1. Model Risk: Daring to Open the Black Box
1.1.1. The Model Risk Working Party’s Phase 1 paper, Model Risk: Daring to Open the Black Box, was well received and was awarded the Institute and Faculty of Actuaries (IFoA) Peter Clark prize for best paper, 2015. As a result a Phase 2 of the working party was established to take forward some of the ideas presented in Phase 1 and these are presented in this paper. We begin by summarising the main themes from the Phase 1 paper including the core notion of a Model Risk Management Framework.
1.1.2. First, we recap the definition of a model. In the words of the Board of Governors of the Federal Reserve System (2011):
1.1.3. [T]he term model refers to a quantitative method, system, or approach that applies statistical, economic, financial, or mathematical theories, techniques, and assumptions to process input data into quantitative estimates. A model consists of three components: an information input component, which delivers assumptions and data to the model; a processing component, which transforms inputs into estimates; and a reporting component, which translates the estimates into useful business information.
1.1.4. The concept of model risk is therefore twofold:
-
∙ models may have fundamental errors and may produce inaccurate outputs when viewed against the design objectives and intended business uses; and
-
∙ a model may be used incorrectly or inappropriately.
1.1.5. The Phase I paper summarised a number of high-profile examples of model error that serve as salutary case studies, including the well-documented Long-Term Capital Management Hedge Fund collapse (1997), the bidding for the West Coast Rail Franchise (2012) and the JP Morgan “London whale” trading event (2012).
1.1.6. We highlighted that model risk is not as well defined and established as other more traditional risks, so the identification, understanding and communication of model risk is crucial.
1.1.7. The paper proposed a Model Risk Management Framework consisting of a cycle as shown in Figure 1 – and elements of this are explored further in this paper. Such a framework should be applied to those models which are most business-critical for the purposes of decision-making, financial reporting, etc.

Figure 1 The Model Risk Management Framework
1.1.8. Each part of the Model Risk Management Framework was explored in some detail and suggestions made as to how such a framework could have prevented or mitigated some of the case studies documented. It is worth recalling here the main features of each part of the framework.
1.1.9. Overall model risk governance: In order to put in place appropriate governance around model risk, an organisation should establish an overarching Model Risk Policy which sets out the roles and responsibilities of the various stakeholders in the model risk management process, accompanied by more detailed modelling standards which set out specific requirements for the development, validation and use of models.
1.1.10. Model risk appetite: The Board’s appetite for model risk needs to be defined and articulated into a risk appetite statement. Specifically, the Board has to establish the extent of its willingness, or otherwise, to accept results from complex models, and its tolerance for accuracy around the results from these models. As with any risk, the risk appetite for model risk should be articulated in the form of appetite statements or risk tolerances, translated into specific metrics with associated limits for the extent of model risk the Board is prepared to take. Examples of metrics that could be considered in a model risk appetite statement are:
-
∙ Extent to which all models have been identified and risk assessed; extent to which models are compliant with Standards applicable to their materiality rating; number of high residual risk models; number of high risk limitations/findings; duration of outstanding or overdue remediation activities; key person dependencies around high materiality models.
-
∙ The company’s position against the model risk appetite should be monitored by the individual or body responsible for the risk management of models on a regular basis, and should allow management to identify where actions are needed to restore positions within risk limits.
1.1.11. Model risk identification: We need to identify the model risks to which the company is exposed. In order to do this, it is necessary to identify all existing models and key model changes or new developments. For existing models, an inventory should be created in which each team or department lists all models in use. All models fitting the definition in section 1.1.2 should be considered. Models should be listed by usage/purpose, in order to ensure consistency in approach and a pragmatic usable inventory. The data collected on each model should be sufficiently detailed to allow a risk rating to be determined for each model and hence the extent to which the Model Risk Management Framework needs to be applied.
1.1.12. Model risk filtering: The model risk identification step will likely identify a large number of models and model developments in an organisation. A materiality filter should therefore be applied (in line with the firm’s model risk appetite) to identify those models which present a material risk to the organisation as a whole and which need to be robustly managed.
1.1.13. Model risk assessment: Having identified the material models, the next step is to assess the extent of model risk for each material model or model development. This can be attempted as a quantitative or a qualitative assessment. For example, sensitivities to key assumptions, outcomes of model validations/audits, or where it cannot be evidenced that model components have been through a recognised testing process then the models and output will generally be accepted as more risky.
1.1.14. Model risk mitigation: As a result of monitoring, the firm should know whether it is within or outside its model risk appetite. If outside then relevant actions to bring the company back into its appetite within an appropriate timeframe should be proposed. For example, model changes to remediate known material issues; additional model validation may be appropriate; an overlay of expert judgement should be applied to the model output to address the uncertainty inherent in the model; applying additional prudence to model assumptions; or explicitly holding additional operational risk capital.
1.1.15. Model risk monitoring and reporting: Model risk management information (MI) presented to the Board should enable effective oversight of model risk. The MI should be set out in terms which are meaningful to the Board, should focus on the company’s material models, and should ideally be tailored to the cultures of the stakeholders on the Board and relevant sub-committees (see section 1.1.16). Example content might include: the organisation’s overall model risk profile compared with its agreed appetite; recommended actions to restore model risk profile back to within appetite; outcomes of key model validations highlighting any issues or areas of weakness; any emerging trends or risks with model risk whether within the organisation or from regulatory/industry developments.
1.1.16. Central to any model risk governance framework is the acceptance that different cultures and user perspectives co-exist within any organisation. We identified four prevalent types or “cultures” of users of models all with valid perspectives on model risk, as shown in Figure 2:
-
∙ confident model users believe that good decision-making can and should be driven by models;
-
∙ conscientious modellers are primarily concerned with the technical validity of a model;
-
∙ uncertainty avoiders view all risks that matter as ever-changing and interconnected and doubt that any model can truly be “fit for purpose”; and
-
∙ intuitive decision-makers make decisions based on instinct and just use models to justify their intuition.

Figure 2 Alternative perceptions of modelling and its uses
1.1.17. Recognising that the four perspectives above are all valid viewpoints, the paper argued that governance and controls to manage model risk often do not consider the different perspectives on the model that can exist in an organisation. Suggestions were made as to how to correct this. In particular, the inclusion of non-technical, commercially oriented perspectives in model governance is necessary, even though this might be uncomfortable for technical model reviewers.
1.1.18. The paper concluded by focussing on model risk measurement and made attempts to quantify model risk, where possible, in the areas of proxy modelling, longevity and financial planning models. Finally, parallels were found in non-financial models such as those used for environmental protection.
1.1.19. Phase 2: In response to feedback from Phase 1, the Working Party was sponsored to continue to develop thought leadership on the subject of model risk through a second phase, with the remit to focus specifically on expanding on the following areas:
-
∙ further case studies and their relevance to the actuarial world;
-
∙ model risk communication – internally to the Board and externally to regulators, investors and other third parties;
-
∙ how practically to implement a model risk management framework;
-
∙ a standard approach to model risk assessment/quantification;
-
∙ insights on good model risk management practices that can be learned from other industries; and
-
∙ application for actuaries working in various fields (Insurance, Pensions and Banking, etc.).
1.1.20. We therefore look to address each of these areas in this paper although we do not attempt to set out any explicit (new) quantitative evidence to support our thinking.
1.1.21. In particular, we present a number of further case studies where model risk has raised itself in the public consciousness (section 2). We then consider what effective communication around model risk to a company’s Board, the regulator and external stakeholders might look like, recognising that all have different perspectives and levels of understanding around the model risk that a company runs (section 3). We then present how to practically implement a model risk management framework (section4) by assigning key model roles and most effectively leveraging a central model inventory, and addressing specifically how third party software risks, model reviews and cultural challenges around models, can be practically managed. In addition, we propose a framework for model risk quantification based on different sources of data. In section 5 we consider lessons learned from other (non-financial) industries including Weather Forecasting and Software. In section 6 we present some practical applications of reducing model risk for actuaries working in various fields based on the authors’ own experiences, and finally in section 7 we conclude with some summary remarks.
2. Further Model Risk Case Studies
Following feedback from Phase 1, we provide further real world case studies of models “badly behaving”, helping bring to life sources of model risk and its management, and we highlight the relevant considerations for actuarial modelling.
We present three examples below: NASA’s loss of its Mars Climate Orbiter (MCO) in 1999; modelling of the Cumbria floods in 2015; and the seminal paper “Growth in a Time of Debt” that was used by policy-makers to promote an austerity agenda following the 2007–2008 financial crisis.
2.1. Loss of NASA’s MCO (1999)
2.1.1. Background: In 1998, NASA launched the MCO with the aim of collecting information to enable better understanding of the Martian climate. In September 1999, the space probe was “lost” (NASA, 2000b). The trajectory of the spacecraft had been incorrectly calculated, which meant that the spacecraft had actually been orbiting much closer to Mars than had been targeted causing the space probe to disintegrate in the planet’s atmosphere (NASA, 2009).
2.1.2. Detail: An investigation was conducted by the MCO Mishap Investigation Board to understand what had caused the error which lead to the destruction of the $125 million space probe. The initial report (NASA, 1999b) released following the investigation described the root cause and other significant factors that contributed to the space probe loss.
2.1.3. The root cause was the use of incorrect units in part of the navigation software. Thruster performance data had been provided by software produced by an external contractor, in English units of pound-seconds. This was contrary to the documentation in place – Software Interface Specification (SIS) – which detailed that the results should be supplied in metric units. The navigation team at NASA’s Jet Propulsion Laboratory mistook this data as being in the required metric units of Newton-seconds. This led to the errors in the spacecraft’s trajectory calculations.
2.1.4. The root cause, although important, was not deemed to be the sole factor causing the MCO loss. This was because “sufficient processes are usually in place on projects to catch these mistakes before they become critical to mission success. Unfortunately for MCO, the root cause was not caught by the processes in-place in the MCO project”.
2.1.5. Other contributing factors that “allowed this error to be born, and then let it linger and propagate to the point where it resulted in a major error in our understanding of the spacecraft’s path as it approached Mars” included:
-
∙ inadequate consideration of the entire mission and its post-launch operation as a total system;
-
∙ inadequate training;
-
∙ lack of complete end-to-end verification and validation of navigation software and related computer models;
-
∙ inadequate communication between the different teams; and
-
∙ absence of a fault-tree analysis process for determining “what could go wrong” during the mission.
2.1.6. In addition, a second report prepared by the MCO Mishap Investigation Board discussed lessons learned from the MCO failure as well as failures from other failed missions (NASA, 2000a).
2.1.7. One key theme that ran through the second report was the need for a shift in culture. The MCO mission had been created under NASA’s “Faster, Better, Cheaper” philosophy and did not “adequately instil a mission success culture that would shore up the risk introduced by these cuts”. It was felt that there had been too much emphasis placed on cost and schedule reduction. The graph below has been taken from page 11 of the second report. It highlights how the board felt that a balance needed to be struck against cost cutting and risk identification and management.

2.1.8. In addition, the second report detailed the Board’s proposal for a new alternative vision of “Mission Success First”. Under this vision it was intended that “all individuals should feel ownership and accountability, not only for their own work, but for the success of the entire mission”. It was intended that Risk “becomes the ‘fourth dimension’ of project management — treated equally as important as cost and schedule”.
2.1.9. Lessons NASA learnt from the MCO loss: The initial report outlined a number of recommendations including:
-
∙ the use of consistent units as well as audits for all data being transferred between teams;
-
∙ models should be validated and a comparison of different navigation methods considered;
-
∙ additional training and specific information should be provided which should include face-to-face meetings between teams. Team members should be trained in software processes but also in the use and the importance of following the documentation;
-
∙ the number of new and relatively inexperienced members should be balanced with the addition of more experienced personnel. Contingency plans should also be prepared for backing up key personnel for mission-critical functions;
-
∙ roles, responsibilities and accountabilities should be defined clearly;
-
∙ it should be stressed to staff that communication is critical and that team members should feel empowered to forcefully elevate any concerns;
-
∙ an increase in the amount of formal and informal face-to-face communications as well as a “routine forum for informal communication between all team members at the same time so everyone can hear what is happening (eg, a 15 minute stand-up tag-up meeting every morning)”. Co-locations of key project team members could also enable this;
-
∙ a “Mission Safety First” attitude should be adopted;
-
∙ independent peer reviews of mission critical events; and
-
∙ a more robust verification and validation process of the software development and testing. The Board recommended that a “system verification matrix for all project requirements” be developed which should be reviewed at all major reviews.
2.1.10. Considerations for actuarial models: The issues highlighted in this case study could arguably be just as easily found in the models used by a wide range of industries. The issues uncovered related to inadequate external data quality and validation as well as inappropriate model methodology and governance.
2.1.11. First, this case study highlights the need for care when dealing with models; the simplest of errors can have a detrimental impact. This can be thought to hold true for most models – inappropriate data are a common source of model risk.
2.1.12. It was undisputed that the root cause of the MCO loss was the human error of the navigation data not being converted into the correct units. However, as was mentioned throughout the Investigation Board’s reports, it was believed that this simple error itself was the not main issue. The main issue was the fact that the error had gone unnoticed despite a number of quality control processes being in place.
2.1.13. “Our inability to recognize and correct this simple error has had major implications”, said Dr Edward Stone, director of NASA’s Jet Propulsion Laboratory, NASA (1999a).
2.1.14. The key reasons behind this inability ran through the two findings reports as two key themes: an inappropriate culture and inadequate communication. These two key themes are applicable to most models and this case study raises a number of questions relating to model risk in other industries such as insurance.
2.1.15. As we have seen through work recently done in response to Solvency II, there is a vast amount of process documentation and data directories now in place to support model use. But can we ensure that such documentation is being used effectively and not just being ignored (similar to the treatment of the SIS in this case study)?
2.1.16. Interestingly, members of the operations navigation team did have concerns about the trajectory of the space probe before the spacecraft was lost. However, these concerns were not effectively communicated to the other teams. The Board found the operation navigation team to be “somewhat isolated” to other teams by “inadequate communication”. Are we using communication effectively as part of our model risk mitigation procedures?
2.1.17. Furthermore, the Faster, Better, Cheaper philosophy may arguably be a philosophy adopted naturally by many companies. Models are increasingly being put to greater use and increasingly being used to inform business decisions. This increase in model use is not always necessarily backed by an increase in resource. This case study emphasises the importance of ensuring that an appropriate culture and mind set is maintained despite cost and time constraints.
2.1.18. Finally, another key lesson to keep in mind is the risk of becoming over-comfortable and complacent when using models. Just before the loss of the MCO space probe, it was perceived that “Orbiting Mars is routine” (NASA, 2000b) since the navigation of such spacecraft had been carried out successfully for several decades. This led to insufficient focus on identifying and mitigating risks relating to spacecraft navigation. The following statement, a recommendation made by the Investigation Board, should be kept in mind:
2.1.19. “Personnel should question and challenge everything—even those things that have always worked”.
2.2. Cumbria Flooding (2015)
2.2.1. Background: Northwest England experienced record rainfall during 5–6 December 2015, claiming two lives and resulting in an estimated 5,200 flooded houses and £500 million of damage across Cumbria, the worst affected area.
2.2.2. Details: Over 5,000 homes were left flooded and 50,000+ left without power after Storm Desmond wreaked havoc in parts of the United Kingdom on 5 and 6 December 2015. Storm Desmond was an extra tropical cyclone and the fourth named storm of the 2015–2016 UK and Ireland windstorm season. Desmond directed a plume of moist air, known as an atmospheric river, in its wake, bringing in moist air from the Caribbean to the British Isles, and meaning that rainfall from Desmond was unusually heavy.
2.2.3. The UK Met Office says Honister in Cumbria received 341.4 mm (13.4 inches) of rain in the 24-hour period from 18:30 GMT on Friday 4 December to 18:30 GMT on Saturday 5 December. This was more than twice the average monthly rainfall for Cumbria in December and beat the previous UK record set at Seathwaite, also in Cumbria, of 316.4 mm (12.4inches) on 19 November 2009.
