A large change in the U.S. food system in recent decades is the growth of the food away from home (FAFH) sector. Food away from home accounted for 25.9 percent of total household food spending in 1970. By 2012, the share had risen to 43.1percent.Footnote 1 Because FAFH is more expensive, its quantity share is smaller, but it nevertheless accounts for a substantial portion of food consumption. The impact of this change on diet has received considerable attention, with many studies finding that the nutritional quality of FAFH is lower than that of food consumed at home (Lin and Frazao Reference Lin and Frazao1997; McCrory et al. Reference McCrory, Fuss, Hays, Vinken, Greenberg and Roberts1999; Carlson and Gerrior Reference Carlson and Gerrior2006; Binkley Reference Binkley2008; Todd et al. Reference Todd, Mancino and Lin2010; Auchincloss et al. Reference Auchincloss, Leonberg, Glanz, Belitz, Ricchezza and Jervis2014; Nguyen and Powell Reference Nguyen and Powell2014).
Nearly all studies dealing with the effects of FAFH on diets have concentrated on nutrient differences with food at home (FAH). In this study, we consider the foods themselves. If individuals obtain different nutrients in restaurants than when at home, either they are eating different foods, or similar foods prepared in different ways, or both. Obtaining more precise measures of the difference in foods eaten in restaurants vis-à-vis at home can help explain some of the changes in consumption of basic foods in the recent past. Such changes are often attributed to growing concerns with nutrition, and there is evidence supporting this (Brown and Schrader Reference Brown and Schrader1990). An example is chicken, a relatively healthy meat, consumption of which more than doubled between 1970 and 2012, from 22.4 to 46.2 pounds per capita.Footnote 2 But over the same period, per capita consumption of milk, a relatively healthy dairy product, fell from 31.1 gallons to 19.6 gallons, while consumption of cheese, a less healthy dairy food, rose from 18.9 pounds to 35.5 pounds per person. Meanwhile consumption of soft drinks more than doubled, growing from 24.3 to 46.5 (2003) gallons per capita. Although these changes are nutritionally inconsistent, they are consistent with the growth of FAFH: they may be at least in part a consequence of these foods’ being featured, or in the case of milk not featured, on restaurant menus. Without full knowledge of such trends, it is difficult to design effective policies to improve diets.
Furthermore, such changes may have had impacts on agricultural production and the structure of consumer demand. This has received relatively little attention. It would seem that a change in the structure of the food delivery system as dramatic as the rise of the restaurant sector would have effects beyond nutrition changes. If so, market studies and analyses of consumer demand may need to take FAFH into account. This is especially the case with demand studies, many of which have been concerned with structural change (Eales and Unnevehr Reference Eales and Unnevehr1988; Moschini and Meilke Reference Moschini and Meilke2004).Footnote 3
In this study, we address both aspects of the growth of FAFH by focusing on what can be termed the commodity composition of the American diet: its constituent basic foods. Using a large sample of FAFH consumers, we compare the components of their FAH and FAFH diets and estimate which commodities have been most affected by FAFH, with some estimation of magnitudes. We use a measure based on nutrient profiling to examine the overall nutritional quality of the food categories, and separately consider energy density. This includes nutrition differences within categories, which depend on preparation methods and the dishes in which foods are used.
We also compare the home diets of those with and without FAFH in the sample. This reveals strong evidence that more frequent consumers of FAFH have less nutritious diets at home, a finding similar to that of Mancino et al (Reference Mancino, Todd and Lin2009). This suggests that sample selection may partly explain the observed lower diet quality of restaurant dining. It also demonstrates the importance of the standard of comparison when assessing the effect of FAFH on dietary outcomes.
