Introduction
Language and working memory are highly related, to the extent that they have been characterized as having a reciprocal relationship (Archibald, Reference Archibald2018). A focus of the current study is phonological working memory, a capacity-limited process that temporarily maintains and manipulates auditory information (Baddeley, Reference Baddeley2000). Phonological working memory is implicated in various linguistic processes, including word learning (e.g., Gathercole, Reference Gathercole2006) and language comprehension (e.g., Robertson & Joanisse, Reference Robertson and Joanisse2010). Language abilities (e.g., vocabulary size) may also influence performance on working memory tasks, such as nonword repetition (e.g., Munson, Kurtz, & Windsor, Reference Munson, Kurtz and Windsor2005), which exemplifies this reciprocal relationship. Early experiences with language may affect working memory development, and vice versa (Delcenserie, Genesee, Trudeau & Champoux, Reference Delcenserie, Genesee, Trudeau and Champoux2020).
Working Memory
Working memory is a prolific topic in psychological literature and has been defined in different ways over the years (Cowan, Reference Cowan2017). Alongside multiple definitions, there exist several models of working memory. These vary in their proposed structure and individual components. Baddeley and Hitch’s (Reference Baddeley, Hitch and Bower1974) well-known multicomponent model consists of two capacity-limited stores – the phonological loop and visuospatial sketchpad – that hold auditory and visual sensory information, respectively. Its third component, the central executive, controls the flow of information and shift of attentional focus between the sensory stores. A fourth component, the episodic buffer, was later added to the model and proposed to bind information from different sources, such as long-term memory and the phonological loop (Baddeley, Reference Baddeley2000). A less modular model was proposed by Cowan (Reference Cowan1988): the embedded processes model. This model includes a central executive that mediates attentional focus, a focus of attention that may be directed at incoming stimuli, long-term memory, or both, as well as a phonological storage and rehearsal mechanism.
In the current study, we discuss working memory in terms of a combined model that has elements of both Baddeley and Hitch’s and Cowan’s models. This combined model was based on seven- to nine-year-old children’s performance on a battery of working memory tasks (Gray, Green, Alt, Hogan, Kuo, Brinkley & Cowan, Reference Cowan2017). Tasks were designed to test the theoretical constructs associated with Baddeley and Hitch’s and Cowan’s models (e.g., central executive, short-term phonological memory, short-term visuospatial memory). Results suggested that the data fit a combined model of working memory consisting of: 1) a central executive, 2) a focus of attention/visuospatial sketchpad, and 3) a phonological storage and rehearsal component, akin to Baddeley and Hitch’s phonological loop (Gray et al., Reference Gray, Green, Alt, Hogan, Kuo, Brinkley and Cowan2017). We use this combined model as a framework for working memory in the current study because the data used for our analyses came from children’s performance on the working memory tasks from which the model was derived.
In this combined model of working memory, processing of phonological material is represented by a single component. Importantly, the model did not provide evidence for further subdivision of this component. That is, there was no evidence for a simple short-term phonological storage component (i.e., simple short-term storage, the process involved in forward digit recall tasks) separate from a component responsible for more complex manipulation of phonological information (i.e., mental reconfiguration of information, the process involved in backwards digit recall tasks). The phonological working memory tasks used in the current study (i.e., nonword repetition, digit span, and phonological binding) statistically significantly loaded onto the phonological component in the working memory model. For this reason, we treat all three tasks as tapping into a unitary process that is responsible for phonological working memory in the current study.
Phonological Working Memory and Sentence Production
In general, empirical evidence for the role of working memory in language production is scarce (Acheson & MacDonald, Reference Acheson and MacDonald2009; Cowan, Reference Cowan2017). However, it is plausible that phonological working memory plays a role in the formulation stage immediately before a speaker physically produces the message with their articulators. Established models of speech and language production, such as Levelt, Roelofs, and Meyer’s (Reference Levelt, Roelofs and Meyer1999) model and Garrett’s (Reference Garrett and Butterworth1980) model (as cited in Rofes, Mandonnet, de Aguiar, Rapp, Tsapkini & Miceli, Reference Rofes, Mandonnet, de Aguiar, Rapp, Tsapkini and Miceli2019) include an intermediate formulation stage in which a speaker’s conceptual message is mapped onto linguistic forms through lexical selection and encoding of grammatical and phonological information (see Figure 1 in Levelt et al., Reference Levelt, Roelofs and Meyer1999; and Figure 2 in Rofes et al., Reference Rofes, Mandonnet, de Aguiar, Rapp, Tsapkini and Miceli2019). Although these models do not explicitly account for phonological working memory, we posit that it is within these intermediate stages where it would have an effect.

Figure 1. Scatter Plot of Raw Formulated Sentences Scaled Scores and Nonword Repetition Scores

Figure 2. Scatter Plot of Raw Formulated Sentences Scaled Scores and Digit Span Scores
Based on available empirical evidence, there is reason to expect a link between phonological working memory and sentence production. Phonological working memory has been hypothesized to be a buffer for speech production (Adams & Gathercole, Reference Adams and Gathercole1995), but it may similarly act as an output buffer for language. When communicating, a speaker retrieves words from long-term memory. They then produce the words in rule-governed order to ensure the sentence is grammatical and coherent. One might expect that the better a person’s working memory, the better they can maintain words, ideas, and rules in mind to form grammatical and coherent sentences. As a result, someone with poor phonological working memory might have qualitatively different (e.g., shorter, less lexically diverse, less complex) sentences than someone with better phonological working memory.
Empirical evidence on the relationship between sentence production and phonological working memory is especially scarce for school-age children. In their study with school-age children, Torrens and Yagüe (Reference Torrens and Yagüe2018) found a strong, positive correlation between these constructs. They examined the correlation between scores on a sentence-level language production task and phonological working memory measures, including nonword repetition and digit span, for school-age children with and without developmental language disorderFootnote 1 (DLD). Their language production task was taken from the Prueba de Lenguaje Oral de Navarra (Navarra Oral Language Test; Aguinaga, Armentia, Fraile, Olangua & Uriz, Reference Aguinaga, Armentia, Fraile, Olangua and Uriz2004) and had two subtasks: (a) one for sentence repetition and (b) another for novel sentence formulation. They calculated an aggregate score based on children’s performance on both components. Results showed a strong correlation between children’s scores on this language production task and the phonological working memory tasks. However, we are limited in the extent to which we can generalize these findings to the relationship between phonological working memory and sentence production as it occurs in the real world. The imitative nature of the sentence repetition subtask was highly similar to the working memory tasks, and therefore might have influenced the strength of the observed correlation. It is unclear how much the novel sentence formulation task added to the correlation. Additionally, sentence repetition is not representative of everyday communication; more generalizable insight could come from examining children’s performance only on formulation of novel sentences. However, to our knowledge, no study has done this. Therefore, one goal of the current study is to fill this gap and examine the correlation between novel sentence production and phonological working memory in school-age children. If a strong correlation were found, it would warrant further research into this area, as it would provide insight into how sentence production difficulties might be tied to an underlying relationship with phonological working memory.
A related area for which there is limited evidence with school-age children is the link between phonological working memory and the length, lexical diversity, and complexity of sentences they produce. Examining this level of sentence production in relation to phonological working memory in school-age children is not only novel, but it is of particular interest to this age group because their oral language skills are still developing in terms of length, vocabulary, and syntactic complexity (e.g., Nippold, Reference Nippold1993). However, it is an open question how phonological working memory skills impact these qualitative sentence characteristics.
Though we have limited direct evidence on the relationship between phonological working memory and sentence length, lexical diversity, and complexity in school-age children, extant studies suggest a link between these variables in preschoolers. Adams and Gathercole (Reference Adams and Gathercole1995) found that preschoolers with low phonological working memory (measured by nonword repetition and digit span tasks) produced shorter, less lexically diverse, and less complex sentences during a language sample than their peers with high phonological working memory. Blake, Austin, Cannon, Lisus, and Vaughan (Reference Blake, Austin, Cannon, Lisus and Vaughan1994) found a positive correlation between performance on a word span task and mean length of utterance for preschoolers under 3.5 years old. To our knowledge, no study has examined the relationship between phonological working memory and the length, lexical diversity, and complexity of novel sentences produced by school-age children. Therefore, a second goal of the current study is to fill this literature gap and determine if better phonological working memory skills in school-age children are associated with production of longer, more lexically diverse, and more complex sentences.
