Obesity causes multiple chronic metabolic disturbances including insulin resistance, diabetes mellitus, dyslipidaemia, hypertension, obstructive sleep apnoea syndrome and arteriosclerosis, which are further associated with impaired cognition( Reference Van den Berg, Kloppenborg and Kessels 1 , Reference Salorio, White and Piccirillo 2 ). However, there is growing evidence that the poor performance of obese individuals on several neuropsychological tests may occur independently of the direct physiological consequences of obesity( Reference Smith, Hay and Campbell 3 ). Cognitive decrements have been reported for memory( Reference Cournot, Marquie and Ansiau 4 , Reference Gunstad, Paul and Cohen 5 ) and executive functions, such as those commonly associated with fluid intelligence( Reference Smith, Hay and Campbell 3 , Reference Boeka and Lokken 6 – Reference Davis, Levitan and Muglia 8 ). The risk of poor executive cognitive function has been found in one study( Reference Fergenbaum, Bruce and Lou 9 ) to be four times higher in obese individuals than in non-obese individuals, independently of their demographic and medical characteristics. Furthermore, it has been suggested that the performance differences found in overweight and obese adults compared with normal-weight peers may be restricted to executive tasks after controlling for potential confounding factors( Reference Gunstad, Paul and Cohen 10 ). Although the physiological consequences of obesity are increasingly better understood, the nature of the association between obesity and cognitive capacity remains unclear. According to one hypothesis, obesity may lead either directly or indirectly to disturbances in brain function manifested by poor cognitive performance. Alternatively, poor executive functions (including planning, cognitive flexibility and logical reasoning ability) may explain obesity development through the adoption of poor health choices given that these abilities are important determinants of everyday decision making (e.g. dietary behaviour, low inhibition of palatable, yet low-nutritional value food consumption, and low physical activity)( Reference Hall 11 , Reference Hall 12 ).
It is now generally accepted that the development of chronic low-grade inflammation (CLGI) may represent the earliest consequence of obesity significantly contributing to the emergence of all other obesity-related pathophysiological co-morbidities. CLGI is usually documented by elevation of the levels of acute reactive proteins such as C-reactive protein (CRP; the most conventional and robust marker of CLGI in obesity and insulin resistance( Reference Aronso, Bartha and Zinder 13 – Reference Lemieux, Pascot and Prud'homme 15 )), serum amyloid A and fibrinogen and those of inflammatory cytokines and chemokines such as IL-6 and TNF-α( Reference Das 16 ). Data from several sources implicate CLGI to be involved in cognitive impairments. For instance, high levels of CRP in mid-life may increase the risk for the development of dementia, independently of the development of cardiovascular consequences( Reference Schmidt, Schmidt and Curb 17 ), whereas amelioration of CLGI has been linked to milder patterns of cognitive impairment in dementia-free middle-aged and elderly individuals( Reference Kuo, Yen and Chang 18 , Reference Ravaglia, Forti and Maioli 19 ). Raised levels of circulating CRP and fibrinogen and elevated plasma viscosity predict, longitudinally, lower late-life cognitive ability( Reference Marioni, Stewart and Murray 20 ). More importantly, there is increasing evidence linking erythrocyte sedimentation rate (ESR), a non-specific marker of CLGI, to reduced performance in neuropsychological tests, even in healthy young adults( Reference Karlsson, Ahlborg and Dalman 21 ). CLGI is the most important cause of obesity-induced insulin resistance, which is, in turn, linked, either concurrently or prospectively, to impaired cognitive performance( Reference Luchsinger, Tang and Shea 22 ). Moreover, obesity lowers the levels of circulating adiponectin, which exerts anti-inflammatory as well as insulin-sensitising effects. Recent work( Reference Han, Sakuma and Shin 23 ) on the pathophysiology of obesity has highlighted the potential role of adiponectin as a mediator of obesity-induced CLGI. More specifically, hyperplastic adipocytes in obese individuals undergo a phenotype change favouring the expression of components of the inflammatory machinery, i.e. the toll-like receptor 4, elevation of inflammatory cytokine production and down-regulation of adiponectin production. Lower levels of circulating adiponectin, which is strongly and negatively associated with anthropometric indices of adiposity and fat accumulation, further activate both innate and adaptive inflammatory mechanisms, resulting in insulin resistance and the development of a full-blown metabolic syndrome( Reference Steffens and Mach 24 ).
The present cross-sectional study examined the association between obesity-linked immune and metabolic effects, including that of adiponectin, and general cognitive capacity. Using data obtained from young and middle-aged Greek volunteers who were largely free of other clinically evident obesity-related medical co-morbidities, the study pursued two specific goals: to examine the possible negative association between body weight/fat content and fluid intelligence, as measured by a non-verbal logical reasoning test, and to compare two alternative path models regarding the direction of the obesity–cognition association, including CLGI as a mediator variable. Importantly, the potential mediating role of CLGI was assessed by controlling for the effects of additional physiological (metabolic dysfunction indices and adiponectin), psychological (anxiety and depression symptoms) and lifestyle (exercise) measures.