2.2.4. The devastation resulted in criticism of the government after multimillion-pound defences built following floods in Cumbria in 2005 failed to keep the deluge out from people’s homes. However, Environment Agency officials said the Cumbria flood defences did work, but no matter how substantial any defences are, “you can always get water levels higher than that, in which case it will go over the top”. The Met Office said Storm Desmond had more impact because the “exceptional” levels of rain fell on already saturated land.
2.2.5. On the other hand, Sandtable, a modelling consultancy, commented: “… investments in flood protection since the last major floodings in 2009 could not be expected to deal with something as unprecedented as 300 mm of rain within 24 hours because it is such a rare event (the monthly average rainfall for Cumbria in December is 146.1 mm). But it is a rare event that has happened three times in the last 10 years” (Sandtable, 2015).
2.2.6. Considerations for actuarial models: The above statements highlight that, in the wake of such a disaster, two alternative interpretations of events can be plausible: (a) that there was a failure of prediction, possibly due to modelling flaws; or (b) that there was no failure of prediction, but the event that occurred was so rare that it was reasonable that no precautions to fully mitigate it were in place.
2.2.7. In the context of extreme events, it is difficult to decide which of those two interpretations more closely reflects reality. Which interpretation is accepted as the dominant narrative of events matters, as it relates to apportioning of blame: to modellers (for not predicting the event) or to decision-makers (for not taking suitable precautions). The argument that it was reasonable to not be fully prepared for an event that did eventually materialise is not easily accepted by the public.
2.2.8. Furthermore, the difficulty of communicating the risk of extreme events is highlighted in this case study. Return periods (“1-in-200 years”) are easier to communicate than probabilities (“probability of 1-in-200 over the next year”). However, the use of return periods implies that the frequency of the phenomenon described is stable over time. For some things like earthquakes that may well be true, but for pretty much anything else, including financial markets and rainfall patterns (in the context of climate change), it most likely is not: the language we use is not neutral, it makes implicit epistemological assumptions.
2.2.9. Additionally, information expressed through return periods and probabilities may be ambiguous to the public, if the reference class of probability statements is not given, that is, the population “out of which” frequencies are evaluated (Gigerenzer & Edwards, Reference Gigerenzer and Edwards2003). For example, if weather patterns were stationary, a 1-in-200 year storm at a particular location would be expected to be exceeded with a probability of 1/200 in any given year. But there is a much higher probability of observing such an extreme storm at some location within a given territory. The more the relevant locations, the higher the frequency of observed “1-in-200 year” events.
2.3. Growth in a Time of Debt (2010)
2.3.1. “Growth in a Time of Debt”, by Harvard economists Reinhart & Rogoff (Reference Reinhart and Rogoff2010) has been a highly influential paper, often cited by policy-makers as justification for slashing public spending following the 2007–2008 financial crisis. The paper’s commonly cited claim is that economic growth slows dramatically when the size of a country’s debt rises above 90% of gross domestic product (GDP).
2.3.2. The key policy question the paper attempted to answer was: Is it better to let debt increase in the hope of stimulating economic growth to get out of a slump, or is it better to cut spending and raise taxes aggressively to get public debt under control?
2.3.3. The paper attracted a lot of interest, including from the economics department at the University of Massachusetts Amherst. Professors Michael Ash and Robert Pollin set a graduate student, Thomas Herndon, the task of picking an economics paper and seeing if he could replicate the results, framed as a good exercise for aspiring researchers.
2.3.4. Herndon’s attempts to replicate the results proved unsuccessful. After Herndon contacted the authors, Reinhart and Rogoff provided him with the actual working spreadsheet they had used to obtain their results. Herndon discovered a number of issues, including:
-
∙ the authors had accidentally only included 15 of the 20 countries under analysis in their key calculation (having excluded Australia, Austria, Belgium, Canada and Denmark);
-
∙ for some countries, some data were missing altogether; and
-
∙ the methodology to average out performance of countries of different sizes was called into question. For example, 1 bad year for New Zealand, was weighted equally with the United Kingdom, a more global economy with nearly 20 years of high public debt.
2.3.5. After correcting for the above issues, the basic conclusion that countries with indebtedness rates above 90% of GDP have lower growth rates still held, but the most spectacular results disappeared, the relationship was much gentler and there were numerous exceptions to the rule (Herndon et al., Reference Herndon, Ash and Pollin2014). These findings substantially weaken the role of Reinhart and Rogoff’s (Reference Reinhart and Rogoff2010) contribution to arguments in favour of adopting of austerity policies in countries with various levels of public debt.
2.3.6. Considerations for actuarial models: The errors in the original paper by Reinhardt and Rogoff could be classified as rather major “blunders”, which should have been discoverable even by an elementary spreadsheet check. So the question of relevance is less how these errors were made, but more how they found their way into the final paper.
2.3.7. We note that Reinhart and Rogoff have made consistently the case for controlling public debt, both before and after publication of their 2010 paper (Cassidy, Reference Cassidy2013). While Reinhart and Rogoff admitted (to an extent) to errors in the original paper, they were quite clear that their views of the related policy issues have not changed. This indicates that the results of the 2010 paper were in line with a wider set of beliefs held by the authors.
2.3.8. The importance of this is illustrated by a counterfactual. Let us assume that the spreadsheet errors had been such that no result was found that supports the thesis of high public debt being associated with low growth. In that case, we can reasonably speculate that the researchers would have been surprised by the findings and may have actively looked for (and eventually discovered) the spreadsheet errors.
2.3.9. This demonstrates the wider point that model checking and validation can be heavily influenced by prior beliefs and biases. As a result, model errors that produce results confirming prior beliefs are less likely to be discovered. Since such beliefs are often not specific to individuals, but widely shared across expert groups and markets, we can see confirmation bias as a potential generator of systemic model risk.
2.3.10. Furthermore, central to this case study is the reproducibility of model results and the openness that Reinhart and Rogoff demonstrated in sharing their spreadsheets with the Amherst researchers. It is exactly this transparency, common in some (though not all) areas of academic research, that allowed the errors to be discovered. Such transparency is not easily attainable for many models deployed within the financial industry. Consequently, one can only speculate as to the number and impact of errors that sit undetected.
2.4. Summary
2.4.1. The three case studies presented in this chapter illustrate a number of important points that can be applied to actuarial models. From the Mars Orbiter loss we learn the value of a “challenge everything” culture and the importance of good and timely communication especially when applied to large modelling teams. The Cumbria flooding case study shows the difficulty of communicating extreme risk events to the public and defending models in light of these events. Finally, the “Growth in a Time of Debt” case shows us the importance of independent model reviews and of providing transparency around key assumptions and methodology.
3. Model Risk Communication
Since model risk is not as well defined and established as other more traditional risks, the identification, understanding and communication of model risk is crucial. We consider here how best to communicate to key internal stakeholders (the Board and Senior Management) and key external stakeholders (regulators and analysts/investors). As an example, a recent paper by the Lloyd’s Market Association (LMA) Exposure Management Working Group (2017) offers a structured way of explaining model risk in practical circumstances to a Board-level audience, in that case offering examples of catastrophe modellers working in the Lloyd’s market.
3.1. Internal Stakeholders
3.1.1. Overall responsibility for managing model risk must lie with the Board (or equivalent). This is because model risk events can impact the financial strength of the company and because the Board is ultimately responsible for the results and decisions of the organisation which are built upon, potentially, multiple layers of models.
3.1.2. It is therefore important that members of the Board, and where applicable the Risk and Audit Committees, are presented with clear, succinct information on model risk which enables them to understand how well model risk is being managed by the organisation and the key model risks of which they should be aware, as well as any actions that are being taken or proposed in order to restore model risk exposures to positions with which the Board is comfortable (within the Board’s risk appetite).
3.1.3. In particular, we would expect communications to these internal stakeholders to cover:
-
∙ any breaches of model risk appetite limits, and high-level commentary on the causes of the breach(es) and the path and timeline to return to within appetite;
-
∙ any key High risk model limitations or weaknesses in model risk governance, identified by the first (Model Owners), second (model reviewers) or third (internal audit) lines of defence, and how they may impact the respective results; and
-
∙ any key model risks associated with regulatory, market or internal developments.
3.1.4. For the next level down (e.g. for sub-committees or accountable individuals responsible for model risk management), more granular MI on model risk should be presented to enable the individual, or body, to manage all aspects of model risk. We would therefore expect communications to these internal stakeholders to cover:
-
∙ the organisation’s overall model risk profile compared with its agreed appetite;
-
∙ any proposed management actions to be taken where necessary to manage the company’s model risk within appetite;
-
∙ key model developments in progress or recently completed;
-
∙ outcomes of recent model validations, reviews or audits, highlighting any medium or high risk issues or areas of weakness identified;
-
∙ actions being taken by management to address these issues, along with associated timelines and progress to date;
-
∙ any breaches of Model Risk Policy or non-compliance with modelling standards, and associated timelines to remediate; and
-
∙ any emerging model risks, whether associated with regulatory, market or other internal developments.
3.1.5. In addition, specific deep-dives on material models may be appropriate, covering:
-
∙ scope and purpose of model;
-
∙ fitness for purpose of model;
-
∙ key model limitations/findings;
-
∙ key expert judgements/assumptions underlying the model, and sensitivities to these judgements;
-
∙ extent of review/challenge/validation of the model; and
-
∙ quality of data underlying the model.
3.1.6. This will allow the individual or body to more holistically understand the nature of and risks associated with each of the key models, and to be able to opine and challenge more robustly in order to effectively meet their responsibilities.
3.2. External Stakeholders
3.2.1. There are two key groups of external stakeholders to which to consider communicating on model risk – regulators and analysts/investors.
3.2.2. Communicating to external stakeholders brings challenges. Internal stakeholders are a known quantity, and will have an understanding of the background and context of the model risks of the business. Regulators will also have a degree of understanding of the context and of the topic of model risk and industry issues; however, other external audiences will have an unknown range of purposes, expertise and cultures.
3.2.3. Furthermore, given the level of challenge and the potential downside, the first consideration is whether we should communicate anything on model risk at all? After all, if the model has been through internal scrutiny it could be argued that the risk is sufficiently minimised, mitigated or managed.
3.2.4. However, without knowledge of the purpose of the recipient it is hard to make the call on their behalf that what is accepted internally as an acceptable level of risk is still acceptable to them, given their potentially different context and criteria.
3.2.5. This leads us on to explore further the types of recipient and the purposes for which an institution’s modelling may be important.
3.2.6. To whom are we communicating externally on model risk?: For any institution, in addition to regulators, investors are likely to be the primary external parties with which we are concerned. The security of financial institutions, such as banks and insurance companies, is inherently reliant on their balance sheets which, in turn, rely upon the veracity of the underlying models. When deciding whether to invest it is reasonable that a potential investor should have some knowledge of the reliance on particular modelling decisions. For example, should a different model turn out to have been more appropriate would this have made a significant difference to the investment decision or made very little change?
3.2.7. Investors may rely upon comment from other parties such as analysts, journalists or ratings agencies. For such comment and analysis to be informed and useful, particularly in carrying out comparisons between different organisations, it is important to understand whether the institution’s results are stable whatever model is used or whether they could vary significantly or contain some heroic assumptions.
3.2.8. Clients, customers, suppliers, etc. are also likely to all be concerned with the financial strength of an institution and the level to which this strength is built on firm foundations, or is impacted by modelling decisions.
3.2.9. There may also be other parties who are making decisions impacted by the model outcomes.
3.2.10. Thus the authors are of the view that it is necessary to convey a sense of the risk inherent in the modelling. However, detailed checks and tests carried out on the models, which may be relevant for internal or regulator communications, would not be possible or appropriate. Finding the balance is key.
3.2.11. What do we need to communicate externally on model risk?: When communicating externally it is generally not likely to be appropriate to use the same approach as for internal communications on model risk. There should be more focus on the company’s model risk management practices, and particularly for communications to investors/analysts we need to convey the information in a more succinct, less technical manner.
3.2.12. For regulators and investors, the key questions we expect they will be asking, particularly as model risk continues to grow in profile, are as follows:
-
∙ Does the firm have a well-understood definition of model risk? Does this have broader reach than just the modelling department?
-
∙ Does model risk have prominence with the Board? Is it a principal risk? Is it included in the Board’s risk appetite?
-
∙ Does consideration of model risk feed into decision-making in an appropriate way?
-
∙ Does the firm apply sufficient resources, tools and independence to modelling and the assessment of model risk? and
-
∙ Ultimately, how much reliance can be placed on the firm’s published results, and how much could these reasonably be over or understated?
3.2.13. We therefore expect that external communication on model risk should directly address these questions.
3.2.14. How should we communicate externally on model risk?: The Own Risk and Solvency Assessment or equivalent, the Annual Report and Accounts, the Solvency and Financial Condition Report and the Regular Supervisory Report, are the primary external documents. These contain information on the principal risks intrinsic in the business. Historically model risk was seen as one of many operational risks. However, we would argue model risk is wider than just the risk of accidentally using wrong parameters. As such, we would now expect to see specific consideration of model risk, which, at the least, would confirm that processes have been undergone to ensure the model is appropriate and applied correctly, and that this has been verified by senior responsible individuals other than the Model Owner.
3.2.15. For some institutions model risk will be sizeable enough to be a principal risk in its own right, for others it will remain a subset of another risk such as operational risk or governance risk, albeit with more prominence.
3.2.16. As the documentation gets more detailed so the granularity on model risk should follow. For example, a bondholder’s prospectus might be expected to contain more detail on model risk than the Annual Report and Accounts.
3.2.17. Why should we communicate externally on model risk?: The concern to date with communicating externally on model risk is that it could carry more downside risk than upside. Because we cannot properly communicate the uncertainty inherent in the models, does it give a false sense of security? Can it leave the Company unreasonably exposed when things go wrong?
3.2.18. However, this is not different from most other risk types, and ultimately good communication adds value and promotes confidence; particularly as model risk events become more prevalent in the media and because model risk relates directly to the results on which regulators and investors rely. This may give an institution competitive advantage. It also makes comparisons against others easier and more valid. And, ultimately, as it becomes industry standard practice to communicate on model risk management the recipients will start to expect the information.
3.2.19. Overall, it is the view of the authors that there is a need for more disclosure on model risk, both to regulators and publicly, as the profile and level of understanding of model risk as a risk type is increasing, as is the complexity and importance of actuarial models. It is also valuable to highlight the risk management practices in place around actuarial models as these are in general strong relative to model risk management in many other fields, due to disciplines instilled through a combination of actuarial standards and regulations such as Solvency II. However, there needs to be additional care when making disclosures around model risk to explain their context, given that precedents at this stage are still limited.
4. Practical Implementation of a Model Risk Management Framework
The concept of a Model Risk Management Framework was developed in the Phase 1 paper; subsequent feedback challenged how this can be implemented in a practical and proportionate manner. Appendix of this paper therefore sets out a full example Model Risk Policy to implement a Model Risk Management Framework; in this section we focus on specific key aspects of the framework which merit fuller explanation. There are similar remarks made in the recent Macpherson Report (HM Treasury, 2013) which we recommend to the reader. That paper reviews the quality assurance of UK Government analytical models and makes recommendations and best practice guidelines with the objective of ensuring all models are of sufficiently high quality, and that their end users – Ministers and, ultimately, the public – can place their trust in them.
4.1. Central Inventory of Core Models
4.1.1. As defined by the Federal Reserve in section 1.1.3, the term model refers to a quantitative method, system or approach that applies statistical, economic, financial or mathematical theories, techniques and assumptions to process input data into quantitative estimates of outcomes or behaviours which are used for a particular business purpose. Models typically rely on approximations, simplifications and judgements to represent a more complex reality.
