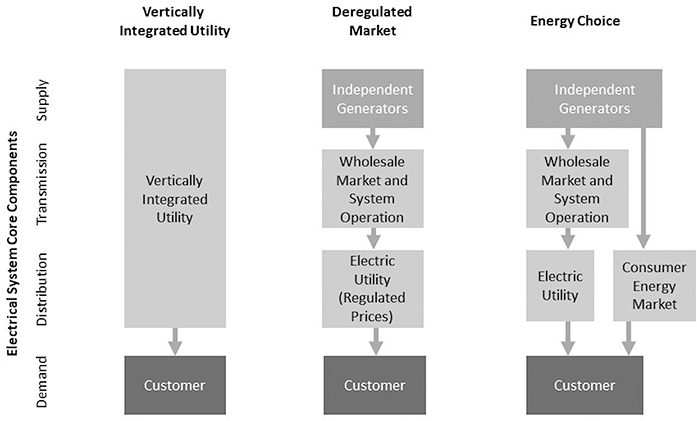
Figure 11.1 Three different systems for electricity generation, transmission, and distribution.
In the twenty-first century there have been considerable advancements in technologies for household electricity generation with renewables. These affordable distributed generation technologies are beginning to challenge the stability of the centralized electricity system (McKenna, Reference McKenna2018). There is a growing embrace of distributed energy resources enabled by new information and communications technologies such as blockchain technology, which enables trusted peer-to-peer distribution; and internet-of-things (IOT) devices, which enable real-time response to changes in supply and demand. These technological changes have precipitated the rapid growth of sharing economy businesses (Hamari et al., Reference Hamari, Sjoklint and Ukkonen2016). A primary argument in favor of the sharing economy model is that collaborative effort among participating actors leads to more efficient, sustainable, and resilient outcomes than centralized decision making (for example, by utilities).
Continued global population growth and resource-intensive consumption practices have increased energy demands, contributing to the global climate crisis. In response, a growing number of consumers are beginning to look for opportunities to participate in alternatives to the current electric energy systems that promise improved efficiency, reduced fossil fuel emissions, and more local control over energy system decision making. There is tremendous disruptive potential associated with sharing economy innovations that will enable a broader group of actors to produce, transmit, store, and consume electric power with each other.
11.3 Taxonomy of Electric Energy Sharing
In this section we explore key trends in sharing of electric energy, looking at forms of sharing that have emerged based on market structures and services being provided.
At face value, electric energy may appear to be a perfectly fungible commodity that can be produced by a variety of methods, transmitted across nations, and consumed and billed through meters. In reality, electric energy markets face unique challenges compared to most other sharing economies, in that the product being traded is not discrete (compared with a ride between two points provided by a ride-hailing service or two nights in short-term rental), cannot be easily stored (generation must match consumption at every instant in time, unless expensive batteries or other storage systems are utilized), and transferring the commodity requires specialized infrastructure (for example, wires and transformers) that have limited capacity (for example, circuit breakers on distribution lines can trip if a large load overheats a transformer).
To facilitate the discussion of the sharing economy in the electricity sector, it will be helpful to classify various types of “sharing” by the market structure: Top-down, in the form of a vertically integrated utility, or bottom-up, where individuals govern the market forces. Additionally, it is important to identify what is being bought and sold in different arrangements of energy sharing: Energy (for example, kilowatt-hours), ancillary services (that is, voltage and frequency control, generator dispatch, operating reserves), storage, and/or transmission.
Figure 11.2 represents a framework and taxonomy of sharing economy approaches in the electric grid along two dimensions – market structure and services provided. The services encompassed by a sharing economy may only include energy, or all of the services required to operate an electricity system (including energy, voltage and frequency control, and asset management). Along the other dimension, the prices and availability of services may depend on the market structure.

Figure 11.2 Taxonomy of sharing economy approaches in the electric grid.
In traditional utility-control approaches to energy sharing, consumers do not generate electricity; instead, they provide value through their flexibility to change demand. This is realized through demand response programs that ask users to modify behavior, or automatically adjust electric loads, for example, by turning off large energy loads such as air conditioners during times when the grid might overload. Similarly, time-of-use pricing enables users to shift their energy use to the times when low-cost, sometimes renewable, energy is available and can be easily transmitted to the consumer. However, such demand-side management approaches have limited capabilities since electricity demand is relatively inelastic to price.
Recent advances in regulatory structures and technology allow consumer choice in which consumers can choose which wholesale provider they buy their power from or generate power themselves. They then sell the excess energy (calculated over hourly, daily, or monthly periods) back to the grid through the wholesale power agreement of their distribution utility. Energy choice legislation in the form of the Energy Policy Act of 1992 enabled consumers to choose who they purchased their energy from, even specifying that only renewable energy be provided. As far back as 1983, consumers with the ability to produce electricity, primarily through rooftop solar, could share any excess, and receive the market value of that energy through net metering.
More recently, peer-to-peer energy sharing uses decentralized ledgers (energy blockchains, such as LO3 Energy) that track energy consumption, storage, and generation through a digital platform and enable users to choose who produces or consumes energy, and when, how and for how much the electricity is produced or consumed. The challenge in the future of the electric energy sharing economy is how to share all aspects of operations, safety, and management of the electric energy system.
Community microgrids promise to realize this future through breakthroughs in automation, machine learning, and digital platforms with both digital and physical aspects that enable sharing of distribution systems, or a fully shared grid. Community microgrids rely heavily not just on shared generation, including large amounts of renewables, but also on shared services through distributed energy resources (DERs). These DERs may include energy storage in parked electric vehicles, smart thermostats that run only when energy is available, and smart solar inverters that can help stabilize voltage and frequency on the shared wires. This sharing of the responsibility to balance supply and demand reduces costly peaks and transmission costs and creates a more resilient electric energy supply that can continue operating even when the larger grid suffers a blackout (Poudel & Dubey Reference Poudel and Dubey2018).
The following sections describe in more detail these four approaches to sharing economies in electric energy systems, moving clockwise around Figure 11.2 from the status quo utility control to future shared grids.
11.4 Utility Control – Demand Response and Time-Of-Use Pricing
Utility control describes top-down (centralized) management of grid services and reserves. In this arrangement, utilities are responsible for all grid services, including managing capacity of transmission lines and ancillary services that may include energy storage or controlling the readiness of power plants to begin producing energy to match demand.
Demand response innovations give some responsibility to energy consumers to help manage the electric grid. For example, during a time of high energy demand that might historically have led to a temporary partial shut off the grid (brownout), consumers can instead respond to signals from the energy provider and suspend operation of nonessential appliances, such as air conditioning units or pool pumps. This enables energy service to be maintained for essential functions and reduces total peak electrical load, reducing the need for costly increases in electric grid capacity. Such demand response (DR) may be fully automated with smart appliances (such as Google Nest) that adjust operation schedules based on signals from the utility or can simply take the form of utilities sending customers texts or other communications to encourage reducing energy use at specific times to avoid overloading the grid (for example, “Shave the Peak!”).
Time-of-use pricing involves changing the electricity rate based on current demand, more closely reflecting real-time energy prices on the wholesale market. For example, customers might be charged a much higher rate to use energy during times of the day when aggregate demand is at its peak. This time-of-use pricing incentivizes consumers to adjust their energy consumption for more efficient operation of the grid. Time-of-use pricing enables users to shift their energy use to the times when low-cost, sometimes renewable, energy is available and can be easily transmitted to the consumer.
Since electricity has become an integral and necessary part of modern life, electricity demand is largely inelastic, therefore limiting the impact of pricing policies on demand (Lee and Chiu, Reference Lee and Chiu2011). Furthermore, price elasticity is nonlinear and asymmetric, complicating the efficient implementation of such dynamic pricing approaches (Haas and Schipper, 1998).
11.4.1 Role of the Users
In these examples of utility control energy sharing, innovations enable consumers to share the value of their flexibility to change aggregate demand in real time. Both demand response and time-of-use pricing can work automatically or by active participation of consumers. Consumers can potentially benefit from sharing by avoiding situations where electrical power would otherwise be interrupted and by reducing their electrical energy bill by adjusting their behavior based on signals from the utility service provider. These utility control sharing mechanisms can be a considerable benefit to customers who have the flexibility and information access necessary to vary their energy usage in response to market signals. However, since the average household spends only 2 percent of its income on electric energy, and various consumption-specific social and behavioral factors make household-level flexibility difficult, the elasticity of energy consumption is low (Drehobl and Ross, Reference Drehobl and Ross2016; Wilson and Dowlatabadi, Reference Wilson and Dowlatabadi2007). Further, as low-income households may spend up to 30 percent of their income on electric energy, demand response and time-of-use pricing may place an inequitable burden on the already disadvantaged if they lack technology or flexibility necessary to adjust energy usage in response to market signals (Drehobl and Ross, Reference Drehobl and Ross2016).
11.4.2 Role of the Platform
For utility control of energy sharing, the utility, whether a private company, consumer-owned cooperative, or state-owned public company, functions as the platform for coordinating all sharing activities. Alternatively, demand response providers may be third-party commercial entities that aggregate the load flexibility of their customers into bids for the wholesale market. This motivates these third parties to help customers with strategies or technology to adjust their electricity consumption in response to market signals.
11.4.3 Notable Examples
Most US utilities offer both commercial and industrial customers options for centralized energy sharing in the form of demand response. Each of the nation’s independent system operators/regional transmission organizations (ISO/RTOs) sponsor demand response programs (Department of Energy [DOE], 2019). The Federal Energy Management Program (FEMP) helps federal agencies and other organizations identify opportunities for energy project incentives and demand response programs, execute these opportunities, and fully capture their benefits (DOE, 2019). Most utilities offer time-of-use pricing options as well: These program structures include simple time-of-use rates, where prices change at set times through a 24-hour cycle to reflect afternoon peak electricity use, overnight off-peak hours, and the shoulder periods in the morning and evening hours. Other variations of time-variable pricing include real-time pricing in which customers’ rates reflect the wholesale electricity market or the utility’s cost of production; day-ahead hourly pricing, where the utility sets prices to reflect the cost of acquisition or production for the coming day; and block-and-index pricing, in which the customer can lock in set energy prices for part of their energy use and pay current market price for additional usage.
11.5 Consumer Choice – Energy Choice and Net Metering
In the context of top-down (centralized) management of energy, without the complexity of real-time control of the grid, various forms of consumer choice energy sharing are possible on hourly, daily, or monthly timescales. These are distinct from what we have described in the previous section in that they engage the consumer in financing distributed energy resources (DERs), either on-site or remotely. DERs are decentralized because they rely on energy sources other than the utility to provide energy, and such sources can and often do include consumers themselves. DERs are not an innovation whose primary purpose is to manage constraints on the electrical grid. Instead, their primary benefits are to allow prosumers to buy energy from their preferred source (for example, renewables), reduce their bills through efficiency upgrades, and/or sell excess renewable energy they generate back to the grid at favorable prices.
Energy choice programs enable consumers to choose who they buy their energy from. For example, they might pay a premium for renewable electricity from wind turbines in another state. While this choice doesn’t directly affect the “source of electrons” transmitted to the household user, the arrangement directly finances clean energy production. In these wholesale markets, consumers are buying from a corporate producer of energy, such as a large wind farm aggregator or financial entity specializing in trading energy.
As far back as 1983, consumers with the ability to produce electricity, primarily through rooftop solar panels, could share any excess, and receive the market value of that energy through net metering. In net metering, consumers have the opportunity to produce energy locally and use their energy first, selling any excess back to grid, typically averaged over the day or the month. Sometimes energy sales back to the grid are managed on a yearly basis, and very rarely on an hourly basis. These systems give consumers the option to choose renewable energy for their own consumption and reduce dependence on the utility provider, thus leading a transition toward renewable energy generation. Electricity consumers who generate their own energy on-site, sell a portion of that energy back to the grid, and store energy or otherwise control their load, are called prosumers. Figure 11.3 depicts a generalized scheme for how energy choice and net metering systems interface with traditional utility businesses.

Figure 11.3 How energy choice and net metering systems interface with traditional utility businesses.