Data
We use two data sets. The first is the National Health and Nutrition Examination Survey (NHANES). Conducted every 2 years, NHANES involves approximately 10,000 respondents, most of whom supply 2 nonconsecutive days of detailed dietary intake data, including where food was obtained. (See www.cdc.gov/nchs/nhanes.htm for full details concerning the NHANES data.) Only respondents with 2 days of data are included in the analysis. We focus on NHANES 2007–8, although data from earlier NHANES are included for comparison. We consider adults (age > 18) and children (3 < age < 19) with 2007–8 sample sizes of 5988 and 2862, respectively. Food away from home is defined as all food obtained from full-service and fast food restaurant, cafeterias, and bars; food consumed at home is all food obtained from grocery stores and other retail establishments. Because one of our purposes is to compare FAH and FAFH, only foods from these two sources are included.Footnote 4
The second data set is the USDA Food Intakes Converted to Retail Commodities Database (FICRCD) (Bowman et al. Reference Bowman, Martin, Carlson, Clemens, Lin and Moshfegh2013). This is available for several years, the last being 2007–8, which is the reason for our focus on that period. FICRCD is designed specifically to convert intake data from NHANES and similar sources to retail equivalents. Thus, a final food as consumed—a serving of spaghetti, a hamburger, an apple, etc. is disaggregated into its basic food components—grain, beef, tomatoes, etc. There are 65 categories, composed of several composites and 55 specific commodities. Our focus is the latter, augmenting the data with a category for soft drinks, which is not included in FICRCD.
Commodity Patterns and Effect of FAFH
We begin with a depiction of consumption across detailed food categories by the full sample for 2007–8, and an assessment of how FAFH may have affected commodity output. For comparison, the corresponding information for 2005–6 appears in appendix Table 1, which involves a completely different sample, because NHANES is not a panel. The earlier data will not be discussed, because all patterns are quite similar across the years. This is important because marked differences in diet from year to year would make it difficult to say anything meaningful about patterns in the American diet.
Table 1 has average daily consumption of the individual foods in our data, in retail equivalent grams, the percent consumed as FAFH, the FAFH intensity, and an estimate of the impact of FAFH on the food system. FAFH intensity is a measure of the food's prevalence in the FAFH diet relative to its importance in the home diet. We define the intensity of A as the percent of FAFH made up of A divided by its corresponding percent for FAH. A food with high intensity (>1) is more likely to be chosen when dining out than when at home.
Table 1. Commodity Patterns and FAFH Effects 2007–8
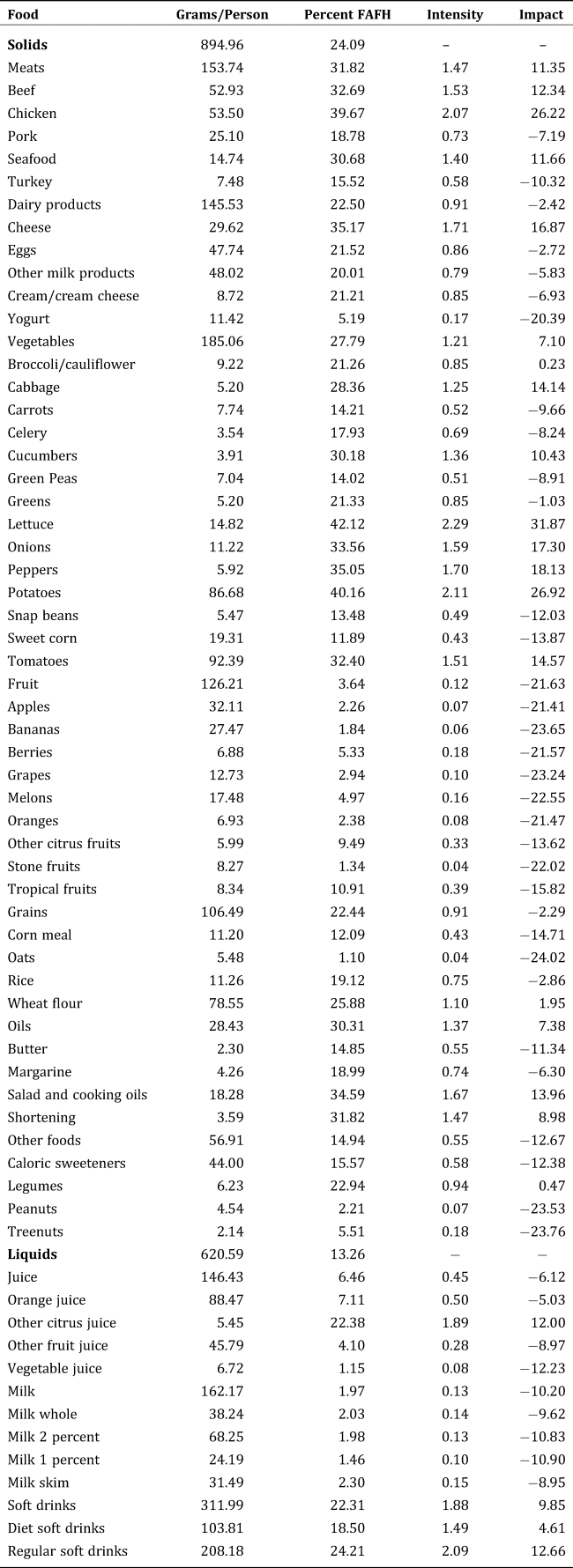
The impact is our estimate of how FAFH has affected the consumption, and hence production, of the foods in the table. This requires an estimate of what the diet of current FAFH consumers would have been in the absence of the restaurant sector, a counterfactual case. To obtain this, it was necessary to make two assumptions. One was that, for each consumer, total grams consumed without FAFH would be the same as they are currently consuming. This can be questioned. Total consumption may have been smaller, because people tend to eat more when dining out, although they also tend to compensate for this by reduced home consumption (Binkley Reference Binkley2008). In any case an assumption that total grams would be the same is unlikely to be a serious departure from reality.
