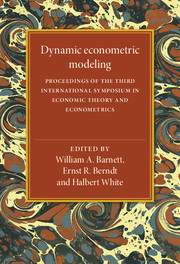
Book contents
- Frontmatter
- Contents
- Editors' introduction
- List of contributors
- Part I Dynamic structural modeling
- 1 Efficient instrumental variables estimation of systems of implicit heterogeneous nonlinear dynamic equations with nonspherical errors
- 2 Envelope consistent functional separability
- 3 Flexible functional forms for profit functions and global curvature conditions
- 4 Likelihood inference in the nonlinear regression model with explosive linear dynamics
- 5 Exact inference in models with autoregressive conditional heteroscedasticity
- 6 Control of a linear regression process with unknown parameters
- 7 Some tests of nonparametric regression models
- Part II Linear time series modeling
- Part III Chaotic attractor modeling
- Part IV Applications
5 - Exact inference in models with autoregressive conditional heteroscedasticity
Published online by Cambridge University Press: 03 May 2010
- Frontmatter
- Contents
- Editors' introduction
- List of contributors
- Part I Dynamic structural modeling
- 1 Efficient instrumental variables estimation of systems of implicit heterogeneous nonlinear dynamic equations with nonspherical errors
- 2 Envelope consistent functional separability
- 3 Flexible functional forms for profit functions and global curvature conditions
- 4 Likelihood inference in the nonlinear regression model with explosive linear dynamics
- 5 Exact inference in models with autoregressive conditional heteroscedasticity
- 6 Control of a linear regression process with unknown parameters
- 7 Some tests of nonparametric regression models
- Part II Linear time series modeling
- Part III Chaotic attractor modeling
- Part IV Applications
Summary
Introduction
Virtually without exception, inference in dynamic econometric models of aggregate economic time series data is based on the asymptotic sampling–theoretic distribution of estimators, often maximum–likelihood estimators. There is no population for which sets of observations of aggregate economic time series can be drawn repeatedly, as may be the case with longitudinal or panel data. Sampling–theoretic results for independent populations have been extended to inference from a single realization of a time series, but the length of periods for which parametric models of economic time series may reasonably be regarded as stable usually limit attention to modest departures from the linear model. There is no way to incorporate carefully the inequality restrictions that often emerge from economic theory. Improvements on asymptotic theory, especially for highly nonlinear estimators, are arduous theoretical tasks whose occasional completion seems to have had little effect on the way applied work is carried out.
This situation and recent drastic reductions in computing costs suggest that alternatives to this standard approach to inference for economic time series be contemplated. This chapter explores a formal numerical Bayesian approach with diffuse priors, relying on cheap computing to disregard the analytical intractability of useful priors and functions of the parameters of interest. The ability to select diffuse priors arbitrarily means that inequality restrictions can be imposed, and the power to choose functions of interest for their bearing on empirical questions means that issues relegated to equivocal discussions can be treated formally.
- Type
- Chapter
- Information
- Dynamic Econometric ModelingProceedings of the Third International Symposium in Economic Theory and Econometrics, pp. 73 - 104Publisher: Cambridge University PressPrint publication year: 1988
- 5
- Cited by