8.1 Setting Up the Field
Algorithms – generally understood as sequences of precise instruction unambiguously specifying how to execute a task or solve a problem – are such a natural ingredient of regulation that some may wonder whether regulation could even be understood without recognising its algorithmic features, and without realising algorithms as a prime subject for regulation. In terms of the algorithmic features of regulation, somewhat simplistically and without suggesting in any way that the algorithmic language captures regulation in its entirety – far from it – algorithms are relevant to the three dimensions of regulation: the regulatory process, the modalities of regulation, and the regulatory approaches (or attitudes). By the regulatory process, we refer to the process that, stylistically, commences with political and economic pressures to find a solution to a certain problem and continues with the formation of policy goals, data gathering, and the mapping of possible regulatory responses to achieve these goals (which ought to include the sub-processes of regulatory impact assessment upon choosing the preferred measure). The chosen measures are translated into regulatory norms and implemented (or enforced), resulting, if all went well, with some improvement of the conditions related to the initial social problem (as can be analysed by a back-end regulatory impact assessment). By regulatory modalities, we mean the set of regulatory measures available to the state (or, more accurately, to state agencies acting as regulators): regulation through (and of) information, permits and licensing, civil, administrative and criminal liability, taxes and subsidies, or insurance schemes. By regulatory approaches, or attitudes, we mean the top-down command and control attitude, performance-based regulation, and the managerial approach, with the latter two also including co-regulation or private regulation.
Algorithms are relevant to all three dimensions of regulation, as they may assist most, if not all, stages of the regulatory process, may inform or even be a component of the regulatory modalities, and may similarly inform and be integrated into the regulatory attitudes. Conversely, algorithms may be the subject matter of regulation. Their development and deployment may be considered (as part of) the social problem triggering the regulatory process; they may then enlist one or more of the regulatory modalities to address the structure of incentives that generate harmful use of algorithms, and stand at the focal point of the policy question regarding which regulatory attitude fits best to address the host of risks associated with algorithms, and in particular with machine learning and AI.
In the following section, we will first introduce a general concept of an algorithm, which then can be applied both to human action and to computer systems. On this basis, we shall consider the jurisprudential debate on prospects and limits of ‘algorithmicisation’ or ‘mechanisation’ of law and government.
We shall then address computer algorithms and consider the manner in which they have progressively entered government. We shall focus on artificial intelligence (AI) and machine learning, and address the advantages of such technologies, but also the concerns their adoption raises. The motivation of this analysis is to shed an important light on the relationship between the state and AI, and on the need to consider regulating the state’s recourse to algorithms (including via attention to the technology itself, usually referred to as ‘regulation by design’).
8.2 Algorithmic Law before Computers
An algorithm, in the most general sense, is a sequence of instructions (a plan of action, or a recipe) that univocally specifies the steps to be accomplished to achieve a goal, as well as the order over such steps.Footnote 1 It must be directed to executors that are able to exactly perform each of the steps indicated in the algorithm, in their prescribed order. The order may include structures such as sequence (first do A, then B), conditional forks (if A is true then do to B, otherwise do C), or repetitions (continue doing B until A is true).
The execution of an algorithm should not require a fresh cognitive effort by the executor, when the latter is provided with a suitable input: every action prescribed by the algorithm should either be a basic action in the repertoire of the executor (such as pushing a button or adding two digits) or consist of the implementation of an algorithm already available to the executor. Algorithms, in this very broad sense, may be directed to humans as well as to automated systems.
Precise and univocal instructions to use hardware or software devices, install appliances, get to locations, or make mathematical calculations, can be viewed as algorithms. There is, however, a special connection between algorithms and computations. The term ‘algorithm’ in fact derives from the name of a Persian scholar, Muhammad ibn Mūsā al-Khwārizmī, who published in the 9th century a foundational text of algebra, providing rules for solving equations, with practical applications, in particular in the division of inheritance. The idea of a mathematical algorithm however is much earlier. For instance, the Greek mathematician Euclid is credited with having invented, in the 4th century BC, an algorithm for finding the greatest common divisor between two integer numbers.
In any case, algorithms, as plans meant to have a ‘mechanical’ implementation (i.e., whose execution does not require a fresh cognitive effort nor the exercise of discretion), should always lead to the same outcome for the same input, whenever they are entrusted to a competent executor. This idea is often expressed by saying that algorithms are deterministic or repeatable (though, as we shall see, some algorithms go beyond this idea; i.e., they also include elements of randomness).
The idea that at least some state activities could be governed by algorithms in a broad sense – unambiguous and repeatable impersonal procedures, leading to predictable decisions according to precise rules – was viewed as a characteristic feature of modern bureaucracies by the social theorist Max Weber according to whom: ‘The modem capitalist enterprise rests primarily on calculation and presupposes a legal and administrative system, whose functioning can be rationally predicted, at least in principle, by virtue of its fixed general norms, just like the expected performance of a machine.’Footnote 2
The same Weber, however, also observed an opposite tendency in contemporary administration and adjudication, namely, the pressure toward ‘material justice’, which evades air-tight codification because it is concerned with the effective pursuit of interests and values. Approaching the exercise of administrative and judicial power as a goal-directed activity, meant to satisfy certain interests or values rather than satisfying exact application of rules, involves, to some extent, an original cognitive effort by decision makers. Some discretion in the identification of the interests or values to be pursued, as well as choices regarding the means to achieve them, cannot be avoided. This cognitive presence, in turn, is a site of agency, representing substantive, or material, moral reasoning (and, it seems, not only rationality but also empathy, and perhaps other virtuous sensibilities and emotions). We will return to this matter when we further discuss machine-generated (i.e., learnt) algorithms (sometimes referred to as AI).
Focusing on adjudication – a key function of the state in exercising its official power – the ideal of a mechanical (or algorithmic, as we say today) approach has most often been the target of critique. Adjudication in many cases cannot, and indeed should not, be reduced to the application of precisely defined rules. The very term ‘mechanical jurisprudence’ was introduced, more than a century ago, by US legal theorist Roscoe Pound,Footnote 3 in a critical essay where he argued that judicial decision-making should not consist of the ‘automatic’ application of precedents’ rulings, legislative rules, and legal conceptions. Pound stated that such an approach, to the extent that it is viable, would have the law depart from shared ideas of correctness and fair play, as understood by citizens, and would lead to the law being ‘petrified’, and more generally unable to meet new challenges emerging in society, to ‘respond to the needs of present-day life’.