The second assumption deals with how the FAFH transferred to FAH would be allocated among commodities. Consider one commodity x, and let x0 and x1 be the grams of x consumed with and without FAFH, respectively. That is, x1 is current consumption, x0 is what consumption of x would be if FAFH did not exist. We express these as


where A is total home grams, B is total grams currently eaten in restaurants, α is the proportion of A allocated to x, θ is the corresponding proportion of B when it is consumed as FAFH, and δ is the proportion when B becomes home consumption.Footnote 5
The problem is determining the value of δ. There are two obvious choices: δ is either θ or α. If δ = θ , the “new” home consumption is the same as when consumed in restaurants; if δ = α, it takes on the proportion of existing home consumption. It is almost surely the case that these bound the possibilities. For example, if before the elimination of FAFH x is 2 percent of A and 5 percent of B, it is not likely that 8 percent of B will be devoted to x when it becomes FAH. Furthermore, because with δ= θ the elimination of FAFH brings no change in the consumption of x, the fact that δ is within the interval bounded by θ and α implies that the maximum change, increase or decrease, occurs if δ=α. Lacking any reason to choose a value in the interval,Footnote 6 it thus makes sense to choose δ=α for the computation of the change brought by FAFH. This gives us an estimate of the bounds on the likely change. It ranges between zero, when δ= θ, and the maximum, which occurs if δ = α, which is what is presented in the table. Importantly, our method should provide an accurate delineation of which food commodities have been most affected by the growth of FAFH.
Thus, for each current FAFH consumer, we reallocated their FAFH consumption in accordance with the pattern of their current FAH consumption. The counterfactual estimate of total consumption of each commodity was obtained by summing over these consumers, and adding this to total current FAH consumption by everyone in the sample. To obtain the impact of FAFH, we then computed the percent change for each food in Table 1, using the counterfactual as the base, and the change as (current actual consumption) minus (counterfactual consumption).
The table is divided into subcategories of liquid and solid foods. Computations for these that involved percents were done separately. This was done because liquids tend to be heavier than solids. Computing percents with all foods combined would exaggerate the importance of liquids in the diet. All computations in the paper maintained this separation.
Among the solid foods, the results show some large differences between what is eaten at home and in restaurants, particularly for meats and fruits and vegetables. Food away from home is meat-intensive: when dining out, meat is eaten approximately 50 percent more frequently than when at home. Chicken is particularly FAFH-intensive, being twice as likely to be eaten when dining out. 41 percent of total chicken consumption occurs in restaurants. Pork is the only meat with an intensity less than 1. Correspondingly, according to our estimates, meat output has risen by as much as 10 percent due to FAFH, with chicken 25 percent above what it would have been. Only pork has not gained.