11.5.1 Role of the Users
The act of producing energy locally and sharing it back to the grid transforms “energy consumers” to “energy prosumers.” A growing body of research explores prosumers’ behavior and interactions with the electrical energy system. A survey of prosumer perceptions found that prosumers are motivated to collaborate with utilities to contribute to the societal goal of creating more renewable energy (Silva et al., Reference Silva, Karnouskos and Ilic2012). Information sharing and communication innovations are key to the successful management of energy sharing in prosumer engagement systems (Zafar et al., Reference Zafar, Mahmood, Razzaq, Ali, Naeem and Shehzad2018). However, since prosumer systems that require the individual to own an asset (for example, rooftop solar) are in general limited to those individuals that own their home, renters and other low-income citizens find it difficult to benefit from prosumer engagement, aside from energy choice.
11.5.2 Role of the Platform
In the case of the prosumer systems based on energy choice markets and net metering, traditional utility companies maintain all physical hardware and assets of the energy distribution system and real-time controls, while prosumers begin increasing their share of ownership or investment in energy generation assets. Energy choice and net metering programs do not specifically address the challenge of managing the electrical load on the grid to match supply with consumer demand. A growing body of research focuses on the problem of peak shaving, that is, reducing the maximum points of electricity demand. An index termed the sharing contribution rate (SCR) quantifies users’ contributions to energy sharing and peak shaving (Wang et al., Reference Wang, Zhong, Wu, Du, Xia and Kang2019).
11.5.3 Notable Examples
Globally, a broad array of programs for energy choice and net metering are emerging. In the United States, over a dozen states have deregulated electricity markets, meaning that electricity providers can compete to sell energy to consumers. One example of a platform that provides individual energy consumers with the opportunity to invest in solar energy projects is Mosaic, a solar lending platform. Traditionally, only individuals with sufficient starting capital could invest in household solar panels. However, new technologies have improved the efficiency and lowered the cost of manufacturing, installing, and permitting solar energy. Solar lending companies enable consumers to become prosumers by financing their initial solar panel installation, and then collecting back the loans over time with interest as these prosumers sell energy back to the utility. New platforms have emerged to connect investors with borrowers seeking financing for solar power projects.
11.6 Peer-to-Peer – Community Solar and Blockchain
Recent advances in regulatory structures and technology have enabled community solar programs in which solar panels or other DERs are installed on shared land, while dividends for the energy produced are paid out to the community. Even more recently, energy blockchains have emerged, functioning as decentralized ledgers that track energy consumption, storage, and generation through a digital platform and enable energy consumers and producers to choose when, how, by whom and at what price their electricity is purchased and sold.
Community solar and blockchain-based energy sharing are examples of peer-to-peer electricity sharing that integrates decentralized management of energy systems and does not directly address the technical considerations associated with real-time control of the electrical grid. In these systems, transmission lines and distribution infrastructure are still managed by the traditional utility. Because there are substantial costs to operating the grid, such as controlling voltage and managing storage, electricity consumers in these types of peer-to-peer arrangements continue to pay utilities for use of their infrastructure (but not for the generation of electricity).
11.6.1 Role of the Users
When prosumers and consumers trade self-produced energy in a peer-to-peer manner, they can both profit, and this could provide incentives for different kinds of investments. Previous research indicates that compared to traditional peer-to-grid models of energy sharing, peer-to-peer electricity sharing may confer economic benefits to consumers and environmental benefits in the form of carbon footprint reduction (Secchi and Barchi, Reference Secchi and Barchi2019).
11.6.2 Role of the Platform
In peer-to-peer sharing, the utility still manages (although, may not own) the physical assets to satisfy real-time needs. Recent research explores the potential for blockchain-based microgrid energy markets that do not require central intermediaries to facilitate transactions between producers and consumers, thus reducing costs associated with these traditionally labor-intensive markets.
11.6.3 Notable Examples
Examples of peer-to-peer sharing include community choice aggregation programs, as well as more sophisticated electricity trading platforms. One example of an electricity trading platform is Vandebron, a startup in the Netherlands that allows people to buy energy from independent producers (Vandebron, 2020). The mission of this company is to connect people who have surplus renewable energy with energy consumers who are not producing their own electricity. Utilities are not involved in the transaction. Functioning as an “Airbnb for electricity,” this platform allows energy consumers to search for producers. For example, a consumer could share their information on the platform to arrange for the purchase of their power from a farmer in a neighboring community who has wind turbines or solar panels in their fields. Such business models may be easier to implement in deregulated energy markets, such as the Netherlands, in contrast to other countries and many states in the United States (Schiller, Reference Schiller2014).
While the case has been made that blockchains are an effective technology to decentralize the energy system, to date there are only limited examples of blockchain-based, local, peer-to-peer energy sharing operating in practice (Mengelkamp et al., Reference Mengelkamp, Gärttner, Rock, Kessler, Orsini and Weinhardt2018; Noor et al., Reference Noor, Yang, Guo, van Dam and Wang2018). The Brooklyn Microgrid project (BMG) is one such example (Brooklyn Microgrid, Reference Microgrid2020). Note that this example differs from true shared grid systems described in detail later, because while consumers are able to trade and share energy with fellow consumers in the BMG project, the energy distribution infrastructure is owned and managed by a centralized utility. The network connects prosumers and consumers by enabling people to buy and sell locally generated renewable energy through a Brooklyn Microgrid mobile app, which gathers and records energy data for users. Through blockchain technology, BMG developed Exergy, a data platform that creates localized energy marketplaces for transacting energy across existing grid infrastructure. The BMG also acts as an educational and community engagement initiative: For example, the organization facilitates workshops in partnership with public schools in New York City to teach community members about the technology behind electric microgrid systems and encourage broader participation in energy sharing.
11.7 Shared Grid – Community Microgrids
When shared ownership of electricity generation infrastructure is combined with shared ownership of distribution and energy management infrastructure, these systems can be classified as a shared grid. Shared grid systems are characterized by decentralized management of real-time energy generation and advanced grid controls, made possible by breakthroughs in automation and machine learning. They promise improved physical reliability and resilience to cyber threats, opportunities to improve energy system sustainability, reduce reliance on fossil fuels, and improved economic efficiency. These innovations help scale and reduce the costs associated with the hundreds of people required to run a regional grid, to a handful of contractors to operate a microgrid.
Community microgrids rely heavily not just on shared generation or renewable energy, but also on shared grid management services provided by distributed energy resources (DERs). These DERs may include energy storage in a parked electric vehicle, smart thermostats that run only when energy is available, and smart solar inverters that can help stabilize voltage and frequency on the shared wires. Thus, DERs represent a pathway for sharing the responsibility to balance supply and demand among all participants in the system. When integrated into a community microgrid system, these technologies have the potential to reduce costly peaks in energy demand as well as transmission costs. Many researchers also argue that community microgrids are a viable solution for more resilient local electric energy systems that can continue operating even in instances when the larger national or regional electric grid suffers a blackout (Jiménez-Estévez et al., Reference Jiménez-Estévez, Navarro-Espinosa, Palma-Behnke, Lanuzza and Velázquez2017; Marnay et al., Reference Marnay, Aki, Hirose, Kwasinski, Ogura and Shinji2015; Wu et al., Reference Wu, Ortmeyer and Li2016).
11.7.1 Role of the Users
In a shared grid system, all users contribute to the cost of grid management. There are various ways that such cost sharing might be structured in real-world shared grid systems. For example, third-party platforms, decentralized algorithms, and/or self-governed user decisions can determine how the resources are managed.
11.7.2 Role of the Platform
In shared grid systems, the role of a traditional utility significantly diminishes. When energy generation, assets, and management of the grid are controlled by third-party platforms and decentralized algorithms that integrate a system of DERs, electrical energy systems can potentially function without any involvement of a centralized utility. A market of third-party platforms may even operate simultaneously in the same way that Uber and Lyft both operate in the same city. This future vision for shared grid systems requires, however, new technologies for information sharing between users in the system. For example, the algorithms needed to control millions of household-scale renewable energy generators and the load at millions of homes is very different than the algorithms that control load and generation in the traditional centralized generation system.
In addition to developing information sharing technologies to facilitate operation of shared grid systems, some scholars regard resource optimization technologies to be critical for continued innovation of smart grids. For example, Zafar et al. (Reference Zafar, Mahmood, Razzaq, Ali, Naeem and Shehzad2018) discuss linear and nonlinear optimization programming in the context of prosumer-based energy management and sharing. Other researchers present the optimization technique in a two-stage approach. For instance, Cui et al. (Reference Cui, Wang, Xiao and Liu2019) argue that the bi-level optimization problem could be transformed into a single-level mix integer linear programming problem through proper linearization techniques. In the second stage, an online optimization model is proposed for each prosumer to make the energy schedule according to the latest system situation and prediction error (Cui et al., Reference Cui, Wang, Xiao and Liu2019). Alternatively, Long et al. (Reference Long, Wu, Zhou and Jenkins2018) present a nonlinear programming optimization in the first stage with a rule-based control to update the control set-points through real-time measurement in the second stage.
11.7.3 Notable Examples: Community Microgrids
To date, most examples of shared energy generation and grid management can be found in institutional microgrids. The best examples of shared grid systems can be found at US military bases, where there are systems in place for coordinated management to govern when and how system users produce and consume energy (Hossain et al., Reference Hossain, Kabalci, Bayindir and Perez2014; Prehoda et al., Reference Prehoda, Schelly and Pearce2017). Shared grid examples also exist in hospitals and corporate landscapes. For example, East Boston has a microgrid network made up of institutions that share renewable electricity generation and backup generators among the buildings (Sheehan, Reference Sheehan2015).
Looking ahead toward the possibility of more shared grid systems being implemented throughout the US and globally, there is considerable potential for increased production of renewable energy and efficient management of supply and demand enabled by DERs and algorithms for real-time grid management. However, while these innovations suggest potential benefits of the sharing economy for the electric energy sector, there are important access and equity considerations to be addressed. In a future where corporations and wealthy neighborhoods shift toward community microgrids, less wealthy and powerful energy consumers could suffer negative consequences. If a subset of energy consumers invests in their own microgrid’s reliable distribution and generation infrastructure, they are no longer effectively investing in the reliability and efficiency of the whole electrical energy system. Such a trend in consumer behavior would effectively create islands of energy resilience in a sea of energy vulnerability.
11.8 Policy Considerations
In the future, in much the same way that sharing economy platforms such as Airbnb have simplified the process of contracting with an independent short-term lodging provider, electricity sharing has the potential to radically change how electricity consumers interact and behave. Indications of the future impacts can already be observed in the form of smart devices such as thermostats, operated by third-party platforms, that optimize their demand based upon real-time energy markets.
In earlier chapters, authors have discussed how the rise of the sharing economy in some sectors may increase consumption without improving sustainability. However, in the case of energy sharing, we anticipate a different trajectory that does not lead to increased consumption of fossil fuels. In part, this is because energy sharing is connected with a larger transformation toward renewable energy and a reduced carbon footprint.
The other benefits of utility control, consumer choice, and peer-to-peer energy system innovations primarily involve the reduced cost of energy generation and delivery. These reduced costs and impacts could theoretically result in an increase in energy consumption, but due to the price inelasticity mentioned earlier, we do not expect energy sharing to significantly impact energy consumption rates. That is, while reducing the price of lodging (for example, with the growth of Airbnb) may lead to more travel, energy consumption is unlikely to increase appreciably as the cost of energy goes down, particularly in developed economies (US Energy Information Administration, 2021). Any future increases in electricity consumption will instead likely be driven by increased adoption of electric vehicles and heat pumps as opposed to the specific influence of the sharing economy on energy. Finally, even with the rise of smart metering, demand response, and time-of-use pricing, there is relatively limited transparency in energy pricing. For example, the average consumer will most likely not be aware of the cost of running their dishwasher. This is due to the traditional monthly electricity billing cycle, temporally separating the saliency of the energy costs from the energy consumption occasions. New real-time IoT devices bring the potential to better synchronize consumption and costs; however, care must be taken to not overload users with too much information in real-time.
The potential societal benefits of a transition toward peer-to-peer and shared grid energy systems include improved efficiency, an increased share of renewable energy production, and more consumer engagement. In addition, these new mechanisms for local control and management provide individuals, households, and communities with more economic and political power as well as electrical power. Thus, increased energy sharing can be understood as a pathway toward realizing the goals of the energy democracy movement. Originating from trade union activism, energy democracy focuses on restructuring the political, economic, and social makeup of the energy system by transitioning to renewables while establishing democratic energy decision-making processes, equitable access to energy and energy-ownership for marginalized groups, and widely distributed and renewable energy resources (Sweeney Reference Sweeney2012; Burke and Stephens Reference Burke and Stephens2018).