4.1.2. This said, we recognise that any large business, especially in financial services, typically has a great number of “models” that are often much simpler and do not meet the definition above especially by involving little judgement. Such “calculator” models might include, for example, those used for data manipulation (data in are manipulated to data out by following robust and pre-defined rules) or well-defined validation checks which aggregate and summarise data for review.
4.1.3. Model risk for these models can be greatly reduced through appropriate processes and controls. For example: testing the code before release; version controlling the production versions of each models and ensuring staff only use the most up-to-date version; maintaining detailed documentation around each model from the perspective of both developers and users; analysis of the model results using rules-of-thumb; and checking integrity and reasonableness of model inputs and outputs.
4.1.4. For the smaller subset of models within an organisation that do genuinely meet the definition of section 1.1.3, the authors recommend the maintenance of a central inventory, maintained at either a department or business unit level, or at a global level across a company’s entire operation. The inventory should be kept up-to-date, for example, in sync with the reporting cycle, and for good practice might contain the following for each model:
-
∙ model’s name and version number ideally with a unique reference number;
-
∙ drive location;
-
∙ Model Owner/team responsible for model;
-
∙ a categorisation of the model (see section 4.6) into High/Medium/Basic control risk;
-
∙ when model was last reviewed and by whom;
-
∙ link to user documentation;
-
∙ link to model testing documentation;
-
∙ link to model specification documentation; and
-
∙ link to model methodology/appropriateness review notes and who conducted the review.
4.1.5. Such information might be time-consuming to obtain, but once a model inventory has been created maintenance of it should become a straightforward exercise. Moreover, the inventory will then continue to provide management with an at-a-glance view of all “live” models in use in their organisation, with key audit information, and ranked by risk materiality.
4.2. Assigning Key Model Roles
4.2.1. Once models have been identified, the most important step is to assign the key roles around each model. The need to put specific named people in these roles is heightened by the introduction in the United Kingdom of the Senior Insurance Managers’ Regime in March 2016 which requires named individuals to be accountable for key models (Table 1).
Table 1 Key Model Roles and Responsibilities
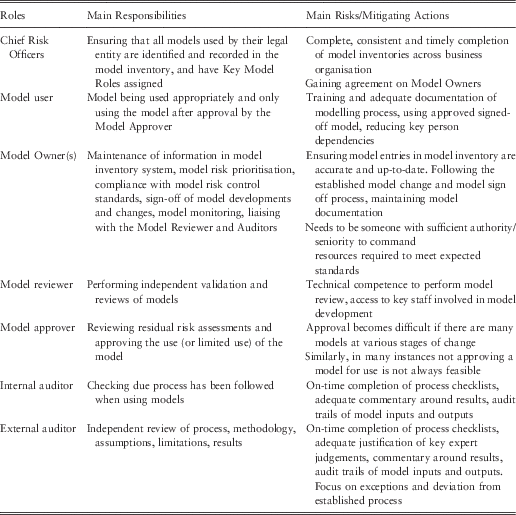
4.2.2. The Financial Conduct Authority and Prudential Regulation Authority (PRA) introduced the Senior Managers and Certification Regime (SMR) for banking and the Senior Insurance Managers Regime (SIMR) on 7 March 2016. There is a proposal to introduce this for all FSMA (Financial Services and Markets Act 2010) authorised firms during 2018. SMR/SIMR focusses on the most senior individuals in firms who hold key roles or have overall responsibility for whole areas of relevant firms and reinforces the importance of individual accountability at the most senior level of organisations (see https://www.fca.org.uk/firms/senior-managers-certification-regime).
4.2.3. As part of SMR, firms need to:
-
∙ ensure each Senior Manager has a Statement of Responsibilities setting out the areas for which they are personally accountable; and
-
∙ produce a Firm Responsibilities Map that knits these together.
4.2.4. We expect that any such Statement of Responsibilities must include those models within the business unit for which the Senior Manager has responsibility.
4.2.5. Furthermore, we would expect the Key Model Roles to potentially align to SMR/SIMR roles.
4.3. Third Party Software
4.3.1. Discussions in this paper so far have focussed on those models for which the user has sole responsibility, either as the model developer, or as the user of a model that has been developed in-house. However, many modelling suites are reliant on core software and models provided by third parties. For example, most UK life insurers use the same well-known provider for the economic scenario generators (ESGs) that drive their stochastic modelling. Similarly, software from external providers is frequently used to value derivatives and other exotic instruments for asset modelling, and for investment portfolio risk analysis/management.
4.3.2. The working party submits that a third party software provider is akin to “another team, in a separate room” – integral to the success of our business but working remotely from it. As such, all the standards that we hold our own modelling to must equally apply to any third party software that we use – or else we are explicitly acknowledging an unacceptable “weakest link” in our Model Risk Management Framework.
4.3.3. The following advice is given for management of all third party software:
-
∙ Ensure you have done enough due diligence. Is the third party software you are using really appropriate for the task and how have you gained comfort with this decision? A consensus view should be established before software is installed and incorporate into your modelling suite.
-
∙ Record all third party software alongside your regular models in your central inventory – with the same triage categories of High/Medium/Basic risk.
-
∙ Ensure that there are personnel in your organisation with sufficient knowledge to use and, where required, parametrise the software appropriately. Further, can you challenge the assumptions and methodology in sufficient depth that any limitations can be communicated as they would be with an in-house model?
-
∙ Keep your versions of third party software up-to-date unless there is good reason not to do so. This can often drive your in-house model development cycle, with software lifetimes and support being communicated months and years ahead. (As an example: 90% of UK National Health Service (NHS) Trusts are still using Windows XP despite Microsoft having withdrawn support for this version in April 2014 (Inquirer, 2016).) (This comment was made before the high-profile ransomware cyberattacks on the NHS and other global organisations in May 2017.)
4.3.4. Before a new version of any third party software is installed, ensure rigourous user acceptance testing (UAT) has been carried out. Are results expected to stay the same? If not, are they expected to change and do they by an acceptable amount. Record UAT tests and outcomes as you would for your own models.
4.3.5. Insist on sufficient and up-to-date user documentation. For technical models, ask for white papers to enable personnel to gain a solid understanding of what is “under the bonnet”.
4.4. Independent Review and Frequency
4.4.1. Models with a “High” control level, as defined in section 4.6, are likely to be ones which would have a large financial impact if they are materially “wrong” or used inappropriately, or which are complex and/or involve a significant amount of judgement in assumptions or methodology.
4.4.2. For these models, we recommend a systematic programme of independent review, as is now standard in the banking industry following the financial crisis. Throughout this paper, whenever we refer to validation, we mean independent validation by people who have no involvement in the design and operations of the particular model being validated. The frequency of review will be at management’s discretion but we suggest as a minimum each High risk model being reviewed at least once every 3 years on a rolling basis.
4.4.3. All reviews should be evidenced and recorded in the central inventory (see section 4.1.4) alongside the model being reviewed. We suggest reviews should cover the following:
-
∙ Model review date; the model and version being reviewed as shown in the inventory.
-
∙ Is it clear what is the purpose of the model, and has it been used for that purpose?
-
∙ Review of model user documentation to ensure its adequacy and a judgement made on whether it could be followed by a “technically competent third party”.
-
∙ Is there clarity around all model inputs and outputs, and the key judgements used in the model?
-
∙ Is there evidence of requirements and testing documentation, and recent model sign-off?
-
∙ Based on the above, are there any action points that should implemented and, if so, is there an agreed date for their completion?
4.4.4. It is our view that such review evidence, if maintained regularly and held alongside the central inventory, will serve to greatly reduce model risk around an organisation’s key High risk models.
4.5. The culture challenge
4.5.1. Developing, testing and running models, as well as using their outputs in decision-making, are complex endeavours that can involve many different participants across an organisation. As such, we must recognise that a company’s culture plays an important part in the ways model risk emerges and the ways it can be managed.
4.5.2. As reviewed in section 1, the Phase I Model Risk Working Party Report (Aggarwal et al., Reference Aggarwal, Beck, Cann, Ford, Georgescu, Morjaria, Smith, Taylor, Tsanakas, Witts and Ye2016) argued that successful model governance is reliant on representing and addressing the concerns of different professional cultures within the organisation, with potentially conflicting perceptions of models. Here we outline some of the practical challenges that model governance faces. They revolve around:
-
∙ opening up the model to a wider set of stakeholders;
-
∙ social pressures relating to the difficulty of expressing dissenting views; and
-
∙ balancing model change and innovation.
4.5.3. Opening up the model: The complexity of some models can lead to a lot of power resting in the hands of experienced developers and technical experts, with models seen as black boxes by other stakeholders. This means that there may be insufficient opportunity for the technical judgements made to be challenged by a wider set of experts, such as model users and the Board. An obvious risk is that substantial weaknesses in a model may remain unidentified.
4.5.4. Key person dependencies, especially if adequate technical and user model documentation are lacking, is a related source of risk.
4.5.5. The lack of opportunities to challenge and discuss a model’s structure, assumptions and output, can also prevent the building of confidence in the model. Model users often find it hard to trust the output of black boxes. As a result, the lack of wider challenge can lead to a very different type of risk: that a good model is insufficiently deployed and the insights it may provide are passed by.
4.5.6. The above risks can be mitigated by making a model’s methodology and key judgements as transparent as possible – with parallels to good and timely communication and collective accountability for models and their appropriate use, as described in the NASA satellite example in section 2.1.
4.5.7. In practice, it is not possible to “open” the full model specification for debate – apart from constraints on people’s time, some aspects may be too technical and others too uncontroversial to merit wider challenge. Therefore, it is important to decide what are the key judgements that need wider discussion. The Model Risk Triage discussed in section 4.6 can be a useful tool for that purpose.
4.5.8. Social pressures: Another issue arises when arguments highlighting the flaws or limitations of a model are not welcome within the organisation. For example, if regulatory approval of a model has substantial economic implications, arguments that may be seen to undermine the chances of such approval may be seen as damaging to the company’s interests.
4.5.9. Technical experts can experience conflicting incentives. On the one hand, in-depth understanding of their models means that they can be the people most capable of identifying limitations. On the other hand, technical experts often play a leading role in championing model use within their organisations. This means that technical experts may have an incentive to self-censor when communicating limitations of a model.
4.5.10. Model limitations often arise from deep uncertainties that cannot be easily quantified (let alone resolved) through statistical modelling. At the same time, model users and Boards can sometimes appear disinterested in hearing about these uncertainties. This may also relate to the inability of companies to operationalise such information. Risk quantification, as performed with the aid of actuarial models, routinely informs decisions. But there is typically no process for translating insights about the impact of uncertainty on model outputs into meaningful action.
4.5.11. Social pressures also manifest themselves in problems of group-think and herding. Most actuaries and other finance professionals follow very similar education and training paths. Furthermore, the dissemination of “best practices”, through formal and informal channels, means that the ways of approaching modelling problems can be very similar across professionals and companies.
4.5.12. This is compounded by the use of proprietary models, such as catastrophe models, ESGs, or investment portfolio risk models, and perceived external pressures towards conformity of modelling approaches across the market. The strength of this perception manifests itself in the marketing materials of model vendors. For example, a promotional brochure for Moody’s ESG contains the quotation: “If an insurer tells its regulator that it’s using the [Moody’s] ESG, that would probably mean only one meeting. If the insurer says that it’s going for a less widely used ESG that might mean seven meetings” (Moody’s Analytics, 2014).
4.5.13. There are systemic risk implications to the social pressures discussed above. First, if technical experts are wrong about a particular modelling aspect, they are likely to be wrong in the same way. Second, coordinated behaviour across the market, facilitated by the use of similar models can have an impact on market conditions and structure. Thus, the act of measuring risk changes the very nature of the risk being measured: systemic risk arises endogenously (Danielsson & Shin, Reference Danielsson and Shin2003).
4.5.14. It is not easy to mitigate such risks. At the organisational level, we would expect documented evidence of peer review of key judgements and methodology – with challenge – to be evidenced on a rolling basis, for example, by realising the independent review framework described in section 4.4. The broader challenge, not specific to model risk management, is to maintain a culture that encourages the expression of substantiated dissent and does not seek to suppress discomfiting views.
4.5.15. Addressing model risk at a market level is even harder and certainly beyond the reach of any individual company. We would hope that key stakeholders, such as regulators, do not provide incentives for further homogenisation of modelling approaches across the market.
4.5.16. Balancing model change and innovation: Insurance processes have to a great degree changed to meet Solvency II reporting timescales. This has also affected the modelling development lifecycle. To meet more rigourous control standards, models can now only be changed following an agreed and resourced development pipeline.
4.5.17. This sometimes conflicts with the urge of well-meaning developers, who, brought up in a culture of “Agile” development, might be tempted to proceed with what they see as small but necessary changes (“fixing a bug”), without going through a formal process. More broadly, the need to follow time-consuming processes for approving and reporting model changes can lead to disincentives for model improvement.
4.5.18. We counter that there must be scope in development plans to achieve the same outcomes of continual improvement, while making all model changes visible to all model users. If model risk management processes in practice undermine necessary model improvement, they cannot be judged successful.
4.6. Model Risk Assessment/Quantification
4.6.1. The model risk management effort should be proportionate to the risk a model poses. It is easy to warn against under-investment in model risk management, leaving a firm exposed to the risk of financial and reputational losses; on the flip side, it is also possible to over-invest in model risk controls, with benefits, in terms of reducing model risk, that are limited and/or hard to measure.
4.6.2. The aim of triage is to assess/quantify the risk associated with models, as well as material components of those models.
4.6.3. The materiality of model risk is a function of the uncertainty in the model (i.e. likelihood of error) and the resulting monetary/reputational impact (i.e. severity). Materiality can be assessed with various degrees of accuracy and effort depending on the level of data available. It is worth bearing in mind that part of the purpose of triage is to reduce the amount of effort for the less material models. Typical data sources are:
-
∙ Meta data – model attributes that are known before the model is run (e.g. purpose, methodology, number of developers, etc.);
-
∙ Scheduled run data – information from model runs already executed for a purpose different to model risk management; and
-
∙ Test run data – information from model runs executed specifically for the purpose of model risk management.
4.6.4. Meta data: In terms of availability and effort required, model meta data provide a reasonably straightforward way to determine the risk associated with a model. These model attributes should usually be accessible and should be stored as part of the central model inventory.
4.6.5. Good meta data act as proxies to the likelihood of model error. A non-exhaustive list of examples, categorised under each stage in a model lifecycle, is provided in Table 2.
Table 2 Meta Data as a Proxy for Likelihood of Model Error.

4.6.6. Each attribute and/or a combination of attributes can be scored based on pre-defined rules. An extreme example of a “High” control level model would be one that uses “cutting-edge” methodology and has only one in-house developer. The rules should ensure that the number of models in each control level classification is appropriate and aligns to available resource.
4.6.7. If a sizeable inventory of manually classified models (based on expert judgement) is available, supervised machine learning technique(s) can be used to formalise and/or check the classification rules. An illustrative classification (“High”, “Medium”, “Basic”) flowchart generated using the “Decision Tree” method is provided in Figure 3. Once trained and validated,Footnote 1 such a model can automate the classification of new or other models in the inventory.

Figure 3 Visualisation of model classification rules
4.6.8. Scheduled run data: Scheduled run data comes from model information available for a purpose different to model risk management, such as a business application. This typically includes input data and output data, perhaps under a variety of scenarios. Scheduled run data should be easy to access but it is not usually stored within the model inventory.
4.6.9. The periodical analysis of change carried out to attribute the movement in published income or balance sheet items (e.g. European Embedded Value, Solvency Capital Requirement (SCR), etc.) is an obvious source of scheduled run data. Movements due to “model restatements” or “out-of-model adjustments” would provide a monetary amount to quantify the materiality of model risk. A risk level classification can then be assigned based on pre-defined thresholds.
4.6.10. Quantitative triage techniques that rely only on other scheduled run data include:
-
∙ back-testing; and
-
∙ reverse sensitivity testing
4.6.11. Back-testing: In the context of predictive/forecast models, model output can be compared to the actual historical outcome; the divergence between the two provides an estimate of the likelihood of model error.