Among solid dairy products, only cheese is FAFH intensive, reflecting its prevalence in pizza and fast food sandwiches. As a result, output of cheese has risen sharply. Yogurt and milk and cream products (ice cream, cream cheese, baked goods, etc.) are consumed more at home. Yogurt has been particularly affected by FAFH, with output estimated to be as much as 20 percent lower than it would have been without FAFH.
As a category, vegetables are FAFH intense. This might seem surprising, but it is primarily due to the intensity of tomatoes and especially potatoes, which have large shares of the vegetable category. In view of the prevalence of French fries on restaurant menus, the intensity of potatoes is not surprising. FAFH is estimated to have led to a substantial increase in potato production. For tomatoes, the majority of retail equivalents are from processed tomato products: raw tomatoes comprised 9 percent of the total in the 2007–8 data. Much of the remaining 90 percent is made up of pizza and spaghetti sauce, salsa, and catsup, major ingredients in numerous restaurant items. Producing a pound of such products requires considerably more than a pound of tomatoes, causing production to increase. The other vegetables with high FAFH intensities are primarily salad vegetables, especially lettuce. Indeed, results indicate that the single largest change induced by FAFH is the increase in lettuce production.
For fruit, the situation is very different. Diners at FAFH establishments eat little if any fruit. As a consequence, fruit consumption is estimated to be lower, perhaps substantially lower, because of FAFH, a reduction fairly evenly distributed across fruit types. These numbers provide strong evidence that FAFH is an important reason why Americans fall short of recommended fruit intakes.
As a group, grains have been little affected. The exceptions are declines in cornmeal and especially oats, a food with a miniscule FAFH intensity. The primary use of oats is in cereals, a category with declining consumption overall.Footnote 7 The negative effect of FAFH on oats suggests a contributing cause of this is the growth of breakfasts in restaurants, especially fast food outlets, where breakfast cereal is not featured.
For the remaining solid categories, notable differences between home and away are the high FAFH intensity of fats and oils, and a low intensity of added sugars (which does not include sugars in soft drinks). The first is due to oils used in food preparation, a reflection of the prevalence of fried foods on restaurant menus. The latter is most likely due to the relative absence of cakes, cookies, and similar confections, as well as candy and sweetened cereals, on these menus. The final solid foods in the table are nuts, a small category by weight, but one of the foods most negatively affected by FAFH. According to the data, nuts are mostly consumed in the form of snacks or peanut butter sandwiches, items seldom found in restaurants.
For liquids, FAH-FAFH differences are sharp. Fruit and vegetable juice and especially milk do not comprise a significant component of FAFH consumption. The intensities for all types of milk are very low and of similar magnitude. FAFH is estimated to have reduced their consumption substantially, by as much as 10 percent. As seen above, most dairy products have also been negatively impacted. From this, it would seem that milk producers have suffered because of the growth of the restaurant industry. However, this is likely counterbalanced by the large increase in cheese output, which requires approximately 10 times its weight in milk.
Soft drinks, especially regular soft drinks, are very FAFH intensive. With the advent of FAFH has come a large increase in total soft drink consumption. As noted above, in the period from 1970 to 2000, the period of intense growth in FAFH, milk consumption declined by a third while soft drinks nearly doubled. The results in the table suggest that FAFH is indeed likely have played an important role in these changes.
From these results, it is justified to conclude that food away from home has had discernible impacts on the production and consumption of specific foods in the US. It is unlikely that these changes result from spontaneous changes in consumer tastes, and that they would have occurred with or without FAFH. They are surely at least partly attributable to what amounts to a new manner of providing foods, one that changes the relative cost and ease of obtaining them. For example, commercial food establishments are likely to have a comparative advantage in foods requiring more preparation, such as fried chicken and French fries, so that the relative cost, in terms of time as well as money, are likely to differ from costs of home preparation.