Methods
Participants and procedures
In total, 199 participants were recruited through direct contact during regularly scheduled appointments for routine clinical evaluations (e.g. complete blood count or other more specific tests appropriate for their health condition) at primary care facilities (private endocrinology practice and the University Hospital outpatient clinic). The participants were free of major and chronic autoimmune or connective tissue diseases. Other existing medical co-morbidities were quantified using the Charlson Comorbidity Index( Reference Hall, Ramachandran and Narayan 25 ). Individuals with self-reported history of mental diseases, including depression, and neurological disorders or traumatic brain injury (resulting in >10 min loss of consciousness) were excluded from further analyses (n 7). Furthermore, individuals with a recent history of infection (reported or diagnosed during clinical examination) or demonstrating leukocytosis (leucocytes >10·000) were not included in the final sample. Obesity indices, including BMI, waist:hip ratio (WHR) and body fat composition, were measured on site by a trained research assistant in a quiet examination room during a scheduled appointment. The assistant also administered the fluid intelligence test and the self-reported questionnaires and conducted the semi-structured mini-interview on health-related issues (e.g. smoking, alcohol consumption and past medical history).
The participants were assigned to three groups: normal weight (BMI range: 18·5–24·99 kg/m2); overweight (BMI range: 25–30 kg/m2); obese (BMI ≥ 30 kg/m2). Sample clinical and demographic information is given in Table 1.
Table 1 Clinical and demographic information for each group of participants (Mean values, standard deviations and ranges)

WHR, waist:hip ratio; STAI-T, State-Trait Anxiety Inventory; BDI-II, Beck Depression Inventory II; Godin, leisure-time physical activity (total raw scores); CCI, Charlson Comorbidity Index.
** Mean value was significantly different from that of the overweight group (P< 0·01).
†† Mean value was significantly different from that of the normal-weight group (P< 0·01).
The study was conducted according to the guidelines laid down in the Declaration of Helsinki, and all procedures involving human subjects were approved by the University of Crete Hospital Ethics Committee (protocol no. 3842). All participants provided written informed consent following a detailed explanation of the protocol. Participation was voluntary, and the participants were not given financial compensation for their time and effort.
Measures
Cognitive, psychological and activity measurements
The General Ability Measure for Adults (GAMA)( Reference Naglieri and Bardos 26 ), developed by one of the co-authors (A. N. B.), is a non-verbal measure of general (primarily fluid) intelligence, independent of verbal factors both in content and in administration, making the test useful for diverse populations (e.g. ethnicity, language and literacy). It consists of sixty-six problems utilising coloured, abstract designs that require the examinee to match a sample design (Matching scale), complete a pair of stimuli through analogy to a model pair (Analogies scale), identify logical sequences (Sequences scale) or combine pieces mentally to form a complete geometric pattern (Construction scale). The correct answer is selected from a set of six choices. Comparison data were available for 453 Greek adults (257 women and 196 men), aged 17– 82 (mean 39·96 (sd 14·47)) years with 2–24 years of formal education (mean 13·09 (sd 3·68) years), recruited from six broad geographical regions in the Greek mainland and islands (296 from urban areas and 157 individuals from rural areas or small towns (defined as population under 10 000)). The sample was divided into nine subgroups representing full cross-over of age and education with a minimum of thirty individuals per group. Education was converted into a discrete variable with three levels: 0–9 years of formal education; 10–12 years; 13+ years. Age was also grouped into three levels (17–37, 38–50 and 51–65 years). Raw total GAMA scores were converted into intelligence quotient (IQ)-equivalent scores (mean 100 (sd 15)) adjusting for age and education level. Raw scores on each of the four GAMA subscales (Matching, Analogies, Sequences and Construction scales) were converted into appropriate standard scores (mean 10 (sd 3)). Performance in this test is strongly correlated with scores on more comprehensive IQ measures, such as the Wechsler Adult Intelligence Scale-Revised (WAIS-R)( Reference Naglieri and Bardos 26 ). GAMA correlated 0·74 with WAIS-R performance IQ, 0·65 with WAIS-R verbal IQ, and 0·75 with WAIS-R full-scale IQ. The magnitude of these associations was similar in the presence of acute brain damage (r 0·74, 0·71 and 0·81, respectively)( Reference Donders 27 ) and among young adults experiencing academic difficulties (r 0 .69, 0·36 and 0·60, respectively( Reference Lassiter, Leverett and Safa 28 )). Given that GAMA was originally designed as a measure of fluid intelligence, achieving high scores in the test requires adequate engagement of functions generally considered to be ‘executive’( Reference Grafman and Litvan 29 ). Such presumed, higher-order, yet diverse, cognitive functions serve the ability to coordinate goal-directed thought and action and include complex attention, mental flexibility, inhibition, problem solving and decision making. These functions are believed to be primarily carried out by prefrontal areas( Reference Alvarez and Emory 30 ). Solving the logical problems in GAMA requires successful, continuous management of working memory resources( Reference Conway, Kane and Engle 31 , Reference Kyllonen and Christal 32 ) and the ability to switch cognitive strategies while dealing with different types of alternating problems( Reference Obonsawin, Crawford and Page 33 , Reference Rabbit and Rabbit 34 ). In fact, in the present Greek adult community cohort, performance in GAMA was found to correlate strongly (r >0·60) with such measures. Internal consistency (Cronbach's α = 0·93) and test–retest reliability (r 0·84; n 48) in the same cohort were adequate.