4.6.12. However, it should be noted that the past may not be representative of the future especially if the model is concerned with rare events. To complement the back-testing result, the model could be calibrated and tested against artificial data generated by known processes (see section 4.6.30 for “Ersatz model test”).
4.6.13. Reverse sensitivity testing: In models that use Monte-Carlo simulation, pseudo-random scenarios are generated from a number of risk factors and are subsequently fed into an aggregation function that may represent, for example, a portfolio structure. The output of the aggregation function consists of a large number of random scenarios pertaining to a variable of interest, for example, Net Asset Value. Repeated evaluations of the aggregation function for each scenario can be computationally expensive, which is the reason behind the long runtimes of Monte-Carlo models such as ones used in some SCR calculations.
4.6.14. The reverse sensitivity testing method (Pesenti et al., Reference Pesenti, Millossovich and Tsanakas2017) employs ideas from importance sampling, to re-weight scenarios in order to stress the distribution of inputs or outputs. Such re-weighting allows the exploration of the alternative model specifications from a scheduled model run, without the need to generate new scenarios and evaluate the aggregation function again.
4.6.15. As an example, consider a simplified insurance risk model with four input risk factors (X1, X2, X3 and X4). A re-weighting scheme is devised such that the 90%-value at risk of the output is scaled up by 20%. Distributions derived with those weights correspond to a stressed model. Figure 4 shows the percentile functions of the four risk factors, according to the baseline model (in black) and the stressed model (in red).Footnote 2

Figure 4 Visualisation of reverse sensitivity testing for an illustrative example
4.6.16. Where there is a substantial difference between the input distributions under the baseline and stressed models (as is the case here for the first and fourth risk factors), a high sensitivity to inputs is indicated. When there is also uncertainty around the distribution of those same inputs, a cause for concern can be flagged. By using statistical deviation measures to quantify such differences between distributions, the numbers of flags raised for a particular model can be used as a metric for model risk management.
4.6.17. Test run data: Test run data is model information created specifically for the purpose of model risk management. This data should be available as part of model implementation and testing.
4.6.18. Sensitivity tests requiring test runs are typically performed by varying a particular aspect of the model and analysing the resulting change in model output. Without employing more advanced methods, each sensitivity test requires a separate model test run. Sensitivity tests may be derived from varying estimated model parameters or changes in methodology.
4.6.19. Sensitivity to estimated parameters can provide a measure of the potential impact of statistical uncertainty on the model output. If changes in a parameter, for example, a volatility or a correlation coefficient, lead to substantial changes in output and if, additionally, the parameter is subject to high estimation error, then an area of model risk is indicated.
4.6.20. In practice, there are several challenges with the above approach:
-
∙ First, one needs to decide the extent to which parameters should be changed in sensitivity tests. This should reflect statistical uncertainty, for example, by setting parameters to their confidence limits.
-
∙ Second, one has to decide whether parameters will be varied one at a time or all at once. The latter approach may be too conservative, but reflects situations where parameters are set using consistent expert judgements.
-
∙ Third, it remains a challenge to derive clear conclusions from the data that such exercises produce. For example, many insurers observe that increasing correlations between risk factors can lead to large changes in SCR. This observation on its own does not indicate a meaningful course of model risk mitigation.
4.6.21. Where alternative methodological choices to those employed in a model are plausible, the impact of method changes on model outputs can also be tested. In some cases that is relatively straightforward to implement, for example, when changing the family of distribution for a risk factor (e.g. from Gamma to LogNormal). However, other methodological changes (e.g. a change in dependence structure or valuation method) are too time-consuming to implement for test purposes. One needs to remain mindful that the methodological choices made are often subject to contingent factors such as modelling legacy or software capabilities.
4.6.22. Types of error to test: When attempting to classify models according to their risks, it must be recognised that an attempt at modelling could fail for several different classes of reasons. A model could be rated as high risk at the triage stage for several different reasons.
4.6.23. Conceptually, the simplest type of model errors are the typographical errors, programming bugs or formula mistakes which should, in principle, be detectable by expert inspection of a model’s internal formulas. We refer to these human errors as blunders.
4.6.24. Next to these errors are those arising from various forms of statistical uncertainty. These include uncertainties in models and parameters because of data being limited. An example of this is the peso effect (which actuaries sometimes call Events not in Data), where a rare event such as peso devaluation is over-represented if it occurs in the analysed data and under-represented if it does not.
4.6.25. There are also errors associated with broader, non-statistical uncertainties, such as whether the data are accurate, whether favourable points have been cherry picked or arbitrary points have been censored.
4.6.26. A further source of errors can arise when some problem aspects are not captured either in the fitted model or in the reference model. For example, financial models may treat market prices as statistical processes unaffected by the decisions to be taken, when in reality a firm’s decision to buy or sell an asset might change the market price of that asset. Behaviour of customers or competitors may be described statistically when in fact a part of those behaviours is a response to a firm’s own strategy. Part of model triage should then consider whether a model has overlooked material feedback loops.
4.6.27. Ersatz Model tests: The idea of model triage is to classify models according to their level of risk while controlling the cost of performing the classification. Subsequent review and validation are then more intensive but applied only to the depth required by the triage stage.
4.6.28. As technology and model governance processes continue to develop, firms are able to automate more of what is currently classified as validation and review. This reduces the cost of those activities, potentially allowing some of them to fall in future within the triage stage.
4.6.29. The automation of model runs potentially allows models to be tested not just on data stressed in one direction, but rather on large numbers of randomly generated data sets. On each of those randomly generated datasets, model parameters are estimated and, subsequently, model outputs are evaluated. For this to work, the precise data input format needs to be specified, and also a reference method for generating the random data. The Ersatz test measures how well the model output replicates the reference process that generated the data, in a suitably defined average sense across multiple simulated data sets.
4.6.30. Ersatz tests are a straightforward way for detecting material model blunders. A model based on a stated set of assumptions should at least perform according to its specification if the reference method produces data confirming to those assumptions. Where even those tests fails, a logical or programming error is the likely culprit.
4.6.31. Ersatz tests can also give valuable insights into model limitations. They can highlight the characteristics of reference data sets which the model does and does not capture. Ersatz tests can also reveal the amount of statistical variability that can be expected in model output as a consequence of the finiteness of data. The materiality of both these sources of uncertainty can be factored into a triage process.
4.6.32. Some manual processes are more amenable than others to automation. A particular hurdle is automating human judgement. While a one-off instance of a model may reflect judgement applied to the actual data set, the execution of Ersatz tests requires a formulation for how that judgement would be applied to arbitrary input data. It is possible that judgement that seems reasonable applied to real data could fail an Ersatz test on generated data. In this way, an Ersatz test may also highlight the consequences of systematically selecting favourable points. It may also be that the process of judgement capture highlights a previously undetected human bias and a firm decides to address the data collection bias rather than reporting a test fail.
5. Model Risk: Lessons Learned From Other Industries
There are many industries that use models to help with their decision-making, not just financial services. This section presents views from other industries gleaned by on-site interviews or from the contributors’ personal work experiences.
5.1. Weather Forecasting
5.1.1. On 6 January 2017 this year, Andy Haldane, Chief Economist at the Bank of England, famously likened the collective failure to predict the 2008–2009 financial crash to what he called a “‘Michael Fish moment for economists” (BBC, 2017). To his eternal chagrin, Michael Fish was unfortunate enough to have been on duty as the BBC evening Met Office weather forecaster a few hours ahead of a most dramatic storm event over the southern United Kingdom in October 1987. Haldane’s jibe seems altogether inauspicious for us, as we look to the meteorological and environmental sectors for some lessons to be learned for our immediate needs in the insurance, finance and banking sectors.
5.1.2. And yet, as it happens, in March of just the previous year (1986), the Royal Society and British Academy had convened a joint Symposium on “Predictability in Science and Society” (Mason et al., Reference Mason, Mathias and Westcott1986). It covered the gamut of disciplines, from “Historical Inevitability and Human Agency in Marxism” (Cohen, Reference Cohen1986) to “The Recently Recognized Failure of Predictability in Newtonian Dynamics” (Lighthill, Reference Lighthill1986) – and a good deal in between, including “Predictability and Economic Theory” (Sen, Reference Sen1986), “Application of Control Theory to Macro-economic Models” (Westcott, Reference Westcott1986) and “The Interpretation and Use of Economic Predictions” (Burns, Reference Burns1986). Unsurprisingly, Sir John Mason (sometime Director of the Met Office) contributed a paper on “Numerical Weather Prediction” (NWP). Significantly, it provides insights into how the use of models in weather forecasting had enabled these forecasts to be improved markedly since the 1960s. In addition, it sets out some principles for gauging and tracking forecasting “skill”, and which principles are still in place.
5.1.3. Making progress: Already in 1986, Mason was able to report on significant progress since the 1960s in NWP. Important for present purposes, some of the progress Mason records is charted in terms of a measure referred to as skill. Thus, we have this:
Although RMS [root mean square] errors and correlation coefficients are useful indicators of the performance of different models for the same area and period, they are only partial indicators of the model’s predictive skill. A better judgement is obtained by comparing the forecasts RMS errors with the long-term climatological variance or with the errors of a persistence (zero-skill) forecast based on persistence (no change) from the initial conditions (Mason, Reference Mason1986, page 53).
5.1.4. In other words, progress can be gauged according to the improvement in, say, the root mean square (RMS) error of the given forecast relative to that of the naïve forecast of tomorrow’s weather being the same as today’s – that most rudimentary of straight-line, indeed horizontal, extrapolations. In 1984, certain features of the 72-hour-ahead forecast showed RMS errors at just 48% of the naïve (persistence) forecast. These errors had been at the level of 80% 10 years previously. Errors at that 80% level in 1984 were not reached until the 6-day-ahead forecast, “suggesting a gain of three days in predictive skill” (Mason, Reference Mason1986, page 53).
5.1.5. What lay behind such progress? Unsurprisingly, it was investment in computing power and model “complexity”. A 10-level northern-hemisphere model had been introduced in 1972 and a 15-level global model in 1982. RMS errors were roughly halved over 1972–1984. 72-hour-ahead forecasts in 1986 were as good as the 48-hour forecasts had been 7 years previously and 48-hour-ahead forecasts as good as the previous 24-hour-ahead forecasts.
5.1.6. Mason proceeds to observe that:
Numerical forecasts are unlikely to provide good or useful guidance for the issue of surface weather forecasts if the RMS error exceeds 75% of the persistence error (Mason, Reference Mason1986, page 54).
5.1.7. From this he goes on to address the matter of the scope for improvements in predictive skill, from which (on the basis of a hypothetical, simulated case study) he concludes:
These figures [numerical details of forecast persistence errors and skill from the case study] suggest that it will, in general, be very difficult to produce useful deterministic forecasts of synoptic-scale developments for more than 14 days ahead … (Mason, Reference Mason1986, page 58).
5.1.8. Yet the 1986 Symposium was about Predictability (and the “failure of predictability in Newtonian dynamics”, as Lighthill (Reference Lighthill1986) put it). Thus, it is to this that Mason turns to close his contribution. Acknowledging his question as a rhetorical one, he asks:
[W]ould it be possible to predict the atmospheric evolution from an initial state with infinite precision infinitely far ahead? (Mason, Reference Mason1986, page 58).
5.1.9. His answer, of course, is “no”, and on two accounts. First, the entirety of the initial state cannot be observed in principle, even if it could be observed in the absence of measurement error. Second, while atmospheric behaviour does have some periodic components (e.g. diurnal and annual fluctuations), it has a strong aperiodic component, notably the movement of cyclones and anti-cyclones across middle-latitude continents and oceans. “An aperiodic system is inherently unstable”, Mason tells us, “so that the imposition of a random disturbance will render it chaotic (i.e., unpredictable) in the long run”.
5.1.10. Today, the Met Office (2017) is still able to report on “Continually Improving Our Forecasts”. No longer is the 15-layer global model of Sir John Mason’s days in use – but the same kinds of error statistics and index of forecasting skill surely are. Specifically, progress has been achieved through “[i]nvesting in technology, scientific expertise and verification”. Among these, technology has amounted to an IBM supercomputer, upgraded in 2012 and capable of 1,200 trillion calculations per second, and a 70-layer model at the global scale (along with similar 70-layer models of progressively more finely resolved spatial detail for Europe and the United Kingdom). Investment in these models and in the function of verification, to which a group of analysts is entirely dedicated, has enabled the Met Office (as it reports) to outperform five of the major operational NWP centres. Over the same period (August 2009 to February 2013) the Met Office’s NWP (verification) Index rose from just over 117 to just over 123 (the target set for March 2013), almost without faltering (Met Office, 2011).
5.1.11. Across three and more decades, then, impressive progress has been made in respect of the statistic of forecasting skill and the accuracy of the models used for NWP.Footnote 3 Targets for forecasting capacity are set by the Met Office; they are to achieve a specified value for an NWP Index by a specified date. And progress towards (or away from) this target is tracked publicly: with transparency, that is, and for all to witness.Footnote 4
5.1.12. Monitoring progress: institutional arrangements: In 2000, the World Meteorological Organisation (WMO) produced its Technical Document TD 1023 “Guidelines on Performance Assessment of Public Weather Services”. The web page introducing this document succinctly (and significantly) shifts emphasis away from the statistics of forecasts and the verification of models towards user satisfaction with the model’s forecasts. It states:
The aim of the evaluation is twofold: firstly, to ensure that products such as warnings and forecasts are accurate and skilful from a technical point of view and secondly, that they meet user requirements, and that users have a positive perception of, and are satisfied with the products (WMO, 2017).
5.1.13. Technical Document 1023 pushes the point further home:
Forecast accuracy is irrelevant if the forecast products are not available to the public at a time and in a form that is useful.
An assessment programme can be seen in the context of a quality system, where it is important to ensure that the information gathered and processed is focussed on user requirements, to be used in making decisions and taking actions to improve performance, rather than just being gathered for the sake of it (WMO, 2000, page 1).
5.1.14. Of course, this is not to say that verification is unimportant. As the web page states:
The main goal of a verification process is to constantly improve the quality (skill and accuracy) of the services. This includes:
-
∙ Establishment of a skill and accuracy reference against which subsequent changes in forecast procedures or the introduction of a new technology can be measured;
-
∙ Identification of the specific strengths and weaknesses in a forecaster’s skills and the need for forecaster training and similar identification of a model’s particular skills and the need for model improvement; and
-
∙ Information to the management about a forecast programme’s past and current level of skill to plan future improvements; information can be used in making decisions concerning the organisational structure, modernisation and restructuring of the National Meteorological service.
5.1.15. In this we can see the virtue of consistency of model and forecast assessment (not just transparency).
5.1.16. Nevertheless, out of a total of 32 pages of text in the main body of WMO TD 1023, 15 are devoted to “User-based Assessment”. Their content covers variously: surveys of the user community (almost the entire appended material is an exemplar of such a survey); focus groups; public opinion monitoring; feedback and response mechanisms; consultations through users’ meetings and workshops; and the collection of what are referred to as “anecdotal data”. We should be left in no doubt about the emphasis national weather forecasting services are urged (by the WMO) to place on user-based assessment, vis-à-vis verification.
5.1.17. Admiring what we cannot have: There are things that transfer readily across the disciplines and sectors, from atmospheric physics and weather forecasting to economics and the insurance industry, and there are things that do not.
5.1.18. On the positive side of the ledger, economic–financial forecasting error statistics can be reported just as they are for weather forecasting. For instance, Sir Terence Burns’ contribution to the 1986 Symposium on Predictability mirrors that of Sir John Mason. Error statistics are plotted for 1-year- and 2-year-ahead forecasts of GDP and the retail price index, for the years 1963–1985 and 1971–1985, respectively (Burns, Reference Burns1986). Salient, however, is the absence of corresponding statistics for (economic) forecasting skill, which, as quite apparent from the foregoing, is distinctively central in weather forecasting.Footnote 5 At the time, Burns was working for HM Treasury.