A similar criticism against a ‘mechanical’ application of the law can be found via a famous US justice at the time, Oliver Wendell Holmes, who made two related somewhat polemical claims: the claim that ‘general propositions do not decide concrete cases’Footnote 4 and the claim that ‘the life of the law has not been logic: it has been experience’.Footnote 5 These two claims clarify that Holmes is attacking the view that the application of the law is a mere matter of deductive inference, namely, a reasoning process that only derives, relative to the facts of a case, what is entailed by pre-existing general premises and concepts. Holmes argued that, on the contrary, the application of law should be geared toward the social good, which requires officers, and in particular judges ‘to consider and weigh the ends of legislation, the means of attaining them, and the cost’.Footnote 6 However, if considered more carefully, Holmes’s perspective while rejecting the algorithmic application of the law (premised on mechanical jurisprudence), as it requires decision makers to obtain knowledge that is not included in legal sources, still adopts a restrictive approach to legal decision-making (premised on optimising a given object, based on past practice). Following this idea, the interpretation and application of the law only require fresh knowledge of social facts – that is, a better understanding (data and analysis) of experience, a clear formulation of the ends of legislation, and a good formula for assessing costs of applying the means towards these ends. It does not involve a creative and critical normative assessment of the goals being pursued and the side-effects of their pursuit, in the given social contexts.
A number of different currents in legal thinking have developed providing descriptive and prescriptive arguments that judges do not and indeed should not apply the law mechanically; they do, and should, rather aim to achieve values, pertaining to the parties of a case and to society at large. We cannot here do justice to such approaches; we can just mention, as relevant examples, the following: legal realism, sociological jurisprudence, interest-jurisprudence, value jurisprudence, free law, critical legal studies, and so forth. According to some of these approaches, the objections against rigid or static approaches to the law have gone beyond the advocacy of teleological reasoning as opposed to the application of given rules and concepts. Rather, it has been argued that legal problem solving, properly understood, goes beyond optimising the achievement of given goals, especially when such goals are limited to a single purpose such as economic efficiency or even welfare.Footnote 7 On the contrary, legal reasoning also includes the reflective assessment and balancing, of multiple social and individual values, which often presuppose moral or political evaluations and processes of communication and justification, inspired by deliberative ideas of integrity and meaningful belonging in a community.Footnote 8
The view that the application of the law is not algorithmic or deductive has also been endorsed by authors that argued that the (private) law should not serve political aims, but rather focus on its ‘forms’, namely, on the internal coherence of its concepts, and its ability to reflect the nature of legal relations and the underlying theory of justice.Footnote 9
A criticism of mechanical approaches to adjudication (and administrative decision-making) can also be found in analytical legal theorists. Hans Kelsen made the radical claim that legal norms never determine a single outcome for individual cases: they only provide a frame for particular decisions; their application requires discretion since ‘every law-applying act is only partly determined by law and partly undetermined’.Footnote 10 For Kelsen, the relationship between a rule and the application in a particular case is always a site for judgment. More cautiously, H. L. A. Hart affirmed that it is impossible to make ‘rules the application of which to particular cases never calls for a further choice’. Enacted laws are meant to address the prototypical cases that the legislator had envisaged; un-envisaged cases may require a different solution that has to be found outside of the legislative ‘algorithm’, by exercising choice or discretion, that is, by ‘choosing between the competing interests in the way which best satisfies us’.Footnote 11 For Hart, then, cases that fall in greyer areas (relative to the core paradigmatic cases envisioned by the norm-giver) are sites of greater discretion. The question then becomes how to differentiate between the core and the penumbra – whether based solely on a conventional understanding of the words used by the rule, or whether also based on the purpose of the rule. A teleological approach may be needed since legal rules are performative (i.e., require action by those governed by the rules), so that the purpose of a rule may inform its meaning. In the latter case, applying the rule requires discretion regarding which application would further the purpose, and whether exceptions exist (either because the conventional meaning may disrupt the purpose or because a non-conventional meaning would further the purpose better).
This brief survey of leading approaches to jurisprudence demonstrates that the application of law is not merely algorithmic, but rather relies upon the discretion of the decision maker, whenever the norms (embedded in legislation or case-law) do not dictate a single outcome to a decisional problem. It is true that some authors have strongly reiterated the view that in order to effectively direct and coordinate the action and the expectations of citizens and officers, the law should provide clear if-then rules specifying the link between operative facts and corresponding rights and obligations (and other legal effects).Footnote 12 However, there is an apparent consensus that legal decision-making cannot be fully driven by rules (or algorithms) alone; it calls for teleological and value-based reasoning and for the assessment of uncertain factual situations, with regard to the specific cases at stake.Footnote 13 Other authors have observed that even when the application of a general norm to given facts is needed, matching the general terms in the norm to the features of specific factual situations involves a ‘concretisation’ of the norm itself, namely, it requires enriching the indeterminate content of such terms, as needed to determine whether they apply or not to the given facts.Footnote 14 Applying the law, therefore, requires that the decision-maker engages in a genuine cognitive effort. This effort may involve interlinked epistemic and practical inquiries: determining the relevant facts and correlation between them, assessing accordingly the impacts that alternative choices may have on relevant interests and values, and determining accordingly which choice is preferable, all things considered. Discretion may also include honing the contours of the values or interests to be pursued, as well as their relative importance. This broad idea of discretion also includes proportionality assessments under constitutional law, which aim to determine whether an infringement of constitutional rights is justified by pursuing non-inferior advantages with regard to other constitutional rights and values, and by ensuring that no less- infringing choice provides a better trade-off.Footnote 15
So far, we have focused on algorithmic approaches to judicial decision-making, which usually involves disputes about the facts of a case or about the interpretation of the applicable legal norms, so that reasoned choices are needed to come to a definite outcome. But legal decisions, on a daily basis, are entered not only – in fact, not predominantly – by judges. Rather, public agencies (sometimes referred to as ‘administrative’ or ‘bureaucratic’ agencies) apply the law routinely, on a large scale. In some domains, such as tax and social security, a complex set of rules, often involving calculations, is designed to minimise discretion and therefore appears to be amenable to ‘algorithmic’ application (even before the computerisation of public administration). Even though controversies are not to be excluded in the application of such regulations, often the facts (i.e., data) are available to the agency per each case (usually as a result of rather precise rules governing the submission of such data), to which precise rules can then be applied, to provide definite outcomes that in standard cases will withstand challenge (if the rules are applied correctly).