Why might there be a link between phonological working memory abilities and the length, lexical diversity, and complexity of sentences that school-age children produce? In regard to length, phonological working memory may serve as a mental holding place for the covert formulation of sentences immediately prior to their overt production. This perspective treats phonological working memory as an output buffer for language, akin to the hypothesis that it is a buffer for speech output (Adams & Gathercole, Reference Adams and Gathercole1995). Individuals with better phonological working memory capacity might be able to retrieve and maintain more words in working memory during the covert formulation phase, in turn allowing them to overtly produce longer – but still grammatical and coherent – sentences. In contrast, when those with poorer phonological working memory produce grammatical, coherent sentences, they may tend to be shorter; if they do produce longer sentences, these sentences would predictably contain more grammatical or lexical errors because the length of the sentence would exceed what they can maintain in working memory. In regard to lexical diversity, speakers with better phonological working memory might be more likely to have a larger lexicon to draw from when speaking, thus resulting in increased lexical diversity. This is expected given evidence of positive links between phonological working memory and word learning and vocabulary knowledge (Baddeley, Gathercole & Papagno, Reference Baddeley, Gathercole and Papagno1998; Gathercole, Reference Gathercole2006; Gupta, Reference Gupta2003; Montgomery, Magimairaj & Finney, Reference Montgomery, Magimairaj and Finney2010; Stokes, Klee, Kornisch & Furlong, Reference Stokes, Klee, Kornisch and Furlong2017). Speakers with better phonological working memory might therefore be more likely to produce more lexically diverse sentences because their strong phonological working memory abilities have positively impacted their word learning and vocabulary knowledge. In regard to complexity, speakers with better phonological working memory might produce more complex sentences from the aforementioned perspective that phonological working memory is an output buffer for language. Complex sentences, defined here as sentences with embedded clauses or prepositional phrases, require speakers to maintain grammatical relations across multiple grammatical and semantic elements. In the complex sentence, The girl who is wearing a yellow shirt walks her dog, there are multiple grammatical dependencies which speakers must keep track of if they are to produce a grammatically correct, coherent sentence. Within the embedded clause, is must appear with -ing in order to form the present progressive tense. The singular subject girl must appear with the singular verb is in the embedded clause, as well as with the third person singular -s in the verb of the main clause, in order to maintain correct subject-verb number agreement. In the simple sentence, The girl walks her dog, there are fewer grammatical dependencies for speakers to keep track of. A complex sentence that is coherent and grammatical, therefore, might place greater demands on phonological working memory because the speaker must be aware of even more grammatical and semantic dependencies. The speaker’s working memory capacity might confine how many of these dependencies they can maintain and track. Poorer phonological working memory may limit a speaker’s ability to keep track of these dependencies and so, when they do produce a grammatical, coherent sentence, the sentence itself may be less complex. Logically, there are many reasons to expect a link between these sentence-level measures and phonological working memory.
Working Memory in Children with Language-Based Disorders
The current study includes participants with typical language, dyslexia, or comorbid dyslexia and DLD. Children from these groups represent a wide range of working memory and spoken language abilities. There is substantial evidence that as a group, children with dyslexia-only and those with comorbid dyslexia and DLD demonstrate poorer phonological working memory skills than their typically developing peers. These deficits have been found when measured using digit span and nonword repetition tasks (dyslexia-only: Carvalho, Kida, Capellini & Avila, Reference Carvalho, Kida, Capellini and Avila2014; Jeffries & Everatt, Reference Jeffries and Everatt2004; Schuchardt, Bockmann, Bornemann & Maehler, Reference Schuchardt, Bockmann, Bornemann and Maehler2013; comorbid dyslexia and DLD: Catts, Adlof, Hogan & Ellis Weismer, Reference Catts, Adlof, Hogan and Ellis Weismer2005; Fraser, Goswami & Conti-Ramsden, Reference Fraser, Goswami and Conti-Ramsden2010; Schuchardt et al., Reference Schuchardt, Bockmann, Bornemann and Maehler2013; however, there is evidence that group-level differences do not always represent differences at the individual level, Gray, Fox, Green, Alt, Hogan, Petscher & Cowan, Reference Gray, Fox, Green, Alt, Hogan, Petscher and Cowan2019).
Dyslexia, though it primarily affects written language and has hallmark deficits with decoding and spelling (Adlof & Hogan, Reference Adlof and Hogan2018; Catts et al., Reference Catts, Adlof, Hogan and Ellis Weismer2005), has been linked to delayed spoken language development in childhood (for brief review, see Bishop & Snowling, Reference Bishop and Snowling2004) and syntactic and vocabulary-related deficits in particular (Alt, Hogan, Green, Gray, Cabbage & Cowan, Reference Alt, Hogan, Green, Gray, Cabbage and Cowan2017; Alt, Gray, Hogan, Schlesinger & Cowan, Reference Alt, Gray, Hogan, Schlesinger and Cowan2019; Altmann, Lombardino & Puranik, Reference Altmann, Lombardino and Puranik2008; Kida, Ávila & Capellini, Reference Kida, Ávila and Capellini2015; Rispens & Been, Reference Rispens and Been2007; Robertson & Joanisse, Reference Robertson and Joanisse2010; Wiseheart & Altmann, Reference Wiseheart and Altmann2018). Given these spoken language deficits and common phonological working memory deficits in this population, it is possible that the latter influences the former. DLD is associated with broad deficits in oral language in addition to difficulty with written language (e.g., reading comprehension). Hallmark deficits include difficulties with syntax and morphology (Bedore & Leonard, Reference Bedore and Leonard1998). Similar to dyslexia, vocabulary-related deficits are also present (Alt & Plante, Reference Alt and Plante2006; Gray, Reference Gray2003; McGregor, Oleson, Bahnsen & Duff, Reference McGregor, Oleson, Bahnsen and Duff2013). Children with comorbid dyslexia and DLD would be expected to show patterns of spoken language and phonological working memory deficits common to both disorders.
Including children from these different diagnostic groups was intended to provide a more holistic picture of the link between sentence-level language production and phonological working memory in school-age children. Children with these disorders are most likely to have sentence-level language production problems due to issues with either poor sentence formulation, poor working memory skills, or both – it is important to better understand this relationship in order to potentially improve their outcomes. Additionally, there was reason to wonder whether a correlation existed between phonological working memory and sentence production when children with varying degrees of phonological working memory and sentence production skills were included in the analysis. Children with dyslexia but not DLD, for example, were screened to have normal oral language but, as a group, tend to have poorer phonological working memory skills than their peers with typical language. Therefore, this screening process might eliminate any correlation between phonological working memory and sentence formulation.
The Current Study
In the current study, we aimed to explore the relationship between phonological working memory and novel sentence production in school-age children. We did this by analyzing performances on phonological working memory and sentence production tasks by children with typical language, dyslexia-only, or comorbid dyslexia and DLD (hereafter, “dyslexia+DLD”). Our phonological working memory measures included a Nonword Repetition, Digit Span, and Phonological Binding Span task that were completed as part of a larger working memory battery (Cabbage, Brinkley, Gray, Alt, Cowan, Green, Kuo & Hogan, Reference Cabbage, Brinkley, Gray, Alt, Cowan, Green, Kuo and Hogan2017; Gray et al., Reference Gray, Green, Alt, Hogan, Kuo, Brinkley and Cowan2017). We used children’s scaled scores from the Formulated Sentences subtest of the Clinical Evaluation of Language Fundamentals – Fourth Edition (Semel, Wiig & Secord, Reference Semel, Wiig and Secord2003) to measure their sentence production abilities. In this task, children must produce novel sentences; performance is therefore more generalizable to real world sentence production (as compared to a sentence repetition task) while allowing a level of experimental control that could not be achieved through language sampling. We analyzed sentences children produced on Formulated Sentences in terms of their length, lexical diversity, and complexity.
The specific goals of the study were twofold. First, we aimed to examine the correlation between children’s scores on phonological working memory tasks and a sentence production task that required formulation of novel sentences. Using this type of task would allow us to better generalize findings to the relationship between phonological working memory and real-world language production and better understand if the relation between phonological working memory and sentence production is as strong as the current – but scarce – extant evidence suggests (e.g., Torrens & Yagüe, Reference Torrens and Yagüe2018). We included children with typical language, dyslexia-only, and dyslexia+DLD in order to determine if a relationship between phonological working memory and sentence production skills would be observed when children with a wide range of language and phonological working memory abilities were included.