The revised edition of the Beck Depression Inventory-II( Reference Beck, Steer and Brown 35 ) is a twenty-one-item self-report questionnaire, designed to assess the intensity of Diagnostic and Statistical Manual of Mental Disorders IV (DSM-IV) depressive symptomatology in clinical and community adult samples. Each item consists of four statements arranged in order of increasing severity on a 0–3 scale (e.g. 0 = ‘I do not feel sad’ to 3 = ‘I am so sad and unhappy that I can't stand it’). The respondents are asked to rate each set of the statements according to how they have been feeling in the past 2 weeks, including the date of questionnaire completion. A total score of less than 14 points indicates minimal depression symptoms, that of 14–19 points indicates mild depression symptoms, and that of 20–28 points indicates moderate depression symptoms, whereas a score between 29 and 63 points indicates severe depression symptoms. The Greek version of Beck Depression Inventory-II( Reference Fountoulakis, Papadopoulou and Kleanthous 36 ) used in the present study has Cronbach's α =0·87.
The State-Trait Anxiety Inventory (STAI, Y form)( Reference Spielberger 37 ) is a self-report measure of the severity of anxiety feelings in adults. The Greek version of the Trait Anxiety subscale (STAI-T)( Reference Kosmidou, Roussi, Stalikas, Triliva and Roussi 38 ) was used in the present study, assessing more general and long-standing anxiety. Sample items include ‘I feel nervous and restless’, ‘I lack self-confidence’ and reverse-scored items such as ‘I am content’. Questions are rated on a four-point Likert scale resulting in a score range between 20 and 80 points, with higher scores indicating greater anxiety. Cronbach's α was 0·90.
Leisure-time physical activity was assessed using Godin's self-administered questionnaire( Reference Godin and Shephard 39 ). The participants reported the number of times spent in physical activity lasting for at least 15 min in an average week and classified them as strenuous, moderate or light (corresponding to 9, 5 or 3 metabolic equivalents). The total score was derived by multiplying the reported frequency by its corresponding metabolic equivalent value and summing the three products. Test–retest reliability of the total score ranged between 0·62 and 0·81( Reference Godin and Shephard 40 ). As with the majority of available self-administered physical activity questionnaires, Godin scores derived from broad community samples demonstrate a somewhat inconsistent validity profile. Correlations between Godin scores and measures of energy expenditure are generally low (r 0·10–0·32), although stronger associations with body fat have been reported( Reference Godin and Shephard 40 , Reference Jacobs, Ainsworth and Hartman 41 ). In all analyses involving Godin's data, square root-transformed scores were used to correct for significant positive skewness in the data.
Somatometrics
Total height and weight were measured to calculate BMI (kg/m2). WHR (waist circumference:hip circumference) is commonly used as a convenient index of body fat centralisation (visceral obesity). Waist and hip circumferences were measured at the level of umbilicus and gluteus, respectively. Total body fat percentage (Fat%) was estimated using bioelectrical impedance analysis employing Akern BIA 101 (Akern, S.r.l.). To validate this technique, body fat composition was determined using dual-energy X-ray absorptiometry using Lunar DPX (GE Healthcare) in a random subgroup of the participants (n 23), which along with computed tomography is known to be the most accurate assessment method of body fat( Reference Snijder, van Dam and Visser 42 ). The correlation between bioelectrical impedance analysis and dual-energy X-ray absorptiometry in this subgroup was linear and high (r 0·91).
Biochemical indices
Morning fasting blood samples were collected in a serum-separating tube from all participants, allowed to clot at room temperature for 30 min, centrifuged, aliquoted and stored at − 80°C in plastic vials for subsequent measurements. Biochemical analyses were performed at the University of Crete, Laboratory of Clinical Chemistry. Among the volunteers recruited, twelve demonstrated leukocytosis and were excluded from further analyses. The following indices were available for the remaining 180 participants: (a) high-sensitivity C-reactive protein (hs-CRP) measured by immuno-nephelometry assay on a Cobas 6000 analyser with a detection limit of 0·18 mg/l (Roche Diagnostics International, Ltd); (b) fibrinogen; (c) ESR. Insulin resistance was assessed using the Homeostasis Model Assessment of Insulin Resistance (HOMA-IR) index computed by multiplying serum levels of glucose (mg/dl) by those of insulin (μU/ml) and dividing by 405( Reference Matthews, Hosker and Rudenski 43 ). Finally, total plasma adiponectin was quantified using the Human Adiponectin ELISA Kit (catalogue no.: KHP0041; Life Technologies Corporation).