In these domains too, however, fully eliminating discretion may undermine the purpose of the scheme and thus not only be counter-productive but also potentially raise legal validity concerns, to the extent the legal system includes more general legal principles according to which particular rules incompatible with the purpose of the statutes (or the values of the constitution) are subject to challenge. More specifically, a tension may emerge on occasion between the strict application of rules and a call, based on the purposes of the empowering statute (or on more general legal principles and values), to take into account unenumerated particular circumstances of individual cases. For instance, in social security, there may be a tension between taking into account the conditions of need of benefit claimants and applying a law that appears prima-facie not to include such claimants.
More generally, we may observe that algorithms – whether computerised or not – are less applicable when the legal terrain is not paved fully by rules but is interspersed with standards, which by definition are more abstract and thus less amenable to codification based on the clear meaning of the language (more on that in Section 8.10). Moreover, analytically, algorithms are less applicable when more than one norm applies (without a clear binary rule on which norm trumps in case of potential clashes). This is often the case, as various rules on different levels of abstraction (including, as mentioned, standards) may apply to a given situation. Lastly, it should be noted that the debate on mechanical application of the law has thus far assumed a rather clear distinction between the application of a legal norm and the generation (or enactment) of the norm. At least in common law jurisdictions, this distinction collapses, as application of norms (precedents or statutes) is premised on interpretation, which may lead to refining the existing doctrine or establishing a novel doctrine. Norm-generation is even less amenable to algorithmicising, as it is difficult (for humans) to design rules that optimise this process, given the value-laden nature of generating legal norms.
The general conclusion we can derive from this debate is that the application of the law by humans is governed by algorithmic instructions only to a limited extent. Instructions given to humans concern the substance of the activities to be performed (e.g., the legal and other rules to be complied with and implemented, the quantities to be calculated, the goals to be aimed at, in a certain judicial or administrative context). They do not address the general cognitive functions that have to be deployed in executing such activities, such as understanding and generating language, visualising objects and situations, determining natural and social correlations and causes, and understanding social meaning. In particular, the formation and application of the law requires engaging with facts, norms, and values in multiple ways that evade capture by human-directed algorithmic instructions. Consider the following: determining what facts have happened on the basis of evidence and narratives; ascribing psychological attitudes, interests, and motivations to individuals and groups on the basis of behavioural clues; matching facts and states of mind against abstract rules; assessing the impacts of alternative interpretations/applications of such rules; making analogies; choosing means to achieve goals and values in new settings; determining the contours of such goals and values; quantifying the extent to which they may be promoted or demoted by alternative choices; assessing possible trade-offs. Even when officers are provided with plans to achieve a task, such plans include high-level instructions, the implementation of which by the competent officers requires human cognitive activities, such as those listed previously, which are not performed by implementing handed-down algorithmic commands. Such activities pertain to the natural endowment of the human mind, enhanced through education and experience, and complemented with the intelligent use of various techniques for analysis and calculations (e.g., methods for general and legal argumentation, statistics, cost-benefit analysis, multicriteria decision-making, optimisation, etc.). They result from the unconscious working of the neural circuitry of our brain, rather than from the implementation of a pre-existing set of algorithmic instructions, though qualitative and quantitative models can also be used in combination with intuition, to analyse data, direct performance, detect mistakes, and so forth.
But the question remains: does the problem lie with algorithms, in the sense that algorithms are inherently unsuited for tasks involving learning or creativity, or with humans, in the sense that the human condition (the way we acquire and process information, based on our natural endowment) is incompatible with engaging in such tasks by following algorithmic instructions? Put differently: is it the case that no set of algorithmic instructions, for any kind of executor, can specify how to execute such tasks, or rather that humans are unable to engage with such tasks by diligently executing algorithmic specifications given to them, rather than by relying on their cognitive competence?
A useful indication in this regard comes from the psychologist David Kahneman, who distinguishes two aspects of the human mind:
System 1 operates automatically (i.e., without the need of a conscious choice and control) and quickly, with little or no effort and no sense of voluntary control.
System 2 allocates attention to the effortful mental activities that demand it, including complex computations.Footnote 16
If following algorithmic instructions for humans requires exploiting the limited capacities of system 2 (or in any case the limited human capacity to learn, store and execute algorithms), then the human capacity for following algorithmic instructions is easily overloaded, and performance tends to degrade, also with regard to tasks that can be effortlessly performed when delegated to system 1. Therefore, some of the tasks that system 1 does automatically – those tasks that involve perception, creativity, and choice – cannot be performed, at the human level, by implementing algorithmic instructions handed in to a human executor. However, this does not mean, in principle, that such instructions cannot be provided for execution to a machine, or to a set of high-speed interconnected machines.Footnote 17
As we shall see in the following sections, machines can indeed be provided with algorithmic specifications (computer programs), the execution of which enables such machines to learn, in particular by extracting knowledge from vast data sets. This learned knowledge is then embedded in algorithmic models that are then used for predictions (and even decisions). As machines can learn by implementing algorithmic instructions, contrary to humans, the algorithmic performance of state functions though machines could expand beyond what is algorithmically possible to humans. Algorithms for learning can provide machines with the ability to adapt their algorithmic models to complex and dynamic circumstances, predict the outcome of alternative courses of action, adjust such predictions based on new evidence, and act accordingly.
Nevertheless, this does not mean that all tasks requiring a fresh cognitive effort by their executors can be successfully performed in this way today or in the near (or even mid-range) future; some can, and others cannot. We will address such issues in the following sections, as we turn our attention to state activities and the possible integration of algorithms into the apparatus of state agencies.
8.3 Computer Algorithms before AI
In the previous section, we considered the possibility of adopting an ‘algorithmic approach’ toward human activities concerned with the formation and application of the law, and more generally to state functions concerned with the administration of official functions. We have observed that such an algorithmic approach to decision-making within government existed much before the introduction of computers, but that it had a limited application. In this section, we consider the changes that have taken place following the automation of the execution of algorithms within government with the assistance of computer systems. Before moving into that, we need to discuss the nature of computer algorithms. Computer algorithms correspond to the general notion of an algorithm introduced previously, with the proviso that since such algorithms are directed to a computer system, the basic actions they include must consist of instructions that can be executed by such a system.