The second aim of our study was to extend Adams and Gathercole’s (Reference Adams and Gathercole1995) study with preschoolers to (a) school-age children and (b) to those with language-based disorders to determine if similar patterns could still be found with a more diverse sample. Examining the relationship between phonological working memory and sentence production in this older and more diverse population could help us understand the role that phonological working memory plays in sentence production at a later point in language development. Similar to Adams and Gathercole, we examined the length, lexical diversity, and complexity of children’s novel sentences relative to their performance on phonological working memory tasks. We matched children between each language group and conducted three between-group comparisons: typical language vs. dyslexia-only, typical language vs. dyslexia+DLD, and dyslexia-only vs. dyslexia+DLD. We compared groups on phonological working memory scores and sentence-level measures (i.e., length, lexical diversity, and complexity, measured from sentences produced in the Formulated Sentences task). Adams and Gathercole found that preschoolers with higher phonological working memory produced sentences that were longer, more lexically diverse, and more complex than preschoolers with lower phonological working memory. We aimed to determine whether a similar pattern is still present (a) later in childhood and (b) when children with different language diagnoses were included. Our research questions were:
-
1. How do children’s scores on phonological working memory tasks (i.e., Nonword Repetition, Digit Span, and Phonological Binding Span) correlate with their scaled scores on Formulated Sentences?
-
2. How do the groups with typical language, dyslexia-only, and dyslexia+DLD compare in terms of their scores on phonological working memory tasks and the length, lexical diversity, and complexity of grammatically correct sentences?
For our first research question, we predicted that children’s scores on the three phonological working memory tasks would correlate positively with their scaled scores on Formulated Sentences. This prediction is based on the idea of phonological working memory as a buffer for language output. Phonological working memory might influence how well a speaker can formulate a grammatically correct, coherent sentence; when an individual produces a sentence, they might use phonological working memory during the sentence formulation phase when they are retrieving or holding words in memory and putting words into a grammatical order to communicate an idea. Stronger phonological working memory abilities, therefore, could be expected to correlate positively with sentence production abilities, as measured by this Formulated Sentences task.
To answer the second research question, we conducted three between-group comparisons after matching pairs between each group and analyzed a subset of sentences unique to each pair. Specifically, we only analyzed sentences which both children in a matched pair produced grammatically correctly. We matched pairs across groups to eliminate potential artifacts resulting from the Formulated Sentences task. For example, some children completed later trials which obligate use of complex syntax while others did not complete those same trials. Examining all sentences all children produced during the task might, therefore, have erroneously influenced our sentence-level measures, such as complexity. We elaborate on this matching process and rationale in the Method section. We had three predictions for this research question, one for each between-group comparison, outlined below.
Typical language vs. dyslexia-only
Our first prediction was that the group with typical language would score higher on the phonological working memory tasks than the group with dyslexia-only, given the aforementioned evidence of phonological working memory deficits in the latter group. The other part of this prediction was that the group with typical language would produce sentences that were 1) longer, 2) more lexically diverse, and 3) more complex than the group with dyslexia-only. This prediction was based on the above discussion proposing a link between phonological working memory and these sentence-level measures.
Typical language vs. dyslexia+DLD
For our second group comparison, we predicted similar outcomes. We predicted that the group with typical language would score higher on the phonological working memory tasks than the group with dyslexia+DLD, given the evidence of phonological working memory deficits in this latter group. The other part of this prediction was that the group with typical language would produce sentences that were 1) longer, 2) more lexically diverse, and 3) more complex than the group with dyslexia+DLD. Again, this prediction was based on the earlier discussion proposing a link between phonological working memory and these sentence-level measures.
Dyslexia-only vs. dyslexia+DLD
Finally, we predicted that the groups with dyslexia-only and dyslexia+DLD would show no difference in performance on the phonological working memory tasks, based on evidence in the literature that both groups show deficits in this area. If groups were not expected to differ on phonological working memory, then they should not differ on the sentence-level measures, given the proposed link between these variables. We predicted that they would produce sentences that were comparable in terms of 1) length, 2) lexical diversity, and 3) complexity. This prediction may be surprising for a number of reasons. First, spoken language deficits are more of a hallmark deficit in DLD than dyslexia, leading some to automatically assume poorer sentence outcomes for the group with dyslexia+DLD compared to those with dyslexia-only. However, a dyslexia-only diagnosis does not preclude children from spoken language weaknesses (Alt et al., Reference Alt, Gray, Hogan, Schlesinger and Cowan2019; Altmann et al., Reference Altmann, Lombardino and Puranik2008; Bishop & Snowling, Reference Bishop and Snowling2004; Kida et al., Reference Kida, Ávila and Capellini2015). Additionally, although we would expect the group with DLD to produce more errors overall, we did not analyze sentences with errors. We only analyzed sentences that were grammatically correct (for rationale, see Method). Given our discussion of how phonological working memory might influence production of grammatically correct, coherent sentences, few differences were therefore expected between these groups. Lastly, it may be surprising because children who have similar weaknesses in underlying cognitive abilities, such as working memory, do not necessarily have the same behavioral manifestations of that deficit in their language (Bishop & Snowling, Reference Bishop and Snowling2004). However, we note that this prediction was motivated by a strong interpretation of the influence of phonological working memory on sentence production; this interpretation would suggest that phonological working memory may have a stronger influence on the sentence-level measures of interest compared to the presence or absence of DLD.
Method
Participants
Data used in the current study was collected as part of a larger project, Profiles of Working Memory and Word Learning for Educational ResearchFootnote 2, that investigated working memory and word learning in school-age children with and without language-based disorders (Gray et al., Reference Gray, Green, Alt, Hogan, Kuo, Brinkley and Cowan2017). Following IRB approval, participants were recruited from public and private charter schools in Arizona, Nebraska, and Massachusetts, where all second-grade children received consent packets for guardians to sign and return if they wanted their child to participate. Children were seven to nine years old and monolingual English speakers. Various diagnostic groups were recruited, including typical language, dyslexia, and comorbid dyslexia+DLD. All children were classified as either expected typical language, dyslexia, or comorbid dyslexia+DLD based on relevant standardized test scores. The Test of Word Reading Efficiency-Second Edition (TOWRE-2; Torgesen, Wagner & Rashotte, Reference Torgesen, Wagner and Rashotte2012) was used to classify expected dyslexia. The Clinical Evaluation of Language Fundamentals – Fourth Edition (CELF-4; Semel et al., Reference Semel, Wiig and Secord2003) was used to classify expected DLD. The Kaufman Assessment Battery for Children – Second Edition (KABC-II; Kaufman & Kaufman, Reference Kaufman and Kaufman2004) was administered as an exclusionary criterion for intellectual disability. The Goldman-Fristoe Test of Articulation – Second Edition (GFTA-2; Goldman & Fristoe, Reference Goldman and Fristoe2000) was administered as well, and children who fell below our cut-off were still included in the study if their errors were due to a single consonant (e.g., /ɹ/). Table 1 contains cut-score criteria for each group; although not all children had a clinical diagnosis, we will refer to the groups hereafter as typical language, dyslexia-only, and dyslexia+DLD. In addition to these standardized assessments, children had to pass hearing, visual acuity, and color blindness screenings and were excluded if they had other linguistic influences (e.g., speaking a second language), history of neuropsychiatric disorder, or prior special education services for other diagnoses (e.g., autism spectrum disorder).
Table 1. Qualifying group cut-scores on standardized assessments.
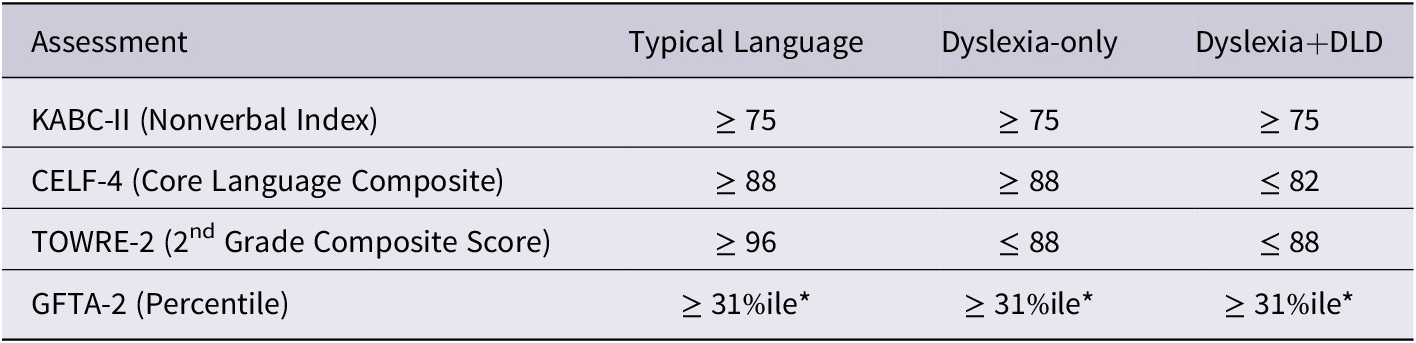
Note. DLD = developmental language disorder; KABC-II = Kaufman Assessment Battery for Children–Second Edition; CELF-4 = Clinical Evaluation of Language Fundamentals–Fourth Edition; TOWRE-2 = Test of Word Reading Efficiency–Second Edition; GFTA-2 = Goldman–Fristoe Test of Articulation–2.
