The first electroencephalography (EEG) on a human was reported by Hans Berger in 1920. The fundamental principle of EEG is to record the electrical activity produced by the brain, using electrodes. In considering the role of EEG in psychiatry almost 25 years later, Dennis Reference WilliamsWilliams (1954) observed that studies of EEG recordings had offered the clinical psychiatrist little of positive value. He suggested several reasons for this: EEG does not give a true record of cerebral action potentials; attempts at correlating EEG recordings from millions of cells, the electrical activity of which was not understood, with patients’ clinical picture proved meaningless; the recording techniques used were crude; and EEG records simple physiological changes in neuronal activity which are not reflected in the complex integration of the human brain. Nevertheless, Williams did not dismiss EEG out of hand. He outlined its usefulness in the exclusion of gross organic disorder; the study and modification of local cerebral disorders related to abnormal behaviour; the correlation of changes in EEG recordings with single aspects of temperament or personality; and the use of activation techniques to demonstrate hidden pathologies. There have been technological advances since his erudite article was published, but the fundamental problems remain.
In this article, we focus on the role of EEG in current psychiatric practice, EEG findings in specific psychiatric syndromes, clinical indications for requesting EEG, and interpretations of EEG recordings. We will also highlight measures to ensure appropriate and competent use of EEG in the routine clinical practice of psychiatrists.
EEG in current psychiatric practice
A retrospective review of EEG requests over a 12-month period found that 6.2% of referrals were made by psychiatrists but that psychiatric referrals had the lowest abnormality detection rate (Reference O'Sullivan, Mullins and CassidyO'Sullivan 2006). A history of epilepsy, being on clozapine and possible convulsive seizures were found to be the only significant predictors of an abnormal EEG recording. Another study reported the presence of an organic factor identified during clinical assessment to be significant predictor, but none of the abnormal recordings helped in discovering an aetiology that was not already suspected from assessment (Reference Lam, Hurwitz and WadaLam 1988). It also reported cases in which psychiatrists ignored abnormal recordings.
Of 187 requests for EEG on patients showing aggressive behaviour, 71% were made to ascertain evidence of epilepsy and 22% to determine the presence of organic brain dysfunction. Only one recording showed unequivocal evidence of an epileptic focus (Reference Stone and MoranStone 2003). The low diagnostic yield reported in the Stone study is similar to the 0.5–2% found in EEG conducted to screen for epilepsy (Reference Gregory, Oates and MerryGregory 1993). Another issue regarding the diagnostic yield emerged from a study of psychiatric in-patients (Reference Warner, Boutros and PeabodyWarner 1990): although a third of the EEG recordings were abnormal, this led to a change in diagnosis in only 1.7% of cases.
Limitations of EEG
Why is it that EEG produces findings that some consider to be clinically irrelevant? The most important limitation that one should take into account is that, by and large, EEG abnormalities are non-specific. With its poor spatial resolution, EEG cannot pinpoint the pathological process, nor can it aid the differentiation between functional diagnoses. In organic dysfunction, EEG would miss slow-growing, deep-seated tumours. Given that the epileptiform activity in epilepsy is not consistently present, one can expect a false-negative rate of around 50% on interictal EEG recordings (Reference Hopkins, Garman and ClarkeHopkins 1988) – and a false-positive rate of 2% in the general population (Reference Hughes and JohnHughes 1999). Apart from such theoretical and clinical limitations, the cumbersome procedure in itself leads to artefacts that can be difficult to interpret. The comparative lack of psychiatric training among physiologists and the lack of training in observing and interpreting EEG among psychiatrists is another problem.
Despite these shortcomings, EEG is attractive for clinicians: the procedure is non-invasive, can measure spontaneous brain activity, requires a lower level of patient cooperation than functional magnetic resonance imaging, and is relatively inexpensive and easily available.
The EEG procedure
An EEG wave is the representation of the differences in electrical potential between an electrode placed on the scalp and a reference electrode placed elsewhere on the head. The transient nature of this electrical difference leads to oscillation, which produces the EEG pattern or trace.