To simplify the set of regression and path analyses described below, composite indices for somatometric (BMI, WHR and Fat%), and inflammation (hs-CRP, ESR and fibrinogen) markers were assessed, expressed as the mean of the respective Z-scores in the present study sample. An exploratory factor analysis with Varimax rotation on the six measures revealed two factors with eigenvalues >1. Factor 1 was mainly accounted for by variance of fibrinogen, ESR and hs-CRP (factor loadings ranged between 0·68 and 0·83), whereas factor 2 mainly reflected variance of the three somatometric measures (factor loadings ranged between 0·69 and 0·86). Cross loadings did not exceed 0·39. Correlations among somatometric indices ranged from r 0·23 (Fat%–WHR) to r 0·81 (BMI–Fat%). Correlations among inflammation indices ranged from r 0·48 (fibrinogen–hs-CRP) to r 0·56 (ESR–hs-CRP).
Statistical analysis
The first aim of the study was explored through ANOVA on GAMA standard scores with BMI group as the between-subjects variable with three levels (normal weight, overweight and obese). Physiological and clinical variables, for which the three groups should be found to vary significantly, were also entered in a separate one-way ANOVA as covariates in SPSS (version 20; SPSS, Inc.).
The second aim of the study was explored through structural equation modelling analyses comparing the two alternative, non-nested models shown in Fig. 1. Model 1 postulates a direct effect of obesity (somatometric index) on cognition (GAMA total score), whereas model 2 represents the reverse relationship. Each model included a number of parallel mediators representing psychological (Beck Depression Inventory-II and STAI-T scores), lifestyle (Godin's scores) and physiological (HOMA-IR and adiponectin) factors and estimated both direct and indirect associations (i.e. through each of the mediating variables) between obesity and cognition. The two models were directly compared on fit indices calculated in AMOS version 20 (SPSS, Inc.). This statistical technique allows testing of theoretical pathways involving causal relationships and is thus suitable for inferential analysis of cross-sectional data( Reference Byrne 44 ).

Fig. 1 Comparison of the two alternative path models: model 1 examines the impact of obesity (somatometric) on general cognitive ability (General Ability Measure for Adults (GAMA, raw score)), while model 2 tests the reverse path with general cognitive ability impairment resulting in obesity through psychological, inflammation and physiological parameters. Significant standardised coefficients (P< 0·01) and R 2 values are shown. Somatometric: composite indices BMI, waist:hip ratio, and Fat%; Inflammation: composite indices high-sensitivity C-reactive protein, erythrocyte sedimentation rate, and fibrinogen; Metabolic profile: Homeostasis Model Assessment of Insulin Resistance; Godin (sqrt): square root-transformed Godin's raw scores; BDI-II: Beck Depression Inventory-II total score.
Results
BMI group comparisons
The series of one-way between-subjects ANOVA confirmed the expected group differences in WHR (F(2,177) = 30·65, P= 0·0005, η2= 0·261) and Fat% (F(2,177) = 81·03, P= 0·0005, η2= 0·568). As shown in Table 1, all pairwise group differences were significant. Further tests showed that BMI groups did not differ in Beck Depression Inventory-II, STAI-T or Godin's scores (P>0·5). The χ2 tests did not reveal group differences in the distribution of Charlson Comorbidity Index scores, marital or financial status, or type of job. Data on all metabolic and inflammation indices for each BMI group are given in Table 2. In addition to somatometric indices, the three groups also differed in inflammation indices (ESR: F(2,177) = 14·53, P= 0·0005, η2= 0·115; hs-CRP: F(2,177) = 26·65, P= 0·0005, η2= 0·218; fibrinogen: F(2,177) = 6841, P= 0·001, η2= 0·07) and metabolic indices (insulin: F(2,177) = 13·13, P= 0·0005, η2= 0·135; glucose: F(2,177) = 10 268, P= 0·0001, η2= 0·096; HOMA-IR: F(2,177) = 12·05, P= 0·0005, η2= 0·137; adiponectin: F(2,177) = 7·74, P= 0·001, η2= 0·080). With the exception of adiponectin, the obese group had higher indices than both normal-weight and overweight groups. As expected, the opposite pattern was observed for adiponectin. Given that the proportion of men was higher in the overweight group (37 %) than in the normal-weight group (12 %) and obese group (15 %; φ = 0·213, P= 0·013), the analyses were repeated with sex as an additional factor, which failed to reveal any significant main effects or interactions (P>0·1).