* Children whose scores fell below the cut-score but whose errors were related to a single consonant (e.g., /ɹ/) were included in the study.
To answer our first research question, we used data from 289 children. Within this sample, 165 children had typical language, 81 dyslexia-only, and 43 dyslexia+DLD. Although there were children identified as having DLD-only in the larger sample, we omitted them because of their comparatively small group size (N = 9). Table 2 details group-level performance on standardized assessments. Table 3 contains demographic information for all participants and each diagnostic group.
Table 2. Group scores on standardized assessments.

Note. N = 289. DLD = developmental language disorder; KABC-II = Kaufman Assessment Battery for Children–Second Edition; CELF-4 = Clinical Evaluation of Language Fundamentals–Fourth Edition; TOWRE-2 = Test of Word Reading Efficiency–Second Edition; GFTA-2 = Goldman–Fristoe Test of Articulation–2.
Table 3. Participant demographic information.

Note. DLD = developmental language disorder.
To answer our second research question, we used data from a subset of participants whose Formulated Sentences audio files were accessible, which allowed us to analyze sentences for length, lexical diversity, and complexity. We created matched pairs of children from each group. Pairs were matched on age (+/- 6 months), sex, and maternal level of education (MLE; +/- 3 years) to control for group differences on our sentence production measures (e.g., lexical diversity) that may have been influenced by these factors. We were able to create 14 matched pairs of children with typical language and children with dyslexia-only, 12 matched pairs of children with typical language and children with dyslexia+DLD, and 10 matched pairs of children with dyslexia-only and children with dyslexia+DLD.
Tasks
Phonological Working Memory
As part of a larger study, participants completed the Comprehensive Assessment Battery for Children – Working Memory (Cabbage et al., Reference Cabbage, Brinkley, Gray, Alt, Cowan, Green, Kuo and Hogan2017), which consisted of a randomly ordered set of computerized working memory tasks. Data analyzed in the current study came from results of three tasks – Nonword Repetition, Digit Span, and Phonological Binding Span – that loaded significantly onto the phonological factor of a working memory model that was statistically derived from children’s performances across the entire working memory battery (Gray et al., Reference Gray, Green, Alt, Hogan, Kuo, Brinkley and Cowan2017). Although some working memory frameworks subdivide the phonological component into maintenance and manipulation or phonological and verbal working memory (e.g., Pierpont, Richmond, Abbeduto, Kover & Brown, Reference Pierpont, Richmond, Abbeduto, Kover and Brown2011), this model did not.
In the Nonword Repetition task, children listened to and verbally repeated nonwords that increased in length from 2 to 5 syllables. Each child heard 16 nonwords in total. Nonwords had no phonological neighbors and consisted of phoneme sequences that occur infrequently in English (Gray et al., Reference Gray, Green, Alt, Hogan, Kuo, Brinkley and Cowan2017). Scores were calculated by multiplying syllable length by the number of correct nonwords repeated at each span length. A nonword was considered correct if the child repeated it with all correct consonants. Products calculated at each span length were then summed (Cabbage et al., Reference Cabbage, Brinkley, Gray, Alt, Cowan, Green, Kuo and Hogan2017). Possible scores ranged from 0 to 56.
In the Digit Span task, children listened to and verbally repeated lists of one-syllable digits (1-9, excluding 7) that increased in length from 2 to 8 digits. Each child heard two lists per span length for a total of 14 lists. The order of digits within each list was randomized. Scores were calculated by multiplying span length by the number of correct trials per span length. Correct trials had zero errors. Products calculated at each span length were then summed (Cabbage et al., Reference Cabbage, Brinkley, Gray, Alt, Cowan, Green, Kuo and Hogan2017). Possible scores ranged from 0-70.
In the Phonological Binding Span task, children heard nonwords (consonant-vowel-consonant structure) paired with nonspeech sounds (e.g., beeps). After hearing all pairs in a trial, children heard one of the nonspeech sounds from the trial and were instructed to verbally reproduce its paired nonword. In the first stage, there were two trials, each containing 1 nonword-nonspeech pair. The number of nonword-nonspeech pairs presented within the trials increased incrementally with each stage: in the last stage, there were two trials, each containing 4 nonword-nonspeech pairs. In total, children were asked to recall 20 pairings. Scores were calculated by multiplying span length by the number of correct trials per span length. Correct trials had zero errors. Products calculated at each span length were then summed (Cabbage et al., Reference Cabbage, Brinkley, Gray, Alt, Cowan, Green, Kuo and Hogan2017). Possible scores ranged from 0-20.
Sentence Production
We used the Formulated Sentences subtest of the CELF-4 to measure sentence-level language production. In this task, children hear a target word they must use in a sentence to talk about a picture. There is a maximum of 24 trials, but administration may be stopped early based on children’s performance, which occurred for some participants. Each trial is scored based on the number of errors in the sentence produced. Error-free sentences receive a score of 2. Participants were audio-recorded during this task for offline scoring.
To answer our first research question, we used children’s Formulated Sentences scaled scores (x = 10, SD = 3). To answer our second question, we analyzed sentences that children produced on this subtest for sentence-level measures: length, lexical diversity, and complexity. However, we did not analyze and compare all sentences produced by all children because of possible task-related artifacts. Certain target words (e.g., “because”) obligate different sentence-level characteristics (e.g., subordinate clauses or prepositional phrases). Trials that obligated certain characteristics such as complex syntax were not completed by all children because administration was stopped early for some children, as allowed in the standardized administration of this task. Therefore, analyzing all sentences children produced might have artificially affected findings related to differences in children’s sentence-level measures.
To avoid these task-related artifacts, we created matched pairs based on individual variables discussed above (sex, age, maternal level of education) between each language group: typical language versus dyslexia-only; typical language versus dyslexia+DLD; and dyslexia-only versus dyslexia+DLD. We then identified the trials on which both children in a matched pair earned a score of 2. As a result, the exact trials we analyzed from each pair across groups were not the same. For example, the second matched pair of children from the typical language and dyslexia-only groups both scored a 2 on trials 1-5, 7, 8, and 11-14, and those sentences were analyzed. For the second matched pair from the typical language and dyslexia+DLD groups, trials 1-3, 5, 7, 9-11, 13, 15, and 17 were analyzed because both children in that matched pair scored a 2 on them. In total, 140 sentence pairs were included in the comparison between the groups with typical language versus dyslexia-only. For the comparison between the groups with typical language versus dyslexia+DLD, 94 sentences pairs were analyzed. For the comparison between the groups with dyslexia-only versus dyslexia+DLD, 66 sentences were analyzed. Some analyzed sentences came from the final third of the task, but for all group comparisons, the majority came from the first two thirds of the task. This is because earlier test items are easier than later items, and so children in a matched pair were more likely to both score a 2 on earlier items.
We chose to analyze sentences that scored 2 because these were error-free and analyzing sentences containing errors would have been problematic for the sentence-level measures we were interested in. For example, an ungrammatical run-on sentence would have inflated sentence length measurements. Our matching procedure placed matched pairs under the same semantic and syntactic constraints associated with target words to minimize the error in our analyses of group differences that might have been influenced by artifacts of the sentence production task itself. Once we had matched sentences within each matched pair, the sentences were transcribed. Appendix A contains our transcription rules. Transcribers were blind to children’s diagnoses. Sixteen percent of sentences were re-transcribed by another transcriber and used to calculate reliability between the transcribers. Inter-rater transcription reliability was strong (91.9% agreement).