However, while sharing economy innovations enable consumers to have more say in where and how their electricity is produced, there are possible negative consequences associated with a transition to a shared grid and associated “energy democratization” (Stephens et al Reference Stephens, Wilson and Peterson2015). Increased development of community microgrid systems could potentially lead to greater inequality in access to reliable and affordable electric energy. The traditional electric grid doesn’t just provide electric energy, it provides electric energy reliability. That reliability is critical to economic productivity and, in some cases, social wellbeing. If the transformation underway in the electric energy sector does in fact represent a death spiral of the utility business model, the result could be that only wealthy energy consumers could afford to have reliable community microgrids, and fewer resources would go toward maintaining the grid. This could lead to a decrease in energy reliability for consumers who do not have access or cannot afford to participate in microgrid systems. One possible solution would be to increase public investment in shared grids for communities who may not otherwise have access to emerging energy sharing systems. This may in practice resemble the move in recent decades toward public–private partnerships that support charter schools in low-income communities (Koirala et al., Reference Koirala, Koliou, Friege, Hakvoort and Herder2016).
Another way that energy sharing is tied to disruption and transformation in the electric energy sector is through altering the geographic distribution of energy generation sites (Stephens Reference Stephens2019). Historically, power generation occurred near coal reserves or at hydroelectric facilities. With increased local solar- and wind-powered electricity generation, preferred sites for power generation are changing and power lines built for transmission over long distances are no longer optimally placed, leading to increased potential for congestion. However, building new transmission lines is exceedingly expensive. There is a market opportunity to invest in reducing energy consumption demand. For example, it is cheaper to give away smart thermostats and selectively adjust them automatically during peak demand times than it is to build a new transmission line. This example points to just one of the ways that new technologies and changing social and economic priorities may transform the electrical energy sector.
11.9 Conclusions
As the pace of transformation in electrical energy generation and distribution systems continues to accelerate, the possibilities for innovative ways to “share” electricity are rapidly evolving. This chapter has reviewed this dynamic landscape and provided a taxonomy to characterize different modes of energy sharing. There is a transition underway away from legacy systems with high levels of utility control and toward new opportunities for prosumer engagement, peer-to-peer sharing, and ultimately in the direction of fully integrated sharing of all aspects of electricity generation and grid management. In this transition from utility control, to prosumer engagement, to peer-to-peer sharing, toward a decentralized market structure and integrated end-to-end management energy and assets, we recognize a possible end of the traditional utility business model. As third-party platforms for energy sharing and technologies enabling local renewable energy generation, storage, and supply and demand management systems emerge, we anticipate that the traditional integrated power supply and grid management roles of utilities may shrink across many regions.
In addition to a rise in prosumers, who are both energy users and energy generators, future energy sharing could also lead to a growing number of passive electricity consumers who are integrated into community microgrids. We anticipate that prosumers, currently primarily homeowners who own private renewable energy generation infrastructure, will increasingly be outnumbered by energy consumers whose energy purchasing decisions are regulated by smart devices. For example, a customer who wants to charge their electric vehicle at their home will have their default charging algorithm set to only charge when electricity is most readily available or cheapest.
Previous research on energy sharing has focused on technological innovation and prosumer behavior, including both empirical case studies and theoretical analyses. This research provides a valuable foundation for future studies. There is a need for more research on policy mechanisms to promote and regulate sharing in real-world energy systems. Barriers to redesigning the current energy system include current regulations and policies, public awareness and acceptance of new technologies, and established corporations’ resistance to change, which can lead to manipulation of markets and disinformation campaigns that question the legitimacy and reliability of alternative energy systems. There is also need for more social science research on how sharing could contribute to more inclusive energy systems including opening up opportunities for women, people of color, and indigenous people who have been historically excluded from energy sector jobs and economic empowerment through energy systems (Allen et al., Reference Allen, Lyons and Stephens2019).
Government and regulatory bodies have an important role to play in facilitating a transition to increased electrical energy sharing. Policy makers could, for example, establish incentive programs in the form of subsidies for new energy sharing enterprises entering the market, and punitive measures such as a carbon tax or pollution charges for fossil fuel-based energy systems. By enacting policy measures such as these and providing an environment that supports fair competition, government regulatory bodies can facilitate increased energy sharing systems that balance the goals of energy efficiency, sustainability, resilience, and equity.
12.1 Introduction
Last mile delivery is the movement of products from a warehouse or store to their final delivery points, most commonly residential locations. One of the largest and growing components of last mile delivery operations is parcel delivery. The total volume of the parcel delivery market in the United States was 14.8 billion packages in 2018 [1], with the United Parcel Service (UPS) having the largest market share at 5.2 billion shipped packages [Reference Del Rey2].
E-commerce is a major contributor to the global parcel delivery market and is one of the fastest growing business sectors in the world. Retail e-commerce revenue is expected to rise to $6.5 trillion in the world and to $565 billion in the United States by 2023 [3, 4]. The number of packages shipped increases along with revenue growth. Amazon alone, which accounts for about 49 percent of all e-commerce in the United States [Reference Lunden5], shipped more than 5 billion items to its Prime customers in 2017 [6] and delivered more than 3.5 billion packages through its own delivery network in 2019, almost half of its total number of shipped packages [Reference Del Rey2]. The size of the last mile delivery market increases rapidly as the share of e-commerce in the whole retail industry continues to grow and new contributors to last mile logistics activities, such as food and grocery deliveries, that rely on the same last mile infrastructure emerge. It is thus a vital matter for many businesses to manage last mile operations efficiently.
Last mile delivery related costs are estimated to reach up to 53 percent of total delivery costs [Reference Dolan7]. There are several factors that make last mile delivery operations challenging and more expensive than the other parts of the delivery journey. One factor is that last mile delivery generally takes place within urban areas where the speed limit is lower and there is more traffic congestion. Hence more vehicles are required to deliver parcels by their due time. Furthermore, while multiple and cheaper transportation modes, such as those using railroads and waterways, are usually available for intercity logistics, mostly the only available mode for last mile delivery in an urban area is road transportation.
Meanwhile, the size and number of urban areas also keep growing. By 2030 the number of megacities, which are cities with a population of 10 million or more, is expected to rise to 41 [8]. High population density in cities leads to increased transportation activity and high emissions, as more people and packages travel. In 2017, the transportation sector was the largest contributor to greenhouse gas emissions production by generating 28.9 percent of greenhouse gas emissions in the United States [9]; Figure 12.1 compares sectors with high emissions.
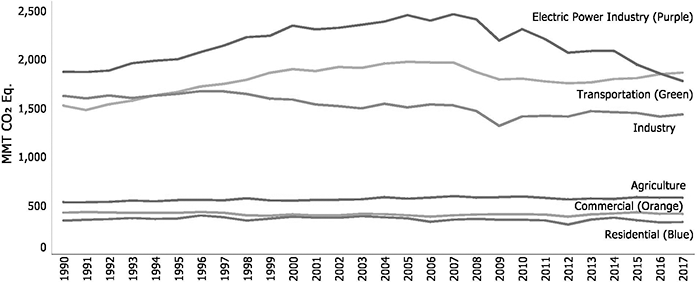
Figure 12.1 U.S. greenhouse gas emissions allocated to economic sectors (MMT CO2 Eq.) [9].
In general, objectives regarding increasing efficiency and reducing negative externalities may contradict each other. Furthermore, unexpected externalities, such as due to changing consumer behavior, may result from policy and/or operational changes leading to outcomes that are the exact opposites of the originally intended ones. For example, traffic congestion and emissions, two negative externalities of last mile delivery, are among the major concerns of city administrations, because they reduce residents’ quality of life. Shared last mile delivery attempts to make the logistics operations in urban areas more efficient and also is thought to have the potential to reduce negative externalities such as emissions by reducing the number of vehicles in traffic and by converting deliveries performed by trucks to crowdsourced cars since cars produce less emissions than trucks. However detailed analysis of some of these sharing-based last mile logistics systems have shown that opposite impacts might occur under certain city and service system characteristics. It is observed that in a subset of last mile logistics systems using crowdsourced drivers increases congestion and emissions because the small carriage capacity of cars ends up increasing the number of vehicles required and the deadheaded distance to deliver all packages [Reference Qi, Li, Liu and Shen10]. Therefore, these systems and the characteristics of the environment they exist in must be analyzed comprehensively and designed and operationalized in a way that creates a win-win-win outcome for businesses, customers, and the society.
There are different applications of crowdsourcing and sharing economy practices in last mile delivery. Since they differ from conventional business models they introduce new system characteristics to be considered in both practice and research. In this chapter, we briefly introduce the existing and potential sharing economy business models in last mile delivery, and discuss the aspects that distinguish them and make their operations more complex to manage.
12.1.1 History of E-Commerce
Home shopping existed before the Internet was widely used by the public. People could buy products via phone calls. They could choose a product they saw in catalogs or TV or newspaper advertisements and order it by calling the number given. In 1994, around 98 million consumers made $60 billion worth of home shopping purchases, and almost all of the purchases was ordered through phone calls [Reference Tuttle11].
The history of e-commerce transactions and online bookstores goes back to as early as 1991 and 1992 when Computer Literacy Bookstores and Book Stacks Unlimited started selling books online through email and their websites [Reference Van Schewick12]. The first item sold online through a secure and encrypted system using a credit card was made possible by Dan Kohn, a 21-year-old entrepreneur. It was the compact disc “Ten Summoners’ Tales” by the rock musician Sting and was sold through the website of Net Market Company of Nashua [Reference Arcand13]. The transaction took place on August 11, 1994, and it was important enough to be featured in the New York Times the next day [Reference Lewis14]. In 1995, Amazon started as an online bookstore [15] and eBay (starting as AuctionWeb) was established as an online auction and shopping website [16].
Since 1995, many online shopping websites have opened. The revenue from e-commerce continues to grow as the number of people who use the Internet grows as shown in Figures 12.2(a) and 12.2(b). The only period e-commerce retail revenue did not grow significantly was 2008–2009 due to the 2008 financial crisis (see the GDP growth in Figure 12.2(c)). It is clear, by comparison of Figures 12.2(a) and 12.2(c), that even a recession could not bring a sustained halt to the growth of e-commerce sales; in fact, in terms of percentage of total US retail, online retail grew during the recession [17].

Figure 12.2 (a) E-commerce retail sales in the United States between 2002 and 2018 [17], (b) percentage of adults who use the Internet in the United States between 2000 and 2018 [18], (c) GDP growth in the United States between 2000 and 2018 [19], and (d) the number of mobile device owners in the United States between 2012 and 2018 [20] (d)
12.1.2 History of Algorithmic Research for Optimizing Last Mile Delivery Operations
One of the earliest challenges of last mile delivery operations was faced by traveling salesmen, as they had to determine the order of visitation of potential customers’ homes or towns, often taking into account the products that they carried and the expected demand at each location. Peddlers have existed for more than 2000 years [Reference Friedman21]. After loading products, such as toiletries, clothing, and accessories, on their pack animals, or in vehicles in recent times, they travelled in one trip to several towns and villages to sell them. Both for the peddlers and the modern travelling salesman, efficiency often is equal to minimizing the cost of travel, which usually incorporates multiple components, such as energy and vehicle depreciation costs that are all a function of the total distance travelled.
The famous Traveling Salesman Problem (TSP) aims to optimize a traveling salesman’s route. The objective of TSP is to find the sequence of customers or towns to be visited which has the minimum travel cost. The sequence must begin and end at the same location and each location must be visited exactly once. The earliest known mention of the problem is in a handbook titled “The Traveling Salesman – how he should be and what he has to do to get orders and be sure of happy success in his business” published in 1832 [Reference Voigt22]. The first known work on the problem by a mathematician goes back to Menger’s work in 1930 [Reference Menger23], after which the attention of many mathematicians was drawn to the problem [Reference Schrijver24], especially after the 1954 seminal work of Dantzig, Fulkerson, and Johnson [Reference Dantzig, Fulkerson and Johnson25], as discussed in [Reference Little, Murty, Sweeney and Karel26–Reference Naddef and Rinaldi31]. Many variations of the problem have also been developed [Reference Gutin and Punnen32]. Nowadays, TSP is a well-studied problem among researchers and continues to attract the energies of both mathematicians and practitioners alike due to its complex and interesting theoretical nature and its applicability in a wide variety of real-world problems. The methods developed to solve TSP are the fundamental methods for solving many combinatorial optimization problems that aim to find the optimal solution in a countable set of solutions.
