Book contents
- The Handbook of Medical Image Perception and Techniques, Second Edition
- Reviews
- The Handbook of Medical Image Perception and Techniques
- Copyright page
- Dedication
- Contents
- Contributors
- 1 Medical Image Perception
- Part I Historical Reflections and Theoretical Foundations
- Part II Science of Image Perception
- Part III Perception Metrology
- Part IV Clinical Performance Assessment
- Part V Computational Perception
- Part VI Applied Perception
- Index
- References
1 - Medical Image Perception
Published online by Cambridge University Press: 20 December 2018
- The Handbook of Medical Image Perception and Techniques, Second Edition
- Reviews
- The Handbook of Medical Image Perception and Techniques
- Copyright page
- Dedication
- Contents
- Contributors
- 1 Medical Image Perception
- Part I Historical Reflections and Theoretical Foundations
- Part II Science of Image Perception
- Part III Perception Metrology
- Part IV Clinical Performance Assessment
- Part V Computational Perception
- Part VI Applied Perception
- Index
- References
Summary
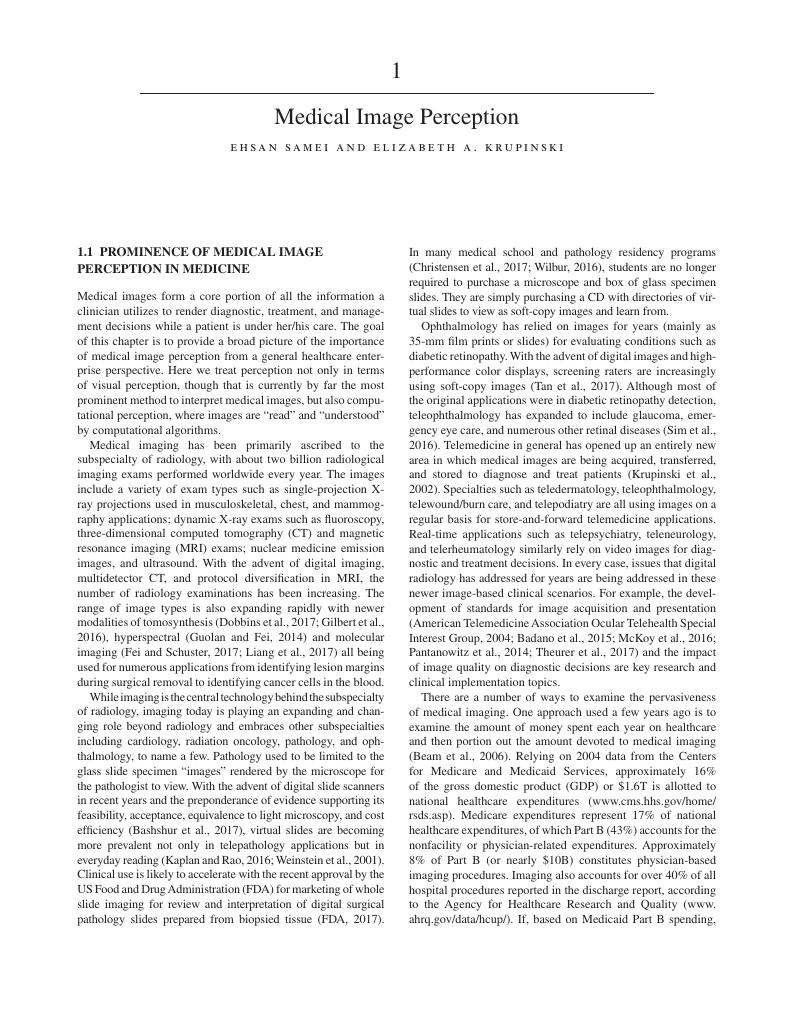
- Type
- Chapter
- Information
- Publisher: Cambridge University PressPrint publication year: 2018
References
- 4
- Cited by