After transcribing the sentences, we analyzed them in the research version of the computer program Systematic Analysis of Language Transcripts (SALT; Miller & Iglesias, Reference Miller and Iglesias2010). This program provided us with measurements of length (i.e., mean length of utterance in words) and lexical diversity (i.e., number of unique words). We analyzed sentence length based on the number of words rather than morphemes, as Adams and Gathercole (Reference Adams and Gathercole1995) had done, because the latter is only a valid measure for children whose average utterance is 4.5 morphemes or fewer (Blake, Quartaro & Onorati, Reference Blake, Quartaro and Onorati1993), and the children in our study were beyond this morphological stage. We measured complexity in terms of the number of 1) clauses and 2) prepositional phrases children produced per sentence. Though Adams and Gathercole used the Index of Productive Syntax (Scarborough, Reference Scarborough1990) as a measure of complexity, this measure has not been validated in school-aged children. We used number of clauses and number of prepositional phrases as separate measures of complexity in our analyses. Clauses and prepositional phrases were hand-counted by trained coders blind to children’s diagnoses. A main coder counted all the data. A subset of 20% of the data was recounted by a second coder (for instructions see Appendix B). Inter-rater reliability was 93.0% for clauses and 86.4% for prepositional phrases. Discrepancies were resolved by direct consultation with the coding instructions. All discrepancies occurred when the second coder had miscounted a single clause or prepositional phrase – therefore, no additional steps were taken to edit the main coder’s counts.
Statistical Analyses
We conducted Bayesian statistical tests for all analyses. Bayesian tests provide a Bayes factor that represents the likelihood odds of one hypothesis (or model) against others, including the null. In addition to providing evidence in favor of the null or alternative hypothesis, a Bayes factor also indicates the strength of the evidence. We opted against using traditional null hypothesis significance testing because it provides less insight than a Bayesian approach, only allowing us to accept or reject the null hypothesis. Furthermore, traditional statistical analyses are more sensitive to negative effects of small sample sizes compared to Bayesian approaches (Krypotos, Blanken, Arnaudova, Matzke & Beckers, Reference Krypotos, Blanken, Arnaudova, Matzke and Beckers2017).
Interpreting Bayesian Results
A Bayes factor of 1 indicates no evidence in favor of either the null or the alternative hypothesis. A Bayes factor above 1 indicates evidence in favor of the alternative hypothesis, and the larger it is, the stronger the evidence in favor of the alternative. It represents how much more likely the data are under the alternative than the null (e.g., a Bayes factor of 8 means the data are 8 times more likely under the alternative). A Bayes factor below 1 indicates evidence in favor of the null hypothesis, and the smaller it is, the stronger the evidence in favor of the null. It represents how much more likely the data are under the null than the alternative (e.g., a Bayes factor of .02, for example, means the data are 50 times – calculated as 1/.02 – more likely under the null). All analyses were conducted using the software JASP (JASP Team, 2018). We classified the effect sizes of the Bayes factors using the classification scheme in Wagenmakers et al., Reference Wagenmakers, Love, Marsman, Jamil, Ly, Verhagen, Selker, Gronau, Dropmann, Boutin, Meerhoff, Knight, Raj, van Kesteren, van Doorn, Smíra, Epskamp, Etz, Matzke, de Jong, den Bergh, Sarafoglou, Steingroever, Derks, Rouder and Morey2018).
Results
Phonological Working Memory and Formulated Sentences
Our first research question addressed how children’s scores on our phonological working memory tasks correlated with scaled scores on Formulated Sentences, a task requiring production of novel sentences. To answer it, we used the full data set (N = 289). Table 4 contains means, standard deviations, and ranges for Formulated Sentences scaled scores and phonological working memory task scores, both across all participants and within groups. We predicted that there would be a positive correlation (the alternative hypothesis).
Table 4. Group sentence production and phonological working memory task scores.

Note. DLD = developmental language disorder.
Initial visual inspection of the scatter plots of these data suggested possible group differences (see Figures 1-3). Therefore, we used group mean-centered data for this analysis, which removed between-group variance and isolated individual differences. In essence, this allowed us to examine the correlation between working memory and sentence production while controlling for between-group differences. Figures 4-6 show scatter plots of these data with trendlines corresponding to the Pearson’s r and Bayes factors in Table 5. For this Bayesian correlation, we used a default stretched beta prior of 1.0.

Figure 3. Scatter Plot of Raw Formulated Sentences Scaled Scores and Phonological Binding Span Scores

Figure 4. Scatter Plot and Trendline of Group Mean-Centered Formulated Sentences Scaled Scores and Nonword Repetition Scores

Figure 5. Scatter Plot and Trendline of Group Mean-Centered Formulated Sentences Scaled Scores and Digit Span Scores

Figure 6. Scatter Plot and Trendline of Group Mean-Centered Formulated Sentences Scaled Scores and Phonological Binding Span Scores
Table 5. Results of Bayesian correlation between Formulated Sentences scaled scores and the phonological working memory task scores.

Note. FS = Formulated Sentences. CI = Credible Interval.
Table 5 contains results of our Bayesian correlational analyses, including Pearson’s r values as well as Bayes factors, which indicate the strength of the evidence for the alternative hypothesis (i.e., a positive correlation). Pearson’s r values indicated positive but weak correlations between Formulated Sentences scaled scores and the individual working memory task. The Bayes factor from the analysis between Formulated Sentences and Nonword Repetition suggested strong evidence in favor of a positive correlation. From the analyses between a) Formulated Sentences scaled scores and Digit Span scores and b) Formulated Sentences scaled scores and Phonological Binding Span scores, the Bayes factors suggested only anecdotal evidence in favor of positive correlations.
Phonological Working Memory and Sentence-Level Measures
Our second research question addressed how groups with typical language, dyslexia-only, and dyslexia+DLD compared both in terms of scores on phonological working memory tasks and length, lexical diversity, and complexity of sentences produced on Formulated Sentences. We used Bayesian paired samples t tests to compare the sets of matched pairs from each language group (typical language vs. dyslexia-only, typical language vs. dyslexia+DLD, and dyslexia-only vs. dyslexia+DLD). For these analyses, we used the default prior Cauchy distribution of .707. A summary of our predicted and observed outcomes are in Table 6.
Table 6. Summary of predicted and observed outcomes for between-group comparisons.

Note. TD = typical language development. DLD = developmental language disorder. PWM = phonological working memory. Asterisks (*) indicate outcomes that differed from predictions.
Comparison One: Typical Language versus Dyslexia-Only
Results for the comparison between children with typical language vs. dyslexia-only are in Table 7. We predicted that the children with typical language would have higher scores on the phonological working memory tasks compared to their matched pairs with dyslexia-only (the alternative hypothesis). We found anecdotal to moderate evidence in favor of this prediction according to the Bayes factors. For the sentence-level measures, we predicted that children with typical language would produce sentences that were longer, more lexically diverse, and more complex than those produced by their matched pairs with dyslexia-only (the alternative hypothesis). We found no evidence for our prediction. Instead, the Bayes factors indicated moderate evidence for the null hypothesis, which was that children with typical language did not produce sentences that were more lexically diverse, longer, or more complex than sentences produced by their matched pairs with dyslexia-only.
Table 7. Results from one-tailed Bayesian paired samples t-test comparing children with typical language vs. dyslexia-only on phonological working memory scores and sentence-level measures.

Note. The alternative hypothesis is that scores for the group with typical language are greater than scores for the group with dyslexia-only. CI = Credible Interval.
Comparison Two: Typical Language versus Dyslexia+DLD
Results for the comparison between children with typical language vs. dyslexia+DLD are in Table 8. We predicted that the children with typical language would have higher scores on the phonological working memory tasks compared to their matched pairs with dyslexia+DLD (the alternative hypothesis). We found anecdotal to strong evidence in favor of this prediction according to the Bayes factors. For the sentence-level measures, we predicted that children with typical language would produce sentences that were longer, more lexically diverse, and more complex than those produced by their matched pairs with dyslexia+DLD (the alternative hypothesis). The Bayes factors indicated anecdotal to strong evidence in favor of this alternative hypothesis, which was that the matched pairs with typical language produced sentences that were longer, more lexically diverse, and more complex.
Table 8. Results from one-tailed Bayesian paired samples t-test comparing children with typical language vs. dyslexia+DLD on phonological working memory scores and sentence-level measures.

* Bayes factor > 10 indicates strong evidence for alternative hypothesis
Note. The alternative hypothesis is that scores for the group with typical language are greater than scores for the group with dyslexia+DLD. CI = Credible Interval.