TSP was later generalized into the Vehicle Routing Problem (VRP), which first appeared in a paper by Dantzig and Ramser in 1959 [Reference Dantzig and Ramser33] and is the most common form of modeling last mile delivery optimization problems. The objective of the VRP is to find the minimum cost assignment of orders to a fleet of homogeneous vehicles and delivery routes for each vehicle starting from the depot and ending at the depot. Since 1959, an immensely rich literature has been established for VRP models and the development of exact, approximation, and heuristic algorithms to solve them (see [Reference Balinski and Quandt34–Reference Solomon41] for some of the early major works).
Many variants of VRP have been introduced over the years (see [Reference Laporte42] for a detailed history) to reflect the changing and complex characteristics of real-world delivery operations. The most important VRP variants are obtained by changing or relaxing some of the assumptions such as considering multiple depots, a heterogeneous fleet of vehicles, allowing both pickup and delivery at the destination locations, adding time-dependent costs, or removing the requirement for a vehicle to return to the depot. Furthermore, new constraints such as delivery (or pickup) time windows and load-balancing for all vehicles can be added to the problem. Finally, one can use different objective functions, such as minimizing fuel costs, minimizing emissions, and a combination of different goals to produce variants of the VRP. Mostly, these new variants of the VRP are proposed and then studied by researchers as a result of novel needs emerging from the markets by the development of new technologies or changing customer requests. For example, time windows, which are the time frame when a pickup or delivery operation must take place, are important in same day delivery; in crowdsourced delivery models, heterogenous vehicles must be considered, as drivers may have different cars; and time-dependent costs are common in urban mobility where the time to travel from one point to another point may drastically change depending on the time of day.
12.2 Sharing Economy Models in Last Mile Delivery
Digital platforms enable the interaction among different participants who supply or demand a set of services or products. Generally, sharing platforms themselves do not offer the services or products, but only match the available supply and demand. Matching as a service has been around for more than three millennia [Reference Evans and Schmalensee43]. However, large scale and rapid matching of supply and demand became available with the pervasiveness of the Internet and smartphones. The Internet enabled the exponential growth of digital platform economy businesses, since it lowered the entry barrier by not requiring a large-scale initial investment in hard assets. Especially after the 2008 financial crisis, a wide variety of online platforms started services such as DoorDash, Uber, TaskRabbit, and AirBnB. The growth of online platforms was also accelerated as smartphones became widespread and the development of mobile technologies and applications accelerated (Figure 12.2d).
Currently in shared last mile delivery, many businesses operate with different sharing models. In the following, we categorize different business models that currently exist or are being discussed as options for the near future.
12.2.1 Crowdsourced Delivery
Crowdsourced delivery platforms do not perform shipments with their own dedicated vehicle fleets or professional drivers. Individual drivers join these platforms as independent contractors, commonly after a security background check, and deliver the packages to customers using their own vehicles. In many platforms, crowdsourced drivers have different levels of flexibility in choosing when to start and stop working.
The landscape of last mile delivery platforms evolved over time. Different platforms, past and present, such as Deliv, GoShare, Instacart, GrubHub, and Postmates, provide last mile delivery using crowdsourced drivers for different types of products such as parcels, groceries, and meals. Furthermore, while some platforms focus on delivery of one type of product, others deliver multiple types. The type of product delivered impacts the constraints of service. For example, some groceries must be carried in cold boxes to prevent spoilage and must be delivered rapidly, while parcel delivery may have a longer delivery time period and can be heavier or bulkier.
Sharing economy delivery platforms also differ in terms of how they match delivery orders and drivers. Some platforms post the delivery orders with origin, destination, and earnings information. Drivers login to these platforms, check the posted orders, and can select the orders that they would like to complete. Other platforms perform the matching themselves given the availability of the drivers and time windows of the orders. They assign the orders to drivers so as to maximize the platform’s profit. Afterwards, drivers can choose to accept the order or refuse it. Furthermore, some of these platforms only make the assignment and leave the delivery sequence up to drivers, while others generate efficient delivery routes and ask drivers to follow these routes. All of these supply–demand matching strategies, complemented with different pricing mechanisms, create digital marketplaces with different characteristics and externalities.
It is commonly believed that crowdsourced drivers provide greater flexibility for delivery companies compared to a dedicated fleet of vehicles, because the driver pool can be adjusted to meet service demand dynamically and with lower costs. However, since crowdsourced drivers are currently not full-time employees, they usually are less stable and predictable, which introduces a high level of uncertainty in workforce and capacity planning. Additionally, crowdsourced driver performance is generally observed to be worse compared to delivery employees of the platform, as the latter have more experience with the business and its customers.Footnote 1 ReputationFootnote 2 mechanisms and other incentives are designed to encourage crowdsourced drivers to display more stable behavior and deliver services with an eye towards higher customer satisfaction.
From an optimization perspective, the crowdsourced delivery problem builds upon the rich body of VRP literature; see [Reference Berbeglia, Cordeau, Gribkovskaia and Laporte44] for an overview. In addition, recent studies have focused on the optimization of crowdsourced delivery systems, the challenge of finding the right balance with respect to the above-mentioned criteria, and the design of appropriate incentives [Reference Arslan, Agatz, Kroon and Zuidwijk45, Reference Archetti, Savelsbergh and Grazia Speranza46–Reference Torres, Gendreau and Rei48]. However, many questions related to market design and comprehensive optimization of crowdsourced delivery platforms, including externalities, still remain open.
12.2.2 Shared Urban Distribution Centers
Urban distribution centers are facilities that are designed and built close to city centers to efficiently handle warehousing operations, such as loading and unloading trucks and sorting packages, as well as cross-docking operations. Their objective is to reduce last mile delivery mileage and fuel consumption and benefit from economies of scale by pooling deliveries into larger vehicles at the urban distribution centers. While it is generally believed that delivery operations using urban distribution centers benefit both delivery companies by reducing their costs and the general public by reducing pollution and traffic congestion, comprehensive analyses of these systems sometimes point to a net negative impact depending on the characteristics of the demand and the urban area.Footnote 3
In operational models that use a shared urban distribution center, larger trucks bring packages from different companies’ warehouses to an urban distribution center. After sorting the packages according to their destinations, packages are loaded to usually smaller vehicles and delivered to customers. At this stage, as an additional benefit of shared distribution centers, orders from multiple companies can be delivered by the same vehicle. Urban distribution centers have multiple docks to load and unload the delivery trucks which reduces the truck queue as the operations can be completed in parallel. Also, these docks complete loading and unloading operations faster than most brick-and-mortar stores, as they are designed for this purpose.
Furthermore, sharing might occur at different levels among companies in the urban distribution centers. They can share only the floor of the building which would increase the space utilization and could reduce the energy consumption, as heating, air conditioning, and lighting could be achieved more efficiently. The participating companies can also share some or all of the operations mentioned earlier. This would further increase the benefits, as the idleness of the shared machinery and equipment would be reduced. Additionally, a collaboration could be formed among the delivery companies, so that the deliveries of different companies could be pooled together, and better routes could be formed in terms of cost efficiency, traffic congestion, and emission production.
On the other hand, convincing e-commerce and/or delivery companies to participate in an urban distribution center is a challenge. The competition among these companies may prevent them from joining a collaborative system. They must be assured that benefits will be greater than costs. Several related problems regarding stable sharing of distribution centers, such as costing, space allocation, and delivery scheduling are discussed in [Reference Quak, Tavasszy, Nunen, Huijbregts and Rietveld49–Reference Cattaruzza, Absi, Feillet and González-Feliu51].
12.2.3 Pickup Lockers
Pickup lockers are secure delivery locations similar to post office (PO) boxes. Generally, however PO boxes are rented for a long time period, whereas lockers are assigned to deliveries for a short time, mostly up to a few days. For this reason, lockers are typically shared by customers. Companies, such as GoLocker, Neopost, and Amazon Locker, currently utilize pickup or product return lockers in their last mile delivery operations.
To use locker delivery service, a customer would choose a pickup location as the delivery address. The delivery company ships the package to this pickup location. When the package is placed into a locker, the customer receives a one-time passcode that is used to open the locker which contains the order. After the customer picks up the packages, the locker is assigned to other deliveries.
Pickup lockers provide benefits for both delivery companies and customers. For customers, a locker station is a secure place to receive their packages in case they do not have a safe area, such as a lobby, in their building. Also, customers do not have to wait at home for delivery since they can pick up their packages from the locker anytime they want. For delivery companies, the locker delivery may reduce costs, since multiple deliveries are expected to be consolidated if their destination is the same locker location. Additionally, drivers avoid delivery problems, such as a wrong address, since the lockers are at known locations.
Pickup locker stations must be designed so that they are an attractive option for consumers. For example, the station must be user friendly and close to areas that customers visit frequently. Additionally, incentivizing customers, for example by providing discounts to encourage consumers to use the service, may increase both the cost and environmental benefits. Designing incentive schemes that consider several factors, such as demand and geographical area characteristics, for each customer dynamically may amplify these benefits. Hence system-wide analysis of all benefits and costs for these shared locker model must be made carefully to inform its design and operations. Comparatively, this area in last mile delivery systems has been studied less completely (see, for example, [Reference Punakivi and Tanskanen52–Reference Ulmer and Streng54]).
12.2.4 Autonomous Vehicles
Autonomous vehicles (AVs) including self-driving cars, automated guided vehicles (AGVs), and unmanned aerial vehicles (drones) may release a big potential in last mile delivery operations. There are several AV brands that are working to produce autonomous vehicles that can follow a given route without human input along the way or be guided by an operator in a semi-automated manner. Currently no AVs are fully autonomous, because such vehicles still require human supervision to prevent accidents when unexpected events occur.
Development and commercial availability of fully autonomous vehicles in the future will open up the possibility for owners of AVs both to make personal use of them and share them with delivery companies. A future scenario could include people going to work with their AVs and then, while they are at work, allowing those AVs to be used by last mile delivery platforms. In a more advanced scenario, AVs could communicate and choose their paths so that traffic congestion could be reduced. Such future sharing of AVs can significantly reduce the idle hours of vehicles sitting in parking lots, while also benefiting the public and environment, as fewer vehicles would be required to achieve both urban mobility and freight transport.
Similar to AV sharing, and in the much nearer future, drones could be shared between their owners and delivery companies. When owners are not using their drones, they can lend them to make deliveries. Currently, one of the limitations of personal drones is their small size, which constrains delivery capacity and allows only small parcels to delivered. Also, personal drones currently have batteries that are much smaller compared to commercial drones, thus reducing their delivery radius. This poses a significant problem especially in cities with tall buildings, where drones cannot fly in a straight line.
Autonomous vehicles can transport packages between locations, but a system to load and unload packages to and from AVs is also required in a last mile logistics operation. An automated system to handle loading to different brands of vehicles, as might be the case in a shared AV system, might be hard to develop in the near future. Additionally, customers might need to be present for delivery, as there will be no one to pick up a package from the AV and deliver it to them. A customer picking up a wrong package can cause significant problems. Therefore, the safest approach currently could be distributing packages one at a time on an AV, which would increase inefficiency and potentially the resulting energy use and pollution of the operations. Incorporating both system optimality and energy usage in operational models may help mitigate the side effects of using autonomous vehicles (see [Reference Levin55].
12.2.5 Public Transportation for Package Delivery
In most cities public transportation network capacities are designed to handle a significant portion of the rush hour commuter needs. However, this causes the public transportation network to have a high idle capacity during long stretches of the day. This idle capacity during low volume hours could be used for last mile delivery, which in turn would reduce the number of vehicles in traffic, especially in the most congested parts of the cities. For example, instead of sending additional vehicles to downtown areas to pick up or deliver packages, last mile delivery operations could utilize the existing public transportation networks in similar ways as described in several studies [Reference Trentini and Mahléné56–Reference Mourad, Puchinger and Van Woensel59]. Synergistic usage of public transport for package delivery could also produce extra revenue for the public transportation authority of an urban area, which in turn can be invested in infrastructure, benefitting the general population.
