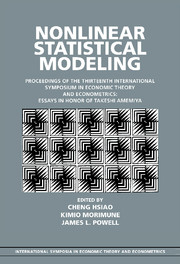
Book contents
- Frontmatter
- Contents
- Series editor's introduction
- Editors' introduction
- Contributors
- 1 Local instrumental variables
- 2 Empirically relevant power comparisons for limited-dependent-variable models
- 3 Simulation estimation of polychotomous-choice sample selection models
- 4 A new approach to the attrition problem in longitudinal studies
- 5 Semiparametric estimation for left-censored duration models
- 6 Semiparametric estimation of censored selection models
- 7 Studentization in Edgeworth expansions for estimates of semiparametric index models
- 8 Nonparametric identification under response-based sampling
- 9 On selecting regression variables to maximize their significance
- 10 Using information on the moments of disturbances to increase the efficiency of estimation
- 11 Minimal conditions for weak convergence of the sample standardized spectral distribution function
- 12 Unit root tests for time series with a structural break when the break point is known
- 13 Power comparisons of the discontinuous trend unit root tests
- 14 On the simultaneous switching autoregressive model
- 15 Some econometrics of scarring
- 16 A censored switching regression approach to evaluating the effect of sunk costs and firm-level disequilibrium on export performance
- Curriculum vitae of Takeshi Amemiya
- Index
9 - On selecting regression variables to maximize their significance
Published online by Cambridge University Press: 05 June 2012
- Frontmatter
- Contents
- Series editor's introduction
- Editors' introduction
- Contributors
- 1 Local instrumental variables
- 2 Empirically relevant power comparisons for limited-dependent-variable models
- 3 Simulation estimation of polychotomous-choice sample selection models
- 4 A new approach to the attrition problem in longitudinal studies
- 5 Semiparametric estimation for left-censored duration models
- 6 Semiparametric estimation of censored selection models
- 7 Studentization in Edgeworth expansions for estimates of semiparametric index models
- 8 Nonparametric identification under response-based sampling
- 9 On selecting regression variables to maximize their significance
- 10 Using information on the moments of disturbances to increase the efficiency of estimation
- 11 Minimal conditions for weak convergence of the sample standardized spectral distribution function
- 12 Unit root tests for time series with a structural break when the break point is known
- 13 Power comparisons of the discontinuous trend unit root tests
- 14 On the simultaneous switching autoregressive model
- 15 Some econometrics of scarring
- 16 A censored switching regression approach to evaluating the effect of sunk costs and firm-level disequilibrium on export performance
- Curriculum vitae of Takeshi Amemiya
- Index
Summary
Introduction
Often in applied linear regression analysis one must select an explanatory variable from a set of candidates. For example, in estimating production functions one must select among alternative measures of capital stock constructed using different depreciation assumptions. Or, in hedonic analysis of housing prices, one may use indicator or ramp variables that measure distance from spatial features such as parks or industrial plants, with cutoffs at distances that are determined as parameters. In the second example, the problem can be cast as one of nonlinear regression. However, when there are many linear parameters in the regression, direct nonlinear regression can be computationally inefficient, with convergence problematic. It is often more practical to approach this as a linear regression problem with variable selection.
This chapter shows that selecting variables in a linear regression to maximize their conventional significance is equivalent to direct application of nonlinear least squares. Thus, this method provides a practical computational shortcut that shares the statistical properties of the nonlinear least-squares solution. However, standard errors and test statistics produced by least squares are biased by variable selection, and are often inconsistent. This chapter gives practical consistent estimators for covariances and test statistics, and shows in examples that kernelsmoothing or bootstrap methods appear to give adequate approximations in samples of moderate size.
- Type
- Chapter
- Information
- Nonlinear Statistical ModelingProceedings of the Thirteenth International Symposium in Economic Theory and Econometrics: Essays in Honor of Takeshi Amemiya, pp. 259 - 280Publisher: Cambridge University PressPrint publication year: 2001
- 1
- Cited by