Comparison Three: Dyslexia-Only versus Dyslexia+DLD
Results for the comparison between children with dyslexia-only vs. dyslexia+DLD are in Table 9. We predicted that there would be no difference in scores on the phonological working memory tasks between the children with dyslexia-only and their matched pairs with dyslexia+DLD (the null hypothesis). Bayes factors indicated anecdotal to moderate evidence in favor of this prediction. In terms of sentence-level measures, we predicted that there would be no differences between the matched pairs in the length, lexical diversity, and complexity of their sentences (the null hypothesis). Bayes factors indicated anecdotal evidence for this null hypothesis for one measure, the number of clauses, which was a measure of complexity. For all other sentence-level measures (mean length of utterance in words, number of different words, and number of prepositional phrases), Bayes factors indicated anecdotal to strong evidence in favor of the alternative hypothesis, which was that matched pairs from the two groups differed on these measures. Specifically, results showed that the children with dyslexia-only produced longer, more lexically diverse, and more complex sentences than their matched pairs with dyslexia+DLD.
Table 9. Results from two-tailed Bayesian paired samples t-test comparing children with dyslexia-only vs. dyslexia+DLD on phonological working memory scores and sentence-level measures.

* Bayes factor > 10 indicates strong evidence for alternative hypothesis
Note. The alternative hypothesis is that scores for the group with dyslexia-only and group with dyslexia+DLD are different. CI = Credible Interval.
Discussion
Phonological Working Memory and Formulated Sentences
The first goal of our study was to determine how children’s scores on three phonological working memory tasks correlated with their scores on a task of novel sentence production. By answering this question, we aimed to fill a gap in the literature on the relationship between these constructs in school-age children.
Our results indicated weak, positive correlations between children’s Formulated Sentences scaled scores and phonological working memory task scores. This finding suggests that phonological working memory and sentence formulation are not closely related in the school-age children. If phonological working memory does act as a language output buffer, it only minimally influences sentence production in this age group. By this time in childhood, language production may be more automatic, relying less heavily on phonological working memory in the way that preschool children’s language production might (Blake et al., Reference Blake, Austin, Cannon, Lisus and Vaughan1994).
Bayesian analyses revealed strong evidence in favor of the correlation between Nonword Repetition and Formulated Sentences, but only anecdotal evidence in favor of the other correlations. This pattern was somewhat surprising, given that all tasks loaded significantly onto a single phonological storage component of a novel, statistically-based model of working memory (see Combined Model in Gray et al., Reference Gray, Green, Alt, Hogan, Kuo, Brinkley and Cowan2017), and merits further discussion. In spite of their unified representation of a phonological component in the Combined Model of working memory, some may consider the demands of Nonword Repetition and Digit Span as tapping into simpler phonological short-term memory and Phonological Binding Span as tapping into more complex working memory. However, if this were the case, we would have expected more parallel outcomes between Nonword Repetition and Digit Span. A possible reason why Nonword Repetition stood out from the others is that it might have tapped into participants’ well-established mental phonological representations (Rispens & Baker, Reference Rispens and Baker2012). Because phonology is a core component of language, this task is arguably the most language-heavy of the three phonological memory tasks. This might have influenced the degree to which it correlated with the language-heavy Formulated Sentences task. The other two working memory tasks are arguably less language-heavy: Digit Span requires participants to maintain high frequency lexical entries that may be less linguistically burdensome, and Phonological Binding Span includes nonspeech sounds which do not tap directly into language. The difference in how heavily the working memory tasks tapped into language might explain the varying level of evidence for the correlations between them and Formulated Sentences. Future studies examining the relationship between working memory and sentence production should consider the linguistic nature of the working memory tasks and how the underlying linguistic demand might mediate the relationship. Another point is that if a nonword repetition task is used, the degree to which the nonwords reflect speakers’ native language should be considered (e.g., does it have phonological neighbors or phoneme sequences that frequently occur in a real language?). If the stimuli are dissimilar to one’s native language, then more pure conclusions could be made about the relationship between phonological working memory and sentence production. If the stimuli are similar to one’s native language, then one might have to take into consideration any mediating influence of the speaker’s linguistic knowledge (e.g., vocabulary) that might affect both the nonword repetition task and sentence production outcomes. Furthermore, studies could consider the degree to which tasks that are meant to tap phonological working memory tap specifically into phonology as opposed to a more general auditory working memory resource. It may be that tasks that are not purely phonological in nature and contain non-linguistic stimuli do not correlate as strongly with sentence production measures, which is consistent with what we saw with Phonological Binding Span.
There is another possible reason why evidence was strongest for the Nonword Repetition and Formulated Sentences correlation. Examination of the working memory model that these tasks were selected from (Gray et al., Reference Gray, Green, Alt, Hogan, Kuo, Brinkley and Cowan2017) reveals that Nonword Repetition had the strongest loading factor onto the phonological component. Because of this, we could expect that if there is a correlation between sentence production and phonological working memory, the strongest correlation would be found between a sentence production measure and the task that loaded most strongly onto the construct of phonological working memory. The other two tasks loaded less strongly onto the phonological component, which could explain why there was less evidence for their correlation with Formulated Sentences scores.
Few studies have examined the relationship between phonological working memory and sentence production in the school-age population of our current study, but these findings may have implications for which types of measures to use in future studies. Our rationale for using our three measures was because all three loaded significantly onto a phonological working memory factor in a statistically-based model of working memory (Gray et al., Reference Gray, Green, Alt, Hogan, Kuo, Brinkley and Cowan2017). It would not have made sense to include one but not another. Given that we had the strongest Bayesian evidence in favor of the Nonword Repetition task and sentence production, we suggest that future studies use similar nonword repetition tasks. There was simply insufficient Bayesian evidence in favor of the other correlations to warrant using those tasks in similar future studies. However, we note that future studies should acknowledge the linguistic nature of nonword repetition tasks and how that could influence the observed correlation between phonological working memory and sentence production. If multiple working memory measures are available, another suggestion for future studies is to further explore whether there is a difference in results between tasks that tap into simple phonological short-term memory, which some define as only requiring maintenance, versus those that tap complex phonological working memory, which some define as requiring maintenance and manipulation of information (Aben, Stapert & Blokland, Reference Aben, Stapert and Blokland2012). It is an open question whether sentence production abilities are more closely tied to one over the other; however, evidence is mixed whether these theoretically distinct concepts do in fact measure distinct constructs (Engel de Abreu, Conway & Gathercole, Reference Engel de Abreu, Conway and Gathercole2010; Hornung, Brunner, Reuter & Martin, Reference Hornung, Brunner, Reuter and Martin2011; Unsworth & Engle, Reference Unsworth and Engle2007).
Phonological Working Memory and Sentence-Level Measures
Adams and Gathercole (Reference Adams and Gathercole1995) found that preschool children with high phonological working memory produced sentences that were longer, more lexically diverse, and more complex than sentences produced by their peers with low phonological memory. The second goal of our study was to determine whether a similar pattern existed in older school-age children with and without language-based disorders.
We did find a similar pattern when comparing children with typical language vs. dyslexia+DLD, but not children with typical language vs. dyslexia-only or children with dyslexia-only vs. dyslexia+DLD. In summary, groups that differed in phonological working memory ability did not always differ in sentence-level measures, and groups that were similar in phonological working memory ability were not always similar in sentence-level measures. This pattern of results suggests that phonological working memory likely does not play an explanatory role in how long, lexically diverse, or complex school-age children’s sentences are, which contrasts with the strong interpretation of the influence of phonological working memory on sentence production we based our predictions on. The lack of evidence for an explanatory role of phonological working memory in two of our three comparisons might be because the relationship between phonological working memory and sentence production changes as children’s oral language systems mature. Specifically, as children develop more mature language systems, they might rely less on their phonological working memory system when formulating and producing sentences. Preschool-age children are in earlier stages of oral language development, and so they might rely more on their phonological working memory when producing sentences than older children with more mature language systems. Therefore, preschool-age children with poorer phonological working memory might show differences in their spoken language output relative to peers with better phonological working memory, which aligns Adams and Gathercole’s (Reference Adams and Gathercole1995) findings. As preschool-age children develop more mature language systems, they might rely less on phonological working memory during language production tasks, which may mitigate any negative influence of poor phonological working memory on sentence production.
Our comparison between school-age children with typical language vs. dyslexia-only revealed group differences, where the group with dyslexia-only as a whole showed deficits in phonological working memory relative to peers with typical language. However, there were no differences between these groups in the length, lexical diversity, or complexity of sentences. One interpretation of this finding is that even though children with dyslexia have phonological working memory deficits, these deficits do not influence the quality of their sentences. It is possible that they have strong enough oral language skills in general to compensate for any negative influence of phonological working memory on sentence production. Another interpretation of these results relates to a changing relationship between phonological working memory and sentence production as children’s language systems mature. It may be that children with dyslexia do rely more heavily on phonological working memory when producing sentences but only in earlier childhood. By the time they are school-age (i.e., 7- to 9-years-old), their oral language systems might have matured enough that they no longer rely as heavily on phonological working memory skills when producing language. As a result, deficits in phonological working memory may not influence their sentence-level language production, which is consistent with our findings.