In any case, the magnitude of the changes identified here suggests that the growth of the restaurant sector is a major structural change, which may need consideration when examining other aspects of the food system. A salient example is food demand studies, especially the demand for meat. Several studies have considered the possibility of structural change in meat demand (Eales and Unnevehr Reference Eales and Unnevehr1988), but seldom is FAFH deemed an important aspect of this change, and it has not often been explicitly considered (an exception is Tonsor et al. Reference Tonsor, Mintert and Schroeder2010). Results in Table 1 suggest this may be a serious omission.
Food Patterns by Source and by Groups
Table 2 has 2007–8 information for a less detailed set of foods but more detailed groups of consumers. The groups are children and adults, further broken into those without and with FAFH during the sample period. For convenience we will call these “group 1” and “group 2,” respectively. While having no FAFH during the period does not mean group 1 individuals never dine out, we can say that anyone who in fact does not is necessarily in group 1. It is also more likely to contain those who do so with less frequency.Footnote 8
Table 2. Percentage Allocation across Foods, by Groups, 2007–8

Significance: *** 0.01; ** 0.05; * 0.10.

Significance: *** 0.01; ** 0.05; * 0.10.
It is important to compare these groups because of the possibility that part of the reason people obtain less healthy food in restaurants is that those with a natural preference for such food (and thus would choose it in any case) are more likely to dine out (Mancino et al. Reference Mancino, Todd and Lin2009). Furthermore, people who dine out more frequently tend to have different characteristics from people who do not (Powell et al. Reference Powell, Nguyen and Han2012). They may thus have different eating preferences and different concerns with nutrition (Stewart et al. Reference Stewart, Blisard, Joliffe and Bhuyan2002; Binkley Reference Binkley2006).
That these two sets of consumers do have different characteristics is evident from Table 3, which has averages for selected demographics of each of them. Those with FAFH tend to be younger, have higher incomes, and to spend more time working. These are factors likely to increase FAFH. They also have a greater proportion of males and are somewhat better educated. We investigate the effect of these differences below.
Table 3. Demographic Characteristic of Sample

Table 2 shows the average percentage distribution across the foods.Footnote 9 It includes home consumption for both groups (columns 1 and 3), and group 2's FAFH consumption (column 5). Columns 2 and 4 have the t-values for tests of differences between these, as shown in column headings.
For home consumption, the patterns for solid foods are broadly similar between the groups, for both children and adults. There are, however, some notable specific differences. The most salient involves sweeteners, for which group 2 has significantly greater consumption in both cases. The same applies to fats and oils, but only for adults. These suggest healthier choices by group 1. Most of the remaining significant differences also suggest their food choices are more concentrated in healthier categories: for adults, more vegetables and less beef; and more seafood, legumes, and tomatoes for children. The one exception is that group 2 consumes a greater percentage of fruit, which for children is highly significant and quantitatively large.
The most striking difference between the home consumption of the groups involves liquids. Among the three major liquids we examine, group 2 has a much larger percentage of soft drinks in their home consumption, with a corresponding lower percentage of the others. Milk, in particular, is less important for this group. The differences for these two are highly significant for both adults and children. Juice does not greatly differ between the groups, although it is weakly significant for adults.
Column 5 in the table shows the distribution of away from home consumption, which only applies to group 2. The t-values in column 4 test for differences with their home distribution. For both adults and children, the large majority, 14 of the 20 categories, are highly significant. It is clear that the commodity composition differs for the two food sources. As would be expected, the strength of the differences reflects the FAFH intensities in Table 1,Footnote 10 with very pronounced differences for fruit and milk, important components of home dining but largely ignored when dining out, and much greater percentages of poultry, beef, cheese, and soft drinks when away from home. Soft drinks dominate the liquids group. For adults, this is somewhat deceptive, for the figures do not include coffee or alcoholic beverages, which for adults are relatively important in restaurant meals. But the table shows that FAFH is associated with the heavy consumption of soft drinks by children.
