Book contents
- Frontmatter
- Dedication
- Contents
- Preface
- 1 Introduction
- 2 Basic Concepts
- 3 Shallow Networks and Shallow Learning
- 4 Two-Layer Networks and Universal Approximation
- 5 Autoencoders
- 6 Deep Networks and Backpropagation
- 7 The Local Learning Principle
- 8 The Deep Learning Channel
- 9 Recurrent Networks
- 10 Recursive Networks
- 11 Applications in Physics
- 12 Applications in Chemistry
- 13 Applications in Biology and Medicine
- 14 Conclusion
- Appendix A Reinforcement Learning and Deep Reinforcement Learning
- Appendix B Hints and Remarks for Selected Exercises
- References
- Index
- References
References
Published online by Cambridge University Press: 17 April 2021
- Frontmatter
- Dedication
- Contents
- Preface
- 1 Introduction
- 2 Basic Concepts
- 3 Shallow Networks and Shallow Learning
- 4 Two-Layer Networks and Universal Approximation
- 5 Autoencoders
- 6 Deep Networks and Backpropagation
- 7 The Local Learning Principle
- 8 The Deep Learning Channel
- 9 Recurrent Networks
- 10 Recursive Networks
- 11 Applications in Physics
- 12 Applications in Chemistry
- 13 Applications in Biology and Medicine
- 14 Conclusion
- Appendix A Reinforcement Learning and Deep Reinforcement Learning
- Appendix B Hints and Remarks for Selected Exercises
- References
- Index
- References
Summary
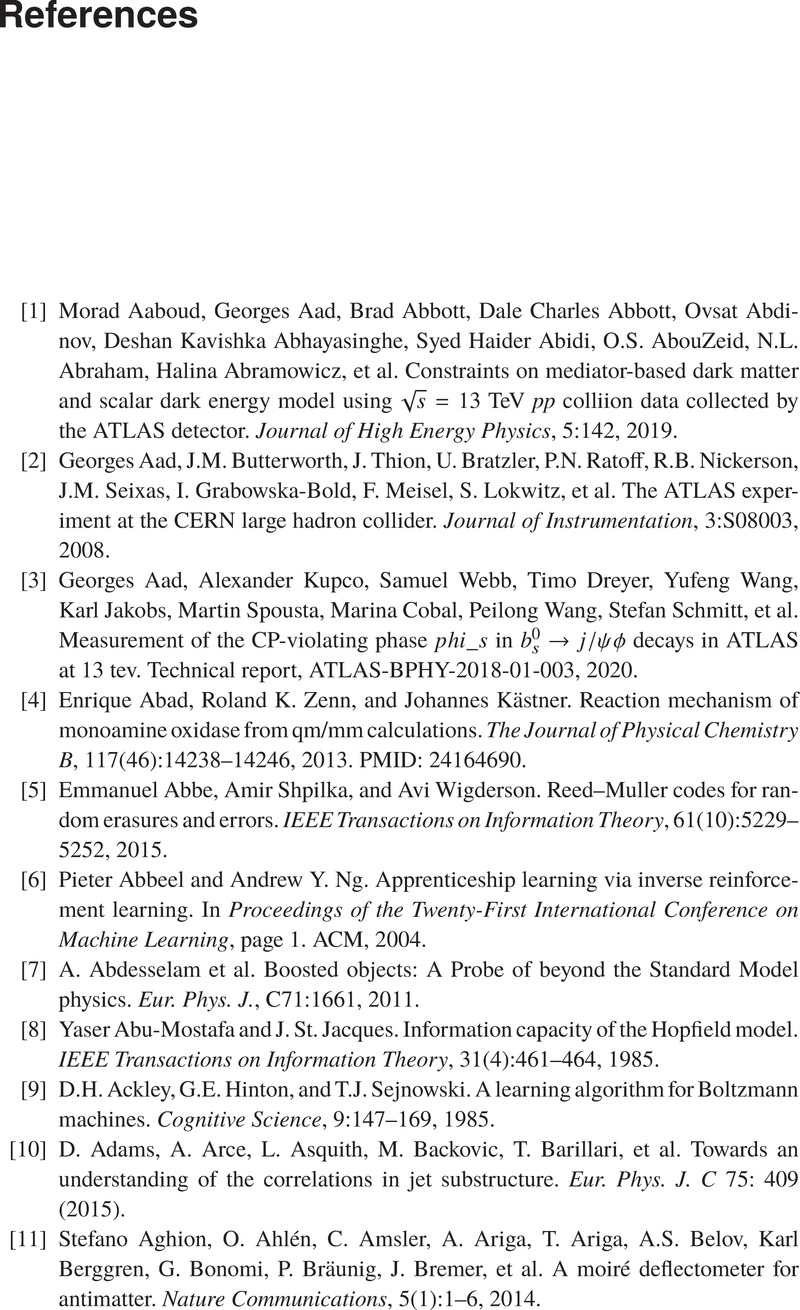
- Type
- Chapter
- Information
- Deep Learning in Science , pp. 313 - 364Publisher: Cambridge University PressPrint publication year: 2021