To make an algorithm executable by a computer, it must be expressed in a programming language, namely, in language that provides for a repertoire of exactly defined basic actions – each of which has a clear and univocal operational meaning – and for a precise syntax to combine such actions. Different programming languages exist, which have been used at different times and are still used for different purposes. In every case, however, the instructions of all such languages are translated into operations to be performed by the computer hardware, namely, in arithmetical operations over binary numbers. This translation is performed by software programs that are called compilers or interpreters. The automated execution of algorithms has much in common with the human execution of algorithms, when seen at a micro-level (i.e., at the level of single steps and combinations of them). This analogy, however, becomes more and more tenuous when we move to the macro level of complex algorithms, executed at super-high speed and interacting with one another.
The variety of algorithms (computer programs) which are and have been used within public administrations for different functions is amazingly vast. However, it may be possible to distinguish three key phases: a computer revolution, an Internet revolution, and finally an AI revolution, each of which has brought about a qualitative change in state activities.
The computer revolution consisted in the use of computers to perform what could be taken as routine tasks within existing state procedures, typically for making mathematical calculations, storing, retrieving data, and processing data. The history of computing is indeed, from its very beginning, part of the history of the modern states. Many of the first computers or proto-computers were built in connection with public activities, in particular in relation to warfare, such as decoding encrypted messages (e.g., the Colossus, developed in the UK in 1942) and computing ballistic trajectories (e.g., Harvard Mark I and Eniac in the US). Other state tasks to be conferred to computers were concerned with censuses (IBM was born out of the company that automated the processing of population data before computers were available) and the related statistics, as well as with scientific and military research (e.g., for space missions).
However, it was the use of computers for keeping vast sets of data (databases), and the retrieval and processing of the data, that really made a difference in more common governmental operations. Databases were created in all domains of public action (population, taxation, industries, health, criminal data, etc.), and these data sets and the calculations based on them were used to support the corresponding administrative activities. This led to a deep change in the governmental information systems, namely, in those socio-technical structures – comprised of human agents, technologies, and organisational norms – that are tasked with providing information to governments. The ongoing collecting, storing, and processing of data were thus integrated into the operational logic of the modern state (characterised by providing basic services and regulating the industry as well as the provision of these services). In a few decades, states have moved from relying on human information systems, based on paper records created and processed by humans, to hybrid information systems in which humans interact with computer systems. Multiple computer systems have been deployed in the public sphere to support an increasing range of administrative tasks, from taxation, to social security, to accounting, to the management of contracts, to the administration of courts and the management of proceedingsFootnote 18. As of the 1980s, personal computers entered all public administrations, providing very popular and widespread functions as text processing and spreadsheets, which increased productivity and facilitated digitisation. However, this technological advance did not, in and of itself, change the fundamental division of tasks between humans and automated devices, computers being limited to routine tasks supporting human action (and providing data to humans).Footnote 19
The emergence of networks, culminating with the Internet (but comprising of other networks as well), brought a fundamental change in the existing framework, as it integrated computational power with high-speed communications, enabling an unprecedented flow of electronic data. Such flow takes place between different government sections and agencies, but also between government and citizens and private organisations (and of course within the private sphere itself). Even though the private sector was the driving force in the development of the Internet, it would be a mistake to ignore the significant role of the government and the deep impact of digitised networks for the manner in which public institutions go about their business. Recall that the initial thrust for the Internet was generated by the Defence Advanced Research Projects (DARPA) of the US government. The security establishment has not withdrawn from this realm ever since (although its activities remain mostly behind the scenes, until revealed by whistle-blowers, such as Snowden). Focusing on the civil and administrative facets of modern governments, and in particular on the tools of government in the digital era, Hood and Margetts observed that all different modalities through which the government may exercise influence on society were deeply modified by the use of computers and telecommunication. They distinguish the four basic resources which the government can use to obtain information from and make an impact on the world: nodality (being at the centre of societal communication channels), authority (having legal powers), treasure (having money and other exchangeable properties), and organisation (having administrative structures at their service). They note that in the Internet era, the flow of information from government to society has increased due to the ease of communications and the availability of platforms for posting mass amounts of information online.
Moreover, and perhaps more importantly, the provision of public services through computer systems has enabled the automated collection of digital information as well as the generation of automated messages (e.g., pre-compiled tax forms, notices about sanctions, deadlines, or the availability of benefits) in response to queries. The exercise of authority has also changed in the Internet age, as the increased possession of digital information about citizens enables states to automatically detect certain unlawful or potentially unlawful behaviour (e.g., about tax or traffic violations) and trigger corresponding responses. Tools to collect and filter information offline and online enable new forms of surveillance and control. Regarding the treasury, payment by and by the government has increasingly moved to electronic transfers. Moreover, the availability of electronic data and the automation of related computation has facilitated the determination of entitlements (e.g., to tax credits or benefits) or has allowed for automated distinctions in ticketing (e.g., automatically sanctioning traffic violations, or charging for transportation fees according to time of the day or age of the passenger).
Finally, the way in which governmental organisations work has also evolved. Not only the internal functioning of such organisations relies on networked and computerised infrastructures, but digital technologies are widely used by governmental agencies and services to collect and process information posted online (e.g., intercept telecommunications, analyse Internet content), as well as deploying other networked sensors (e.g., street cameras, satellites and other tools to monitor borders, the environment, and transfers of goods and funds).
To sum up this point, we may say that in the Internet era the internal operation of the state machinery (in particular, the bureaucracy), and the relation between government and civil society is often mediated by algorithms. However, this major development, in which considerable segments of the daily activities of the government are exercised through computer networks (i.e., algorithms), is primarily confined to routine activities, often involving calculations (e.g., the determination of taxes and benefits, given all the relevant data). This idea is challenged by the third wave of algorithmic government, still underway: the emergence of AI, to which we now turn.
8.4 Algorithms and AI
The concept of AI covers a diverse set of technologies that are able to perform tasks that require intelligence (without committing to the idea that machine intelligence is ‘real’ intelligence), or at least tasks that ‘require intelligence if performed by people’.Footnote 20 AI systems include and possibly integrate different aspects of cognition, such as perception, communication (language), reasoning, learning, and the ability to move and act in physical and virtual environments.