Nutrition Differences
In this section, the nutrition for the food types in Table 2 is examined. To do, so we make use of nutrient profiling, a method designed to assign nutrient scores to individual foods (Scarborough et al. Reference Scarborough, Rayner and Stockley2007; Chiuve et al. Reference Chiuve, Sampson and Willett2011). A nutrient profile is typically a linear combination of nutrients, with models differing by the nutrients involved, how they are weighted, and the criterion on which they are based. We use a profile model developed by Arsenault et al. (Reference Arsenault, Fulgoni and Muth2012), which is based on its relation to the USDA Healthy Eating Index (HEI), the standard measure of the quality of the daily diet (Guenther, Reedy, and Krebs-Smith Reference Guenther, Reedy and Krebs-Smith2008). This has a certain appeal here, because we are interested in rating portions of diets, not just individual foods.
Using NHANES 2005–6 data, they summed individual intakes of 13 desirable and 3 undesirable nutrients for each respondent. They then constructed all possible combinations of these nutrients and regressed them on the individual's HEI. The best model was chosen based on contribution to goodness of fit, in which additional nutrients made no significant contribution. It is a weighted sum of protein, fiber, calcium, vitamin C, and unsaturated fat, all with positive coefficients (indicating a positive relation with HEI), and saturated fat, added sugar, and sodium, with negative coefficients (indicating a negative relation). The equation is used to assign a value to individual foods, with higher values indicating more desirable nutrients. The value is not bounded by specific numbers.Footnote 11 It is essentially a relative measure, so that of two foods, that with the higher index is considered to have higher nutritional content.
We calculated a measure for each respondent for each category by taking weighted averages of the indexes of component individual foods consumed by the respondent. The weight is the retail equivalent grams consumed for each food. These were averaged across respondents. The value obtained for a category can be negative, due to the negative components in the index. The category indexes depend not only on the nutritional quality of the commodity involved but also its various preparation methods and its use in various dishes.
Because the index does not provide a measure of calories,Footnote 12 and given their importance, we also calculated the energy density (calories/grams) of the food categories, in the same manner as the index calculation, that is, by taking (retail equivalent) weighted averages of individual foods within the categories. Since lower values indicate lower calorie density, and given the rising obesity in the U.S. population, most would regard low values of this measure as healthier.
The nutrition and density measures are presented in the left and right halves of Table 4. In each case, the arrangement is the same as that in Table 2. Beginning with nutrition, for adults the table shows substantial differences in the nutritional quality of home consumption of the two groups. We emphasize that in this comparison the nutrition for group 2 involves only their home consumption. The t-values test the significance of the difference, calculated as (Group 1)-(Group 2). For the nutrition index most are positive, which suggests that those with no FAFH during the sample period had a healthier home diet. Many are highly significant (p > 0.05). This is true for 9 of the 20 individual categories and both aggregate categories. There are few negative differences, and none of significance.
Table 4. Nutrition and Energy Density Comparison, 2007

Significance: *** 0.01; ** 0.05; * 0.10.

Significance: *** 0.01; ** 0.05; * 0.10.
The energy densities are consistent with the results for nutrition. In this case, negative t-values indicate lower density and hence healthier diets, for the respondents with no FAFH. In 10 cases they are significantly negative, with only one category, nuts, being significantly positive. (The very high density for nuts for both groups results because they are frequently eaten “straight,” which, for example, oils virtually never are.) The aggregate density measure for solid foods is also significantly negative, with a magnitude suggesting 20 percent, fewer calories per gram for group 1. The corresponding measure for liquids, however, is positive and significant, even though the individual differences are small or negative. This seeming inconsistency is due to the perhaps surprising fact that of the three liquid categories, soft drinks has the lowest calorie density (in part due to diet drinks), and group 1 has a much lower proportion of soft drinks in their consumption.Footnote 13 Reducing the proportion of the least dense component increases the density of the total. In this case, it also increases nutrition.
For children, the differences in nutrition measures are less pronounced. Although the majority are positive, only grain products is highly significant, and neither of the category aggregates is significant. The results are somewhat stronger for energy density. They indicate that the home diets of children without FAFH are of lower density, with many categories significantly lower. The difference for aggregated solid foods is significantly negative and suggests an even larger difference in calorie density than that for adults. However, liquids show no differences of any consequence.