5.1.19. On the negative side of the ledger, and as the Preface to the 1986 Predictability Symposium observes (Mason et al., Reference Mason, Mathias and Westcott1986), there is this:
The weather forecast does not affect the weather, but the economic forecast may well affect the economy!
5.1.20. Adding to this obvious (and profound) difference, if not elaborating it expressly, Sen opened the Symposium with his paper on “Prediction and Economic Theory”. In this he reasoned that the origins of why economic predictions are so difficult lay then (as doubtless still now) in the complexity of what he called “the choice problem” and “the interaction problem”:
One source of this complexity [in how economic influences operate] lies in the difficulty in anticipating human behaviour, which can be influenced by a tremendously varied collection of social, political, psychological, biological and other factors. Another source is the inherent difficulty in anticipating the results of interactions of millions of human beings with different values, objectives, motivations, expectations, endowments, rights, means and circumstances, dealing with each other in a wide variety of institutional settings (Sen, Reference Sen1986, pages 4–5).
5.1.21. The choices resulting from human behaviour may well subsume the processing of forecasts of future system behaviour deriving from a computational model – something we have referred to in the discussions of our Working Party as the problem of “endogeneity”. But Sen (Reference Sen1986) makes little reference to the quantitative side of economic forecasting.
5.1.22. Nearly three decades later, Greenspan (Reference Greenspan2013) certainly does. Indeed, his book bears the title The Map and the Territory, qualified (significantly) by the subtitle Risk, Human Nature, and the Future of Forecasting. The book is replete with tables and time-series of economic and financial statistics; regression analysis is prominent. What Greenspan has to say of the future of (economic) forecasting deserves to be reported in some detail. In doing so, we seek to redress the rather negative balance in our comparison (from 1986) of the gulf between weather forecasting and economic forecasting.
5.1.23. To begin, Greenspan reaches back to a time well before 1986. He wants to anchor what he refers to as the “propensities” of human nature in what Keynes called “animal spirits”:
My enquiry begins with an examination of “animal spirits”, the term John Maynard Keynes famously coined to refer to “a spontaneous urge to action rather than inaction, and not as the [rational] outcome of a weighted average of quantitative benefits multiplied by quantitative probabilities”. Keynes was talking about the spirit that impels economic activity, but we now amend his notion of animal spirits to its obverse, fear-driven risk aversion (Greenspan, Reference Greenspan2013, page 8).
5.1.24. Greenspan proceeds to define a dozen and more of his human propensities, ranging from fear and euphoria over herd behaviour to time preferences, home bias and family dependency. He does so because what Haldane dubbed the “’Michael Fish’ moment” for economic forecasting – the Great Financial Crisis of 2008–2009 – was something of an epiphany for Greenspan:
[For] now, after the past several years of closely studying the manifestations of animal spirits during times of severe crisis, I have come to the view that there is something more systematic about the way people behave irrationally, especially during periods of extreme economic stress, than I had previously contemplated. In other words, this behavior can be measured and made an integral part of the economic forecasting process and the formation of economic policy. [Emphasis added]
In a change of my perspective, I have recently come to appreciate that “spirits” do in fact display “consistencies” that can importantly enhance our ability to identify emerging asset price bubbles in equities, commodities, and exchange rates — and even to anticipate the economic consequences of their ultimate collapse and recovery (Reference Greenspan2013, page 9).
5.1.25. And so it is that in the closing chapter (“The Bottom Line”), we find Greenspan’s manifesto for the future of (economic) forecasting, summarised by this sequence of quotes. First, on page 291:
When I was first contemplating the substance of this book, I was fully aware that a basic assumption of classical and neoclassical economics — that people behave in their rational long-term self-interest — was not wholly accurate. Moreover, the crisis of 2008 had impelled me to reassess my earlier conclusion that our animal spirits were essentially random and hence impervious to economic modeling. I was amazed, however, during the early months of this venture at just how many supposedly random variables were explained by statistically highly significant regression equations. Many, if not most, economic choices, the data show, are demonstrably stable over the long run for as far back as I can measure (Greenspan, Reference Greenspan2013).
5.1.26. Second, on page 292:
Producing a fully detailed model is beyond the scope of this book.
These models [those of the future] should embody equations that, when possible, measure and forecast systematic human behavior and corporate culture (Greenspan, Reference Greenspan2013).
5.1.27. Then, third, on page 293:
But we are far removed from the halcyon days of the 1960s, when there was great optimism that econometric models offered new capabilities to accurately judge the future.
This journey of analysis has finally come to rest in a place I could never have contemplated when I first began to recalibrate my economic views in the light of what the crisis of 2008 was telling us about ourselves (Greenspan, Reference Greenspan2013).
5.1.28. Thus, to conclude, on page 299:
[W]e are driven by a whole array of propensities — most prominent, fear, euphoria, and herd behavior [at most, three of the thirteen] — but, ultimately, our intuitions are broadly subject to reasoned confirmation (Greenspan, Reference Greenspan2013).
5.1.29. Considerations for actuarial models: What, then, are the lessons to be learned from this look over our professional, disciplinary boundaries across to weather forecasting? What does all this mean – the weather forecasting of today and 1986 and economic forecasting of 1986 and today – for practical progress in communicating and managing model risk in the insurance industry?
5.1.30. Significantly, we (as actuarial professionals) cannot enjoy the detachment of the mechanics of future weather from today’s model-generated forecast of it. Neither may we cling to the aspiration that (one day) the truth of the matter will be revealed in some gargantuan set of differential equations and an unbelievably all-encompassing, finely granulated, real-time observing system for generating (objective) facts and data – about all those human intentions and interactions to which Sen (Reference Sen1986) refers.
5.1.31. Yet, there might be scope for reporting (somewhere) the statistics of our forecasting skill, with consistency, so that progress (or not) may be tracked over the years and decades, and with transparency – for those who have a “right” to see into the black boxes of modelling in the insurance industry. True, the user audiences and constituencies served by national weather forecasting institutions may be very different from those served within and by insurance businesses large and small.
5.1.32. Nevertheless, a leaf or two might be taken out of the WMO’s Technical Document TD 1023 “Guidelines on Performance Assessment of Public Weather Services”. We have much in sympathy with its focus on user satisfaction and users’ positive perceptions of models and model-generated forecasts. After all, given Andy Haldane’s jibe, and as observed by several members of our Working Party, modellers, models and their forecasts – dreaded experts with their dreaded expertise, no less – are not held in high public esteem at present (see also Williams, Reference Williams2017). At the very least, this case study (in particular, the WMO Technical Document) re-emphasises what the insurance industry and profession already seek to achieve with their Continuing Professional Development activities and their Technical Actuarial Standard (TAS) protocols.
5.1.33. The skill of our models and the skills of our modellers are ever “works in progress”; and as such they are in need of active continual improvement. That much we can take from our admiration of the practice of weather forecasting. But are we questioning whether we have the right skills for our sector, that is, ones that motivate improvement, as opposed to enabling more boxes to be ticked with ever greater routine efficiency?
5.1.34. The key is that there are some “positives” involved in the use of models in the insurance industry, not just the perceived “negatives” of yet more procedures to be followed for the purposes of complying with regulations. How exactly our profession might go about this in a sincere and genuine manner may be a sizeable challenge. We have no wish to be accused of yet more “spin” and obfuscation with what the public already looks down on as the “black boxes” of our models.
5.1.35. In the short term – building upon the use test of Solvency II, for instance, and taking the pragmatic business-person’s perspective – we might seek to lessen and dilute out the presently overly strong association of models with the “burdens”, “obligations” and the “worries” of capital allocations. Imagine, for example, a firm’s model users (as opposed to the model developers) parameterising its models directly, something which would not be possible for the consumers of weather-forecasting products. Indeed, given Greenspan’s reported success in encapsulating his “human propensities” in the statistical forms of fat-tailed distributions and regression relationships, the nature of the model and the language surrounding its discussion and parameterisation might thus be a step closer to the familiar, colloquial terms of everyday business (as opposed to the abstractions of computer software). We might even suggest there could be a certain user-friendly “greying” or “colouring” of the model in this. Furthermore, accounting better for these human propensities lies at the root of reversing the low esteem in which economic forecasting is held, relative to weather forecasting.
5.1.36. In the longer term, there may be scope for developing ways of designing and using models to address the challenges of “group-think” forming and then crystallising out in the making of insurance business decisions. The HM Treasury Report of 2013 (HM Treasury, 2013) was well aware of the difficulties associated with group-think in respect of the use of models in support of government decision-making, as already discussed in our Sessional Paper from Phase 1 (Aggarwal et al., Reference Aggarwal, Beck, Cann, Ford, Georgescu, Morjaria, Smith, Taylor, Tsanakas, Witts and Ye2016, pages 291–292, in particular). Group-think suggests a firm is, as it were, “touching just one base” in its deliberations prior to coming to the actionable decision. The firm is using just a single rationality: a single view of how markets work, with a set of business aspirations and risk preferences for the future similarly aligned with just this single mental model of the way the world works. In particular, in the context of Figure 2 (see sections 1.1.16–1.1.17) group-think would correspond to parameterising the computational model according to just one of the four cultures of model users: that of solely the “Confident model users”, or solely that of the “Conscientious modellers”, or the “Uncertainty avoiders”, or the “Intuitive decision makers”. In other words, this is the situation in which just the one predominant view in the firm is aired before the decision is made (and probably alternative views may not be heeded, nor even canvassed). There are precedents for how a plurality of views and aspirations might be explored computationally, that is, the means to “touch all four bases” before settling upon the decision. Oddly enough, these precedents can be found in the differential-equation-dominated worlds of climate change (van Asselt & Rotmans, Reference van Asselt and Rotmans1996) and environmental protection (Beck, Reference Beck1991, Reference Beck2014). There are distinct echoes in them of the Reverse Sensitivity Testing touched on above (in sections 4.6.13–4.6.16). But as we say, technically facilitating this line of enquiry, for then its implementation in practice, might be something for the more distant future.
5.2. Aerospace
5.2.1. The aerospace industry is a sophisticated user of modelling techniques. Two well-known areas include: (i) computational fluid dynamics (CFD) modelling of aerodynamic responses in support of airframe design; and (ii) and the use of automated flight controls systems that underpins both “fly by wire” assistance for human controlled flight and autopilot functionality.
5.2.2. When things go wrong, detailed investigations into the cause of any incidents are carried out by independent investigators and learning points for design, manufacture or operation are published. The learning points often become regulatory imperatives.
5.2.3. Key points for CFD: The reason for using these techniques is to make the overall design-time processes more efficient and to reduce the time to market. This does not remove the need for testing in wind tunnels and flight-testing in the latter phases of development because ultimately the physical aircraft is the product that must fly in the real world, not the model in a simulation.
5.2.4. Whilst CFD modelling can make the overall process more efficient it comes with its own costs of supplying modelling expertise and the need for considerable computer power to provide the accuracy required.
5.2.5. Amongst the significant modelling challenge are the need to divide the three dimensional modelling space using a practical-sized grid, ensuring that the individual “cells” of the grid communicate adequately between each other and the modelling of discontinuities of the physical world.
5.2.6. Once the CFD modelling phases are complete then the testing moves into real-world validation with a series of physical models. Differences between the modelled result and the physical results are a driver for change as the physical development continues. The differences between modelled and physical results may also reveal potential enhancements to the modelling tools.
5.2.7. Aerospace components are designed and tested within a complex “envelope” covering multiple parameters such as weight, altitude, velocity, attitude, banking angles and so on. To ensure the integrity of the individual components and the safety of the overall aircraft, operation outside of the accepted “envelope” is not permitted.
5.2.8. Considerations for actuarial models: Although actuarial models do not have a physical representation there will be opportunities to compare the results of an actuarial model with the real world that is it intended to represent. This should form part of a model review process that regulations or good practice require.
5.2.9. The independence of CFD modelling and wind tunnel tests is self-evident. The latter will form a key sense-check on computed modelling errors arising from flaws in the coding or execution. In the actuarial modelling world there may only be a single model and its software implementation. Where the model is very complex and perhaps contains counter-intuitive results in some circumstances, there may be benefits in constructing an independent model that can be used to validate key features. “Back of the envelope checks” are much harder to compute with a calculator in the 2010s.
5.2.10. Modern financial instruments, consumer products, demographics and customer, and management actions may all contain discontinuous distributions and non-linear responses. Actuarial model design should identify these features and assess their potential to create material discontinuities in the model results that are used for decision-making. The selection of modelling granularity is likely to have greater relevance in one or more of the modelling dimensions where discontinuities exist.
5.2.11. The laws of physics do not change, but the markets and demographics that actuarial models are created to represent do. Actuarial models may benefit from having an “operating envelope” defined for them that may reduce the risk of a model being used in inappropriate or untested environments where the results are not yet proven to be correct.
5.2.12. Key points for flight control systems: Flight control systems have been created to reduce workloads for flight crew and the current generations of systems are now capable of carrying out nearly all of the phases of flight without human intervention.
5.2.13. These applications prevent the flight crew from attempting to push individual settings or perhaps the performance of the aircraft in one particular area outside the operating envelope. There have been cases where the envelope, which is a complex set of inter-related factors, was incorrectly implemented in software and was a contributing factor in the loss of life.
5.2.14. In some military applications the flight control system goes a step further as the intentional instability of such aircraft, designed this way to provide additional manoeuvrability, means that flight computers must be used as a human could not normally fly the aircraft without their assistance.
5.2.15. Critical systems areas may be engineered with multiple levels of redundancy to reduce the risks arising from single points of failure. The redundancy may involve physical components such as sensors and actuators and also multiple software routines. These can also be combined to take a majority “vote” on actions to be taken in the event of conflicting or missing signals and “fail safe” designs which reduce the risk of a wider problem arising when some components or processes fail.
5.2.16. Considerations for actuarial models: The processes wrapped around many actuarial models have already required significant investment to automate and streamline, to meet shorter reporting cycles and enable operating efficiencies. There is little new to be found in considering automation per se.
5.2.17. A more interesting area to explore is whether actuarial models and their processes have clearly defined operating envelopes to ensure that they are not used beyond the boundaries of their design.
5.2.18. Actuarial models will often be run within organisations that have business continuity plans that provide for redundancy in office locations or computer systems. At a more localised and granular level there may be some benefit in exploring how a model would be run in the absence of one or more areas of input data. For example, if there was a significant change in market values and a model needed to be re-run, but a set of scenarios required as input to the model was not available, consideration could be given to how an approximation to the inputs could be created or how previous model results could be reused to allow for the new conditions.
5.2.19. Key points for incident investigation: The purpose is to learn lessons for the future and reduce the risk of loss of life, injury and also the consequent financial impacts. Independent investigators examine, with the widest of remits, any and all factors that may have contributed to the incident. Investigations may also be carried out into near misses and other events that exhibit the potential to have caused a more significant incident.
5.2.20. Areas of investigation cover design, manufacture, maintenance, operation, security, procedural and human factors, and in many cases an incident will be found to have been caused by multiple factors, often from different areas.
5.2.21. A key foundation of all investigations is the data retrieved from the flight data recorder and the voice recorder – the so-called black boxes. Consequently the performance and resilience of these recorders are standardised and mandatory, depending on the category of aircraft and its type of operation.
5.2.22. The human factors involved include the relationship between the flight crew and how this may have impacted on their performance. Factors that may be examined include experience, corporate seniority, procedures and training.
5.2.23. Considerations for actuarial models: Problems with actuarial models have consequences that are on a lower scale than those in the aerospace industry, but they may still have high financial and social costs.
5.2.24. Individuals with the relevant professional skills and independence should therefore carry out investigation into significant model failures or underperformance.
5.2.25. Investigation into model performance should not, however, be limited to failure, but be built into the normal operating processes. In many areas of actuarial modelling this review process is built into the regulatory framework.