While AI has been around for a few decades – in 1950 Alan Turing pioneered the idea of machine intelligence,Footnote 21 and in 1956 a foundational conference took place in Dartmouth, with the participation of leading scientistsFootnote 22 – only recently is AI rising to play a dominant role in governments, following and complementing AI successes in the private sector. In fact, an array of successful AI applications have been built which have already entered the economy, and are thus used by corporations and governments alike: voice, image, and face recognition; automated translation; document analysis; question-answering; high-speed trading; industrial robotics; management of logistics and utilities; and so forth. AI-based simulators are often deployed as part of training exercises. The security establishment, it has been reported, has also developed AI systems for analysing threats, following the 9/11 attacks. We are now witnessing the emergence of autonomous vehicles, and soon autonomous unmanned flying vehicles may join. In fact, in very few sectors AI is not playing a role, as a component of the provision of services or the regulation of society, in the application and enforcement segments or the norm-generation stages.
The huge success of AI in recent years is linked to a change in the leading paradigm in AI research and development. Until a few decades ago, it was generally assumed that in order to develop an intelligent system, humans had to provide a formal representation of the relevant knowledge (usually expressed through a combination of rules and concepts), coupled with algorithms making inferences out of such knowledge. Different logical formalisms (rule languages, classical logic, modal and descriptive logics, formal argumentation, etc.) and computable models for inferential processes (deductive, defeasible, inductive, probabilistic, case-based, etc.) have been developed and applied automatically.Footnote 23 Expert systems – like computer systems including vast domain-specific knowledge bases, for example, in medicine, law, or engineering, coupled with inferential engines – gave rise to high expectations about their ability to reason and answer users’ queries. The structure for expert systems is represented in Figure 8.1. Note that humans appear both as users of the system and as creators of the system’s knowledge base (experts, possibly helped by knowledge engineers).

Figure 8.1 Basic structure of expert systems
Unfortunately, such systems were often unsuccessful or only limitedly successful: they could only provide incomplete answers, were unable to address the peculiarities of individual cases, and required persistent and costly efforts to broaden and update their knowledge bases. In particular, expert-system developers had to face the so-called knowledge representation bottleneck: in order to build a successful application, the required information – including tacit and common-sense knowledge – had to be represented in advance using formalised languages. This proved to be very difficult and, in many cases, impractical or impossible.
In general, only in some restricted domains have logical models led to successful application. In the legal domain, logical models of great theoretical interest have been developed – dealing, for example, with arguments,Footnote 24 norms, and precedentsFootnote 25 – and some expert systems have been successful in legal and administrative practice, in particular in dealing with tax and social security regulations. However, these studies and applications have not fundamentally transformed the legal system and the application of the law. The use of expert systems has remained, in the application of legal norms, and more generally within governmental activity, confined to those routing tasks where other computer tools were already in use.
It may be useful to consider the connection between algorithms and expert systems. The ‘algorithm’ in a broad sense, of such systems, includes two components: the inferential engine and the knowledge base. Both have to be created, in all their details, by humans, and may be changed only by human intervention, usually to correct/expand the knowledge base. Thus the capacity of such systems to adequately address any new cases or issues depends on how well their human creators have been able to capture all relevant information, and anticipate how it might be used in possible cases. It is true that such systems can store many more rules than a human can remember and process them at high speed, but still humans must not only provide all such rules but also be able to understand their interactions, to maintain coherence in the system.
AI has made an impressive leap forward since it began to focus on the application of machine learning to mass amounts of data. This has led to a number of successful applications in many sectors – ranging from automated translation to industrial optimisation, marketing, robotic visions, movement control, and so forth – and some of these applications already have substantial economic and social impacts. In machine learning approaches, machines are provided with learning methods, rather than (or in addition to) formalised knowledge. Using such methods, computers can automatically learn how to effectively accomplish their tasks by extracting/inferring relevant information from their input data, in order to reach an optimised end.
More precisely, in approaches based on machine learning, the input data provided to the system is used to build a predictive model. This model embeds knowledge extracted from the input data – that is, it consists of a structure that embeds generalisations over the data, so that it can be used to provide responses to new cases. As we shall see such responses are usually called ‘predictions’. Different approaches exist, to construct such a model. For instance, the model may consist of one or more decision trees (i.e., combinations of choices), based on the features that a case may possess, leading to corresponding responses. Alternatively, it can consist of a set of rules, obtained through induction, which expresses connections between combinations of features and related responses. Or it can consist of a neural network, which captures the relation between case features and responses through a set of nodes (called neurons) and weighted connections between them. Under some approaches, the system’s responses can be evaluated, and based on this evaluation the system can self-update. By going through this process again (and again), optimisation is approximated.
8.5 Approaches to Machine Learning
Three main approaches to machine learning are usually distinguished: supervised learning, reinforcement learning, and unsupervised learning.
Supervised learning is currently the most popular approach. In this case, the machine learns through ‘supervision’ or ‘teaching’: it is given in advance a training set (i.e., a large set of answers that are assumed to be correct in achieving the task at hand). More precisely, the system is provided with a set of pairs, each linking the description of a case, in terms of a combination of features, to the correct response (prediction) for that case. Here are some examples: in systems designed to recognise objects (e.g., animals) in pictures, each picture in the training set is tagged with the name of the kind of object it contains (e.g., cat, dog, rabbit); in systems for automated translation, each (fragment of) a document in the source language is linked to its translation in the target language; in systems for personnel selection, the description of each past applicants (age, experience, studies, etc.) is linked to whether the application was successful (or to an indicator of the work performance for appointed candidates); in clinical decision support systems, each patient’s symptoms and diagnostic tests is linked to the patient’s pathologies; in recommendation systems, each consumer’s features and behaviour is linked to the purchased objects; in systems for assessing loan applications, each record of a previous application is linked to whether the application was accepted (or, for successful applications, to the compliant or non-compliant behaviour of the borrower). And in our context, a system may be given a set of past cases by a certain state agency, each of which links the features of a case with the decision made by the agency. As these examples show, the training of a system does not always require a human teacher tasked with providing correct answers to the system. In many cases, the training set can be the side product of human activities (purchasing, hiring, lending, tagging, deciding, etc.), as is obtained by recording the human choices pertaining to such activities. In some cases, the training set can even be gathered ‘from the wild’ consisting of the data which are available on the open web. For instance, manually tagged images or faces, available on social networks, can be scraped and used for training automated classifiers.