It is clear that there is a difference in the nutritional quality of home diets for the two sample groups. Those who did not have FAFH during the sample period have a healthier diet at home, for both children and adults. This is evidence that selection may be important when it comes to nutritional questions associated with restaurant food. People with less concern about the quality of their diet may be more inclined to consume FAFH. As noted above, indications of this have appeared in previous research. It is considered in the final section of the paper.
The second comparison in Table 4 is between FAH and FAFH for those who ate in restaurants during the sample period. Thus the “home” in this case only involves group 2. The t-values test whether the difference FAH-FAFH is zero, so positive values for nutrition and negative values for density indicate healthier home diets. Results for adults and children are nearly identical in terms of sign of the t-statistics. For nutrition, the majority are positive, suggesting better home diets. For adults they are somewhat stronger statistically. For example, all but four of the differences in the nutrition measure are highly significant (p < 0.05), while approximately half of those for children are. Of the significantly negative t-values, a notable case is cheese, which reflects the use of cheese with less saturated fat for items like pizza, a staple in FAFH. The others are less explainable, but in any case the magnitude of their differences is generally smaller than for those that are positive. As a result, the differences for both aggregate solid and liquid categories are positive and highly significant, for both children and adults.
The results for energy density are similar: the majority of the t-values are large in absolute value and the majority of these are negative. This especially applies to meats, whose methods of preparation in restaurants tend to increase energy density. Again, however, there are several categories having positive differences and, hence, lower density in restaurants. This includes cheese, for reasons just given. For grain products, restaurant consumption is mostly breads and pasta; home consumption includes a greater amount of energy dense items such as cakes and cookies. A perhaps surprising outcome for energy involves potatoes, which is shown to have higher density at home than in restaurants, significantly so for children. This is due to the fact that the potato product with greatest energy density is not French fries but potato chips, which are heavily consumed by children, and primarily at home. Note that both grain products and potatoes are estimated to have a higher nutrition index when consumed at home, which shows that high density need not suggest low nutrition.
The aggregate liquid category also indicates lower energy density for FAFH. For children, it is highly significant, despite the fact that all three components are significant in the opposite direction. This again reflects the phenomenon noted above, the relatively large share held by soft drinks in restaurant consumption.
Overall, then, food away from home is less healthy than food consumed at home. This arises due to the mix of foods used and how they are used: that is, what is consumed and how it is prepared. For liquids it is mostly the first of these, primarily due to the very large change in the share of soft drinks when away from home. For solid foods both effects are present. To investigate their relative importance, we calculated the FAH-FAFH difference in the aggregate measures by (a) retaining the FAH food mix and applying the actual FAFH nutrition measures; and (b) using the actual FAFH food mix but applying the nutrition measures for FAH. With method (a) nutrition fell from 22.97 (Table 4) to 15.69; with (b) it declined less, to 19.98. The implication is that lower nutrition is a result of both the commodity inputs used by restaurants and how they are processed, with the latter somewhat more important.
Are FAFH Consumers Different?
The results support the well-known result that FAFH is less healthful than food prepared at home. But we also found that those with FAFH during the sample period had lower home nutrition than those in the sample with no FAFH. Indeed, there are many cases in Table 4 for which the home food differences between the two groups are larger than the FAH-FAFH differences.
There are two questions regarding this result. One is the extent to which it is sample specific. The second is whether it simply reflects the different demographic characteristic shown in Table 3. These characteristics are likely to affect both the propensity to dine out and individual dietary choice. For example, older consumers are known to have healthier diets, and are also likely to dine out less (Binkley Reference Binkley2008). Then the nutrition differences between groups 1 and 2 may simply be due to the greater proportion of older consumers in group 1.
To consider sample specificity, we repeated the nutrition analysis for adults using the 2005–6 NHANES data. The results are in Appendix Table 2. We will not discuss these beyond stating that they are very similar to those for 2007–8. Corresponding columns for the nutrition and density measures are highly correlated, so that both sets of results would lead to similar conclusions.
