Book contents
- Frontmatter
- Contents
- Preface
- 1 Greedy approximation with regard to bases
- 2 Greedy approximation with respect to dictionaries: Hilbert spaces
- 3 Entropy
- 4 Approximation in learning theory
- 5 Approximation in compressed sensing
- 6 Greedy approximation with respect to dictionaries: Banach spaces
- References
- Index
- References
References
Published online by Cambridge University Press: 05 August 2012
- Frontmatter
- Contents
- Preface
- 1 Greedy approximation with regard to bases
- 2 Greedy approximation with respect to dictionaries: Hilbert spaces
- 3 Entropy
- 4 Approximation in learning theory
- 5 Approximation in compressed sensing
- 6 Greedy approximation with respect to dictionaries: Banach spaces
- References
- Index
- References
Summary
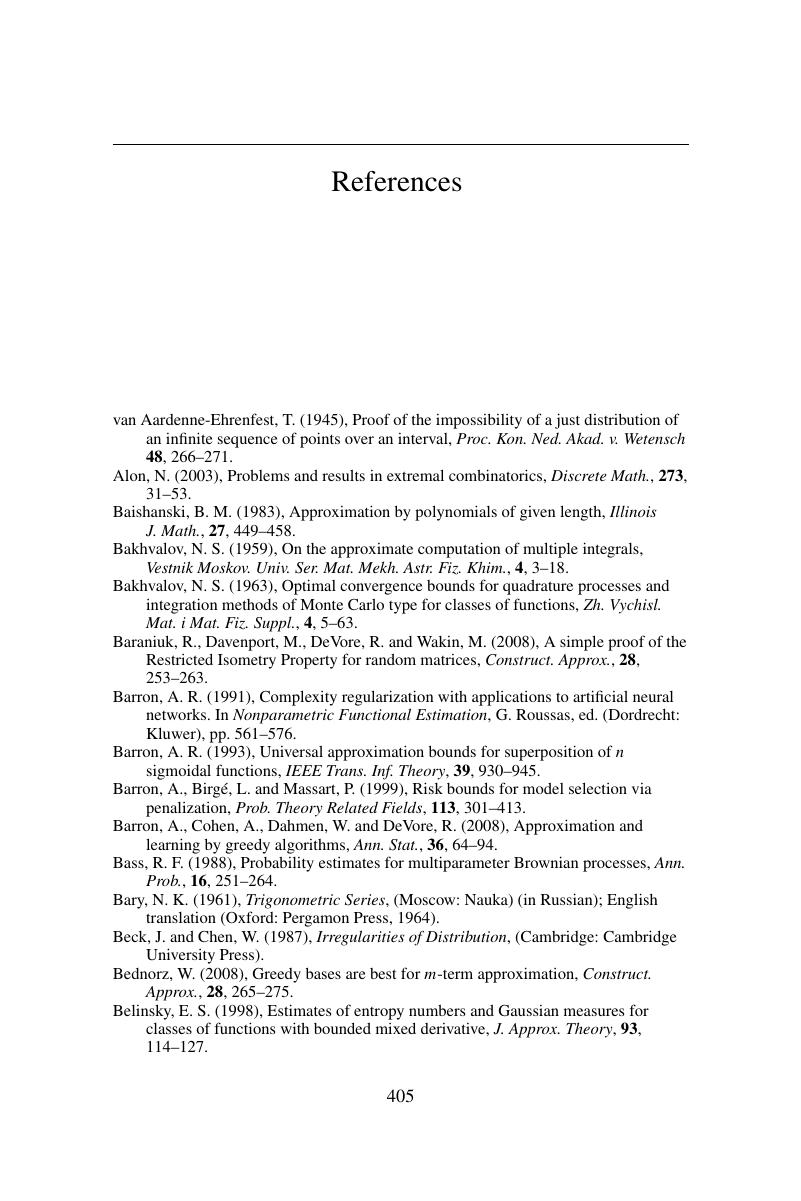
- Type
- Chapter
- Information
- Greedy Approximation , pp. 405 - 414Publisher: Cambridge University PressPrint publication year: 2011