Table 2 Metabolic and inflammation indices for each group of participants (Mean values, standard deviations and ranges)

ESR, erythrocyte sedimentation rate; hs-CRP, high-sensitivity C-reactive protein; HOMA-IR, Homeostasis Model Assessment of Insulin Resistance.
** Mean value was significantly different from that of the overweight group (P< 0·01).
†† Mean value was significantly different from that of the normal-weight group (P< 0·01).
‡ To convert insulin in μU/ml to pmol/l, multiply by 6·945. To convert glucose in mg/dl to mmol/l, multiply by 0·0555.
As the three groups differed in age (F(2,177) = 5·94, P= 0·003), years of formal education (F(2,177) = 15·21, P= 0·0005) and sex distribution, group-level analyses of cognitive ability were conducted on age- and education-adjusted GAMA IQ scores and subscale standard scores. At the group level, the key finding was a main effect of BMI group (F(2,177) = 7·09, P= 0·001, η2= 0·083). Planned pairwise comparisons revealed that the obese group scored significantly lower than both the normal-weight (P= 0·001; Bonferroni-corrected) and overweight (P= 0·029) groups, which did not differ from each other (P>0·7). Main group effects were also significant for each of the four GAMA subscales (P< 0·001), and pairwise tests revealed a similar pattern of group differences (Table 3). Importantly, group differences in GAMA IQ scores remained significant after controlling for STAI-T, BDI, Godin's and Charlson Comorbidity Index scores, as they did after controlling for metabolic function (insulin, HOMA-IR and adiponectin). However, on controlling for individual variability in the systemic inflammation composite, group differences in GAMA IQ scores were all but eliminated (P>0·24). Results were essentially identical when ANCOVA were performed on GAMA total raw scores controlling for participant age and education. Neither the main effect of sex nor the group × sex interaction approached significance (P>0·8). Power analyses indicated that for the effect size of group differences observed in the study, the estimated power for detecting significant group main effects ranged between 0·96 and 0·99 at α = 0·05 and between 0·84 and 0·99 at α = 0·001.
Table 3 Average General Ability Measure for Adults (GAMA) total intelligence quotient-equivalent scores and subscale standard scores for each BMI group (Mean values and standard deviations)
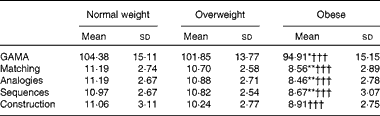
Mean value was significantly different from that of the overweight group: * P< 0·03, ** P< 0·01 (Bonferroni-corrected).
††† Mean value was significantly different from that of the normal-weight group (P< 0·001; Bonferroni-corrected).
The percentages of individuals with ‘normal-range’ IQ were 90, 88 and 78 % for the normal-weight, overweight and obese groups, respectively. The remaining participants had estimated IQ scores in the 70–85-point ‘borderline’ range.
The role of low-grade systemic inflammation
Table 4 reveals a largely expected pattern of intercorrelations between the somatometric, inflammation and cognitive indices (GAMA raw scores). Correlation coefficients were in the moderate range meeting the essential requirement to further assess direct and indirect effects of obesity on GAMA (and the reverse) through inflammation. As shown in Fig. 1, results suggested that model 1, which tested the hypothesis that obesity (somatometric index) affects cognitive ability (GAMA) through inflammation, controlling for psychological, lifestyle and physiological factors, fitted the present data significantly better (χ2= 9·6, df = 10, P= 0·48, normed fit index (NFI) = 0·953, comparative fit index (CFI) = 1·00, root mean square error of approximation (RMSEA) = 0·0001) than the poorly fitting alternative model 2 (χ2= 34·51, df = 10, P= 0·001, NFI = 0·830, CFI = 0·866, RMSEA = 0·114). Model 2 examined the reverse hypothesis that lower cognitive abilities would lead to higher rates of obesity (again controlling for psychological, lifestyle and other physiological factors). Finally, Akaike's information criterion (AIC), which is widely used to compare non-nested models originating from the same data, was considerably smaller (AIC = 59·61) for model 1 than for model 2 (AIC = 84·10), indicating a better fit of the former. Notably, the smaller R 2 values in model 1 (describing the regression of GAMA on each of the mediators) when compared with model 2 (describing the regression of the somatometric index on the same mediators) is explained by the stronger direct paths of each physiological/exercise variable with the somatometric variable. It is further expected that these associations will be stronger than the direct effects of the same physiological/exercise variables on the (conceptually more distal) cognitive measure.
Table 4 Pearson's correlations between variables examined in the structural equation modelling analyses

Somatometric, composite indices BMI, waist:hip ratio, and Fat%; Inflammation, composite indices high-sensitivity C-reactive protein, erythrocyte sedimentation rate, and fibrinogen; GAMA, General Ability Measure for Adults (raw score); HOMA-IR, Homeostasis Model Assessment of Insulin Resistance; Godin, leisure-time physical activity; STAI-T, State-Trait Anxiety Inventory; BDI-II, Beck Depression Inventory-II.