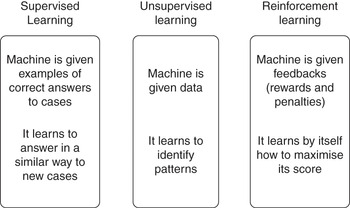
Figure 8.2 Kinds of learning
The learning algorithm of the system (its trainer) uses the training set to build a model meant to capture the relevant knowledge originally embedded in the training set, namely the correlations between cases and responses. This model is then used, by the system – by its predicting algorithm – to provide hopefully correct responses to new cases, by mimicking the correlations in the training set. If the examples in the training set that come closest to a new case (with regard to relevant features) are linked to a certain answer, the same answer will be proposed for the new case. For instance, if the pictures that are most similar to a new input were tagged as cats, the new input will also be tagged in the same way; if past applicants whose characteristics best match those of a new applicant were linked to rejection, the system will propose to reject also the new applicant; if the past workers who come closest to a new applicant performed well (or poorly), the system will predict that also the new applicant will perform likewise; if past people most similar to a convicted person turned out to be recidivists, the system will predict that the new convict will also re-offend.
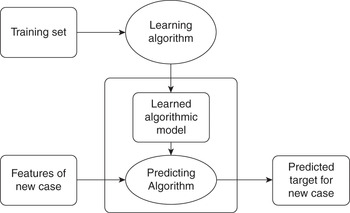
Figure 8.3 Supervised learning
Reinforcement learning is similar to supervised learning, as both involve training by way of examples. However, in the case of reinforcement learning the system also learns from the outcomes of its own actions, namely, through the rewards or penalties (e.g., points gained or lost) that are linked to such outcomes. For instance, in the case of a system learning how to play a game, rewards may be linked to victories and penalties to defeats; in a system learning to make investments, to financial gains and penalties to losses; in a system learning to target ads effectively, to users’ clicks; and so forth. In all these cases, the system observes the outcomes of its actions, and it self-administers the corresponding rewards or penalties in order to optimise the relationship between the response and the goal. Being geared towards maximising its score (its utility), the system will learn to achieve outcomes leading to rewards (victories, gains, clicks), and to prevent outcomes leading to penalties. Note that learning from one’s successes and failures may require some exploration (experimentation): under appropriate circumstances, the system may experiment with randomly chosen actions, rather than performing the action that it predicts to be best according to its past experience, to see if something even better can come up. Also note that reinforcement learning must include, at least to an extent, a predefined notion of what counts as a ‘success’.
Finally, in unsupervised learning, AI systems learn without receiving external instructions, either in advance or as feedback, about what is right or wrong. The techniques for unsupervised learning are used, in particular, for clustering – that is, for grouping the set of items that present relevant similarities or connections (e.g., documents that pertain to the same topic, people sharing relevant characteristics, or terms playing the same conceptual roles in texts). For instance, in a set of cases concerning bail or parole, we may observe that injuries are usually connected with drugs (not with weapons as expected), or that people having prior record are those who are related to weapons. These clusters might turn out to be informative to ground bail or parole policies.
8.6 AI Systems as Prediction Machines
Machine-learning systems are still based on the execution of algorithmic instructions, conveyed through software programs, as any computer is. In the end, such programs govern the functioning of a digital computer, and their execution is reduced to the simple operations of binary arithmetic performed by one or more processors. However, such algorithms are different, in an important way, from the non-learning algorithms we have described previously, including algorithms meant to govern the behaviour of humans (see Section 8.2) and algorithms directed to machines (see Sections 8.3 and 8.4).
As noted previously, the difference is that to create a non-learning algorithm, humans have to provide in advance all knowledge that is needed to address the task that the algorithm is meant to solve. Thus the use of such algorithms is restricted to the cases in which it is possible, for humans, to give in advance all such information. A further restriction comes from the extent to which a human is able to process this information (in the case of algorithms directed to humans) or to which a human is able to grasp connections and impose coherence over the information (in the case of algorithms directed to computers).
With regard to learning algorithms, we enter a different domain. Once given a training set (in supervised learning), relevant feedback (in reinforcement learning), or just a set of data (in unsupervised learning), the learning algorithm produces a predictive model (i.e., a set of rules or decision trees or a neural network) which embeds information extracted from the training set. This information basically consists of correlations between certain data on objects or events (i.e., the predictors to be used) and other data concerning the same objects or events (i.e., the targets that the system is meant to determine), based on the predictors. Thus, for instance, in a system dealing with recidivism, the model might embed the correlations between features of offenders (age, criminal record, socio-economic conditions, or any other factors) and the crimes they are expected to commit after being released.Footnote 26 In a system dealing with case law, the model may embed correlations between the textual content of the judge’s opinions (plus possibly, further codified information on the case or may other information, regarding social, political, or economic events) and the corresponding decisions. We can consider the predictive model itself (in combination with the software that activates it) as a complex algorithm, an algorithm that is not constructed by humans (who may only specify some parameters and features of it), but by the learning algorithm. The predictive model can be applied to a new object or event, given the values of the predictors for that object or event, and asked to assign corresponding values for the target. It can evolve by being further modified by the learning algorithm, so as to improve its performance. Moreover, to the extent the learning process is given access to a very large (and ever-increasing) data set, it can find within this data set statistical patterns that predict given outcomes in ways that are difficult to foresee when the algorithm was first launched.
Thus, machine learning systems can be viewed as ‘prediction machines’.Footnote 27 To understand their impact on public activities, we need to clarify this notion of prediction. Within machine learning, predicting a target datum based on a set of input data (predictors) just means to suggest what the target datum is likely to be, on account of its correlation with such input data; it consists in ‘filling the missing information’ based on the information we have.Footnote 28 Prediction in this sense does not always, though it does often, refer to future events. As examples of prediction focused on the present, consider an image recognition system that labels pictures (as dogs, cats, humans, etc.), face recognition systems that label faces (with people’s names), or a diagnostic system that labels radiographies with possible pathologies. For predictions focused on the future, consider a system that predicts the likelihood that a person will have a certain health issue, or that a certain student admitted to a university will do well, that an applicant for parole will escape or engage in criminal activities, that a traffic jam will happen, or that crimes are likely to take place in a certain area of a city under certain circumstances.
Having systems that can make predictions, in a cheap and effective way, has three distinct implications:
Predictions currently made by humans will, partially or completely, be delegated to machines, or in any case machine predictions will be integrated with human ones.
A much larger number of predictions will be executed, in a broader set of domains.
A much larger set of data will be collected to enable automated predictions.
Moreover, the learning process may reveal factors that we have not yet realised to be relevant to the ‘correct’ outcome or may even suggest a different outcome as a correct outcome, if such an outcome correlates better with other outcomes identified as preferable.
