109 results
ActuaryGPT: applications of large language models to insurance and actuarial work
-
- Journal:
- British Actuarial Journal / Volume 29 / 2024
- Published online by Cambridge University Press:
- 21 November 2024, e15
-
- Article
-
- You have access
- Open access
- HTML
- Export citation
Detection of suicidality from medical text using privacy-preserving large language models
-
- Journal:
- The British Journal of Psychiatry , FirstView
- Published online by Cambridge University Press:
- 05 November 2024, pp. 1-6
-
- Article
-
- You have access
- Open access
- HTML
- Export citation
Realizing the potential of social determinants data in EHR systems: A scoping review of approaches for screening, linkage, extraction, analysis, and interventions
-
- Journal:
- Journal of Clinical and Translational Science / Volume 8 / Issue 1 / 2024
- Published online by Cambridge University Press:
- 10 October 2024, e147
-
- Article
-
- You have access
- Open access
- HTML
- Export citation
PopBERT. Detecting Populism and Its Host Ideologies in the German Bundestag
-
- Journal:
- Political Analysis , First View
- Published online by Cambridge University Press:
- 01 October 2024, pp. 1-17
-
- Article
-
- You have access
- Open access
- HTML
- Export citation
Stance detection: a practical guide to classifying political beliefs in text
-
- Journal:
- Political Science Research and Methods , First View
- Published online by Cambridge University Press:
- 19 September 2024, pp. 1-18
-
- Article
-
- You have access
- Open access
- HTML
- Export citation
Detecting suicide risk among U.S. servicemembers and veterans: a deep learning approach using social media data
-
- Journal:
- Psychological Medicine / Volume 54 / Issue 12 / September 2024
- Published online by Cambridge University Press:
- 09 September 2024, pp. 3379-3388
-
- Article
-
- You have access
- HTML
- Export citation
Quality of legislation and compliance: a natural language processing approach
-
- Journal:
- Political Science Research and Methods , First View
- Published online by Cambridge University Press:
- 15 August 2024, pp. 1-9
-
- Article
-
- You have access
- Open access
- HTML
- Export citation
EHMMQA: English, Hindi, and Marathi multilingual question answering framework using deep learning
-
- Journal:
- Natural Language Processing ,
- Published online by Cambridge University Press:
- 24 May 2024, pp. 1-29
-
- Article
-
- You have access
- Open access
- HTML
- Export citation
35 - Natural Language Processing
- from Part 6 - Experimental and Quantitative Approaches
-
-
- Book:
- The Cambridge Handbook of Slavic Linguistics
- Published online:
- 16 May 2024
- Print publication:
- 23 May 2024, pp 732-750
-
- Chapter
- Export citation
The ENACT network is acting on housing instability and the unhoused using the open health natural language processing toolkit
-
- Journal:
- Journal of Clinical and Translational Science / Volume 8 / Issue 1 / 2024
- Published online by Cambridge University Press:
- 16 May 2024, e98
-
- Article
-
- You have access
- Open access
- HTML
- Export citation
Predicting the Utility of Scientific Articles for Emerging Pandemics Using Their Titles and Natural Language Processing
-
- Journal:
- Disaster Medicine and Public Health Preparedness / Volume 18 / 2024
- Published online by Cambridge University Press:
- 10 May 2024, e103
-
- Article
-
- You have access
- HTML
- Export citation
7 - Computational Approaches to Language
-
- Book:
- The Philosophy of Theoretical Linguistics
- Published online:
- 25 April 2024
- Print publication:
- 02 May 2024, pp 157-182
-
- Chapter
- Export citation
The innovation paradox: concept space expansion with diminishing originality and the promise of creative artificial intelligence
-
- Journal:
- Design Science / Volume 10 / 2024
- Published online by Cambridge University Press:
- 19 April 2024, e11
-
- Article
-
- You have access
- Open access
- HTML
- Export citation
Identifying incarceration status in the electronic health record using large language models in emergency department settings
-
- Journal:
- Journal of Clinical and Translational Science / Volume 8 / Issue 1 / 2024
- Published online by Cambridge University Press:
- 11 March 2024, e53
-
- Article
-
- You have access
- Open access
- HTML
- Export citation
Actuarial applications of natural language processing using transformers: Case studies for using text features in an actuarial context
-
- Journal:
- British Actuarial Journal / Volume 29 / 2024
- Published online by Cambridge University Press:
- 07 March 2024, e4
-
- Article
-
- You have access
- Open access
- HTML
- Export citation
Contrasting the semantic space of ‘shame’ and ‘guilt’ in English and Japanese
-
- Journal:
- Language and Cognition , First View
- Published online by Cambridge University Press:
- 01 March 2024, pp. 1-23
-
- Article
-
- You have access
- Open access
- HTML
- Export citation
4 - Connectives between Syntax and Discourse
-
- Book:
- Connectives and Discourse Relations
- Published online:
- 22 February 2024
- Print publication:
- 29 February 2024, pp 72-91
-
- Chapter
- Export citation
1 - Introduction
-
- Book:
- Deep Learning for Natural Language Processing
- Published online:
- 01 February 2024
- Print publication:
- 08 February 2024, pp 1-7
-
- Chapter
- Export citation
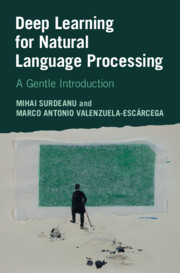
Deep Learning for Natural Language Processing
- A Gentle Introduction
-
- Published online:
- 01 February 2024
- Print publication:
- 08 February 2024
On clustering levels of a hierarchical categorical risk factor
-
- Journal:
- Annals of Actuarial Science , First View
- Published online by Cambridge University Press:
- 01 February 2024, pp. 1-39
-
- Article
-
- You have access
- HTML
- Export citation