Standard scalp EEG, which uses 21 electrodes, can take up to 40 minutes. In addition to the external placing used in standard EEG, the electrodes can be placed directly inside the skull (intracranial EEG), under the skin of the scalp (subdural) or on the external brain surface and dura mater (electrocorticography).
If abnormality is strongly suspected but the resting EEG is normal, activated EEG is used to increase the probability of recording abnormal patterns. Strenuous hyperventilation and photic stimulation (stimulation of the visual cortex using light) are the most common activation procedures.
In EEG-video monitoring, a standard EEG is conducted with simultaneous video recording of the patient. The advantage of EEG-video monitoring lies in the observation of the temporal relationship between the behaviour under investigation and the EEG recording. Serial and continuous EEG recordings can help to increase the diagnostic yield.
Quantitative EEG (qEEG) utilises analytical algorithms that automatically assess resting and evoked activity of the brain (Box 1). This method involves comparing data from a specific individual with those of database of a large population or of a defined population with a specific disorder.
BOX 1 Quantitative EEG
Frequency analysis is the most powerful analytical tool used in qEEG to limit artefacts. It acts by reducing EEG data to a manageable number of coefficients. The fast Fourier transformation, based on Fourier analysis, simplifies computation of spectral coefficients. As EEG traces contain regular patterns, they may be better understood by their spectral (frequency) content. In a spectral analysis, voltage amplitudes are translated into frequencies because mental activities are better reflected in periodicities.
The power spectral density (power spectrum) approach has always been a popular method for classifying EEG signals. The power spectral density (PSD) describes how the power (or variance) of a time series is distributed with frequency. The first step in EEG classification is to determine whether the signals have distinguishable features in their power spectrum, where power is a state parameter that can be used instead of the magnitude of the frequency of the wave. Power is sometimes represented as log power (base 10 logarithm).
Frequency or spectral analysis involves selection of elementary shapes or frequencies (waveforms) which are added together like weights on a scale until their total matches the pattern under investigation. The height or intensity of a waveform – its amplitude – is computed in microvolts for each frequency.
For further information see Reference KaiserKaiser (2005).
In qEEG, event-related (evoked) potentials – neuronal electrical responses to stimuli – are extracted from EEG recordings by time domain analysis (analysis of the EEG trace with respect to time). Event-related oscillations are changes in the power spectrum frequency of the ongoing EEG, which can be directly or loosely time-related. They are complementary to the routine EEG as they provide an understanding of higher brain functions, including cognition.
Physiological factors that influence EEG
EEG and age
Electroencephalogram patterns (Table 1) are influenced by age. Infant EEG recording shows slow-wave and high-amplitude rhythms. The asynchronous, more mature rhythms develop between 2 and 6 years of age. Generalised low-amplitude beta activity starts to appear in puberty, and adult EEG shows posterior alpha and anterior beta activity. In old age, there is a slowing of the alpha frequency accompanied by a decrease in delta activity.
TabLE 1 EEG rhythms

Wave type | Frequency, Hz | Feature |
---|---|---|
Delta | < 4 | Frontal in adults and posterior in children |
High-amplitude waves | ||
Seen in slow-wave sleep of adults | ||
Theta | 4–7 | Can be found in young children |
Transient theta waves can be seen in up to 15% of the normal population | ||
Alpha | 8–12 | Bilateral and occipital |
Higher amplitude on the dominant side | ||
Seen in relaxed state with the eyes closed | ||
Attenuated with attention | ||
Beta | 12–30 | Bilateral, frontal |
Low-amplitude waves | ||
Seen in alert stage | ||
Mu | 7–11 | Associated with motor activity and seen in precentral areas |
Attenuated with contralateral limb movement | ||
Lambda | Occipital | |
Single sharp wave | ||
Associated with ocular movement | ||
Gamma | 34–100 | Associated with cognitive and motor functions |
EEG and state (awake v. sleep pattern)
Electroencephalograms are also affected by the level of alertness or consciousness. A normal ‘eyes-closed’ wake EEG trace is characterised by highly rhythmic alpha waves (Table 1). Beta waves are not uncommon, especially over the frontocentral regions in a normal adult wake EEG trace. Theta activity is very limited on wake EEG traces and there should be no delta activity. Alpha coma is a diffuse alpha wave occurring in a comatose state. Table 2 shows the stages of sleep and related EEG patterns.