8.7 From Prediction to Action
Automated predictions may empower decision makers by enabling them to better assess the situation at stake and take consequential actions. Alternatively, such actions too may be entrusted to an automated system. In certain cases, a system’s prediction may be subject to human control (‘human in the loop’, or ‘human over the loop’), in other cases, they may not be challenged by humans. For instance, the prediction that a patient suffers a pathology based on the automated analysis of his or her radiology is, to date, subject to endorsement by the doctor, for it to become the basis of subsequent treatment. Similarly, a prediction of recidivism has to be endorsed by a judge before it becomes the basis for a judgment. On the other hand, the prediction that there is a pedestrian in the middle of the road, for obvious reasons of time, will lead directly to the action of an autonomous car (without necessarily removing human intervention from the autonomous car altogether).
The link between prediction and decision may take place in different ways. A human may have the task of deciding what to do based on the prediction – that is, of determining whether to grant bail, or whether to approve a loan (and at which rate), after the system has predicted the likelihood that the convict will escape or recommit a crime or the likelihood of default on the loan. The choice to entrust a certain decision to a human, even when prediction is delegated to a machine, is ultimately a normative choice. When decisions – including legal decisions by judicial or administrative bodies – involve selecting one course of action among alternatives, based on the way in which the selected alternative promotes or demotes the values (individual rights, public interests) at stake, the process often entails evaluating the comparative importance of these values. To date, no machine has the ability to make such an assessment, but this does not mean that such choices can never be delegated to a machine.
First, a hard-coded automated rule may specify that given a prediction, a certain decision is to be taken by the system (e.g., that a loan application has to be rejected if the applicant is predicted to default with a likelihood that is above a given threshold); similarly, an online filtering system may reject a message given the likelihood that it is unlawful or inappropriate.Footnote 29 This ex-ante choice (i.e., the decision rule specifying what the systems should do, given its prediction), of course, is where the normative work is being done, and hence we would expect it to be rendered by humans.
In case no hard-coded rules are available for linking predictions to choices, but the goals to be achieved , as well as their relative importance, are clear (again, in the sense that humans have made a prior decision regarding these goals), the system may also be entrusted with learning the best way to achieve such goals under the predicted circumstances, and implement it. For instance, in the case of online advertising, a system may learn what kind of messages are most likely to trigger a higher response by certain kinds of users (the maximisation of users’ clicks or purchases being the only goal being pursued) and act accordingly. As this example shows, a problem arises from the fact that, in order to delegate a choice to a machine, the multiple values that are at stake (profit of the supplier, interests of the consumers, overall fairness etc.) are substituted by a single proxy (e.g., number of clicks or purchases) that is blindly pursued.
When even the goals are not clear, the system may still be delegated the task of suggesting or even taking actions, after it has acquired the ability to predict how a human would have acted under the given circumstances: the action to be taken is simply the action that the system predicts that a human would have taken, after training itself on a relevant data set that captures the inputs humans receive and their subsequent decisions. For instance, a system may learn – on the basis of human-made translations, documents, or paintings – how to translate a text, write a document, or draw a painting, by predicting (after adequate training) how humans would translate the text, write the document, or draw the painting. Similarly, a system may forecast or suggest administrative or judicial decisions, after having been trained on data sets of such decisions, by predicting how a human administrator or judge would decide under the given circumstances. This is what is aimed at in the domain of predictive justice: the system should forecast or suggest decisions by predicting what a judge would do under the given circumstances.
A learning process needs to be calibrated on the manner in which a human would make a decision, whenever hard facts, ground truths, or clear consequences which distinguish a correct decision from a faulty decision are hard to come by. Contrast for instance medical and legal decision-making. In medical decision-making, the evolution of the physical conditions of a patient may tell whether a diagnosis was right or wrong, or whether a therapy was effective or not; in the law, matters are more complicated. Whereas we may have facts regarding recidivism or ‘jumping bail’ (which, however, may reflect societal inequities or biases in and of themselves), it is much more difficult to generate a factual method with which to evaluate whether a correct decision has been entered regarding the validity of a contract or a will, or on whether a certain interpretation of a statute is more correct than another. The methodological precondition that requires learning by mimicking human decision makers is obviously a double-edged sword: the AI system will learn to replicate the virtues and successes of humans but also their biases and failures.
On this basis, we may wonder to what extent AI (predicting machines) do and may contribute to state activity. As prediction is key to most if not all decision-making, it appears that a vast domain of possibilities exists. A learning system can provide indications that pertain to different domains that are relevant to the government. For instance, such a system may predict the chances that a person is going to re-commit an offence (i.e., has certain recidivist tendencies) or violate certain obligations, and on this basis, it can suggest measures to be adopted. It can predict where and at what time crimes are most likely to take place, so that appropriate measures can be taken. Or it may predict the occurrence of traffic jams, and possibly suggest how to direct the traffic in such a way that jams are avoided. Or it may predict the possibility of environmental issues, and possible responses to them. It may predict the spread of a disease and the effectiveness of measures to counter it. More generally, it may predict where social issues are going to emerge, and how to mitigate them. The context of the system’s use often determines whether its proposals are interpreted as forecasts, or rather as suggestions. For instance, a system’s ‘prediction’ that a person’s application for bail or parole will be accepted can be viewed by the defendant (and his lawyer) as a prediction of what the judge will do, and by the judge as a suggestion for her decision (assuming that she prefers not to depart from previous practice). The same applies to a system’s prediction that a loan or a social entitlement will be granted. Depending on the context and on the technology used, such predictions can be associated (or not) with a probability score. In any case, such predictions are uncertain, being grounded on the data in the input set provided to the system, and on the statistical correlations between such data.
However, we must not forget that the fact that a machine is able to make predictions at a human and even at a superhuman level does not mean that the machine knows what it is doing. For instance, a system for automated translation does not know the meaning of the text in the input language, nor the meaning of the output in the target language; it has no idea of what the terms in the two languages refer to in the physical or social world. It just blindly applies the correlations – learned from previous translations – between textual expressions in the source and target language. It has indeed been argued that the success of automated translation does not show that machines today understand human language, since it rather consists of ‘bypassing or circumventing the act of understanding language’.Footnote 30 Similarly, a machine predicting appeal decisions – based on the text of the appealed sentence and the arguments by the parties – does not know what the case is about. It is just blindly applying correlations linking textual patterns (and other data) to possible outcomes; it is suggesting legal outcomes by bypassing or circumventing the act of understanding laws and facts.Footnote 31
It is true that the impacts of a choice on the real world may be fed back to, and taken into account by, a learning machine, but only to the extent that such impacts are linked to quantities that the machine can maximise. This may the case for investment decisions, where a quantification of the financial return of the investment may be fed back, or even directly captured by the machine (e.g., in the stock market); the situation is more difficult in most instances of administrative and judicial decision-making, where the multiple goals, values, and interests at stake have to be taken into account. Completely relaying decisions to the ‘blind’ machine assessment may involve a violation of the rule of law (as will be further discussed in Section 8.9, where we will address other concerns the recourse to AI raises).