Public transportation vehicles, such as buses, trams and subways, are designed to carry people and not packages. To use them to deliver packages, operational strategies must be developed and required tools must be deployed at the stops for loading and unloading operations. This would require a high initial installation cost. Furthermore, loading and unloading operations would increase time spent at stops, thereby extending travel times. A small tail car designated for carrying packages that can be automatically and quickly removed and replaced at specific stations is a possibility for carrying packages in and out of highly congested areas using public transportation. Additionally, not all customers might be willing to come to pick up their orders. Therefore, a system to distribute packages from the stops to customers’ houses could complement such a system. An alternative approach, that may be ideal for certain types of customers and packages, could be to combine public transport of packages with delivery lockers at metro stations. This way customers could pick up their packages at their local stations, perhaps at the end of the day on their way home.
12.3 Sharing Economy Platforms vs. Conventional Business Models
Sharing platforms and conventional last mile delivery businesses differ in several ways, including workforce, competition, structure of their corresponding markets, and the externalities that may arise as a result of their operations. In this section we discuss these differences.
12.3.1 Workforce
Conventional delivery companies have dedicated and professional drivers to deliver packages. Since these drivers are traditionally also full-time employees, they have certain benefits which may include health insurance, paid sick leave, and retirement plans. In crowdsourced delivery businesses, generally drivers do not have such benefits, as they are categorized as independent contractors and not as employees, which continues to be a subject of many heated debates and regulatory initiatives from a labor perspective [Reference Conger and Scheiber60].Footnote 4
From an operational perspective, although professional drivers have responsibilities regarding the use of the instruments and vehicles given to them, their employers take care of the cost and planning of insurance and maintenance of these instruments and vehicles. However, crowdsourced drivers have to manage these issues because they use their own equipment and vehicles.
Furthermore, traditional delivery companies have well-established procedures for making deliveries. The decisions on package sorting, loading, and delivery routing are mostly made by specialized professionals or software packages following established procedures. In these traditional business models, drivers have little freedom or flexibility on how to perform these operational processes. Their work shifts may also not be as flexible. In contrast, crowdsourced drivers working as independent contractors are thought to have more freedom of choice on these operational issues. Depending on the platform, they can decide when to work, which deliveries to accept, and how to route their journey. While there appears to be more freedom for an independent contractor, in many situations these drivers’ actions are also restricted through rules or incentive mechanisms. For example, drivers might be held responsible for late or missed deliveries if they occur because of drivers’ actions. In some cases, the flexibilities given to crowdsourced drivers are increasing as platform companies are reacting to recent regulatory efforts and trying to fortify the often-blurred boundary between the definition of a worker and an independent contractor [Reference Paul61].
In traditional delivery companies, the fluctuation in the workforce on a given day is very limited, as drivers must inform their employers in advance if they cannot work on a day and this does not occur frequently. As a result, the delivery capacity on a given day is known in advance, which allows for better operational planning of deliveries. However, crowdsourced drivers may choose not to work and do not even have to inform the company in advance. These conditions create a high degree of uncertainty, which leads to higher operational costs.
12.3.2 Competition
Traditional businesses mostly compete with others in the same business sector. For example, parcel delivery companies compete with other parcel delivery businesses and not with taxi companies. However, crowdsourced last mile delivery companies must compete with crowdsourced urban mobility companies as well as other crowdsourced delivery companies, because drivers can choose to work for any of these businesses as independent contractors. Drivers can check multiple platforms at the same time while sitting in their cars and pick the offer with the best payment and/or work conditions from all the platforms they have joined. Drivers can simply switch from a parcel delivery platform to a ride-sharing platform as soon as they complete an order.
Although payment is an important factor for drivers in choosing among platforms, there are other factors to be considered. A driver might be indifferent to small changes in earnings depending on other features of the platforms. For example, if a driver prefers to socialize while driving, it is more likely that they will choose a mobility platform even though a delivery platform might be offering higher earnings. Level of freedom of choice (orders being assigned by the platform vs ability to choose orders), pressure from the platform (receiving frequent calls/texts to induce fast delivery), and incentives offered by the platform are among possible factors that might impact drivers’ decisions.
The factors that impact drivers’ choice of platform and their performance must be explored. These factors are important as they impact the loyalty of a driver to a platform and their dedication to providing quality service to the customers of the platform. Additionally, incentive schemes for the drivers can be developed. The incentives can be customized for each driver according to their individual preferences. Individualized incentives can improve drivers’ job performance and happiness.
Most platforms do not offer employment benefits to crowdsourced drivers. However, recently Deliv started to offer full time benefits, such as retirement plans and health coverage, to its drivers in California [Reference Said62]. While this decision by Deliv was taken partly in anticipation of CA AB5 regulation [Reference Conger and Scheiber60], Deliv also believed that converting to a full-time employee model might improve service quality and reduce operational costs as well as attract and keep high-performing drivers since offering these benefits provides a bargaining leverage for drivers against other platforms. In a similar recent move, Uber announced that it will consider paying healthcare benefits to workers proportionate to the number of hours they work [Reference Sonnemaker63].
Alternatively, multiple platforms might collaborate instead of competing with each other. For example, a mobility and a delivery platform can collaborate to share their driver pool, and the platform with low demand can transfer some of its drivers to the other one with high demand. However, formation of such collaborations must be regulated and watched carefully in order to prevent the emergence of monopolies or trusts and to ensure drivers are not harmed as a result.
12.3.3 Market Structure
In order to survive in the harshly competitive environment, platforms need to have a certain level of demand and supply in their systems and a continuous dynamic balance between the two must be kept. Dynamic matching of supply and demand in sharing platforms has been a very active research area in recent years [Reference Arslan, Agatz, Kroon and Zuidwijk45, Reference Agatz, Alan, Savelsbergh and Wang64–Reference Masoud and Jayakrishnan66]. For crowdsourced delivery platforms, if there are not enough drivers at any given time, the orders in the systems will end up not being delivered on time or get canceled, increasing the risk of customers switching to another platform. On the other hand, if there are not enough orders in the system, drivers will not have enough work to do resulting in low earnings that incentivize them to switch to another platform.
Combined with the dynamic competition for labor, having to match supply to demand dynamically leads platforms to develop complex pricing policies. Reducing service prices is an effective method for competing for demand. However, this contributes to the major problem of unprofitability experienced by most platform-based businesses [Reference Morozov67, Reference Conger and Griffith68]. Moreover, if the platform lowers prices too much, its drivers might switch to other platforms. This might in turn result in unsatisfied customers who might also leave. On the other hand, surge pricing, in which prices are increased at times of high demand and low supply, could instigate gaming behavior by the drivers (such as turning their apps off to generate “low supply”). Setting the prices to achieve an equilibrium for both supply and demand is a very challenging problem. While the gold standard in two-sided markets is to develop a dynamic pricing policy that balances supply and demand and drives desired customer and driver behaviors, the adaptive behavior of stakeholders and the continuously changing environment make this a moving target. Additionally, in principle, establishing good and clear communication with both sides of the market about the nature of the pricing policies might provide further benefits, as behaviors of customers and drivers can be shifted to a desired setting.
12.3.4 Social and Economic Externalities
Shared delivery platforms have the potential to reduce negative externalities, such as pollution and congestion. They can reduce the number of vehicles operating or the distance travelled. For example, the use of urban distribution centers can reduce distance travelled by increasing truck capacity utilization and integrating public transportation. Similarly, freight transportation networks can reduce the number of vehicles in traffic, especially in congested areas of the city.
However, simple implementations that do not consider all impacts and that lack necessary adjustments to the local needs of urban areas have led shared delivery systems to produce negative externalities in recent years [Reference Ranieri, Digiesi, Silvestri and Roccotelli69]. For example, as discussed previously, crowdsourced delivery cars could be expected to reduce pollution and congestion because they produce less emissions than delivery trucks and delivery trucks are required to return to company parking lots, thereby increasing the distances driven. However, cars are smaller and cannot deliver as many items as trucks. Therefore, more cars are required to complete all deliveries than trucks, and this in turn may produce just as much or more congestion and pollution.
Innovative solutions and strategies are required to reduce the potential negative externalities of shared delivery systems (see [Reference Ranieri, Digiesi, Silvestri and Roccotelli69, Reference Digiesi, Fanti, Mummolo and Silvestri70] for the literature on such strategies). For instance, self-driving cars (that might also be electric) and drones can be used for deliveries (or mobility) when they are not used by their owners. This might reduce the number of vehicles in a city which can lighten the traffic congestion and pollution. However, some people might buy autonomous cars only to produce revenue for themselves. Such a pattern may increase both congestion and pollution in a city.Footnote 5
12.4 Conclusion
Crowdsourcing and shared delivery platforms are among possible solutions which might help to reduce the externalities. They can reduce traffic congestion and pollution by decreasing miles travelled and the number of vehicles in the traffic. Moreover, they can provide additional income opportunities and improve the sense of community. On the other hand, large-scale sharing platforms are relatively new, growing with the speed of internet and mobile technologies. They have many differences with classical business models because they require a level of collaboration of participants to provide benefits for all involved parties. As a result, many questions arise on how to implement and operate these platforms.
To achieve significant benefits from the shared last mile delivery applications for the society, environment, and participating companies, each business model must be analyzed as a system, considering all of its unique set of challenges and impacts. The analyses should take all stakeholders, such as employees, city administrations, and residents, and the interactions among them into account. Additionally, multiple business models should be assessed together. For example, public transportation can be used to bring packages to urban distribution centers from a company’s warehouse, and after the sorting operations, they can be delivered to customers by crowdsourced drivers. Although creating and maintaining such a large collaboration among multiple companies would be very complex, this kind of synergy can amplify the overall benefits in the last mile delivery ecosystem.
One of our goals in this volume has been to demonstrate that interdisciplinary, convergent research and analysis are indispensable to the ideal of optimizing for a more equitable, democratic, sustainable, and just sharing economy of the future. The contributions to this volume are thus central to our demonstration because they epitomize this ideal by drawing scholars from different disciplines into conversations about the most fundamental questions and challenges related to reengineering the sharing economy. But these contributions have also provided important information about some of the answers to key questions that must be addressed as we move forward. In this concluding chapter, we draw from the rich analyses undertaken by our contributors to outline important substantive lessons that can contribute to a framework for reengineering the sharing economy.
In particular, we focus on five core dimensions that are central to optimizing for a just sharing economy: understanding socioeconomic externalities; pursuing resilience; charting more just and systems-oriented business directions; defining the future of work; and prioritizing access and equity. Our effort in this chapter is more modest than to provide detailed conclusions about the relevance of any of these dimensions. Rather, it is to highlight the multiple ways in which the analyses throughout this volume intersect with these dimensions. As we describe, we believe that the centrality of each of these dimensions is itself an important lesson about the future of the sharing economy. Additionally, these dimensions convey significant information about the values that must be prioritized in the next generation of sharing economy platforms. Finally, and crucially, they help to highlight key questions that remain for future research and exploration.
13.1 Socioeconomic Externalities
Digital platforms are becoming more integrated into our daily lives, collectively adding tens of millions of new users every year. As multiple chapters in this volume have discussed, however, the effects of these platforms go far beyond their users. Most platforms indirectly impact the socioeconomic wellbeing of people in many ways. These indirect effects are often referred to as externalities, and given their broad scale and scope, it has been a major research effort to understand, measure, and regulate them. Such externalities have also fueled public debate since the early days of sharing economy platforms. In important respects, the analyses in this volume push beyond the current frontiers of research about socioeconomic externalities.
For example, the two chapters on urban mobility companies investigate the socioeconomic externalities of sharing platforms in urban contexts, and in doing so, they provide important clues to solving complicated puzzles about the hidden effects of the shared mobility industry. As Behroozi’s chapter shows, despite initial promises that ride-sharing services could reduce urban traffic congestion, this is not always the case. In practice, ridesharing can even increase congestion for a number of reasons, including by substituting for public transportation in some cities. Evidence suggests that some of these concerns can be addressed if the industry moves from car-hailing to ride-pooling. However, moving to ride-pooling often requires an array of incentive mechanisms and technical design considerations that have heretofore been less well-charted, as Koutsopoulos, Ma, and Zahedi discuss in Chapter 9.