Values were significantly different: * P< 0·05, ** P< 0·01.
To ensure that the dataset possessed adequate power for model testing, the structural model in which GAMA was a function of BDI, inflammation, adiponectin, metabolic profile and Godin's scores, all being a function of somatometrics, was simulated with between-construct paths equal to 0·25, to be on the conservative side. On using a Monte Carlo simulation with 500 replications and sample sizes equal to 180 participants, results pointed to the presence of minimal bias. Specifically, the bias of the χ2 statistic was equal to zero in terms of probabilities of rejected χ2 values and the actual estimate on the χ2 values between the expected mode (with 10 df) and the observed bootstrapped model was equal to 0·140.With an expected RMSEA of 0·059, estimates were equal to 0·067, indicating a bias of 0·008, which was negligible. Lastly, the mean square error of the path estimates ranged between 0·0044 and 0·0079.Thus, all power analysis results corroborated with the premise that 180 participants were sufficient to obtain solutions with valid path estimates and proper rejection rates of the χ2 statistic.
Discussion
In the present study, we investigated the association between obesity, CLGI and cognitive ability in a community sample of young and middle-aged Greek adults free of any obvious medical or psychiatric diseases and grouped as per their BMI. The results of the present study indicated an inverse association between elevated BMI and cognitive performance (assessed using a test of non-verbal logical reasoning ability and fluid intelligence), confirming previously published reports. More specifically, obese participants exhibited significantly poorer performance compared with age-matched overweight and normal-weight participants. The novel finding of the present study is that obesity-linked CLGI, the principal cause of all metabolic consequences of obesity (insulin resistance, diabetes mellitus, atherosclerosis, etc.), appears to be directly associated with cognitive defects as demonstrated by both group-level analyses and mediated regression models.
It is now increasingly being recognised that the deleterious effects of obesity on cognition arise much earlier than previously thought and are not solely mediated by the commonly observed clinical consequences of obesity, such as hypertension, diabetes and atherosclerosis( Reference Elias, Goodell and Waldstein 45 ). Instead, these effects may result from obesity-related early pathophysiological effects on innate immunity( Reference Calder, Ahluwalia and Brouns 46 ), which, in turn, directly harm the central nervous system. Indeed, there is evidence derived from animal models suggesting that high-fat diet-induced obesity may result in inflammation-mediated harmful effects on several brain areas, including the neocortex and hippocampus( Reference Souza, Araujo and Bordin 47 , Reference Zhang, Dong and Ren 48 ). Furthermore, peripheral inflammation due to maternal obesity as a result of high-fat diet can be transferred to offspring's brain, resulting in increased microglial activity in the hippocampus at birth, elevated pro-inflammatory cytokine responses in adulthood, anxiety and spatial learning difficulties( Reference Bilbo and Tsang 49 ). In another study, mice fed a very-high-fat lard diet (60 %) presented with weight gain and exhibited areas of brain inflammation associated with poorer performance in a challenging maze task, compared with mice fed a high-fat, Western diet (41 % fat), resulting in weight gain but not in brain inflammation( Reference Pistell, Morrison and Gupta 50 ). Taken together, these experimental findings suggest that CLGI constitutes a pathway via which obesity causes impaired cognition much earlier and independently of the other obesity-induced co-morbidities.
The medical literature suggests that in addition to the degree of systemic inflammation, obese individuals may differ in other pertinent physiological characteristics as well. A considerable percentage of the adult obese population (up to 20 %)( Reference Karelis, St-Pierre and Conus 51 ) appears to be less susceptible to the unfavourable metabolic profile that typically accompanies obesity. ‘Metabolically healthy’ as opposed to ‘metabolically unhealthy’ obese individuals have been found to have higher insulin sensitivity levels, no signs of hypertension, normal lipid levels, low TAG levels, and high HDL-cholesterol and adiponectin levels and carry a lower risk for developing type 2 diabetes and CVD( Reference Denis and Obin 52 ). However, it should be noted that metabolically healthy obese individuals are characterised by much lower levels of inflammation compared with other obese individuals( Reference Karelis, Faraj and Bastard 53 ). Given the present results on the mediating role of inflammation in obesity-linked cognitive performance decline, it appears reasonable to hypothesise that metabolically healthy obese individuals are less prone to cognitive difficulties compared with metabolically unhealthy obese individuals. To our knowledge, the only study that has addressed this question( Reference Singh-Manoux, Czernichow and Elbaz 54 ) did not support this hypothesis, failing to find differences between metabolically abnormal and metabolically normal obese participants with regard to a global cognitive score either at baseline or at the 10-year follow-up. However, given that Singh-Manoux et al. ( Reference Singh-Manoux, Czernichow and Elbaz 54 ) did not include measures of inflammation, the potential role of low-grade inflammation could not be assessed in this intriguing obesity phenotype. The small size of the present study sample notwithstanding, the group-level analysis results do not support this claim, by failing to differentiate between obese individuals with relatively high insulin resistance levels and those demonstrating a more typical metabolic profile.
