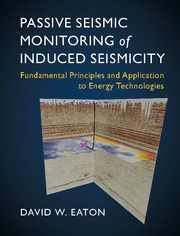
Book contents
- Frontmatter
- Contents
- Preface
- List of Symbols
- Part I Fundamentals of Passive Seismic Monitoring
- Part II Applications of Passive Seismic Monitoring
- 5 Passive-Seismic Data Acquisition
- 6 Downhole Microseismic Processing
- 7 Surface and Shallow-Array Microseismic Processing
- 8 Microseismic Interpretation
- 9 Induced Seismicity
- Appendix A Glossary
- Appendix B Signal-Processing Essentials
- Appendix C Data Formats
- References
- Index
7 - Surface and Shallow-Array Microseismic Processing
from Part II - Applications of Passive Seismic Monitoring
Published online by Cambridge University Press: 07 June 2018
- Frontmatter
- Contents
- Preface
- List of Symbols
- Part I Fundamentals of Passive Seismic Monitoring
- Part II Applications of Passive Seismic Monitoring
- 5 Passive-Seismic Data Acquisition
- 6 Downhole Microseismic Processing
- 7 Surface and Shallow-Array Microseismic Processing
- 8 Microseismic Interpretation
- 9 Induced Seismicity
- Appendix A Glossary
- Appendix B Signal-Processing Essentials
- Appendix C Data Formats
- References
- Index
Summary
Indeed, we often mark our progress in science by improvements in imaging.
Martin Chalfie (Nobel Lecture, 2008)Although the goals of surface-based microseismic monitoring are the same as those for downhole monitoring, the data-processing methods are quite distinct. For acquisition programs that are deployed at or near the surface, the relatively large number of sensors (up to thousands), lower S/N environment and larger aperture lead to a processing approach that is typically more akin to imaging methods used for controlled-source seismic exploration than to methods used for earthquake seismology (Duncan and Eisner, 2010). In order to detect weak events, surface microseismic monitoring exploits the power of stacking and other signal-processing methods for noise reduction and enhancement of signal coherence. Shallow-well arrays constitute a hybrid approach; with this acquisition design, the seismic wavefield is recorded with sparser spatial sampling in a higher S/N environment, but the aperture and overall recording geometry – that is viewing from above – remain almost the same as for surface arrays.
The theoretical groundwork for source imaging using surface arrays was laid by McMechan (1982), who observed that seismic sources can be imaged by propagation of the observed wavefield backwards in time. This approach, in which the observed seismograms are treated as time-dependent boundary values, provided a foundation for reverse-time imaging (RTI) (Artman et al., 2010). Kao and Shan (2004) proposed an alternative method, which they called a source-scanning algorithm (SSA). This method operates by summing absolute amplitudes observed at all stations at predicted arrival times for a set of trial hypocentre locations and origin times. SSA belongs to a class of source-imaging methods that are based on a form of Kirchhoff migration.
The emergence, starting in 2004, of surface-based microseismic methods for monitoring hydraulic fracturing (Duncan, 2005; Lakings et al., 2006) was accompanied by vigorous technical debate (Eisner et al., 2011b, a). Given then-established low-magnitude characteristics of microseismicity induced by hydraulic fracturing (Warpinski et al., 1998; Maxwell et al., 2002; Rutledge and Phillips, 2003), the debate centred on the ultimate detection capabilities of sensors deployed in noisy surface environments. Skeptics argued that S/N improvements from stacking saturate at an upper limit, as demonstrated by Cieslik and Artman (2016), and suggested that amplitude loss from geometrical spreading and inelastic attenuation are insurmountable, irrespective of array design.
- Type
- Chapter
- Information
- Passive Seismic Monitoring of Induced SeismicityFundamental Principles and Application to Energy Technologies, pp. 190 - 208Publisher: Cambridge University PressPrint publication year: 2018