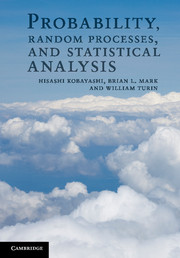
Book contents
- Frontmatter
- Contents
- List of abbreviations and acronyms
- Preface
- Acknowledgments
- 1 Introduction
- Part I Probability, random variables, and statistics
- Part II Transform methods, bounds, and limits
- Part III Random processes
- Part IV Statistical inference
- Part V Applications and advanced topics
- 20 Hidden Markov models and applications
- 21 Probabilistic models in machine learning
- 22 Filtering and prediction of random processes
- 23 Queueing and loss models
- References
- Index
20 - Hidden Markov models and applications
from Part V - Applications and advanced topics
Published online by Cambridge University Press: 05 June 2012
- Frontmatter
- Contents
- List of abbreviations and acronyms
- Preface
- Acknowledgments
- 1 Introduction
- Part I Probability, random variables, and statistics
- Part II Transform methods, bounds, and limits
- Part III Random processes
- Part IV Statistical inference
- Part V Applications and advanced topics
- 20 Hidden Markov models and applications
- 21 Probabilistic models in machine learning
- 22 Filtering and prediction of random processes
- 23 Queueing and loss models
- References
- Index
Summary
Introduction
In this chapter we shall discuss hidden Markov models (HMMs), which have been widely applied to a broad range of science and engineering problems, including speech recognition, decoding and channel modeling in digital communications, computational biology (e.g., DNA and protein sequencing), and modeling of communication networks.
In an ordinary Markov model, transitions between the states characterize the dynamics of a system in question, and we implicitly assume that a sequence of states can be directly observed, and the observer may even know the structure and parameters of the Markov model. In some fields, such as speech recognition and network traffic modeling, it is useful to remove these restrictive assumptions and construct a model in which the observable output is a probabilistic function of the underlying Markov state. Such a model is referred to as an HMM.
We shall address the important problems of state and parameter estimation associated with an HMM: What is the likelihood that an observed data is generated from this model? How can we infer the most likely state or sequence of states, given a particular observed output? Given observed data, how can we estimate the most likely value of the model parameters, i.e., their MLEs? We will present in a cohesive manner a series of computational algorithms for state and parameter estimation, including the forward and backward recursion algorithms, the Viterbi algorithm, the BCJR algorithm, and the Baum–Welch algorithm, which is a special case of the EM algorithm discussed in Section 19.2.
- Type
- Chapter
- Information
- Probability, Random Processes, and Statistical AnalysisApplications to Communications, Signal Processing, Queueing Theory and Mathematical Finance, pp. 573 - 614Publisher: Cambridge University PressPrint publication year: 2011