The second goal of the present study was to explore the potential role of additional physiological factors, namely insulin resistance (HOMA-IR) and adiponectin production, in obesity-related inflammation possibly affecting cognition. However, bivariate correlations between these parameters and GAMA, somatometric index or inflammation were weak, and including them as mediators in the obesity–inflammation–cognition association did not support this hypothesis. Notably, neither adiponectin nor HOMA-IR accounted for significant variability in GAMA scores, contrary to the claim that adiponectin may assume a protective role in cognitive ability as an insulin sensitiser. Studies investigating the association of adiponectin with cognition are to date limited and controversial, focusing on elderly populations( Reference Kamogawa, Kohara and Tabara 55 – Reference Van Himbergen, Beiser and Ai 58 ), and challenge the suggested association of adiponectin with a lower risk of CVD( Reference Kizer, Barzilay and Kuller 59 – Reference Sattar and Nelson 61 ). In summary, controversial findings from previous studies and the present study stress the need for further systematic investigations of the hypothesised inverse association between adiponectin and obesity-related poor cognitive performance. In this direction, use of high-molecular-weight adiponectin as a better indicator of insulin sensitivity instead of total adiponectin has been proposed( Reference Gustafson 62 ).
Other factors addressed as potential mediators in our proposed obesity–inflammation–cognition model were symptoms of depression and anxiety. Compromised cognitive function, especially in the areas of sustained attention and mental flexibility, has been identified as a characteristic of clinical and subclinical depression( Reference Holmes and Pizzagalli 63 , Reference Weiland-Fiedler, Erickson and Waldeck 64 ). Moreover, elevated levels of stress and anxiety have been linked to impaired performance in cognitive tasks( Reference Airaksinen, Larsson and Forsell 65 ) as well as increased body weight( Reference Brunner, Chandola and Marmot 66 ). Thus, psychoemotional well-being, as indexed by the absence of symptoms of depression and anxiety, has been implicated as a potential mediator of the obesity–cognition association( Reference Cserjési, Luminet and Poncelet 67 ). However, self-reported levels of such symptoms did not differ among the three BMI groups in the present study, indicating that obesity is associated with reduced cognitive function in a more direct way, at least in the present study sample. This finding is in contrast to at least one previous report linking self-reported levels of negative emotions to cognitive impairments independently of BMI( Reference Cserjési, Luminet and Poncelet 67 ). Nonetheless, participants of that study were heavily obese women (mean BMI 43·2 (sd 3·8) kg/m2) seeking surgical interventions and, unlike the case in the community-dwelling obese group in the present study (mean BMI 34·22 (sd 3·7) kg/m2), both their inflammatory and psychoemotional profiles may have been overburdened. Consistent with the null findings of the present study, large cross-sectional studies have also failed to find strong associations between obesity and depression, while it was longitudinal studies that established significant bidirectional links between obesity and depression( Reference Shelton and Miller 68 ).
It would be interesting to determine whether the magnitude of the purported effect of obesity on cognition varies with BMI. According to this hypothesis, obese individuals are more likely to have suffered the subtle but adverse effects of low-grade inflammation at a greater intensity and for a longer period of time than non-obese individuals, sufficient to incur detectable cognitive impairment. Preliminary moderation analyses performed in the present study sample (using BMI group as a moderator of the obesity–inflammation–cognition association) suggest that these effects may indeed be stronger among obese individuals, but subgroup sizes were relatively small to allow for firm conclusions to be drawn. Notably, the cross-sectional design of the present study does not permit quantification of the inflammation ‘history’ of participants, necessary to establish critical features of inflammation potentially leading to cognitive decline.
In the long run, persistent through lifetime, obesity-induced CLGI is also destined to coalesce with further inflammatory processes observed in elderly, independently of their earlier weight profile. Indeed, in 2000, Franceschi et al. ( Reference Franceschi, Bonafè and Valensin 69 ) were the first to describe an up-regulation of the inflammatory response in the elderly and coined the term ‘inflammaging’ to refer to this phenomenon. Inflammaging is now generally recognised as another characteristic of old age as part of the general syndrome of immunosenescence. This up-regulation results in the development of chronic low-grade systemic pro-inflammatory state in the elderly, identical to that due to increased adiposity irrespective of age. As expected, it is characterised by an elevation of the levels of several IL as well as those of the acute-phase proteins produced by the liver in response to inflammatory cytokines such as CRP. Genetic, environmental and age-related factors contribute to the development of inflammaging and include polymorphisms to the promoter regions of cytokines, cytokine receptors and antagonists, age-related decreases in autophagy and of course increased adiposity. Although the present study focused on adults aged < 65 (mean age 38·3) years, it is predicted that obesity will contribute to the worsening of inflammaging in the elderly, further affecting ‘natural’ cognitive decline.