Our findings for the dyslexia-only vs. dyslexia+DLD comparison did not follow the pattern observed by Adams and Gathercole (Reference Adams and Gathercole1995). We found no group differences in phonological working memory scores between children with dyslexia-only vs. dyslexia+DLD but did find group differences in sentence-level measures: children with dyslexia+DLD produced sentences that were shorter, less lexically diverse, and less complex. Perhaps the most parsimonious explanation is that the presence of DLD has such a strong influence on sentence production that it results in even poorer spoken language than is expected from the negative influence of phonological working memory alone. If phonological working memory had a stronger influence, then the groups would have been more similar in their sentence-level measures. Whether children who have DLD rely on their phonological working memory system or their oral language system, or both, neither is strong enough for them to meet the skill level of peers without DLD.
This group comparison also speaks to the complexities of the relationship between cognition and language and may allude to fundamental differences in dyslexia and DLD. As Bishop and Snowling (Reference Bishop and Snowling2004) note, similar underlying cognitive impairments do not always equate to similar profiles of language impairment. In other words, although dyslexia and DLD each typically present with phonological working memory deficits, these deficits could differentially impact their language profiles, with one impacting written language more and the other spoken language. This finding might also be explained by the maturation of the phonological working memory system and its possible changing role in sentence production in later childhood. School-age children with dyslexia-only might rely less on phonological working memory during sentence production by relying more fully on their more mature oral language systems. Despite still having weak phonological working memory systems, it no longer seems to affect their sentence-level language production. As for their peers with dyslexia+DLD, however, it may not simply be the presence of DLD that explains this group’s production of shorter, less lexically diverse, and less complex sentences. We speculate that children with dyslexia+DLD may experience a protracted reliance on phonological working memory during sentence production later in childhood, despite it being an area of weakness for many of them. This protracted reliance may help them compensate for or avoid full reliance on their weak oral language systems. Because of a continued dependence on a weak phonological working memory system, they may continue to experience its negative influence on their sentence production, resulting in them producing shorter, less lexically diverse, and less complex sentences.
The findings between the groups with dyslexia-only versus dyslexia+DLD are interesting in the context of past literature. Past studies on dyslexia have not consistently described whether participants have concomitant oral language disorder, like DLD. The reason is partially because there has not always been an agreed upon distinction between these disorders (for discussion, see Adlof & Hogan, Reference Adlof and Hogan2018); however, evidence does point to them as distinct (Catts et al., Reference Catts, Adlof, Hogan and Ellis Weismer2005). Our findings show how the two diagnoses might manifest differently: a child with dyslexia-only might exhibit poor phonological working memory while a child with dyslexia+DLD additionally would exhibit deficits in sentence processing. Our results imply that future studies examining dyslexia and language skills should determine whether participants also have DLD, as sentence processing skills may be affected by its presence.
In sum and in the context of Adams and Gathercole’s (Reference Adams and Gathercole1995) findings, we interpret the results of our between-group comparisons to suggest that both age and diagnosis may be factors influencing the relationship between phonological working memory and the length, lexical diversity, and complexity of sentences school-age children produce. The oral language systems of younger preschool-age children are still maturing and so we speculate that they may more heavily rely on phonological working memory during sentence production. Subsequently, at this young age, negative effects of poor phonological working memory may be more apparent on sentence production tasks. However, in later childhood, we may see a divide based on children’s diagnoses and whether they continue to depend on phonological working memory during sentence production or are able to compensate with stronger oral language skills. School-age children with dyslexia-only, despite continued difficulty with phonological working memory, may no longer rely as heavily on that system during sentence production tasks because they are able to rely on their mature oral language systems. Alternatively, their more developed oral language systems may be able to compensate for any difficulties with phonological working memory. In contrast, children with dyslexia+DLD, many of whom have difficulty with phonological working memory, may experience prolonged reliance on phonological working memory during sentence production due to them compensating for their weak oral language systems (relating to their DLD diagnosis). Thus, they may continue to experience the negative impact of a weak phonological working memory system on their sentence production. Alternatively, their relatively poor sentence production (even on correct responses) may simply be because of their poor oral language skills, which may outweigh any negative effect of phonological working memory, no matter their skill level. It will take additional work to determine if poor performance is, in fact, related to prolonged involvement of the phonological working memory system during sentence production in this group, or if it is related solely to their DLD diagnosis.
Limitations and Future Directions
One limitation of the current study is that the screening process might have worked against the correlation we tested between children’s scores on phonological working memory tasks and Formulated Sentences. Nearly one third of the participants in the correlational analysis had dyslexia. These children, as a group, tend to show deficits in phonological working memory. However, they were screened for normal oral language skills, and as a result, data from this group might have counteracted the expected positive correlation between phonological working memory and Formulated Sentences scores. A solution for this would be to use a sentence production measure that was not part of a diagnostic language assessment.
Some might consider another limitation to be the use of Formulated Sentences as our sentence production measure. The highly structured nature of this task might limit its external validity as a tool for measuring real-world sentence production compared to more naturalistic sentence elicitation tasks such as a conversational language sample. However, any sentence a child produces in any context is still a sentence, and we would argue that the structured nature of Formulated Sentences is one of its strengths. In a less structured language production task such as a conversational language sample, children could potentially talk about any number of different topics, which, depending on the child’s personal knowledge and interest, could influence several of our sentence-level measures of interest, such as lexical diversity. The structure of Formulated Sentences allowed us to place children under the same syntactic and semantic constraints, which helped reduce potential error related to our sentence-level measures.
Finally, although we had reason to match participants for our between-group comparisons, it is possible that the similarities and differences observed in our subset of analyzed sentences would have been different had we analyzed a larger set of sentences from each matched pair. It could be informative for future studies to replicate our analyses of phonological working memory and sentence production using a larger sample of sentences. Despite using a smaller subset of data, however, we do still have confidence in our results given that we used Bayesian approaches, which typically offset issues associated with smaller sample sizes.
The primary focus of this study was on the unidirectional relationship between phonological working memory and sentence production. Given the complex relationship between working memory and language (Archibald, Reference Archibald2018), however, exploration of a possible reciprocal relationship between the two constructs may be warranted. For example, Coady and Evans (Reference Coady and Evans2008) found that in early childhood nonword repetition is predicted by children’s vocabulary. Although vocabulary was not measured in the current study, it may be worthwhile for future studies to examine how differences in vocabulary knowledge affect not only nonword repetition but also sentence-level lexical diversity.
Several related areas remain unexplored. One area is the relationship between phonological working memory, sentence production, and literacy. It might be that children’s literacy ability affects their sentence production because written language exposes them to more complex syntax and advanced vocabulary. This relationship may be mediated by phonological working memory abilities. Although many children in the current study were classified as having dyslexia, all were second graders and therefore still at a stage of learning to read, as opposed to reading to learn. The focus of their reading stage was still largely decoding rather than gleaning meaning and getting exposure to complex syntactic structures. Therefore, the relationship between these three variables in our young school-age participants was likely minimal. However, it may be worthwhile to explore this relationship in children who are at a more advanced stage of reading.
Another area is phonological working memory in relation to discourse-level language production. The influence of working memory may be apparent at this level even across school-age children. In discourse, speakers must maintain topics and grammatical agreement (e.g., between an antecedent and subsequent pronoun referents) across an extended period of time to successfully convey a message, and weaknesses in phonological working memory might negatively impact their ability to do so. Another area is to examine how error patterns in spoken language relate to phonological working memory. In our study, we only analyzed error-free sentences, but individuals with poor working memory may be prone to more errors in general, or certain types of errors. For example, they might produce more errors in long sentences relative to short sentences, or they might produce noun-verb agreement errors more frequently in complex sentences (e.g., those containing relative clauses or prepositional phrases). Work involving manipulation of children’s working memory load indicates it can influence errors in spoken language (Adams & Cowan, 2020), but it is unknown whether individual differences in working memory affect sentence production errors in naturalistic settings.
The current study is the first of its kind, to our knowledge, to directly compare the length, lexical diversity, and complexity of sentences produced by school-age children to their performance on phonological working memory tasks. Our results serve to provide preliminary evidence regarding the relation between these variables in this age group and reveal that the nature of the relation may not be precisely what the literature leads us to believe.