Chapter 10, by O’Brien, Heydari, and Ke, is similarly illuminating in discussing lodging, where debates over the consequences of short-term rental platforms on the quality of urban neighborhood life are especially vociferous. As the authors argue, strong penetration of short-term rentals enabled by platforms such as Airbnb can have an array of socioeconomic consequences at neighborhood levels, since such penetration increases the influx of nonlocal people to the neighborhoods and results in different – positive and negative – social and economic consequences. Such consequences could mean higher local rents due to decreased real estate supply or higher quality of local services caused by increased local competition. At the same time these platforms can have longer-term effects, because a high level of short-term rental penetration can poke holes in the social fabric of a neighborhood and disrupt its social organization over time.
The analyses in these and other chapters contribute to two broader insights about socioeconomic externalities in the sharing economy. While discussion of externalities has always been integrated into the literature on sharing economy platforms and multisided markets, most of the focus has been on network externalities and on some economic externalities such as the effect of these platforms on employment and traditional businesses. One of the insights gained from the analyses in this book is that we must define externalities more broadly and integrate this broader definition into designing the technical and regulatory elements of these systems. Indeed, this is part of the agenda for reengineering the sharing economy. As Heydari argues in Chapter 2, the broader definition of externalities expands the range of stakeholders who are affected by sharing platforms to include local residents and businesses, potential second- and third-tier businesses that could emerge in the ecosystem created by a sharing platform, and even other sharing platforms, given the possibility of interplatform interaction across different platforms that provide similar or complementary services.
In addition, much of the debate about socioeconomic externalities has been shaped by anecdotes and opinions that are often rooted in too much optimism or pessimism towards sharing platforms. While there are cases where a particular positive or negative externality of a platform outweighs the rest of the consequences, the reality about most types of socioeconomic externalities is more complex than what these anecdote-based debates suggest. This book highlights that assessing the overall impact of sharing platforms on a given socioeconomic factor (such as traffic congestion, the environment and carbon emissions, and neighborhood economic and criminal activities) depends on understanding the tradeoffs among competing factors through which platforms can either benefit or harm that factor. The relative weight of these competing factors depends on certain design and regulatory parameters on the one hand and the time horizon of the analysis (short-term versus long-term) on the other. Further, evaluating trade-offs requires us to learn the causal mechanisms by which platform parameters are associated with socioeconomic externalities.
As Heydari’s chapter discusses, such a methodology requires steps such as quantifying the effects of these platforms in the short term and long term, determining different stakeholders and soliciting direct or indirect inputs from them, and establishing methods to aggregate inputs from different stakeholders. Moreover, given the importance of identifying different causal mechanisms, the methodology can benefit from combining empirical studies with analytical modeling. Ultimately, outputs of these models can contribute to designing externally imposed regulations, as described by Dyal-Chand in Chapter 7, as well as internal governance mechanisms designed by platform companies.
The chapters in this volume inspire future research in socioeconomic externalities on three vital areas. An initial step in identifying the impacts and mechanisms of socioeconomic externalities will be to improve sociotechnical modeling methodologies, which will allow empirical identification to be integrated with system-level simulation. Second, even when we can model and quantify various types of externalities, design and policy decisions are influenced by how we weigh and rank them. Considering platforms’ algorithmic nature, this can be challenging, especially since rankings and weights must be updated dynamically. Last but not least, these models need to identify lever points that platform designers and regulators can utilize in order to govern socioeconomic externalities.
13.2 Resilience
As several chapters have observed, some digital platforms serve the function of modern critical infrastructure in many parts of the developed world. This fact, laid bare by the COVID-19 pandemic, is a startling indication of the extent to which the sharing economy has transformed modern living for many of us. It is a fact that requires us to comprehensively reevaluate the forms, functions, and values that inhere in the sharing economy today. This reality also means that it is necessary to examine the resilience of sharing platforms, just as we do with other critical infrastructures. The resilience considerations of sharing platforms require us to ask two overarching questions. As is standard practice in considering the resilience of traditional infrastructures, we must examine how resilient sharing platforms are in response to unexpected disruptions. In addition and moving beyond standard practice for other forms of critical infrastructure, we must consider how these platforms can affect the resilience of other socioeconomic activities.
The COVID-19 pandemic served as a giant stress test for the resilience of many industries, social and economic institutions, and sociotechnical systems. Digital platforms can be credited for contributing positively to the resilience of pandemic life in much of the developed world by facilitating quick transitions to working at home, online shopping, and virtual socializing. Such quick transitions were enabled by a number of factors, such as preestablished logistics infrastructures for companies such as Amazon and Wayfair. Another enabler was the quick repurposing of platform capacities. For example, Uber quickly moved resources from Uber Ride Sharing, for which demand was plummeting, to Uber Eat for which demand was skyrocketing.
As the pandemic revealed, several inherent characteristics of sharing economy platforms make it possible for such platforms to respond quickly to sharp changes in demand level, thus contributing to the overall resilience of the broader ecosystem for the type of services they provide. Consider the mobility industry as an example. First, because mobility platforms do not own the underlying assets (namely cars), they are nimbler in changing the supply level by updating the participation rate on the supply side of the platform. These changes are possible within a feasible range, determined in the short term by the existing pool of agents on the supply side (namely, active drivers), but can grow or shrink in the longer term depending on the overall conditions of the platform ecosystem. Second and as a mechanism to reap the benefits of the first factor, sharing platforms can use dynamic incentives, often in the form of dynamic pricing, to close possible gaps that emerge between the supply and demand levels. Finally, the digital and on-demand nature of many of these platforms means that these platforms can quickly estimate sudden changes on their different sides and buy more time to react to those changes. In Chapter 12 Duman, Ergun, and Behroozi discuss some of these factors in the context of the last mile delivery problem, which is considered a major logistical bottleneck in implementing resilient and sustainable e-commerce systems.
Despite the positive contributions of sharing economy platforms to the overall resilience of essential services, several chapters in this volume raise important concerns about the potential negative impacts of these platforms on infrastructure resilience, especially in the future as we become more dependent on them. As the chapters on mobility discuss, mobility platforms can shift some of the demands from public transportation systems to ride-sharing services, resulting in further reductions of available investment budgets in public infrastructures. From a resilience perspective, this is not necessarily concerning as long as the platform-based systems can provide a continuation of widespread affordable service, especially in the aftermath of a major disruption. However, such access is not guaranteed, given the asset-free nature of many sharing platforms on the one hand, and on the other hand their relationship with their workers, as Schor and Vallas discuss in Chapter 6. Both these factors put much of the supply-side management at the mercy of short-term incentives offered by the platforms, which might fail under extreme circumstances.
Relatedly, it is important to understand that, like most resilient systems, the mechanisms that help these platforms to be adaptive to sudden changes can only contribute to resilience up to certain levels. These same mechanisms can become ineffective, and even counterproductive, once the changes in supply and demand go above a certain level. For example, dynamic pricing can result in unacceptable surge prices in the face of a sudden rise in demand. Importantly, too much decrease in the level of supply, in the case of a drop in demand, means that the platform reduces its geospatial coverage and consequently its on-demand nature. For ride-sharing platforms, this means that the average wait time for each passenger will increase because of the low number of drivers, resulting in dissatisfaction on the passenger side. Such dissatisfaction can lead passengers to pursue other options and lower the demand, which in turn lowers the supply, as discussed by Koutsopoulos, Ma, and Zahedi in Chapter 9). This downward spiral, similar to what is often known as the Wild Goose Chase phenomenon, can make platform systems nonresilient. By contrast, public transportation demonstrates a more linear resilience behavior – at least in the short and medium term – in response to demand changes. As Heydari argues in Chapter 2, public–private partnerships between sharing platforms and public infrastructures can address some of these concerns by including resilience considerations in their agreements governing the provision of services to passengers. These resilience-oriented partnerships can go beyond transportation infrastructure and extend to energy systems, as Kane, Allen, Si, and Stephens show in Chapter 11, focused on future energy systems.
Finally, any discussion about resilience and economic externalities is not complete without considering environmental sustainability considerations. As Eckelman and Kalmykova discuss in Chapter 3, despite initial promises about the positive role of sharing platforms on the environment, it is quite challenging to evaluate the actual sustainability orientation of sharing economy platforms. Sharing companies are highly heterogeneous in this regard and can create a wide range of unintended consequences for the environment. The authors describe a number of these unintended consequences and enumerate several back-end and front-end design opportunities for incentivizing beneficial environmental outcomes. However, research about environmental costs and benefits of peer-to-peer sharing platforms has been limited, and more studies are needed to further guide and prioritize such design opportunities.
Resilience of complex sociotechnical systems has been a topic that has attracted increasing interest from several academic communities. Resilience in these system types is the result of a combination of top-down and bottom-up responses at different levels and by various actors, including the synergistic role of policy design on the side of the regulators, behavioral change on the side of human agents, and repurposing of existing capacity and technological adaptation on the side of businesses. Studying resilience in sharing economy platforms can not only prepare us for future disruptions but can also teach us important lessons about multilevel, synergistic responses at the system level that are useful for the broader context of complex sociotechnical systems. We hope the chapters in this book will inspire new thinking about a range of crucial questions regarding system resilience, especially in the wake of the COVID-19 experience. For example, how can we identify and characterize existing capacities in sharing platforms that can be quickly and efficiently repurposed and reallocated in the face of major disruptions? How might we create more synergy between top-down responses (including policy and business decisions) and the behavioral changes that often act as bottom-up adaptation mechanisms in the face of a disruption? How can we create scenario-study models that incorporate these levels of system responses and that can be used both to identify the trade-offs of resilience decisions and to communicate them to the key stakeholders? And finally, how can we better integrate the adaptability aspects of platform-based systems with the objectives of public decisions – such as through public–private partnerships – to better steer the direction of a system’s response towards the public good?
13.3 Future Business Directions
The focus of this volume has been both to provide a systemic perspective on sharing economy platforms and to discuss design and governance issues at the intersection of engineering, regulation, and operations. This is in contrast and complementary to recent books that look at the sharing economy from the perspective of the firm making key business decisions. The recent focus on the firm’s perspective is not surprising, since the trends that gave rise to the modern sharing economy (which are examined in the Introduction to this book) have been associated with substantial new value creation over the last decade. Hundreds of market-based platforms continue to take advantage of growing algorithmic and data capabilities coupled with rapidly advancing technologies to allocate access to goods and expertise in such a way as to keep transaction costs to a minimum while in many cases utilizing available capacities of physical assets as fully as possible. Collectively, these features make sharing economy platforms unique from a business perspective. Although this volume’s focus has been elsewhere, a number of chapters in this volume have raised important business implications that may serve as catalysts for future research in this field.
First, this book makes a case for the possibility of bringing greater shareability to a range of platform services. For example, Chapter 9 observes that on-demand mobility services currently provide very few shared rides, and the authors present models and recommendations designed to improve sharing in these services. Sharing economy models are discussed in Chapter 12 as a way to solve the challenging problem of last-mile deliveries in e-commerce. Similarly, Chapter 11 discusses the possibility of using sharing economy models in energy systems.
Second, several chapters emphasize that establishing and maintaining trust is essential for the operation of sharing economy businesses. Tadelis, in Chapter 5, argues this could be accomplished by designing appropriate feedback mechanisms. Additionally, privacy is becoming a top concern for users of platform-based businesses, and as discussed in Chapter 4, it is important to understand the privacy calculus of platform users in order to identify potential trade-offs associated with privacy protection measures and other business metrics.
Third, several chapters in this volume highlight ongoing concerns about business competition in the sharing economy. For example, looking back at how this sector grew over the past decade, there seems to be a wide gap between the multibillion-dollar valuations of platform companies like Uber and their lackluster profitability. This gap is often attributed to the power of strong network effects, as discussed in Chapter 2, which create entry barriers and effectively lock in users once the platform company succeeds in attracting many of them during the initial growth spurt. The lock-in problem has been typically discussed in the context of competition among online social networks, where it arises due to embedded positive direct network effect (for example, the more of your friends use a social network site, the higher your value of using it). Leading online social networks, such as Facebook and LinkedIn, have pursued this strategy to establish a sustainable competitive advantage. Thus, it is important to gain a better understanding of how various components of switching costs may reduce competition among sharing economy platforms. Reduced competition may result from several factors, including the cross-side network effects, possible multihoming (users joining multiple platforms at the same time), and myopic decisions on the part of users. A great deal more analytical and empirical research about these nuanced factors is needed to better understand concerns about sharing platform competition.
