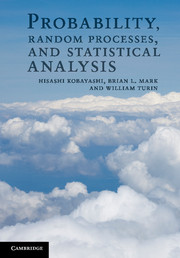
Book contents
- Frontmatter
- Contents
- List of abbreviations and acronyms
- Preface
- Acknowledgments
- 1 Introduction
- Part I Probability, random variables, and statistics
- Part II Transform methods, bounds, and limits
- Part III Random processes
- 12 Random processes
- 13 Spectral representation of random processes and time series
- 14 Poisson process, birth–death process, and renewal process
- 15 Discrete-time Markov chains
- 16 Semi-Markov processes and continuous-time Markov chains
- 17 Random walk, Brownian motion, diffusion, and Itô processes
- Part IV Statistical inference
- Part V Applications and advanced topics
- References
- Index
12 - Random processes
from Part III - Random processes
Published online by Cambridge University Press: 05 June 2012
- Frontmatter
- Contents
- List of abbreviations and acronyms
- Preface
- Acknowledgments
- 1 Introduction
- Part I Probability, random variables, and statistics
- Part II Transform methods, bounds, and limits
- Part III Random processes
- 12 Random processes
- 13 Spectral representation of random processes and time series
- 14 Poisson process, birth–death process, and renewal process
- 15 Discrete-time Markov chains
- 16 Semi-Markov processes and continuous-time Markov chains
- 17 Random walk, Brownian motion, diffusion, and Itô processes
- Part IV Statistical inference
- Part V Applications and advanced topics
- References
- Index
Summary
In this and following chapters, we will discuss random processes. After a brief introduction to this subject in Section 12.1, we will give an overview of various random processes in Section 12.2 and then discuss (strictly) stationary and wide-sense stationary random processes and introduce the notion of ergodicity. The last section focuses on complex-valued Gaussian processes, which will be useful in the study of communication systems and other applications.
Random process
There are many situations in which the time dependency of a set of probability functions is important. One example is a noise process that accompanies a signal process and should be suppressed or filtered out so that we can recover the signal reliably and accurately. Another example is the amount of outstanding packets yet to be processed at a network router or switch, which may lead to undesirable packet loss due to buffer overflows if not properly attended to in time.
Such a process can be conveniently characterized probabilistically by extending the notion of a random variable (RV) as follows: we assign to each sample point ω ∈ Ω a real-valued functionX(ω, t), where t is the time parameter or index parameter in some range T, which may be, for instance, T = (-∞, ∞) or T = {0, 1, 2, …} (see Figure 12.1). Imagine that we can observe this set of time functions {X(ω, t); ω ∈ Ω, t ∈ T} at some instant t = t1.
- Type
- Chapter
- Information
- Probability, Random Processes, and Statistical AnalysisApplications to Communications, Signal Processing, Queueing Theory and Mathematical Finance, pp. 315 - 342Publisher: Cambridge University PressPrint publication year: 2011