5.2.26. Human factors are an important area for users of actuarial models, where user here should be taken to mean everyone from the model operators, through management to the ultimate decision-makers who rely on the outputs. (It is an oversimplifying generalisation to say that the builders and runners of models need to improve their communication skills and that the ultimate decision-makers need to improve their understanding of the construction and the limitations of a model … but when financial models go wrong, those factors are often present.)
5.3. Software Development: Design and Testing
5.3.1. The development of models and software are closely interlinked. For the purpose of this section a distinction is to be made between the “conceptual model” and the “software implementation” of that model. In theory, if not in practice, results from a “conceptual model” could be calculated using a pad of paper, a pencil and a calculator.
5.3.2. From the 1980s, the personal computer revolution placed ever-increasing computer power in the hands of actuaries, enabling ever more sophisticated models to be implemented. Actuarial software implementations use a combination of specialist actuarial tools, general-purpose databases and spreadsheet systems and bespoke code. In all of their software design, build, test and deployment activities, actuaries have had access to the expertise of information technology (IT) professionals and to the evolving tools and techniques of that profession.
5.3.3. As a relatively new industry with ever more diverse application and continued rapid growth, software development methodologies have also continued to evolve and adapt. Over the past decade, the use of “Test-Driven Design” (TTD) and “Behaviour-Driven Design” (BDD) methodologies have been widely adopted to support faster development cycles. These methodologies are often deployed with “Continuous Integration” – a technique where incremental changes are made to software on a frequent basis (often daily) and an evolving development version of a software system is always being run and tested.
5.3.4. Whilst no one single style or methodology of system development can be said to be best suited to the development of actuarial conceptual models and their software implementations, these newer techniques bring from the IT industry some vocabulary, methodology and standardisation that may be of use. In addition, these methods formalise and support some of the styles of rapid application development that many actuarial professionals have used for the past 20+ years.
5.3.5. Key points for TTD/BDD: The essence of these techniques is that the tests for the new software are defined and created up-front before the new code is written. Usually the tests themselves will be part of a testing framework that executes the new software as it is created.
5.3.6. The new software is then incrementally developed to meet the requirements of the tests. In general the “TDD” name is applied when dealing with relatively small pieces of code, whilst “BDD” applies to a system or a subsystem.
5.3.7. Benefits arising from TDD/BDD include clearer documentation of what the software has been designed to do and whether, according to the test status, it is capable of doing it as required.
5.3.8. A corollary of the scope of the TDD/BDD test suite is that the software may not be considered as capable of performing a function or dealing with a situation for which there is no explicit test.
5.3.9. Considerations for actuarial models: TDD/BDD methodologies are useful techniques to consider using to develop both the conceptual models and their software implementation.
5.3.10. Whether or not such a methodology is used for developing a specific software implementation, the underlying thinking should be an important check for the use of a conceptual model. It is important that a model, or the software, is only used in an environment and with inputs that have been tested for and for which it is known to perform as required. (This is the same point as the aerospace operating envelope.)
5.3.11. The creation of sensitivity tests and scenarios for actuarial models is a closely related practice.
5.3.12. Software development: meta data: As noted in section 4.6.3, meta data is information or data that describes other data. Meta data can be somewhat mundane, such as the count of rows and columns in a table, but even this can usefully be the foundation of important tests and controls that will be very familiar and commonplace to actuaries using many types of software.
5.3.13. The increasingly diverse sources of data being collected, transformed and stored by applications in general has increased the attention given to the meta data that is generated by software systems as they carry out their primary tasks. Although the lines of what constitutes meta data versus what constitutes primary data and results may be blurred, it is not necessary to draw a firm distinction between them where the information encapsulated by the meta data is useful.
5.3.14. Considerations for actuarial models: Meta data has for a long time been an important resource for controls of actuarial models, assumptions and results. As new and more complex models are developed it may be helpful to consider areas where meta data may provide additional insights into why the model has performed in the way that it has.
5.3.15. Meta data may also be designed to provide a more efficient way of analysing and comparing results between different runs of a model. For example, when seeking to evaluate the impact of a basis change on a calculation of liabilities, it may be useful to arrange that the outputs of the model provide supporting intermediate data. In this way, the impact of a change to expense assumptions for a sensitivity analysis might be shown as being isolated to the expense meta data with other meta data (e.g. number of policies, premiums, claims and investment returns) being unchanged between the two runs. If these other items were to change in response to a basis that has updated expenses, this could be an indication of a problem with the setup or the execution. (And in a more sophisticated model, where policyholder or management actions in the model are a function of expenses, further layers of meta data could be designed to provide additional insights into the way that these actions have been triggered when expenses differ.)
5.4. Lessons From the Auditors
5.4.1. Much value can be gained from considering model risk from the perspective of a firm’s internal and external auditors. These actors are sometimes referred to as the “third line” of defence in mitigating model risk, behind the “first line” (the day-to-day model users) and “second line” (a firm’s dedicated risk and oversight function).
5.4.2. From discussions with auditors, the following challenges are made which we consider to have great validity and are at the heart of mitigating model risk. We imagine a scenario where a senior decision-maker within a firm is presented with the results of a model and asked to make a significant decision using those results.
5.4.3. From the perspective of the decision-maker, it is reasonable to ask some simple but key questions around this scenario. First, have the model or models used to produce these results been used appropriately? And, second, are those models that have been used “correct”? Is the methodology implemented by the models sound, and has it been reviewed? Could, an audit trail be produced showing a clear lineage from initial model specification, test plans, test evidence and model sign-off dating back to the first version of the model?
5.4.4. We argue that we should be able to answer these questions positively – as being unable to do so must inevitably cast doubt over the model’s results that have been presented. But, how many models that we use have this watertight “specification-test-signoff” audit trail back to the first version of the model? Surely, this is the gold standard for which we should be aiming.
5.4.5. Further, very often modelled results are not simply the result of a single model but can consist of a complex modelling process with many different “cogs” in the wheel. In these circumstances, are the criteria in section 5.4.3 not equally relevant to all the components?
5.4.6. From discussions with auditors, and practical experience, it is imperative that when models are used all controls around those models are evidenced in real time. Examples of controls include: first, that only those models that are listed in your central inventory are used; that all doer/checker processes have been completed and real-time evidence has been provided; and all model results can be satisfactorily explained.
5.4.7. To summarise, from an audit perspective, good model governance and evidence of correct model usage must be at the heart of any model risk governance framework. This includes a rigourous model development process (change requirements, testing and sign-off evidence) and evidence that only the latest signed-off models have been used, and that those models have been used appropriately.
6. Practical Applications of Model Risk Management in Actuarial Fields
6.1. Life Insurance
6.1.1. The authors have found the following approaches to be helpful in mitigating model risk especially now that Solvency II is live and reporting has become a business as usual quarterly activity.
-
∙ all models are logged in a central inventory to give a reliable snapshot at each valuation date of all signed-off models with version number and model purpose, and associated documentation and training notes;
-
∙ education and controls to ensure staff use the “correct” (i.e. latest signed-off version) of each model – and checking to monitor compliance;
-
∙ a model development process that distinguishes between “central” and “local” changes, with differing but similar control processes applicable to each;
-
∙ an established model development process that consists of: idea initiation and feasibility investigation; model change prioritisation to produce an agreed model change stack each quarter; requirements specifications for those developments that become approved; development of agreed model changes based on user requirements. Then, functionality testing based on user requirements; followed by UAT of model changes; and, finally, end-to-end testing of all model changes brought together before the next quarterly reporting date;
-
∙ great benefits have been seen in the automation of time-consuming, repetitive and manual processes (e.g. via Visual Basic for Applications (VBA) macros);
-
∙ detailed commenting of any underlying VBA code such that code is written “for others” with the acknowledgement that models and VBA code base will change over time;
-
∙ where possible, simplification of modelling processes and removal of modelling where this can be justified;
-
∙ production controls performed in “real time” and evidence prepared with the expectation that this will be shared with internal and external auditors and senior management; and
-
∙ common folder structures used to store models, model inputs and results each quarter, making finding models and key input files straightforward quarter on quarter.
6.1.2. Some of these points are now expanded on further.
6.1.3. Maintenance of a central model inventory: At any particular time, a business should know all models that are in use, their names and version numbers, the purpose for which they should be used, and a named owner for each model. We hope that this statement is hard to argue against. Inventories could be at a department level and be owned by the head of department, or more ambitiously be at a company-wide level possibly global in extent.
6.1.4. The key point is this: if we do not know what models are in use, then how do we ensure that the right models have been used in our modelling activities? As a minimum we would expect an inventory to record model name, version number, purpose, ownership, evidence of compliance with End User Application standards, and have sufficiently detailed documentation to support its successful use.
6.1.5. An inventory may run to many hundreds of tools and, while it might take time and resource to compile, we have no doubt of the benefits that arise after the task has been completed. Importantly, the inventory needs to be kept up to date as a living document and should be updated in sync with the quarterly reporting cycle.
6.1.6. Model development process: central versus local change: We have found it beneficial to divide all models into one of two categories: those that are “centrally” managed and those that are “locally” managed. Centrally managed tools will typically be the most important models, for example, those used in financial reporting, pricing or business planning, and these should only change following a well-established model governance process (see section 6.1.8).
6.1.7. Locally managed tools cover all other tools, for example, those “helper” tools in processing and validation teams, for example, which do not directly feed into the central tools. Again, change here should follow a local change process which will have similarities to the central change process but with perhaps less formal requirements gathering and sign-off.
6.1.8. Model development process: practicalities: A robust model development process should be established. This lets the business change its models in a controlled manner and helps balance the competing requirements for more model change (from change owners) against resource demands (the number of staff available to specify, make and test the model change). Frequently, there are more model changes desired than can be accommodated by current resource and so the list of model changes will need to be prioritised with some being deferred to a following quarter, for example. Model changes should be ranked by business benefit, whether that is in terms of monetary benefit or improvements in risk control that a model change can bring.
6.1.9. Once a set of model changes are agreed for a particular quarter, detailed model development requirements need to be written and agreed before model development begins. At the same time, a functionality test plan needs to be written and signed-off by the model change owner. The aim here being to demonstrate that the model changes have been done correctly based on the model change requirements. We have found it very beneficial to share documents using a single source (e.g. SharePoint or company intranet) so that colleagues working on model developments can all easily find the latest documents and to ensure that, at all times, people are working from the same version.
6.1.10. Following successful functionality testing, the model change needs to be passed to UAT. The aim of UAT is to establish that the model change affects the model results (e.g. financial results or pricing quotes) in an understood, anticipated and acceptable manner. A UAT plan needs to be written and signed-off by the model change owner. Frequently, UAT will focus on a regression test back to the previous year end or prior quarterly reporting period.
6.1.11. Following successful UAT of all quarterly model changes, an end-to-end run is done ahead of the next quarterly reporting date to check that all parts of the process (“cogs in the wheel”) work together seamlessly and to ensure a smooth modelling process at the quarterly valuation date.
6.1.12. We note some caveats: we have found that the functionality testing, UAT and end-to-end can work well, but timings are often under-estimated and we suspect that this is an industry wide problem. It is very easy to plan for a model change to come in at a particular date in the future, but much harder to prevent scope creep in development and delays in receiving signed-off requirements, development and testing, such that meeting the original intended date very often becomes a significant challenge.
6.1.13. In general, therefore, we suggest that fewer model changes are brought through each quarter and those that are have been relentlessly prioritised to deliver the most business benefit for the resources employed. We have found it necessary to consider not only model changes but knock-on impacts to upstream and downstream processes, for example, if input formats change (but underlying numbers are unchanged) and this needs to be anticipated in advance. For this, a detailed process map is required and should show all key reporting metrics such that if any part of the “process” changes the impact across the board is readily understood.
6.1.14. We also strongly endorse continuous improvements, no matter how small, to ensure that modelling processes become slicker and progressively more coherent and streamlined quarter-on-quarter. There are always ways that models can work better, or more reliable and efficient controls that can be performed. Given that key models may be run many hundreds of times during a quarter, improvements in run speed can be very beneficial. Likewise, time invested improving model documentation and process notes is rarely wasted if it helps ensure that models are run correctly first time.
6.1.15. Commenting of VBA code: Typically, models such as Excel spreadsheets are automated by the use of macros written in VBA code, very often not by professional computer programmers but instead by technically minded colleagues. Workbooks are usually developed by a single person in a silo, usually without collaboration with anyone else. Because of this “amateur programmer” approach, we find that VBA code is often poorly commented and the level of commenting in VBA macros is significantly poorer than would be required for commercial software.
6.1.16. While poorly commented VBA code is not an automatic source of model risk, we think well-commented code offers a number of benefits to the business: it forces developers to think of other future developers and write their code for others; it reduces key person dependency; and it offers a good degree of future proofing. We have found good commenting of code very helpful. A company should have an agreed set of VBA standards to provide common guidance to developers. Some of the standards will be mandatory and others only recommended.
6.1.17. Our recommended key mandatory VBA features include:
-
∙ use of Option Explicit at the top of all modules to enforce syntax checking and ensure code integrity when Debug >Compile Project is run in the VB Editor;
-
∙ referencing cells by named ranges wherever possible, and avoiding cell addresses in code;
-
∙ meaningful commenting of code particularly highlighting the purpose of subroutines and functions, and logic of code chunks. Comments should be kept up to date. For example, this is discussed further in Bovey et al. (Reference Bovey, Wallentin, Bullen and Green2009, Chapter 3). The following type of comment header at the top of subroutines helps orientate a new developer tasked with adapting the codebase:

6.1.18. It is important to ensure that each time a model is used the model starts in a “clean” state so that there is no risk of “stale” data being entered into the model. This is particularly a risk where models read in data – we must first ensure that all old data are deleted so that there is no chance that a small amount of data read in merely appends to a large set of old data still in the model. The old data need to be completely deleted first.
6.1.19. A consistent naming convention for variables (e.g. intCounter, strMessage) makes code easier to follow, understand and extend.
6.1.20. Saving workbooks containing macros as .xlsb (Excel Binary Workbook) instead of .xlsm (Excel Macro-enable workbook) to save file space, with .xlsb workbooks typically being half or one third the size of their .xlsm equivalents
6.1.21. Automation of process: If there are significant parts of any modelling process that are manually intensive then these should, over time, become progressively automated – either through macro-enabled workbooks or other technology. The benefits of automation are many but chiefly: much less risk of setup issues caused by human error particularly during time pressures of reporting cycles; ability to accommodate extra unplanned model runs if these become necessary; reduction in key person dependencies; and, perhaps, reduction in staff turnover as less frustration in manual activities. Automation of process may well lead to a lot of model change especially if this has not been a priority to date.
6.1.22. Simplification of modelling processes: We have seen good examples of three-stage modelling being simplified to two-stage modelling, affording model production and results validation teams much improved processes. Manual interim model inputs are progressively combined into the main suite of models ensuring that the end-to-end model cycle is quicker with fewer hands-in/hand-offs than before. These savings, especially quarter-on-quarter, can be very significant.
6.1.23. Even better than automating processes is the complete removal of those processes in the first place, often in response to the challenge of “why are we modelling this?”. We have seen successful model simplification, for example, removal of tax liability modelling for stressed scenarios within an Internal model and decommissioning of the tax model where this process was seen as excessively complex and adding little value to the business.
6.2. Banking
6.2.1. In a speech on the topic of Governance and the role of Boards delivered to Westminster Business Forum in November 2015 (Bank of England, 2015), Andrew Bailey (Bank of England Deputy Governor for Prudential Regulation and Chief Executive Officer of the PRA) outlined expectations in the area of Model Risk.
Non-Executives should be put in a position to possess a general understanding of the model … without detailed technical knowledge. That’s the job of the Executive, to explain complexity, provide good Management Information … and enable challenge and thus accountability. If Non-Executives do not feel that they can meet these expectations, they should demand the time and support to enable them to do so.