TabLE 2 Sleep and EEG patterns
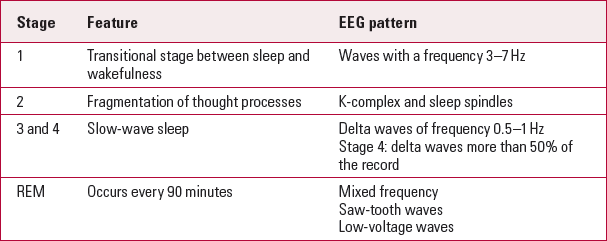
Stage | Feature | EEG pattern |
---|---|---|
1 | Transitional stage between sleep and wakefulness | Waves with a frequency 3–7 Hz |
2 | Fragmentation of thought processes | K-complex and sleep spindles |
3 and 4 | Slow-wave sleep | Delta waves of frequency 0.5–1 Hz |
Stage 4: delta waves more than 50% of the record | ||
REM | Occurs every 90 minutes | Mixed frequency |
Saw-tooth waves | ||
Low-voltage waves |
EEG in major psychiatric disorders in working-age and older adults
Many associations between specific EEG findings and psychiatric disorders are marred by conflicting reports. In this section we list the robust EEG findings in relation to specific psychiatric disorders and drugs.
Delirium
Electroencephalography can be a valuable tool of investigation in delirium. The EEG trace in delirium characteristically shows slowing or dropout of the posterior dominant rhythm (earliest), generalised theta or delta slow-wave activity, a poorly organised background rhythm, and no reaction to opening and closing of the eyes. Serum anticholinergic activity has been suggested as a biomarker for delirium and such activity has been correlated with the following EEG findings: occipital slowing and qEEG with delta and theta increase as well as alpha decrease, as a result of which there is a lower slow-wave ratio compared with traces obtained in dementia (Reference Thomas, Hestermann and KopitzThomas 2008a). On qEEG, theta activity and relative power in the delta band collectively distinguish delirium from dementia (Reference Jacobson, Leuchter and WalterJacobson 1993). Prolonged-activation EEG can be used to diagnose delirium in dementia with 67% sensitivity and 91% specificity based on variations in alpha and delta power density (Reference Thomas, Hestermann and WaltherThomas 2008b).
Dementia
In dementia, qEEG abnormalities include higher frontocentral and parieto-occipital theta values, and lower parieto-occipital beta values. Lower peak frequency has been significantly associated with decline in cognitive function in patients with probable early-stage Alzheimer's dementia (Reference Clauss, Kwa and TeunisseClauss 1998). There is evidence that visual and quantitative analyses of EEG traces can be used to differentiate between Alzheimer's and vascular dementia (Reference Gawel, Zalewska and Szmidt-SalkowskaGawel 2009). Quantitative EEG findings indicate that increased EEG slowing is associated with a poorer cognitive performance in patients with vascular dementia (Reference Muresanu, Alvarez and MoesslerMuresanu 2008).
Electroencephalography can help in discriminating between Alzheimer's disease and Lewy body dementia at the earliest stages of illness. In a study (Reference Bonanni, Thomas and TiraboschiBonanni 2008) that recruited 50 patients with a diagnosis of Alzheimer's dementia, Lewy body dementia or Parkinson's disease dementia, dominant wave frequencies were 8.3 Hz (s.d. = 0.6) for the Alzheimer's disease group and 7.4 Hz (s.d. = 1) for the Lewy body dementia group. Less than half (46%) of the patients with Parkinson's disease dementia exhibited the EEG abnormalities seen in those with Lewy body dementia.
In a smaller study (n = 19), qEEG results for patients with frontotemporal dementia were marked by no increase in slow activities but by a decrease in fast activities (Reference Lindau, Jelic and JohanssonLindau 2003). The relative power from the temporal region in the beta-2 band and from the parietal region in the theta, alpha and beta-2 bands are informative qEEG variables in distinguishing between frontotemporal dementia and Alzheimer's disease, with a diagnostic accuracy of 84.6% (Reference Yener, Leuchter and JendenYener 1996).
