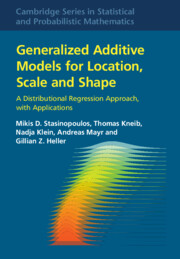
References
Published online by Cambridge University Press: 22 February 2024
Summary

- Type
- Chapter
- Information
- Generalized Additive Models for Location, Scale and ShapeA Distributional Regression Approach, with Applications, pp. 271 - 282Publisher: Cambridge University PressPrint publication year: 2024