Acknowledgements
Funding for this work came from the National Institutes of Health NIDCD Grant #R01 DC010784. Preliminary findings associated with this manuscript were presented at the Symposium on Research in Child Language Disorders. We extend our deepest appreciation to the staff, research associates, school administrators, teachers, children, and families who participated in this research. Key personnel included (in alphabetical order) Shara Brinkley, Gary Carstensen, Cecilia Figueroa, Karen Guilmette, Trudo Kuo, Biorg LeSueur, Annelise Pesch, and Jean Zimmer. Many thanks also to the numerous students who helped with this work including (in alphabetical order) Genesis Arizmendi, Lauren Baron, Alexander Brown, Nora Schlesinger, Nisha Talanki, Hui-Chun Yang, and Atha Zimmermann.
Conflict of Interest
We have no known conflicts of interest to disclose.
APPENDIX A – SENTENCE TRANSCRIPTION RULES
-
1. Type the first sentence that the child says using each target word.
-
2. Note: The child’s first two sentences in the audio file may be the practice sentences. The target words for these are “playing” and “when.” Do not type these sentences.
-
3. Specific rules to follow when typing sentences:
-
a. Type the child’s morphology exactly as it is produced.
-
i. Do not change what the child says.
-
ii. For example, if the child says “dogses,” type “dogses” and not “dogs.”
-
iii. For example, if the child says “They goed,” type “they goed” and not “they went,” “they go,” or any other corrected variant.
-
-
b. Do not include false starts, rephrases, fillers, or mazes.
-
i. These types of words are whole- or part-words that a child produces that are not part of the intended utterance. However, if the child restarts the entire sentence and says a revised version, then only type the second sentence (this is elaborated below).
-
ii. If the child says, “The the chil- children were playing with the bas- I mean were um playing,” then the transcriber types, “The children were playing.”
-
-
c. Use X to represent unintelligible words or phrases.
-
i. For example, the beginning and end of the sentence were intelligible but not a word or words in the middle, the transcribed sentence might look like this: “The children are playing X on the floor.”
-
-
d. Only type the sentence containing the target word. If the child says two different sentences for a single picture stimulus and both sentences contain the target word, only type up the first sentence containing the target word. However, if it the child signals that she is rephrasing the entire first sentence and intended the second sentence to be the final answer, only type that second sentence. The child might signal this by saying “Oh wait, I mean” and starting a second sentence, or by signaling a restart with prosody.
-
i. Note: they have to have rephrased an entire sentence; if they only rephrased part of a sentence, include that partial sentence in parentheses (see section above on false starts, rephrases, fillers, and mazes).
-
ii. Example 1: if the child says, “I see them. The children are playing a game,” type “The children are playing a game.”
-
iii. Example 2: if the child says, “The children are playing a game. The father is not. Only the children are,” type “The children are playing a game.”
-
-
e. Run-on sentences: If the child produces a run-on sentence (i.e., the child keeps adding to the sentence using words like “and” and “but” but does not have a natural pause or end point at these boundaries), keep typing until the child produces a natural pause or intonation marker that signals the end of the sentence.
-
For example, if the child says, “She didn’t know if she wanted strawberries or carrots, but she made a decision quickly and grabbed both of them….And then she walked to the cashier,” and there is a substantial pause between “them” and “and,” then only type “She didn’t know if she wanted strawberries or carrots, but she made a decision quickly.”
APPENDIX B – COUNTING NUMBER OF CLAUSES AND PREPOSITIONAL PHRASES
Instructions for Counting Number of Clauses per Sentence
-
A. Each independent and dependent (e.g., subordinate clause) clause counts as one clause. Dependent clauses can range from adverbial clauses (e.g., “before he left for soccer”) to relative clauses (e.g., “the girl that is sitting on the floor”) to wh- clauses (e.g., “how it happened,” “where they went”).
-
B. To be counted as a clause, the utterance must have its own explicitly stated subject and its own finite/conjugated verb.
-
a. I saw the dog that caught the ball. = 2 clauses
-
b. The children are playing and the dad is watching. = 2 clauses
-
c. Before he played soccer, he ate lunch. = 2 clauses
-
d. My mom wanted to drive her car when she picked me up. = 2 clauses
-
-
C. What not to count as a separate clause: gerund phrases, infinitival phrases, past participles, a second verb without a new subject (i.e., phrases without finite/conjugated verbs).
-
a. I saw children playing video games. = 1 clause
-
b. The children are playing games and sitting on the floor. = 1 clause
-
c. Before playing soccer, he ate lunch. = 1 clause
-
d. My mom wanted me to go home. = 1 clause
-
e. I walked to the car parked outside. = 1 clause
-
-
D. Note: for compound sentences that have one subject and multiple verbs for that subject, count as one clause. For example:
-
a. She was running and racing against her friends. = 1 clause (because there is only one subject, despite there being two verbs)
-
Instructions for counting Number of Prepositional Phrases per Sentence
-
E. For a list of common prepositions, see https://webapps.towson.edu/ows/prepositions.aspx.
-
F. A single prepositional phrase contains a preposition followed by a noun/pronoun (note: this includes gerund) without any finite/conjugated verb.
-
G. She talked about it. = 1 prepositional phrase
-
H. They climbed up the stairs inside the church. = 2 prepositional phrases
-
I. On top of the tree the girl placed the star with five points. = 3 prepositional phrases.
-
J. Before going to soccer, I finished my lunch. = 2 prepositional phrases
-
K. Note: there are other grammatical forms that look similar to prepositional phrases. For example:
-
L. Prepositional phrase versus adverb . In order to be considered a prepositional phrase, the preposition itself must be followed by a noun or pronoun. If it is not followed by a noun/pronoun, it is an adverb.
-
a. Example with “inside”:
-
i. As prepositional phrase: “The boys went inside the house.”
-
ii. As adverb: “Jessica went inside.”
-
-
b. Example with “over”:
-
i. As prepositional phrase: “The family drove over the bridge.”
-
ii. As adverb: “The game was over.”
-
-
c. Example with “by”:
-
i. As prepositional phrase: “Stephanie traveled by airplane.”
-
ii. As adverb: “Stephanie walked by.”
-
-
-
M. Preposition “to” versus infinitival “to.” If the word “to” precedes a verb, then it is an infinitival “to,” and the phrase will not be counted as a prepositional phrase. The word “to” must be followed by a noun in order to constitute a prepositional phrase.
-
a. Prepositional phrase with “to”: “Tracy went to the game.”
-
b. Infinitival phrase with “to”: “Rick wanted to go.”
-
c. Infinitival phrase with “to” followed by prepositional phrase with “to”: “Joseph liked to drive to work.”
-
-
N. Preposition versus conjunction . This distinction might occur with words such as after, before, and until. While a preposition is only followed by a noun or pronoun, a conjunction is followed by a dependent clause. A clause contains both a subject and a verb, whereas a prepositional phrase does not: it only contains the preposition and a noun/pronoun.
-
a. Example with “after”:
-
i. As prepositional phrase: “Cosmo ate after five.”
-
ii. As conjunction: “Cosmo ate after he went outside.”
-
-
b. Example with “before”:
-
i. As prepositional phrase: “Anita said ‘Cheers!’ before everyone.”
-
ii. As conjunction: “Before she said ‘Cheers!,’ Anita raised her glass.”
-
-
-
O. Preposition versus particle . A particle is a function word just like the prepositions listed above, except it is part of the verb. A particle attaches to the verb rather than being a separate entity.
-
a. Example with “up”:
-
i. As prepositional phrase: “She walked up the stairs.”
-
ii. As particle: “She woke up.”
-
-
b. Example with “down”:
-
i. As prepositional phrase: “He fell down the stairs.”
-
ii. As particle: “He wrote down his name” or “He wrote his name down.”
-
-
c. Example with “on”
-
i. As prepositional phrase: “Penelope put a hat on her head.”
-
ii. As particle: “Penelope put her hat on” or “Penelope put on her hat.”
-
-
d. Note: One test to determine whether a word is a prepositional versus a particle is to move it around before and after the direct object of the sentence. If the word can move around and the sentence still makes sense, it is most likely a particle.
-
i. For example:
-
1. The sentences “Derek wrote down his name” and “Derek wrote his name down” are both acceptable, so “down” is a particle.
-
-
-
In the sentences “Kelly climbed down the stairs” and “Kelly climbed the stairs down,” only the first is grammatical. The second sentence is not. Therefore, “down” is a preposition in this case.