Despite such observations, a meta-analysis has showed that evidence of diagnostic utility of EEG in dementia and mild cognitive impairment is still insufficient (Reference Jelic and KowalskiJelic 2009).
Psychotic disorders
One of the difficulties in interpreting EEG findings that show increased frontal delta activity in people with schizophrenia is that such individuals may be more prone to involuntary saccadic eye movements (Reference Matsue, Okuma and HidermitsuMatsue 1986). Antipsychotic medications increase the amount of alpha activity, adding another confounding factor to the EEG recording.
A study of 19 medication-free patients with chronic schizophrenia found greater delta and left-sided beta activity than in the 21 controls (Reference Karson, Coppola and DanielKarson 1988). In the very small number of patients who had reduced alpha frequency, there was an association with larger mean ventricular size. This reduction in alpha frequency has been confirmed in a study of 20 never-treated schizophrenia patients compared with age-matched controls (Reference Omori, Koshino and MurataOmori 1995).
Most research involving qEEG activity power spectra has shown that people with schizophrenia exhibit increased beta and slow-frequency powers and reduced main alpha power (Reference John, Prichep and AlperJohn 1994). One study (Reference Gross, Joutsiniemi and RimonGross 2006), involving 16 patients with treatment-resistant chronic schizophrenia experiencing a relapse, used qEEG to investigate the correlation between the power of different frequency bands over the frontocentral scalp area and Liddle's factors and negative subscale scores on the Positive and Negative Syndrome Scale (PANSS). The only significant finding was a positive correlation between beta power and psychomotor poverty. Similar studies have shown that negative symptoms are correlated with an increase in beta- and delta-band activity (Reference Williamson, Kutcher and CooperWilliamson 1989), whereas positive symptoms are correlated with increased theta and delta activity (Reference Fehr, Kissler and MorattiFehr 2001). Interestingly, a study of qEEG findings in late-onset schizophrenia (n = 10) showed no significant abnormality, thus raising the possibility of a biological difference in the disorder based on the age at onset (Reference Reeves and StruveReeves 2003).
P300 and P50 waves
The finding of reduction in auditorily evoked P300 wave activity and increased P300 latency among patients with schizophrenia is robust, unlike visually evoked P300 potentials (Box 2). As the visual P300 potentials are influenced by clinical variables, one hypothesis is that auditory P300 is a trait marker of schizophrenia, whereas visual P300 is more of a state marker (Reference FordFord 1999). Auditory P300 abnormalities have been detected irrespective of the stage of illness (including presentations in the prodromal stage, in individuals who are not actively manifesting symptoms and are considered to be in remission, and in individuals with a family history of schizophrenia) and also in people with schizotypal personality (Reference Van der Stelt, Lieberman and Belgervan der Stelt 2005). These groups also show a prolonged auditory P300 latency. Sagittal reductions in P300 cognitive event-related potentials and reduced left temporal voltage related to asymmetry in the posterior superior temporal gyrus have been reported in patients with schizophrenia. A note of caution is that although the reduction in left temporal voltage has been replicated, many studies have failed to establish it robustly, possibly because of patient selection and response mode.
BOX 2 Event-related potentials
Event-related potentials (ERPs) are recorded while the individual is performing a sensory or cognitive task. They reflect the summated activity of network ensembles active during the task and are characterised by a specific pattern called the waveform, which is composed of negative and positive deflections (waves). For example, a target stimulus detected amid a series of other non-target stimuli produces a positive wave around 300 ms (latency) after the stimulus. This is known as the P300 response.
Sensory gating refers to the pre-attentional habituation of responses to repeated exposure to the same sensory stimulus. P50 is an electroencephalogram (EEG) event-related potential waveform used to assess sensory gating.
Abnormal P50 sensory gating correlates due to defects in attention have been found not only in people with schizophrenia but also in their relatives (Reference Clementz, Geyer and BraffClementz 1998).
