Book contents
- Handbook of Psychophysiology, Fourth Edition
- Handbook of Psychophysiology
- Copyright page
- Contents
- Figures
- Plates
- Tables
- Contributors
- Foundations
- Systemic Psychophysiology
- 4 Fundamentals of Functional Neuroimaging
- 5 Electroencephalography and Event-Related Brain Potentials
- 6 High-Performance Electrophysiological Microsegmentation and Brain Source Localization
- 7 Application of Non-Invasive Brain Stimulation in Psychophysiology
- 8 The Somatic System
- 9 Cardiovascular Psychophysiology
- 10 The Electrodermal System
- 11 The Respiratory System
- 12 Gastrointestinal System
- 13 Sexual Response
- 14 Ambulatory and Non-Contact Recording Methods
- 15 Behavior Genetics: From Heritability to Gene Finding
- 16 Functional Genomic Approaches to Psychophysiology
- 17 Psychoneuroimmunology
- Topical Psychophysiology
- General Methods
- Index
- Plate section (PDF only)
- References
4 - Fundamentals of Functional Neuroimaging
from Systemic Psychophysiology
Published online by Cambridge University Press: 27 January 2017
- Handbook of Psychophysiology, Fourth Edition
- Handbook of Psychophysiology
- Copyright page
- Contents
- Figures
- Plates
- Tables
- Contributors
- Foundations
- Systemic Psychophysiology
- 4 Fundamentals of Functional Neuroimaging
- 5 Electroencephalography and Event-Related Brain Potentials
- 6 High-Performance Electrophysiological Microsegmentation and Brain Source Localization
- 7 Application of Non-Invasive Brain Stimulation in Psychophysiology
- 8 The Somatic System
- 9 Cardiovascular Psychophysiology
- 10 The Electrodermal System
- 11 The Respiratory System
- 12 Gastrointestinal System
- 13 Sexual Response
- 14 Ambulatory and Non-Contact Recording Methods
- 15 Behavior Genetics: From Heritability to Gene Finding
- 16 Functional Genomic Approaches to Psychophysiology
- 17 Psychoneuroimmunology
- Topical Psychophysiology
- General Methods
- Index
- Plate section (PDF only)
- References
Summary
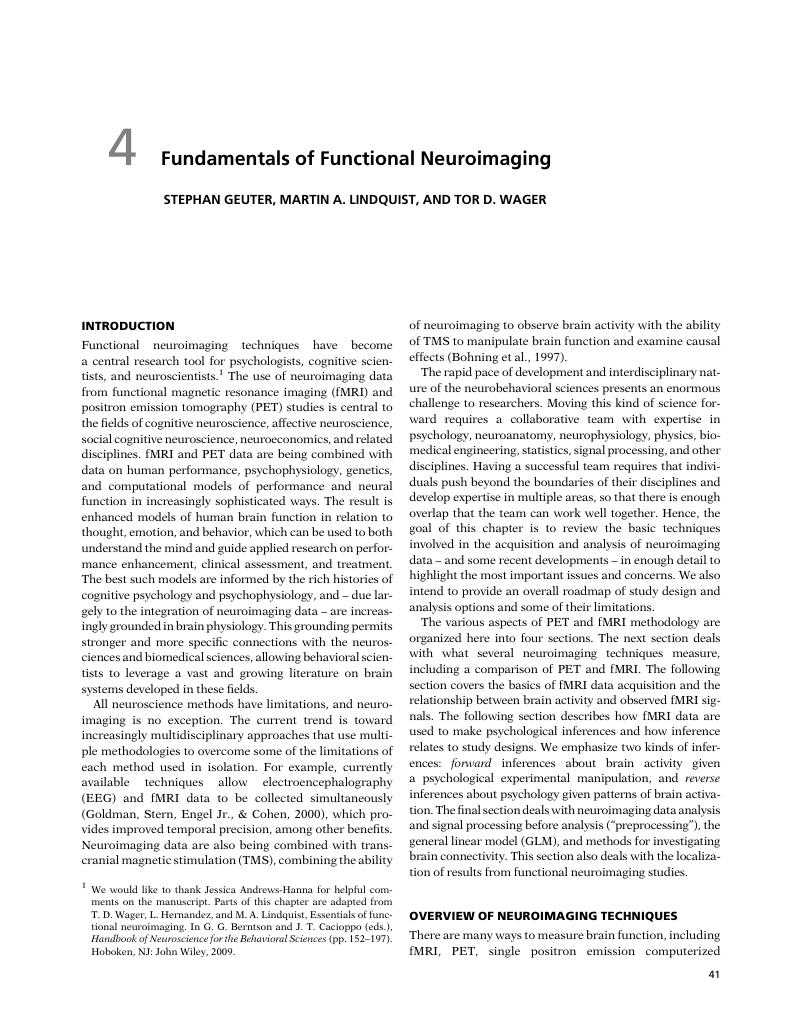
- Type
- Chapter
- Information
- Handbook of Psychophysiology , pp. 41 - 73Publisher: Cambridge University PressPrint publication year: 2016
References
- 3
- Cited by