A second important limitation of the present study was that the sample consisted mainly of women, preventing exploration of potential sex differences in the proposed impact of obesity and associated low-grade inflammation on cognitive ability. In the present study sample, however, there was no evidence of an effect of sex on the association between obesity and cognition. Furthermore, cognitive changes during the menstrual cycle were not considered in the analyses( Reference Farage, Osborn and MacLean 70 ), although participant recruitment and testing schedules should have ensured random distribution of such effects across BMI groups.
A third limitation of the present study was reliance upon a single measure of cognitive ability, while earlier studies focusing exclusively on the obesity–cognition association have examined it more thoroughly, utilising extensive test batteries that address specific cognitive domains. In this context, it was fortuitous that significant effects of obesity and inflammation were found on a measure designed to assess general cognitive ability in the form of fluid intelligence. The sensitivity of GAMA to the effects of obesity and inflammation is not surprising, however, in view of (a) the very high correlations between GAMA scores and, primarily, performance IQ as measured by comprehensive test batteries( Reference Naglieri and Bardos 26 , Reference Lassiter, Leverett and Safa 28 ), (b) that adequate performance in this task requires reasoning ability, as well as cognitive flexibility, given the variety of alternating logical problems featured, and (c) that GAMA is a timed task, rendering performance sensitive to individual differences in processing speed. Notably, both cognitive flexibility and processing speed are particularly susceptible to diffuse brain insults.
It should further be noted that other commonly used measures of CLGI, such as pro-inflammatory cytokines (e.g. IL-6), were not explored in the present study in view of extant evidence that elevated CRP content is the most robust marker of CLGI in obesity and insulin resistance( Reference Aronso, Bartha and Zinder 13 – Reference Lemieux, Pascot and Prud'homme 15 ). The fact that the vast majority of the reports of CLGI in obesity use this marker renders the present data comparable to the published literature( Reference Schmidt, Schmidt and Curb 17 – Reference Marioni, Stewart and Murray 20 ).
Perhaps the most critical weakness of the present study concerns the cross-sectional nature of the data, which rendered them incapable of providing a strong test for the directionality of the obesity–inflammation–cognition association. Cognitive flexibility and logical reasoning ability, which are considered to be among the key components of fluid intelligence, are in principle important determinants of everyday decision making and are probably involved in the adoption of healthy lifestyle and behaviours (nutritional choices and physical activity)( Reference Hall 11 , Reference Hall 12 ). Impairments in such abilities may explain obesity development through poor health choices, while the accumulation of body fat and the ensuing low-grade systemic inflammation may account for further cognitive decline. This hypothetical cycle of events may also explain the long-term failure of common obesity prevention and treatment strategies (information on healthy eating choices and encouragement to perform physical activity and follow appropriate diet)( Reference Green, Larkin and Sullivan 71 ). Although longitudinal evidence is required to clarify this issue, structural modelling of the present cross-sectional dataset provides preliminary support to the notion that obesity adversely affects general cognitive ability through a cascade of physiological events rather than the reverse.
Despite these limitations, we were able to identify cognitive decrements in a carefully screened sample of obese individuals, who were free of other serious medical conditions (e.g. chronic autoimmune or inflammatory diseases) or mental disorders (e.g. depression) and establish a mediating role of CLGI. Bearing in mind that obesity often coexists with other medical conditions (e.g. diabetes, hypertension and sleep apnoea syndrome) known to be independently associated with cognitive deficits, it is expected that in a consecutive, unscreened sample of obese individuals, more severe cognitive deficits would be documented. It should be noted that the incidence of obesity in Greece is rising at an alarming rate both in adults( Reference Panagiotakos, Pitsavos and Chrysohoou 72 ) and in children( Reference Farajian, Panagiotakos and Risvas 73 ) in a country that until recently has followed a beneficial-for-weight-control Mediterranean diet, raising concerns regarding obesity-related cognitive dysfunction in this population.
Acknowledgements
The present study was co-funded by the European Union (European Social Fund – ESF) and Greek national funds through the Operational Program ‘Education and Lifelong Learning’ of the National Strategic Reference Framework (NSRF) – Research Funding Program: Heracleitus II: Investing in knowledge society through the European Social Fund (MIS: 349309. URL: http://www.edulll.gr/). The funding agency had no role in study design, data collection and analysis, decision to publish, or preparation of the manuscript.
The authors’ contributions are as follows: E. C. S., P. S. and A. N. M. designed the study; E. C. S., P. D. A., E. D. and M. V. collected the data; E. C. S. and P. S. analysed the data; E. C. S., P. S., A. N. M. and A. N. B. wrote the article.
None of the authors has any conflicts of interest to declare.