In a meta-analysis looking at the usefulness of spectral EEG as a diagnostic tool for schizophrenia, Reference Boutros, Arfken and GalderisiBoutros et al(2008) draw the conclusion that most of the studies they looked at confirmed the increased preponderance of slow rhythms in people with schizophrenia, irrespective of their treatment status. However, they point to the lack of robust data on the sensitivity and specificity of the findings and the lack of multicentre studies using standardised criteria. Reference Shagass, Roemer and StraumanisShagass et al(1984) reported a sensitivity of 50% and a specificity of 90% in a comparison of individuals with schizophrenia and those with depression using slow-wave rhythms as a diagnostic marker.
Affective disorders
Mania
A review by Reference Shelley, Trimble and BoutrosShelley et al(2008) reports that EEG abnormalities such as small sharp spikes, 6/s spike and positive spikes are more common in patients with mania than in those with depression, in female than in male patients with bipolar disorder, and in non-familial cases of late-age onset mood disorder. Findings such as small sharp spikes, 6/s spike and wave complexes and positive spikes in suicidal patients are of ambiguous clinical significance. There is some evidence to show that interhemispheric asymmetry and decreased coherence of the EEG trace in bipolar disorder can be used to differentiate it from schizophrenia. Anteroposterior P300 topography also differentiates schizophrenia from bipolar disorder with psychotic mania. One study (n = 35 with schizophrenia, n = 20 with psychotic mania) found posterior P300 reductions in the schizophrenia group and anterior reductions in the bipolar group (Reference Salisbury, Shenton and McCarleySalisbury 1999).
Depression
Depression is the most common mental disorder experienced by people with epilepsy (Reference KannerKanner 2003). Consequently, even if epilepsy has not been diagnosed, EEG findings for people with depression need to be interpreted with caution as they may indicate underlying epileptic disorder rather than being related to depression.
Resting EEG shows greater alpha-wave activity with eyes closed in patients with depression, which has been interpreted as indicating a reduction in cortical activity in depression. Similarly, increased alpha and beta activity has been reported in patients with psychotic depression, but this should be interpreted with caution because such changes can be brought on by metabolic alterations due to poor nutrition (Reference Margerison, Anderson and DawsonMargerison 1962) or by alterations in adrenaline levels (Reference Gjessing, Harding and JennerGjessing 1967).
Visual P300 (P300 evoked using a visual stimulus) has been found to be abnormal in patients with major depressive disorder and schizophrenia (Reference Blackwood, Whalley and ChristieBlackwood 1987).
Hypnograms showing the pattern of sleep stages through the night reveal irregular transitions between the different sleep states and frequent awakening in people with depression. There is a reduction in deep-sleep stages associated with long periods of rapid eye movement (REM) sleep (Reference Benca, Obermeyer and ThistedBenca 1992). Antidepressants can resolve such irregular transitions and return the EEG pattern during sleep to normal (Reference Figueroa Helland, Postnova and SchwarzFigueroa Helland 2008).
Resting EEG has also been used to show that patients with depression who respond to fluoxetine (Reference Bruder, Sedoruk and StewartBruder 2008), imipramine (Reference Knott, Telner and LapierreKnott 1996), amitriptyline alone and with an adjunctive anticonvulsant or lithium (Reference Ulrich, Renfordt and FrickUlrich 1986) have a greater alpha power, particularly in the occipital area, than non-responders and healthy controls. Reference Bruder, Sedoruk and StewartBruder et al(2008) confirmed greater alpha waves (suggestive of lower neuronal activity) over the right than over the left hemisphere, with a high test–retest correlation. Increased pre-treatment activity in the anterior cingulate cortex has been recorded in patients who responded to citalopram and reboxetine when compared with non-responders (Reference Mulert, Juckel and BrunnmeierMulert 2007). Reference Deldin and ChiuDeldin & Chiu (2005) report from their EEG study of cognitive restructuring that patients with depression who responded to cognitive treatment exhibited greater overall cortical activity, and cortical asymmetry of greater right relative to left activity in frontal areas, than non-responders.
Pre-treatment alpha measures thus seem to be a predictor of response to antidepressants, but the studies need to be replicated with larger samples to confirm the clinical relevance of these findings.