Fourth, on the operational side, the on-demand service delivery promise of sharing economy platforms, together with a less predictable crowd-sourced contractor resource structure for performing the services, can lead firms to maintain excessive capacities. Perhaps surprisingly, such high (and costly) capacities often correspond to low utilization of resources, contrary to common claims made by sharing economy platforms. An example of this can be seen in ride-sharing platforms: They encourage many drivers to join and be active on the platform app but impose on those drivers to spend a significant amount of time waiting for fares. Another example can be seen in the last-mile delivery context, as discussed in Chapter 12. More research is required to understand these unexpected phenomena on the operational side of platforms.
More broadly, over a decade has passed since most sharing economy leaders started their businesses, and major industry changes have followed the COVID-19 pandemic, thereby raising the possibility that it is time to reassess some of the business assumptions that have been widely and, for the most part, silently accepted in the sharing economy industry. For instance, it has been suggested that one of the factors driving sharing economy growth is the shift from ownership to use among millennials. However, we have seen much evidence of the opposite trend during the COVID-19 pandemic. In response to rising demand, the average price of used cars increased by more than 40 percent in less than two years from the start of the pandemic.Footnote 1 Meanwhile, millennials significantly contributed to the real estate market boom in 2020–2021.Footnote 2 It remains to be seen whether these recent trends are temporary and could be fully explained by supply chain disruptions or whether these are harbingers of long-term structural changes that present a serious challenge for sharing economy platforms.
Finally, we emphasized in the Introduction to this book that most business decisions cannot be divorced from platform governance decisions for sharing economy companies. While this is true for all businesses, governance decisions are crucial for the business success of sharing economy companies for many reasons. These reasons include regulatory compliance, safety imperatives, and resilience and environmental concerns. Therefore, we expect more research to be conducted on integrated modeling and analysis of business and governance decisions in different platform types, allowing public policy stakeholders to better assess regulatory environments and possible trade-offs.
13.4 The Future of Work
The workplace has undergone dramatic changes in recent decades as a result of numerous disruptive forces including globalization and automation. As multiple chapters in this volume discuss, sharing economy platforms are the most recent and rapidly accelerating disruptive force on the structure of work, with both intended and unintended consequences. For example, while digital platforms have made remote work possible during the COVID-19 pandemic, gig-workers employed by many sharing economy platforms have precarious working conditions, as described by Schor and Vallas in Chapter 6. These circumstances have fueled a fierce debate on the employment status of platform economy workers and on the future of work in general. Although some sharing economy platforms have existed for over a decade and research on platform-based work has grown rapidlyFootnote 3, Footnote 4, Footnote 5 it remains unclear how platform jobs affect the quality of employment, whether workers are exposed to risk with potentially adverse effects, and how platform workers view their position as independent contractors. The future of work is thus a key dimension that must be prioritized in any serious effort to reengineer the sharing economy.
Almost all sharing economy platforms have two core characteristics. First, they use Internet-based digital technology and algorithms to mediate transactions between buyers and sellers of goods and services. Second, they define themselves not as employers, but simply as providers of information systems that “match” independent contractors with potential customers or clients – an important economic and legal shift that redefines the nature of employment and that externalizes many financial and legal risks. In turn, many of these risks have been imposed on the workers themselves. As Chapter 12 on last-mile delivery and Chapters 8 and 9 on mobility discuss, crowdsourced independent-contractors help platforms to achieve greater operational flexibility to provide on-demand services, agilely matching supply to demand with minimal risk to the firm. While many on the platform side argue that this flexibility provides the necessary competitive advantage to firms and flexible working hours to workers, it also introduces a significant level of uncertainty for all actors involved in the operational environment.
The challenges of the platform economy impose unforeseen costs on platform firms themselves, which often struggle to scale up their business models in sustainable fashion. As the chapters on labor, urban mobility and last-mile delivery discuss, one major challenge that businesses face flows directly from their use of the independent contractor model. Firms cannot simply impose work schedules on workers, since freedom over working hours constitutes an important selling point for the recruitment of workers. As a direct consequence, firms encounter heightened levels of uncertainty about staffing levels, which are often vital to their business success. Moreover, since platform workers must assume responsibility for many operational costs and risks, they exhibit extremely high levels of turnover, which imposes substantial costs on platform firms in the forms of bonuses, marketing campaigns, and promises of minimum levels of earnings. Dynamics such as these mean that gig-workers can be less reliable (for example, by not showing up on time) and less experienced at the task at hand (for example, by not knowing the details of doing a delivery at a customer location), thereby forcing firms to increase their supply buffers in order to ensure a given service level in their operations. These problems can jeopardize firm viability. They reveal that firms have yet to develop sustainable models for the governance and control of the workforce on whose labor they rely.
Workers participating in the sharing platform economy also face distinctive challenges that differ from those of “traditional” paid employees (as described in Chapter 6). Many workers are attracted to platform work by the possibility of more autonomy over work schedules and greater freedom from supervision. However, the terms of their employment may be obscure. For example, transportation workers must “accept” jobs without knowledge of the destinations. From the perspective of the gig-worker, on top of not having the benefits granted to an employee, this type of work arrangement generates significant anxiety from not knowing the actual income that an intended number of work hours will generate. In addition, gig-workers must satisfy the conditions of reputational management systems in order to avoid “de-activation,” even though such conditions often are unknown to them (as described in Chapter 5).
Another clear danger for workers is that the expansion of platform-based work may open up significant gaps in the social safety net, since platforms seldom provide access to health or retirement insurance and platform workers are ineligible for protections under labor standards and minimum wage laws. Collective action is the traditional approach to balancing these information, economic, and social asymmetries, either through formal labor unions or through informal information sharing. Because platform workers typically are contractors, and not employees, however, they are limited in their ability to unionize. In addition, in traditional workplaces, informal worker collectives result from conversations “around the water cooler.” However, in the sharing economy, which lacks a physical workplace, these conversations come at a greater cost and often are relegated to online forums. Thus, one of the main questions for comprehensive reengineering of the sharing economy is the question of how to determine the optimal conditions of work and the regulatory actions and protection that need to be taken to ensure those conditions, as discussed in Chapter 7 on regulation and Chapter 6 on labor and work.
Urban and state governments, too, face unforeseen challenges from the platform revolution. Since platforms represent new forms of business for which decades-old regulations were not designed,Footnote 6 platforms can often operate in an unregulated space, free of the dictates that constrain their more traditional competitors. Typically, city governments lack the most basic information about platform firm operations, even though the latter have major consequences for the transportation, housing, and employment systems on which the public relies.
More generally, the sharing economy has generated important gaps in the flow of information that is vital to the interests of workers, governments, and firms. For example, large-scale proprietary information generated by ride-hailing platforms such as Lyft is valuable. As a result, firms rarely share such information with regulators, who could use it to better understand the effect of the firm on the public. Nor do firms share such information with workers, who could use it to make career and daily employment decisions. Ironically, firms themselves suffer from information gaps, since they typically lack access to information about the long-term well-being of the workers who provide the lion’s share of their service. As some of our contributors have discussed, new research methodologies can help produce, disseminate, analyze, and share information previously unavailable about the sharing economy, which in turn should help improve market efficiencies, reduce labor market uncertainty, and support proactive regulatory structures, thereby strengthening the entire sharing economy ecosystem.
The multiple observations in this volume about the nature of work in the sharing economy teach us a crucial lesson: Comprehensive optimization of work conditions by platform owners, workers, and regulators should be one of the core concerns of reengineering the sharing economy. Currently, platform owners optimize for efficiency, growth, and profit through the design of their matching and pricing algorithms. Government regulators optimize for the public good through regulations. Workers, as suggested by Hall and Krueger (2018)Footnote 7 and Schor et al. (2020),Footnote 8 currently optimize for both income and flexibility. However, it is not clear if each stakeholder optimizing myopically without a systems perspective of the entire ecosystem can possibly achieve the desired outcomes (see Chapter 2).
Looking ahead to the prospect of reengineering the sharing economy, there remain important open questions for all stakeholders related to the future of work. From the perspective of the firm, many sharing economy platform companies struggle to be profitable even after operating for years with significant market shares, raising questions about the sustainability of the business model as it concerns firms’ relationship with their workforce. This relationship impacts several aspects of a firm’s profitability including how it recruits, maintains, and pays its workforce and how the firm’s operational efficiency is affected by issues such as workers’ hours, dependability, and professionalism. In turn, workers are low-paid and lack meaningful control over working conditions and data (see Chapters 5 and 6). Finally, regulatory authorities have thus far had little success at regulating the underlying business activity and service delivery that sharing platforms make possible, in turn limiting their ability to constrain negative effects on the public good, including the future of work (see Chapter 7). While this limited regulatory success is partially due to the strong lobbying efforts of sharing economy firms, such as in the case of the ballot defeat of Proposition 22 in California (discussed in Chapter 6), in other cases it is due to the challenge of anticipating the externalities that will be caused by the regulation itself. In this complex ecosystem, regulatory and other actions targeting part of the system may broadly impact consumer behavior or workforce dynamics in unintended ways, potentially causing more harm than good, as discussed in Chapter 4. This observation emphasizes the point by Duman, Ergun, and Behroozi (Chapter 12) as well as Heydari (Chapter 2) that a comprehensive analysis of the nature of work in sharing ecosystems is crucial.
13.5 Equity and Access
Although this volume is by no means the first to emphasize the significance of equity considerations in the sharing economy, it does resoundingly affirm equity’s centrality. Indeed, equity is a core theme in many of the analyses contributed by our authors, though regularly only implicitly so. These analyses provide rich detail about the range of equity-related harms and benefits that have occurred in sharing economy markets. They also provide significant information and inspiration for creating a more equitable sharing economy. Before reviewing the lessons learned from this volume about equity, it is important to consider how equity is defined – and how it manifests – in the sharing economy.
Plainly, one crucial vein of concern and analysis that invokes equity considerations relates to race and racial relations. Because the focus of this book is the American sharing economy, analysis of racial equity in the sharing economy could not be a more pressing matter. As Dyal-Chand observes in Chapter 7, the COVID-19 pandemic is not the only pandemic that has plagued the United States for many months now. Racial violence has also reemerged as a crisis that demands cross-disciplinary analysis and response. Not surprisingly then, concerns about racial equity surface throughout this volume. Schor and Vallas describe the emergence of a “third, implicitly racialized employment status,” between independent contractor and employee – a status that is both unequal and “substandard” in the level of protections and value that it affords workers who have it. Dyal-Chand discusses the dawning recognition among those who study the sharing economy that at least some proprietors of sharing platforms seem to be developing their businesses in a direction that capitalizes on the racist results produced by their algorithms. More implicitly, both Chapters 10 and 11 raise troubling questions about the racialized effects of sharing innovations intended for (often commendable) purposes such as providing greater access to goods and services within neighborhoods and the democratization of energy production and control.
Issues of equity and equality also arise with respect to gender, disability, and other identity categories. Research on whether sharing economy platforms discriminate on the basis of gender has only scratched the surface, and this is reflected in the contributions to this volume. Yet it is also apparent that many of the questions raised by the research on disparate racial impact also necessitate a robust research agenda concerning other disparate effects. These effects will no doubt be different from the effects of racism within the sharing economy, but the research on race in the sharing economy can provide helpful clues to guide additional research.
This volume also overwhelmingly makes the case that equity within the sharing economy is defined by level of income and wealth. For example, Heydari’s proposal of a sociotechnical examination of the many positive and negative externalities produced by sharing platforms provides an analytical perspective that reveals the hidden burdens and benefits that depend partly on the wealth of sharing economy participants. Focusing their analytical lens on the increasingly ubiquitous mobility industry, Koutsopoulos, Ma, and Zahedi provide nuanced information about the differential impact of ride-sharing innovations in the first generation of mobility platforms. While their attention is on reducing congestion, increasing sustainability, and improving the profitability of mobility companies, the detailed innovations they propose also provide a template for achieving more equitable access to mobility platforms by consumers with lower incomes and less access to traditional goods and services such as privately owned cars and taxis.