6.2.2. In particular, the following were highlighted as areas a Board needs to understand:
-
∙ Where is the model expected to work well?
-
∙ In what circumstances is it likely to break down?
-
∙ Is the overall model output credible?
-
∙ What “moves the dial” in terms of key assumptions or judgements?
-
∙ Are those assumptions and judgements reasonable?
6.2.3. Thus, banks have had to ensure they develop a framework which addresses these, and other, expectations from the regulatory authorities.
6.2.4. In a bank there exists a multitude of “models”, from the simple spreadsheet put together by an individual to do a little checking job, through to the huge complex models which drive large parts of the business. For a bank, the key risks are generally considered to be financial loss, regulatory censure and/or reputational damage and so this provides the basis of the model risk framework.
6.2.5. This leads to a definition of model in line with the Working Party’s definition, although it may be narrowed to ensure the full weight of the formal governance framework is only applied where needed, that is, to those models which are sufficiently complex and have a real business impact in the context of the bank.
6.2.6. To ensure a model does not creep in or out of such a definition without being noticed, models are classified according to materiality, and the level of governance varied accordingly. Thus, the most attention is given to the major models (level 1, say) and there may only be a handful of these (e.g. below 10). Full governance requirements described below will then be applied to the next level of models with a lighter touch becoming relevant as you progress down though the levels ending with an awareness of those models which are just below the qualification for the framework, in case their significance should evolve. Materiality will be judged against the criteria mentioned earlier, that is, purpose (including regulatory significance), use and financial significance.
6.2.7. A model approved under the framework has a set review date. This is usually annually, but could be earlier where necessary. As part of this assessment a full set of documentation describing the model needs to be submitted to the Model Governance Committee. This is presented by the business area where the model is in use, and has to be signed-off by the second line (the risk team for that business area) and the independent model approval team.
6.2.8. The model approval team is a central team independent of any business area. It will carry out a deep dive into models either when they are introduced or substantially changed, or otherwise on a rolling basis. This deep dive includes building a challenger model, a review of industry practice and a comparison of the impact of alternative options.
6.2.9. The second line will apply subject matter expertise to their review to ensure the technical aspects, as well as the statistical aspects, are being dealt with correctly.
6.2.10. Some models are also subject to regulatory scrutiny and the documentation on the review process may be provided to the regulator as appropriate. Some models require pre approval from the regulator for new models or major model changes (this is similar to insurance models).
6.2.11. The internal model governance committee will review the submission from the three independent teams, and may require further information or investigations to be carried out. As part of the approval, conditions for use will be set, including the review date, any conditions or developments which need to be implemented, the purposes for which the model may or may not be used and the performance monitoring to be carried out.
6.2.12. Performance monitoring, usually carried out quarterly, consists of reviewing Key Performance Indicators which will vary between models. These are likely to consider accuracy, discrimination, stability and usage. This monitoring will be carried out between the business, the second line of defence and the model approval team.
6.2.13. Reporting on the use of the models is then included in internal reporting to senior management. This will be both at a business level and at a consolidated “model risk” level. It includes a Red-Amber-Green status against risk appetite. This gives another route by which any emerging problems should be picked up – senior management will be reviewing the impact of models against risk appetite and so reliance is not on just the model governance processes to pick up any emerging problems.
6.2.14. Finally, as with all aspects of the business, compliance with model governance and risk appetite processes in connection with model risk is subject to internal audit review and regulatory oversight.
6.3. Pensions
6.3.1. Like life insurers, pension schemes put a great deal of reliance on modelling and on experts to use, understand and review these calculations. Within the pensions industry, models are used to value, administer and to project both assets and liabilities into the future. Defined benefit pension liabilities are one of the most material concerns for UK companies and within the industry there is significant pressure on pension schemes due to the low interest rate environment. More and more companies are looking to better understand the value of their pension liabilities, how these will develop in the future and, in some cases, the options available to de-risk their balance sheet by removing the long-tailed pension liabilities through buy-in or full buy-out transactions with bulk-purchase annuity writers.
6.3.2. Within this section, we will consider model risk specific to pension schemes by looking at the regulatory environment, the relationships of the stakeholders and through considering the different calculations that take place. The aim of this section is to ask if there may be an increased level of model risk within pension schemes due to the reliance on key individuals or the long-term, complex, modelling techniques.
6.3.3. The regulatory environment: The current pension regulations within the United Kingdom require auditors to verify the existence and value of scheme assets, and in the case of defined benefit schemes, an appropriate actuary must determine whether the fund’s future liabilities can be met from current assets. These requirements are focussed on the assets that a company holds and the scheme actuary, who will be the person closest to the liability model, is responsible for valuing any deficit that a company might include on their balance sheet. In the circumstance that the scheme actuary does not report a deficit, no formal audit will be required on the liabilities or the models used to produce these values.
6.3.4. This places reliance on scheme actuaries to provide insight to the liability models.
6.3.5. Over the last decade, a number of industries including insurance companies and the banking sector have been required to significantly increase their model documentation and risk management framework in managing their business. Pension schemes will soon be subject to the new EU-wide IORP II (Institutions for Occupational Retirement Provision) regulations which require schemes to implement an effective risk management function similar to the requirements that insurers have seen as part of Solvency II. One of the key aims of IORP II is for firms to actively manage and monitor all key risks to the scheme, which will clearly help to encourage effective model risk management.
6.3.6. Key stakeholders: Considering the current key stakeholders of a pension scheme, there will likely be a variety of experience and views on model risk.
-
∙ The scheme actuary, who will be responsible for the valuation model, will be an experienced actuary and will have a very good understanding of the model, which they have developed. In all likelihood, the actuary is likely to be a confident model user and will put stock in the output of the model.
-
∙ Scheme trustees will have a variety of experience, but it is clear that the majority of focus will fall on the assumptions used within the model and the overall valuation results. A significant amount of reliance will be placed on the expertise of the scheme actuary to ensure the model is appropriate and to inform trustees of any areas of risk.
-
∙ Employers again will likely place reliance on the scheme actuary to understand the model. Employers will focus on the assumptions used to value the liabilities, ensure an appropriate asset return on investments and to understand the level of deficit that the scheme may add to the company’s balance sheet.
-
∙ The regulator will play an important role in reviewing the valuation, however, they may not have the depth of resource to monitor valuations to a level required to consider model risk within the valuation. It is clear that if a scheme announced a significant deficit, then the regulator may take significant interest in the model, but this may only occur in extreme circumstances.
6.3.7. Considering the interactions between the current key stakeholders, there is clearly a question around appropriate levels of challenge to the scheme actuary on the model. It should be noted that while the scheme actuary’s models will fall under the same TAS-M (soon to be TAS-100) requirements as other actuarial models, the pensions’ legislation is not as prescriptive on schemes to develop a risk management framework as for insurers under Solvency II.
6.3.8. As mentioned in section 6.3.5, pension schemes will soon require a risk management function to adopt strategies, processes and procedures necessary to identify, measure, monitor, manage and report risks. This is a clear step forward in risk governance and reduces the overreliance on scheme actuaries.
6.3.9. It should be noted that, even before the implementation of IORP II, there is detailed information provided by the scheme actuary with regards to the model inputs and assumptions. A key part of a valuation report will detail the underlying assumptions of the model, how these have moved since the previous investigation and a number of sensitivities to these assumptions. In communicating changes to the stakeholders, the scheme actuary will often be able to use recent experience within the scheme and the wider industry to frame the reasoning for any changes and to clearly communicate their impact on the valuation. This information helps stakeholders to relate the inputs of the technical model back to the real world and their experiences and will result in significant challenge to the model.
6.3.10. Model uses: The model risk within a pension scheme calculation will differ due to the nature and significance of the calculation. We will consider a number of important modelling calculations separately below.
6.3.11. Scheme liabilities’ valuation: When modelling a tri-annual scheme valuation, any error or mistake within a model would likely have an impact on the funding plan of the scheme.
6.3.12. When valuing the liabilities of a scheme, trustees and employers will primarily focus on the assumptions used within the valuation and the results of the model. Trust is placed in the scheme actuary to develop and run the model and while the assumptions are discussed with trustees, in the past, the models themselves have received limited exposure or review from either the trustees of employers. As already mentioned, more challenge to the actuarial models will be developed as part of the IORP II requirements.
6.3.13. During such a valuation, there are clearly a number of checks that will be performed to ensure the model is appropriate. The scheme actuary will perform checks as with any important actuarial model and trustees will have years of experience to compare against model outputs. Sensitivity testing is provided on the results of a valuation model and will help to highlight the key assumptions. Comparing the requirements of the scheme to other areas of insurance, schemes are not required to do the same level of testing around stress and scenario testing and reverse stress testing that has been developed under Solvency II, which may help to show weaknesses within a model.
6.3.14. Assets: When considering the models used to measure and project the assets held by a pension scheme, due to the complex nature of the investments available, the options for deterministic or stochastic modelling and the long-term projections, this is an area where there is significant tangible model risk, particularly with the asset-liability modelling underlying some of the investment strategy decisions.
6.3.15. Model risk is limited by the requirement of an audit on the asset values and through appropriate actuarial requirements to document and communicate models with key stakeholders.
6.3.16. Risk transfers: A number of pension scheme have undergone risk transfers and de-risking processes. In order to value the transaction, the scheme actuary, the corresponding firm looking to take on the business and the auditors of transfer will all calculate or review a proposed value of the scheme.
6.3.17. In this occasion, there is an increased scope for model risk due to the “one off” nature of the transaction to transfer the scheme, as corrections to any error cannot be implemented after the business has been agreed and transferred. However, the level of model risk within these calculations is mitigated somewhat by having insight from auditors and regulators.
6.3.18. Key models: longevity projections: Longevity risk is an important focus when calculating defined benefit pension liabilities. Due to the long-term nature of this risk, models are required to provide insight of future mortality rates, sometimes decades in advance. These projections have significant impact on the present value of the liabilities within the scheme.
6.3.19. We do know that the nature of longevity risk and the way models are used will vary between schemes and it is helpful to distinguish between three types of longevity risk and assumptions:
-
∙ Level risk: The risk that the best estimate assumption of the scheme’s current mortality experience is inaccurate.
-
∙ Process risk: The risk that the best estimate assumption is not borne out in practice, due to the random nature of mortality.
-
∙ Trend risk: The risk that the assumption made regarding future changes in expected mortality rates is wrong.
6.3.20. The complexity of models used to collect data, analyse trends and to produce assumptions will vary significantly between these risks, meaning there will be different levels of inherent model risk within the calculations. Considering the nature of the models, the most likely area of model risk will relate to the projection of trend risk, which would certainly be the major component for large schemes.
6.3.21. Modelling trend risk: Over the 21st century, the modelling techniques for trend risk have developed significantly. While small- or medium-sized schemes may only have the capacity to develop simplistic deterministic models; larger schemes aim to reduce their longevity risk by modelling future improvements as accurately as possible through a variety of methods (e.g. various standard stochastic models or cause-of-death models). However, in doing this, greater reliance is placed on the scheme actuary’s modelling capabilities and, in advancing the modelling techniques, extrapolating mortality rates into the future still involves unknowable risks.
6.3.22. The level of potential model risk is exacerbated by the technical difficulty that is present in many of the calculations. Communicating the modelling approach used in a less technical setting may be difficult and/or time-consuming. This may suggest that there will be limited challenge of the trend model from trustees and employers.
6.3.23. Model risk, based on misunderstanding or misuse of a model, can be reduced by including sensitivity testing within the communications to the scheme trustees and senior management. This will illustrate the importance of the assumptions produced by the trend model to the overall value of the scheme liabilities. As trustees may have limited interest or understanding of the workings of such a complex model, industry practice is to illustrate what particular future improvements might mean in reality, for example, through a cause-of-death approach. Schemes can also rely on well-regarded professional bodies such as the Institute and Faculty of Actuaries’ Continuous Mortality Investigation who produce industry standards and benchmarking surveys that models can be compared against. The scheme actuary can provide trustees and employers with a comparison of the assumption against the range (e.g. generally long-term rates of mortality improvement sit in a range of 1.25%–1.75% p.a.). This can provide comfort to the final output of the model, even if the actuary has used different methods to get there.
6.3.24. Considerations for actuarial models: Model risk exists in pension schemes just like all other areas of business where the computing power of models provide assistance. Compared to other financial sectors, there may be less scrutiny applied to liability models within pension schemes and significant reliance is placed on the modelling expertise of the scheme actuary. However, we expect an increased focus on risk management going forward following the implementation of IORP II.
6.3.25. We know that due to the long-term nature of the liabilities and the corresponding contributions from employers, individual errors due to model risk may be seen as less material and easily corrected, however, there will be financial impacts and these may be exacerbated if the problem is a deeper misunderstanding that is not highlighted when comparing to benchmarks, analysing sensitivities or undergoing audits.
6.3.26. Considering longevity trend risk, we know that actuarial models have failed to predict the levels of improvements we have seen – both the sustained heavy improvements of recent decades and the slow-down of recent years. These complex models are seen by many within the pensions industry as a “black box”, used to produce results. It is only through encouraging conversations and developing appropriate independent checks that we can try to reduce the risk that future errors are not caused by model errors which could have been avoided.
6.4. Links to TAS-100
6.4.1. A new TAS, TAS-100: Principles for Technical Actuarial Work (TAS-100), has been issued by the UK’s Financial Reporting Council (FRC) and comes into effect on 1 July 2017.
6.4.2. TAS-100 will replace the current TAS’s that cover modelling, data and reporting (TAS-M, TAS-D and TAS-R), and is described fully here: https://www.frc.org.uk/Our-Work/Publications/Actuarial-Policy-Team/TAS-100-Principles-for-Technical-Actuarial-Work.pdf
6.4.3. The purpose of TAS-100 is to promote high-quality technical actuarial work. It supports the FRC’s Reliability Objective that “users for whom actuarial information is created should be able to place a high degree of reliance on that information’s relevance, transparency of assumptions, completeness and comprehensibility, including the communication of any uncertainty inherent in the information”. As such, there are strong links to any discussion of model risk and model risk communication.
6.4.4. The Models section from TAS-100 captures some of key elements we have expanded on throughout this paper. A paraphrased extract from TAS-100 covering the principles for Models is set out below:
-
∙ models used in technical actuarial work shall be fit for the purpose for which they are used and be subject to sufficient controls and testing so that users can rely on the resulting actuarial information;
-
∙ an explanation of how a model is fit for the purpose for which it is used and what it does shall be documented;
-
∙ controls and tests that have been applied to a model shall be documented;
-
∙ communications shall explain the methods and measures used in the technical actuarial work and describe their rationale;
-
∙ communications shall include an explanation of any changes to the methods and measures used from the previous exercise carried out for the same purpose (if one exists); and
-
∙ communications shall include explanations of any significant limitations of the models used and the implications of those limitations.
6.4.5. Considerations for actuarial models: As can be seen in our section 3 (Model Risk Communication) these principles are in line with our approach. We believe that applying these principles will aid in the understanding of the model risk inherent in certain results.
6.4.6. That said, a very detailed approach to these principles could be taken. However TAS-100 also sets out that in applying the principles to a piece of work, a proportionate approach is expected. The discussions in this paper cover many of the principles and hence provide some background when considering how to apply these principles to a specific piece of work.
6.4.7. For example, in section 5.4.3 (Lessons From the Auditors) we discuss the challenges from the auditors around models using appropriate methodology, being “correct” within that methodology, for these models to have been developed and tested in a controlled manner, and so on. The principles set out in the TAS-100 provide a framework for making decisions which will then be robust to the challenge from auditors and other third party users. Compliance will naturally result in evidence that only the latest signed-off models have been used, and evidence of controls around model operation. Where a bespoke model has been developed, we would expect applying TAS-100 to result in evidence of peer review and sense checking of results being available and hence the auditors’ challenge to be easily addressed.