Anxiety disorders
Although resting EEG recordings from people with anxiety disorders do not show typical deviations from normality, under experimentally induced anxiety, recordings show hypersynchronised alpha activity (Reference Herrmann and WintererHerrmann 1996).
Interestingly, a small study (Reference Arriaga and PaivaArriaga 1991) reported that EEG sleep recordings of participants with primary dysthymia showed reduced slow-wave sleep and absence of REM sleep disturbances. These indicate that biologically, primary dysthymia may be closer to generalised anxiety disorder than to affective disorder.
People with panic attacks accompanied by agoraphobic disorders show significant decreases in the power density of the alpha rhythm and increases in the power density of the beta rhythm in the right hemisphere (Reference GordeevGordeev 2008). This reflects a significant activation of the ascending mesencephalic reticular formation. The most characteristic feature in people with panic attacks without agoraphobia is a significant increase in the power density of the theta rhythm in temporal areas of the right hemisphere, reflecting increased activity in temporolimbic structures.
People with obsessive–compulsive disorder (OCD) have increased current density for delta waves in the insula and for beta waves in the frontal, parietal and limbic lobes. Reference Prichep, Mas and HollanderPrichep et al(1993) showed that a group of patients who had a relative excess of alpha waves responded well to serotonin reuptake inhibitors for their OCD.
Studies (Reference Jokic-Begic and BegicJokic-Begic 2003; Reference Chae, Jeong and PatersonChae 2004) show that people with post-traumatic stress disorder (PTSD) have globally reduced complexity in their resting EEG wave forms. However, contrary to almost all hypotheses, Reference Shankman, Silverstein and WilliamsShankman et al(2008) found that individuals with PTSD did not differ from controls on resting EEG asymmetry.
Personality disorders
Enhanced cortical slow activity in the delta range has been consistently reported in violent criminal offenders. Increased slow-wave activity in adolescence has been shown to predict emergence of antisocial behaviour later in life (Reference Raine, Venables and WilliamsRaine 1990). Attenuated alpha rhythm and increase in theta and delta activity in the frontal lobe has been found in violent offenders with antisocial personality disorder (Reference Reyes and AmadorReyes 2009). Diminished P300 activity across varied activity has been reported in people with aggression and impulsivity (Reference Gerstle, Mathias and StanfordGerstle 1998) and in individuals diagnosed with antisocial personality disorder (Reference Bauer, O'Connor and HesselbrockBauer 1994).
Substance misuse and medications
Alcohol, illicit drugs and prescribed medications (discussed under particular psychiatric disorders) significantly affect EEG recordings. As expected, this influence is greatest on alpha-wave patterns, the dominant pattern seen in the wake state, with increased activity seen in cannabis and cocaine withdrawal and decreased activity in opioid misuse.
In alcohol withdrawal, EEG traces may show attenuation of voltage and prominence of beta activity. The findings of a study (Reference Moeller, Gillin and IrwinMoeller 1993) that compared the sleep patterns of people with primary major depression and with major depression secondary to alcohol dependence suggest an additive effect of alcohol dependence and comorbid depression on sleep, with changes to delta sleep, REM and non-REM sleep patterns.
Electroconvulsive therapy
During electroconvulsive therapy (ECT), EEG recordings show a sequence of high-voltage sharp waves and spikes. This is followed by rhythmic slow waves with a definite endpoint. Reference Wahlund, Piazza and von RosenWahlund et al(2009) examined frequency distributions of ictal EEG after ECT stimulation in diagnostic subgroups of depression. Psychotic depression has a high occurrence of delta and theta waves, unipolar depression has a high occurrence of delta, theta and gamma waves, and bipolar depression has a high occurrence of gamma waves (accuracy 94%).
The EEG request
When to ask for an EEG
It is common sense to consider EEG under certain specific circumstances, such as those listed in Box 3. This list is neither prescriptive nor exhaustive, but it implies that clinicians should be highly vigilant for possible organic factors underlying any psychiatric presentation.
BOX 3 Some situations in which an EEG might be requested
An EEG might be requested:
-
• to interpret paroxysmal activity
-
• to analyse sleep disorders
-
• to establish the cause of cognitive decline when there is a suspicion of Huntington's disease, Creutzfeldt–Jakob disease, chronic delirium, etc.