The contributions in this volume additionally make clear that equity concerns exist on both the demand and supply sides of sharing platforms. On the demand side, the analyses by Koutsopoulos, Ma, and Zahedi as well as O’Brien, Heydari, and Ke provide deep empirical insights into how access to first-generation sharing platforms can vary for consumers by neighborhood, income, and other demographics. Dyal-Chand describes the proliferating literature on the genderized and racialized consumer harms wrought by first-generation sharing platforms. Kane, Allen, Si, and Stephens raise similar concerns in the new frontier of energy sharing. As the discussion by Lambillotte and Bart suggests, such fundamental concerns as privacy may intersect in significant ways with the axis of equity.
On the supply side, Schor and Vallas raise deeply troubling questions about the future of equitable work, especially for the sharing economy workers who rely on platform jobs as their primary source of income. Such concerns are amplified when considered in contexts such as last mile delivery (see Chapter 12) and the development of clean energy systems (see Chapter 11). These chapters provide the detailed examples for the conclusion reached by Heydari and Dyal-Chand in their chapters that sharing platforms have been able to develop in a regulatory environment that does not constrain platform proprietors in their treatment of those who provide goods and services through those platforms.
The analyses in this book thus present a vexing puzzle: On the one hand, sharing economy platforms maximize opportunities for maintaining anonymity and for sharing the value of expensive goods and services. Through technology, such platforms reduce the costs of market entry and exit by making it easy and cheap to provide – and also to access – goods and services. They significantly increase access to information at a very low cost. In short, the sharing economy should be a means of equalizing access to an enormous range of markets. Yet, on the other hand, many of these very platforms have innovated in ways that allow proprietors and suppliers to differentiate – and outright discriminate – on the basis of race, gender, disability, income, and other characteristics. In so doing, these platforms have limited access to sharing economy participation on the basis of criteria that should have been rendered invisible and irrelevant by platform technology. They have regularly contributed to inequity rather than increasing equity.
While this volume has contributed to the conversation about equity by providing important empirical and interdisciplinary evidence of this puzzling phenomenon, it has also contributed to a basic diagnosis. As the scholars in this volume have described from a range of disciplinary perspectives, the proprietors of sharing economy platforms innovate in directions that optimize for their priorities. The first generation of sharing economy platforms have overwhelmingly optimized for fast growth, and more broadly, profit. In the course of doing so, they have produced a range of positive and negative externalities, some of them startling. These externalities teach us important lessons about the multiple impacts of the sharing economy – and its potential for achieving equity among other things. Yet, realizing this potential requires more deliberate and concerted action. In short, the current state of the sharing economy demands a rebalancing in the direction of greater equity. Whether by choice, by mandate, or by some combination of the two, such a rebalancing can only occur if platform proprietors optimize for equity in addition to growth and profit.
Moreover, the diagnosis that emerges from this volume makes clear that the problem of inequity in the sharing economy is deeply systemic in nature. Currently, market design, industry practices, and law all provide ample space for sharing platform proprietors to make their own choices about goals, priorities, and innovations, including those that increase inequity. For example, the design of sharing platforms provides ample opportunities to innovate new forms of business transactions that capitalize on reputation and trust. As Tadelis describes, such innovations are exciting and disruptive, allowing a broad range of participants in the sharing economy to rely on new forms of information and new business methods. Transparent rating systems allow suppliers of services on sharing platforms to develop good will rapidly and efficaciously, as compared to traditional businesses. Yet, as other contributors point out, these very forms of market design also can reduce equity by eliminating anonymity and thereby reinstating the ability to discriminate on the basis of race, geography, wealth, and other criteria. Industry practices can exacerbate such effects. By leveraging just such design mechanisms, sharing platforms can use surge pricing and other methods to take advantage of unequal access by consumers. As Schor and Vallas discuss, they can also increase the precarity of low-wage workers who depend on sharing platforms for meaningful income.
Currently also, as the chapters by Heydari, Dyal-Chand, and others discuss, law creates ample space for innovation in market design and industry behavior without systemic analysis of the connection between such behavior and equity considerations. Powerful intellectual property rights, contracts of adhesion, weak labor and employment laws, piecemeal and reactive regulations, and lack of political will or even direction in protecting widespread access to sharing platforms at times combine to nurture and even valorize disruption at the expense of necessary protections.
Crucially, the contributions in this volume have supplemented these diagnostic insights by enhancing our understanding of a range of possible solutions to the problem of rising inequity in the sharing economy. One of the most important messages from the volume as a whole is that, because of the multiple sources for inequitable development and operation within the sharing economy, the solutions must also be cross-disciplinary. To examine the potential of cross-disciplinary solutions to address inequity in the sharing economy, consider one set of solutions that has come to the fore in this volume, and indeed that invokes the title of this volume. Specifically, consider the potential that some of the necessary regulations of the sharing economy may be best imposed by means of the engineering of the platforms. In other words, the concept of “regulation by design,” which has been the subject of much scholarship in the privacy domain, may also be a valuable form of regulation for the purpose of prioritizing equity.Footnote 9
As the analyses in this volume suggest, the incorporation of regulation into the design of sharing platforms would require at least two indispensable ingredients. First, a certain level of what has been described as “self-regulation” would be required. Self-regulation might originate in the design choices made by businesses within a sector that choose to maximize value for different stakeholders, beyond profit, growth, or efficiency. For example, recognizing its role as a necessary component of the transportation infrastructure (especially during crises or other periods when public transportation is disrupted), a ride-sharing company could choose a more socially responsible pricing structure that would reduce or at least stabilize prices during times of crises, instead compensating for this lost opportunity by charging higher prices in business districts or other geographic regions where riders could expect to be subsidized by their employers or would have incomes high enough to support paying higher prices. Just as some platforms have already marketed their products and services on the basis of their greater contributions to environmental sustainability (see Chapter 3), or consumer safety,Footnote 10 such a business could distinguish itself in the market on such grounds.
In addition, government would have an important role in developing and maintaining this kind of self-regulation. In the ride-sharing pricing example just provided, it is possible that the hypothetical company could achieve market success by means of this combination of social responsibility and pricing differentiation, and it is even possible that it could begin a “race to the top,” rousing other companies to explore the benefits (and costs) of fulfilling their function as a necessary part of an urban transportation infrastructure. However, given the many apparent incentives toward monopolization, it is likely that such moves would need to be encouraged by governmental involvement. Fortunately, there already exist numerous regulatory models from which regulators could draw. One interesting model is the development of “certificates of trust,” which could originate either within an industry or with a governmental agency.Footnote 11 Another example could be for regulators to lead the development of an industry-wide code of conduct for platform design. Another would be to provide design guidelines such as those issued by regulatory agencies to ensure compliance with the Americans with Disability Act and similar federal and state laws.Footnote 12 Certainly, also, it may be appropriate for regulators to at times require the incorporation of certain design standards that would optimize for one or more of these principles.
As Chapter 2 makes clear, this kind of coordination between platform design and regulatory design would require a sociotechnical approach that could account for a broad range of positive and negative externalities, design characteristics, and individual and group behaviors on both the supply and demand sides of sharing platforms. The analyses in the chapters on sharing in the neighborhood, sharing and sustainability, sharing and last-mile delivery, and sharing energy all provide vivid examples of the need for system-wide analysis in engineering self-regulatory approaches.
This, however, would be just the beginning. While such an approach to reengineering a more equitable sharing economy holds much promise, crucial questions will arise and will require interdisciplinary research and analysis. Three sets of questions seem particularly salient on the equity front. None can be answered on the basis of the research presented in this volume, though much of this research certainly lays the foundation for an ambitious forward-looking research agenda.
First, how could platforms be inspired to choose to self-regulate in the direction of greater equity? Given the extraordinary impetus to optimize for immediate fast growth – and the reality that many platforms have yet to achieve any meaningful profit while taking in vast amounts of venture and other private capital – how could platforms be motivated to race to the top in designing equitable industry practices? This question seems particularly salient in light of the extraordinary level of social and political polarization in US society (and many other societies) today. Indeed, it is reasonable at least to wonder whether the type of “regulatory entrepreneurship” described by Pollman et al. is possible partly because of such polarization.Footnote 13 If so, then the challenge of solving the contemporary polarization in wealth depends partly on solving these other forms of polarization as well – a daunting task.
Second, while the benefits of governmental regulation to promote reengineering of a more equitable sharing economy may be apparent, it is also important to consider the costs of such regulatory interventions. One such cost could be social and political backlash, thereby leading to even greater polarization. Such a counterproductive result would be to no one’s advantage. A second cost could be the potentially high level of investment required to achieve a regulatory approach that is responsive, thoughtful, and sophisticated enough to nurture industry-led design that could successfully achieve equity over the long-term. Unfortunately, our regulatory history has produced too many examples of analogous regulatory failures, despite the good intentions behind them. A third obvious cost is that regulation could delay and stunt positive industry innovations as well as profits, thereby harming the very individuals and groups, such as low-wage workers and consumers of color, that the regulations would be intended to help. These are serious concerns, and they demand careful attention going forward.
Finally, it will be important for future research and analyses of equity – and the possibility of engineering for equity – to consider the implications for sharing economy governance more broadly. All of the complicating factors just described and many others, including political and other forms of polarization and the globalized nature of the sharing economy, also complicate the prospects of stable governance. The multiple and varied examples of sharing economy platforms provide fertile ground for further research about governance. For example, as the chapters on sharing delivery systems, energy, and mobility discuss, genuinely peer-to-peer platforms have addressed a range of access and even equity issues. Heydari and Dyal-Chand both raise questions about whether these and other examples could point us toward deeper examinations of the emerging democratic principles in some sharing economy contexts.Footnote 14 Here again, the contributors to this volume have raised significant questions that deserve further research attention.
13.6 Reengineering Sharing: What Lies Ahead?
In addition to these core dimensions that define the challenge of reengineering a more just sharing economy, the contributions in this volume have raised a set of questions that might best be described as more philosophical, epistemic, or even existential in nature. These questions present some of the most difficult and complex challenges of all. Yet we believe that it is only wise for those involved actively in reengineering the sharing economy to reflect on these questions, and in so doing, to make their best efforts to proactively address them. While we make no pretense to answer these questions, we conclude this chapter by raising two salient areas of necessary exploration.
13.6.1 How Have Platforms Contributed to Globalization?
The relationship between platforms and globalization is deep and diffuse. Indeed, at this stage, we can only raise more specific questions about this relationship in an effort to define its contours. On the labor side, for example, how has platform work affected patterns of migration and immigration? In what ways has platform access replicated patterns of discrimination, colonialist behavior, and nationalisms, and in what ways has it disrupted those patterns?
Some of these questions are relevant on the consumer side as well. Additional questions also arise: How have consumers benefited from accessing platforms across borders? On the other hand, in what ways have they assumed greater risk?
Finally, a number of crucial questions arise for businesses and those who govern them. For example, how have platform-based businesses responded to taxation, and more generally to other laws that depend in meaningful measure on physical location within a territory? These are just some of the many, many questions inspired by the connection between platforms and globalization.
13.6.2 Who has the Right to Govern the Sharing Economy?
Finally, and relatedly, one of the most vexing set of questions moving forward will no doubt concern platform governance. The diffuse, indeed globalized, nature of platforms deeply impacts the question of governance for the obvious reason that it raises foundational questions about who has the right to govern the platform economy, or any given piece of it. While this question at times feels rhetorical, especially in light of claims that the Internet is too diffuse a phenomenon to be governable, it remains imperative to search for a more substantive answer in response to such claims.
On this question, the editors of this volume have a clear normative position: As we, and many of our contributors, have expressed, we believe it is imperative to develop processes, structures, and norms that move the sharing economy in the direction of genuinely democratic governance. While such a statement is rhetorically powerful, it is also rhetorically straightforward. It will of course be much more difficult to operationalize this statement. Doing so will require serious attention to the current power imbalance between platform owners on the one hand and consumers, workers, and even regulators on the other. It will require reconsideration of intellectual property rights and other legal and market structures that perpetuate this power imbalance. Moreover, just as is the case with any political democracy, it will require vigilance and nurturing over the long term.
Yet we believe that it will be imperative to engage in just such an effort as we seek to optimize for a more just sharing economy. We hope that the analyses in this volume have provided both information and inspiration for future work in this direction.