7. Summary Conclusions
7.1.1. In section 1 we recapped the Model Risk Working Party’s Phase I work (Model Risk – Daring to Open the Black Box). In section 2, we reviewed a number of high-profile case studies which demonstrate that the financial and reputational impacts of model risk can be very substantial. In section 3, examples of model risk communication have been given covering communication to a range of audiences: to the Board; to the regulator; and to external stakeholders.
7.1.2. In section 4, we presented a practical framework for model risk management and quantification with examples of the key actors, processes and cultural challenge. Section 5 presented some lessons learned from other industries that make extensive use of models including the weather forecasting, software and aerospace industries. Finally, in section 6 a series of case studies in practical model risk management and mitigation were presented from the contributors’ own experiences covering primarily financial services.
7.1.3. Key points we wish to summarise from the paper are:
-
∙ As acknowledged by NASA, the two key reasons for the failure of the MCO were: an inappropriate culture and inadequate communication. To mitigate these failures, communication channels needed to be improved and personnel urged to question and challenge everything – even those things that have always worked or which appear routine.
-
∙ From the “Growth in a Time of Debt” case study, we have seen the importance of transparency in fostering confidence in models and their results. It is exactly this transparency, common in some (though not all) areas of academic research, that allowed the errors to be discovered. Such transparency is not easily attainable for many models deployed within the financial industry. Consequently, one can only speculate as to the number and impact of errors that sit undetected.
-
∙ As presented in section 3 (Model Risk Communication), good communication of model risk to internal and external stakeholders adds value and promotes confidence; particularly as model risk events become more prevalent in the media and because model risk relates directly to the results on which regulators and investors rely.
-
∙ Section 4 (Practical Implementation of a Model Risk Management Framework) stressed the importance and benefits of maintaining an up-to-date central inventory of all models and classifying all models them into Basic/Medium/High risk. The model risk management effort should be proportionate to the risk a model poses. Third party software, relied on by a majority of financial services companies, should be treated in exactly the same way as in-house models.
-
∙ The importance of regular independent model validation has been highlighted. By this we mean independent validation by people who have no involvement in the design and operations of the particular model being validated. The frequency of review will be at management’s discretion but we suggest as a minimum each High risk model being reviewed at least once every 3 years on a rolling basis.
-
∙ The lessons learned from other industries (section 5) reiterate some of the points from earlier sections but from a non-financial services perspective. Of relevance from the weather forecasting case study is the emphasis on measuring user satisfaction around a model’s results; do they satisfy the users’ purpose? Studying the aerospace industry suggests there is value in questioning whether actuarial models and their processes have clearly defined “operating envelopes” to ensure that they are not used beyond the boundaries of their design.
-
∙ In section 6 we have brought to life some of the practical challenges and mitigating actions that the contributors have experienced in a variety actuarial fields.
-
∙ Appendix concludes the paper by giving an example model risk policy to show how a model risk management framework has been successfully implemented within a large financial organisation.
Acknowledgements
The authors thank the following people and audiences at the following conferences and events, where parts of this report were presented, for valuable discussions and feedback:
-
∙ Professor Chris Kilsby, Newcastle University, for feedback on section 5.1.
-
∙ Solvency and Global Capital Standards for Insurers, Basel, Switzerland, March 2016.
-
∙ IFoA Pensions, Risk and Investment Conference, Edinburgh, 2 June 2016.
-
∙ The CRO Group Forum, London, 14 November 2016.
-
∙ IFoA Momentum Conference, Newcastle, 2 December 2016.
-
∙ Risks in Life workshop, Staple Inn, 9 February 2017.
-
∙ The paper’s reviewers: two from the IFoA and one from the British Actuarial Journal.
Appendix: Example Model Risk Policy (As Presented to the IFOA CRO Group)
The concept of a Model Risk Management Framework was developed in the Phase 1 paper; subsequent feedback challenged how this can be implemented in a practical and proportionate manner. The Working Party therefore developed a full example “Model Risk Policy” to illustrate how a model risk management framework could be practically implemented in an insurance company. This Model Risk Policy is set out in full here and was presented to the IFoA CRO Group by members of the Working Party (N. Morjaria, L. Witts and C. Hughes) on 14 November 2016. Section 4 focusses on some of these specific areas in further detail.
Model Risk Policy
1. Model Risk Identification
Policy
All models in use must be identified and recorded in the model inventory system, to ensure they are subject to appropriate controls in line with the Model Risk Policy.
Accountabilities
Chief Risk Officers (CROs), Model Owners
Requirements
-
■ CROs are responsible for ensuring that all models used by their legal entity are identified, and recorded in the model inventory system.
-
■ The Federal Reserve’s Model Definition should be used for model identification – a model is a quantitative method, system or approach that produces a prediction or estimate, which directly impacts business, account or transaction-level decisions. The prediction or estimate can take multiple forms (i.e. forecast, distribution, valuation, etc.) and by their nature are subject to error or uncertainty which introduces risk to the organisation.
-
■ Model Owners are responsible for ensuring the information in respect of their models in the model inventory system is complete, up-to-date and accurate.
-
■ For each model, specific mandatory fields (e.g. model type, usage(s), Owner, User(s), risk control level, description) must be completed in the model inventory system.
-
■ Information should be recorded in the model inventory system at a model usage level, unless it is deemed appropriate by the Model Owner to record specific model components separately, for example, for external models
Practical Considerations
-
■ There is a risk, given the definition of a model, that the number of models recorded in the inventory would run to hundreds/thousands, thereby providing a significant overhead for maintenance and also adding limited value to the organisation.
-
■ Therefore, by focussing on usage level, the number of records is likely to be more manageable, and also aligns well with validation (i.e. assessment of models for fitness for purpose). It is expected that entities will have records on the inventory system for at least the following usages (where applicable):
-
- Regulatory Reserves/Capital
-
- Embedded Value
-
- Stress Testing
-
- Economic Capital
-
- Product/Reinsurance Pricing
-
- Business Planning/Forecasting
-
- Asset–Liability Management
-
- Customer Benefit illustration
-
- Discretionary benefits
-
-
■ All CROs and Model Owners (and relevant delegates) will require access and training on the model inventory system.
-
■ It will also be necessary to have central inventory expert contacts to provide support to Model Owners and Users.
-
■ There may need to be a regular (e.g. annual) attestation process from CROs and Model Owners around the completeness and accuracy of the inventory.
2. Assignment of Key Model Roles
Policy
Appropriate individuals or groups must be assigned to each of the key model governance roles for all models.
Accountabilities
CROs
Requirements
CROs are responsible for ensuring that for all models appropriate individuals or groups are assigned to each of the following key roles:
-
■ Model Owners: responsible for maintenance of information in the model inventory system, model risk prioritisation (MRP), compliance with model risk control standards, sign-off of model developments/changes, model monitoring, liaising with the Model Reviewer, submitting Residual Risk Assessment
-
■ Model Users: responsible for the model being used appropriately and only using the model after approval by Model Approver.
-
■ Model Reviewer: responsible for performing independent validation/reviews of models.
-
■ Model Approver: responsible for reviewing Residual Risk Assessments and approving the use (or limited use) of the model.
Practical Considerations
-
■ Key roles may typically be as follows:
-
■ Model Owners: Chief Actuary
-
■ Model Users: Chief Financial Officers (CFOs), CROs
-
■ Model Reviewer: Model Validation team/External reviewers for High and Medium Control level models; less robust independence required for Basic Control level models.
-
■ Model Approver: Dependent on the Risk Control Level of the Model, the Model Approver differs:
-
■ High control level models require Group Model Risk Committee approval (and pre-approval from the entity Model Risk Committee where relevant).
-
■ Medium control level models require entity Model Risk Committee approval.
-
■ Basic control level models require Model Owner approval.
-
-
-
■ However, given the seniority of the Chief Actuary, CROs and CFOs, they are likely to delegate day-to-day activities to relevant team members.
-
■ There is a significant cost (either internal or external) associated with Independent Model Validation/ Review, therefore the frequency and level of independence of review (and therefore the cost) will vary will the Risk Control level of the model (see later section on Model Review).
3. MRP
Policy
Model Owners must ensure that MRP Grades and Risk Control levels are determined for each of their models and recorded in the model inventory system, to enable management to prioritise model governance, activities and resources according to the materiality of models and their potential risks to the business.
Accountabilities
Model Owners
Requirements
-
■ Model Owners are responsible for determining a MRP Grade and Risk Control Level for all of their models.
-
■ The MRP Grade should be determined by assessing the model against centrally specified quantitative model materiality thresholds. Subsequently a Risk Control Level (High/Medium/Basic) should be determined based on the materiality of the model, the extent of its regulatory scrutiny, and its strategic importance within the group.
-
■ Model Owners should review MRP Grades and Risk Control Levels assigned to their models at least annually. The Model Approver should be notified of any changes and the model inventory system updated accordingly.
Practical Considerations
-
■ A generic tool can easily be developed to support the MRP and Risk Control Level assessment process.
-
■ The Risk Control Level drives the components of the Policy which need to be followed (in particular, the level of documentation and validation required). It is therefore key to ensure that the distribution of models between Risk Control Levels is appropriate and aligns to available resourcing.
4. Model Risk Control Standards
Policy
Controls should be applied when developing and operating models to mitigate model risk. The level of controls will depend on the Risk Control Level of the model.
Accountabilities
Model Owners, Model Users
Requirements
-
■ Model Documentation: Model Owners must ensure that their models are properly documented and that the level of documentation is commensurate with the nature, scale and complexity of the model
-
■ Data Quality: Data used in model calculations must be fit for the purpose for which it is being used.
-
■ Model Methodology and Assumptions: The methodologies and assumptions used in models must be based on robust and appropriate techniques and data.
-
■ Expert Judgements are robust, transparent and open to challenge, so that Model Users can place reliance on the judgements and be aware of their impacts on the model outputs.
-
■ Model Limitations are understood by Model Users to avoid misuse of the model or model output.
-
■ Model Implementation and Use: Model Users must mitigate the risk of incorrect or inappropriate use of models or model outputs.
-
■ Model Changes are appropriately tested and signed-off before being implemented.
-
■ External Models must comply with the same model risk control standards as internally developed models.
Practical Considerations
-
■ Basic Control level models only require simple information, covering:
-
■ Model description;
-
■ Summary of model methodology and assumptions;
-
■ Key expert judgements and limitations underlying the model, and supporting rationale for these;
-
■ Data Flow Charts; and
-
■ Logs of model changes.
All of this information can be included in a single document.
-
-
■ There may be separate more detailed standards on setting best estimate assumptions.
-
■ Companies have found it helpful to develop a suite of template documents for items such as expert judgement log, limitations log, etc.
-
■ These standards are generally cut-down/simplified versions of the Solvency II internal model standards made proportionate for the business, so should be familiar for many companies.
5. Model Monitoring
Policy
On-going monitoring must be carried out to evaluate whether changes in business practices, internal or external factors necessitate adjustment, redevelopment or replacement of a model.
Accountabilities
Model Owners, CROs
Requirements
-
■ Model Owners are responsible for assessing that a model remains fit for its purpose (methodologies, assumptions, expert judgements and limitations) on an annual basis.
-
■ For High and Medium Control Level models, model monitoring should assess whether:
-
(1) the model methodology is robust;
-
(2) assumptions, parameters, data, limitations and expert judgements are appropriate and relevant;
-
(3) technical documentation is complete and up-to-date;
-
(4) model process documentation is complete and up-to-date;
-
(5) change control processes have been followed;
-
(6) controls for the model are operating effectively;
-
(7) the model complies with the Model Risk Policy and regulatory requirements.
-
-
■ All findings should be reported to the Model User(s) and Model Approver.
-
■ The performance of financial and regulatory reporting models should be assessed on a quarterly basis by analysing the deviation between actual experience and the expected results.
-
■ CROs must inform Model Owners when there is a change in risk profile of the business.
Practical Considerations
-
■ This may be a new process for some companies.
-
■ Model Owners will likely need to attest that the various components of Model Monitoring have been adhered to. This attestation could be made within the entity Model Risk Committee and within any output reports for models.
-
■ More generally, it would be expected that items such as Expert Judgements and Limitations are tabled for discussion at entity Model Risk Committees to ensure they remain appropriate and relevant.
-
■ A Risk Acceptance process will be required for any findings made during the assessment process (see section 7 of the Policy on Model Risk Acceptance).
-
■ To support the above, a Model Risk Committee template may be helpful to ensure the relevant items are covered regularly.
6. Model Validation/Review
Policy
Models must be subject to validation/review proportionate to their Risk Control level, to assess whether the model is fit-for-purpose and in line with its design objectives and business uses.
Accountabilities
Model Owners, Model Reviewers
Requirements
-
■ Model Owners must include all open findings identified by the Model Reviewer in the Limitations log and Residual Risk Assessment.
-
■ Models with High Risk Control levels require annual review by the Model Reviewer.
-
■ Models with Medium Risk Control levels require periodic review by the Model Reviewer. Model Owners to determine the frequency in conjunction with the Group Model Risk Committee.
-
■ Models with Basic Risk Control levels may not need additional validation / review over and above regular monitoring; the Model Owner should determine whether this is necessary and if so coordinate the appropriate Model Reviewer.
-
■ Model Owners must provide a formal sign-off of their models prior to submission to the Model Reviewer.
-
■ Where the Model Reviewer identifies high risk findings, the Model Owner must develop a remediation plan to address these findings and agree that the proposed remediation actions are appropriate with the Model Reviewer.
-
■ The Risk Acceptance Process outlined in the next section of this Policy must be followed prior to implementation/use of the model.
Practical Considerations
-
■ Annual reviews of High Risk Control level models may seem very frequent; however, these are likely to focus on key changes since previous reviews. (This also aligns to regulatory expectations around the most significant models.)
-
■ The Chair (or relevant deputy) of the Group Model Risk Committee and Model Owners will have to collectively go through a process to determine review frequencies for Medium Control Level models based on underlying risks and available resources.
-
■ For High Risk Control level models, Model Reviewer Reports should be reported to the Group Model Risk Committee. Subsequently remediation plans must also be presented for high risk findings.
-
■ For Medium Risk Control level models, Model Reviewer Reports should be reported to the entity Model Risk Committee; as well as subsequent remediation plans in respect of high risk findings identified.
-
■ Where the Model Reviewer is internal, there will need to be separate detailed standards for the Model Reviewer to follow; where the Model Reviewer is external, the review scope should be set by the CRO (or relevant Deputy).
7. Model Risk Acceptance
Policy
Model Owners must assess the residual model risks resulting from issues identified with their models, and submit their assessments to the relevant Model Approvers for consideration for risk acceptance and approval of the use and/or limitation of use of the model, to ensure that risks arising from models are understood, mitigated and accepted prior to their usage or continued usage.
Accountabilities
Model Owners, Model Users, Model Approvers
Requirements
-
■ Model Owners are responsible for performing a Residual Model Risk Assessment and submitting this to the Model Approver at least annually.
-
■ This is an assessment of the remaining model risk after controls and any mitigation has been applied.
-
■ The Model Approver should review the Residual Model Risk Assessment and formally risk accept residual risks where deemed appropriate, in line with the relevant entity’s (Model) risk appetite.
-
■ Model Users should only use models approved by the Model Approver.
Practical Considerations
-
■ This concept is new and likely to take some time to embed. Logs of outstanding issues will need to be approved by the relevant Model Approver (Committee or individual depending on Risk Control Level).
-
■ The Residual Risk Assessment should incorporate all Medium and High Risk findings identified through Model Monitoring, Model Validation/Review, Internal Audit, and External Audit.
-
■ A Residual Risk Assessment Log should be maintained (the Working Party will develop a template). This should capture:
-
- Model name;
-
- Summary of the issues/findings;
-
- Risks (before mitigation) presented by the issues/findings;
-
- Action Plan (remediation plans and mitigating controls);
-
- Action Owner(s);
-
- Residual Risk Rating; Residual Risks to be accepted; and
-
- Reason(s) why the Residual Risks should be accepted.
-