A serial, ambulatory or video EEG may be useful in:
-
• interpreting periodic behavioural dysfunction
-
• distinguishing certain types of seizures
-
• and, to certain extent, differentiating between true and pseudo-seizures
What information to provide?
The physiologist or physician who reports on an EEG must have access to all relevant clinical information pertaining to the case being investigated. Box 4 lists the most commonly useful information.
BOX 4 Information to submit with the EEG request
-
• Description of symptoms, including onset, frequency and duration
-
• Triggers for those symptoms (e.g. hyperventilation)
-
• Relevant medical history
-
• Relevant psychiatric history
-
• Family history of neurological or neuropsychiatric disorders
-
• Reports and traces from previous EEGs
-
• Medications, particularly those known to cause EEG abnormalities
-
• Results from other investigations
-
• Differential diagnoses under consideration
Training issues
The most important problem highlighted in studies of current practice is the gap in knowledge among psychiatrists, physicians, physiologists and EEG technicians about utilising and interpreting EEG in patients with psychiatric disorders. Although it is probably practically unfeasible and clinically unnecessary to have an EEG laboratory physically attached to a psychiatric unit, having a laboratory in close proximity to the unit can facilitate collaborative work between psychiatrists and EEG technicians, resulting in a more integrated and appropriate use of EEG (Reference Pogarell, Hegerl and BoutrosPogarell 2005).
Psychiatrists require robust training in the diagnostic usefulness of EEG and interpretation of results, and physicians, physiologists and technicians should have a working knowledge of relevant psychiatric disorders. Psychiatric trainees should be encouraged to participate in case discussions with colleagues regarding EEG. The Royal College of Psychiatrists’ curriculum should continue to demand that budding psychiatrists have a good knowledge of this investigation and its clinical relevance. A CPD module on EEG would be of much benefit to both psychiatric trainees and mature clinicians, for whom it might serve as a refresher course.
Conclusions
The application of EEG in psychiatry is in understanding the aetiology and pathological mechanisms of psychiatric disorders, thus aiding clinical diagnosis and guiding appropriate treatment. It can be useful in clinical scenarios where there is a suspected interplay between functional symptoms and organic aetiology. Psychiatrists should base their use of EEG on robust clinical assessment and should be aware of the limitations inherent in the procedure.
MCQs
Select the single best option for each question stem
-
1 Research has shown that:
-
a clozapine therapy is a significant predictor of abnormal EEG recordings
-
b the false-positive rate for an epileptiform pattern on EEG traces in general population is less than 1%
-
c a third of abnormal EEG traces lead to change in diagnosis
-
d psychiatric referrals have a high abnormality detection rate because they are extremely selective
-
e the most common request for EEG is to determine the presence of organic brain dysfunction.
-
-
2 In EEG:
-
a auditory stimulation is the most common procedure used in activated EEG
-
b the frequency range of recorded waves is between 1 and 20 Hz
-
c in old age the trace shows a decrease in delta activity
-
d the normal eyes-closed wake EEG trace is characterised by highly rhythmic delta waves
-
e standard recording uses 14 electrodes.
-
-
3 The earliest change in EEG due to acute confusional state is:
-
a the appearance of delta waves
-
b generalised theta waves
-
c intermittent delta activity
-
d slowing of the alpha rhythm frequency
-
e triphasic waves.
-
-
4 During sleep, the onset of K-complex and spindles is seen in:
-
a stage 1 non-REM
-
b stage 2 non-REM
-
c stage 3 non-REM
-
d stage 4 non-REM
-
e REM stage.
-
-
5 In EEG of people with schizophrenia:
-
a increased beta activity is common among patients treated with antipsychotics
-
b increased delta activity in the left temporal area is associated with negative symptoms
-
c antipsychotic medications decrease alpha activity
-
d relatively high mean alpha is a specific finding
-
e 6/s spike and wave complex are common in patients with command hallucinations.
-
MCQ answers

1 | a | 2 | c | 3 | d | 4 | b | 5 | b |
eLetters
No eLetters have been published for this article.