To account for the possibility of sample selection, we used a propensity score matching procedure to obtain a “matched” sample of home diets. We used a logit model to predict the probability of dining out based on the demographics in Table 3. Members of group 1 were matched with group 2 members on the basis of predicted probabilities (Rosenbaum and Rubin, Reference Rosenbaum and Rubin1984). This was confined to the sample of adults, because children are less likely to decide where they will eat. Matching was based on pairing observations with estimated probabilities differing by no more than .001, using nearest neighbor without replacement. The matched sample had 1728 observations each from group 1 and group 2. Thus we were able to match all but 273 of the original members of group 1 with someone in group 2. This reflects that group 2 is much larger (n=3277).
The results are in Table 5. Comparing these to the corresponding information in Table 4 shows little difference. We expect this for group 1, because the matched sample contains all but 100 of the original sample. But it is also true for the second group, which has slightly more than one half of the original group 2 sample, those most similar to group 1 in terms of demographic makeup. This is evidence that differences in nutrition choice by the FAFH sample go beyond differences in demographics. They may have preferences for foods of lower nutrition. If so, part of the reason that food in restaurants tends to be lower in nutrition is that people who like to dine out are more willing to sacrifice nutrition to obtain foods with greater palatability.
Table 5. Results for Matched Sample

Significance: *** 0.01; ** 0.05; * 0.10.
Summary and Concluding Remarks
It is well known that food away from home differs from food consumed at home in terms of nutritional quality. Numerous studies have found FAFH to be of lower nutrition, mostly by comparing nutrient and calorie contents. In this study we have examined this question from more of a commodity perspective, focusing on differences in consumption across broad food categories, as well as the nutrition within these categories. This permits an assessment of how the growth of the restaurant sector has changed the structure of the American diet, as well as its impact on agriculture.
Using data from the 2007–8 National Health and Nutrition Examination Survey, we found large differences in the types of foods consumed at home and in restaurants. For example, meat and soft drinks are relatively more important in away from home meals, while fruit and milk are seldom consumed. Such differences are one reason for nutrition differences. We verified this by using a nutrient profiling model, which is a method to assign values to individual foods. This showed that FAFH is more concentrated in less healthful food types. It also showed that within food categories, individual foods in restaurants tend to be less healthful and more energy dense. This reflects differences in dishes and methods of preparation, such as frying instead of baking, that tend to be featured on restaurant menus.
Large differences in consumption of basic foods suggest differences in the production sector. We investigated this counterfactually by comparing current consumption with an estimate of what consumption would have been with no restaurant sector. We found some large effects on food production. FAFH has increased production of potatoes by as much as 25%, with chicken similarly affected. Lettuce may have increased more, possibly by over 30%. Beef and cheese have also significantly increased. Fruit usage is as much as twenty percent lower than it would have been with no FAFH, and nuts consumption even lower. Although these are at best approximations, they serve to illustrate that FAFH has had a large impact on agriculture, especially because such changes have secondary effects. For example, increased chicken consumption suggests a higher demand for corn. In any case, there is little doubt that there have been significant impacts on agriculture, which may need to be explicitly considered when studying food demand and agricultural markets.
An important aspect of the study is we separated consumers by whether or not FAFH was consumed during the 2-day NHANES sample period. It is reasonable that these can be classified as more frequent and less frequent consumers of food away from home. We compared the home diets of these two groups and found that those with less frequency have home diets significantly higher in nutrition and significantly lower in energy density. This suggests that frequent restaurant diners may have less concern with nutrition. This result is evidently not due to differing sample characteristics, for it was essentially unchanged using a matched sample, and a similar outcome was obtained when the analysis was repeated using data from a previous NHANES.
It is perhaps reasonable that there may be differences between these two groups of consumers. If foods available in restaurants emphasize palatability at the cost of nutritional quality, then those with less concern with nutrition are more likely to choose FAFH. To the extent this is true, the proper way to assess the effect of FAFH may be a comparison of FAFH with the home dining of only restaurant diners, rather than that of all consumers. Furthermore, if many restaurant diners are indifferent to nutrition, it may increase the difficulty of encouraging restaurant patrons to choose healthier options through such means as menu labels. Taxes and subsidies may be more effective.
Appendix Table 1. Patterns and Effects 2005

Appendix Table 2. Nutrition and Energy Density Comparison, 2005–6, Adults