8.8 Algorithmic Machine Learning as a Regulatory and Policy-Formation Instrument
In this section, we will consider how algorithms can assist governmental agencies in exercising executive functions, focusing first on algorithms as part of the administrative and regulatory apparatus, rather than as a subject for regulation. The state, it should be recalled, acts in three capacities: it is an operator, or an actor (when, for example, it goes to war or uses other forms of direct action); it is an administrative entity (when administering, or implementing, a regulatory scheme, for example, when providing services to citizens and residents); and it also has legislative powers (primary and secondary) to devise a policy and then enact a regulatory regime (which may apply to the state or to the industry). Algorithms can play a part in all three prongs.
First, as a direct actor, or operator, the state may harness AI for its war powers (autonomous or semi-autonomous weapons)Footnote 32 or police powers (when it resorts to AI in the law enforcement context for deploying its forces)Footnote 33 or other operational decisions, including logistics and human resources. In the policing domain, with surveillance sensors expanding to include online cameras, neural network technologies can be used for facial recognition,Footnote 34 and access to law enforcement agencies’ databases may provide real-time predictive policing, for assisting officers in making operational decisions in response or in anticipation of risks. More specifically, predictive policing systems are used to determine the locations and times in which different kinds of criminal activities are more likely to take place, so that a timely preventive action can be undertaken by police forces.
The police power of the state encompasses also the second prong of state power – the administration of a regulatory regime designed to achieve certain regulatory purposes. In that respect, predictive policing is not different from other types of predictive tools, designed to give implementing agencies more efficient capacities. To the extent that algorithmic instructions reach the desired outcome or rigorously reflect the legal criteria underlying a given regulatory scheme,Footnote 35 and so long as the factual input upon which the instructions are then implemented is sound, such algorithms can facilitate the day-to-day bureaucratic machinery, which is faced with the challenge of addressing a large number of decisions pursuant to a regulatory scheme. Among other duties, regulatory agencies perform monitoring routines; publish state-certified information; grant or withdraw permits and licenses; levy fines; assess, collect, and refund fees, taxes, and subsidies; and execute decisions of judicial bodies. Recall that many of these ‘application algorithms’ discussed previously need not necessarily include a machine-learning component, at least to the extent that the language of the legal codes may be translated into computer code and applied in a manner that does not require machine ‘discretion’. Depending on the specificity of the legal criteria undergirding the regulatory regime governing such duties, many such routine decisions are candidates for being coded and translated into algorithms, thereby relieving some of the administrative burden associated with these decisions, as well as assisting in achieving greater consistency in the application of the law to concrete cases. Moving beyond ‘simple’ algorithms, an AI component allows for optimisation of the decision-making process when only some facts are known but not all the facts are easily discernible. In such cases, one (or more) of the basic approaches to machine learning (described in Section 8.5) may be relevant for sifting through a large number of cases and detecting the cases in which the exercise of the regulatory function is most likely appropriate.
For example, when the state agencies are called upon to perform the basic function of ensuring compliance by the industry with rules, procedures, or outcomes, the question of how to allocate compliance resources may be one in which AI may assist and suggest possible resources that may be enlisted to assist. Consider the allocation of financial or other resources to citizens and organisations pursuant to some self-reporting: predicting which cases probably meet the criteria and therefore require fewer checks may promote the overall social good.Footnote 36 The technology may also be used to anticipate who may drop out of school. More generally, it may identify people who, in the near future, may require other forms of governmental assistance, or, for that matter, certain medical treatments. Similarly, AI may be used to assist the grading of public tenders, or other forms of public contracts. In the enforcement context, examples include detecting money laundering by relying on technological approaches such as those used by PayPal, banks, and credit card companies that seek to spot irregular activities based on established spending patterns.Footnote 37 Similarly, governments may use AI to detect welfare fraudsFootnote 38 (and tax frauds more generally). Enforcement may also capture relevant online communications (e.g., organised crimes, or terrorism, but also, in authoritarian states, proscribed opinions).
More fundamentally, algorithms can be harnessed to play a role not only in the implementation of regulatory schemes, technical or discretionary, but also in their evaluation and eventually in formation process of alternative schemes. The development of the predictive algorithms may be useful in assessing not only a particular case, but the more general relationship between regulatory means and ends. It may shed light on what measure is likely to work, and under what conditions. It may also inform the policy makers with respect to the probable cost-benefit analysis of achieving certain policy goals. Such algorithms may be conceptualised as ‘policy algorithms’, since the problem they are designed to solve is the overall risk allocation in a given socio-economic field, or the adequacy (likelihood) of a certain regulatory scheme as applied to achieve its goals, compared to (tested) alternatives. Obviously, such algorithms can also be designed so that they ‘learn’ and adapt, as they analyse policy decisions at the aggregate level, to detect those with greater probabilities of achieving a desired goal (and lower probability for achieving unintended negative consequences).
More specifically, then, to the extent a state agency was able to distil the objectives it seeks to optimise, or to identify key factors underlying a social problem (or which may affect such a problem), the agency may resort to the technology for designing policy, by focusing on what the technology may tell the policymaker regarding the relationship between means and ends.Footnote 39 For example, it may harness machine learning in public health for predicting risks and susceptibility to diseases and illnesses and for predicting which regulatory responses may optimise desired outcomes.Footnote 40 Similarly, machine learning may be used in education, where AI systems can predict educational performance,Footnote 41 including the correlation between such performance and different regulatory approaches. In transportation and urban planning, machine learning may be used to predict traffic, capacity, or urbanisation patterns, and their correlation with different planning policies.Footnote 42 In predicting external events or situations that are relevant to the activities of state agencies, environmental patterns should also be mentioned.Footnote 43 Note that in these cases as well, AI is not concerned with the overall set of values the policy is set to promote, but rather is placed at the level of optimising the means for achieving these goals. Furthermore, we can appreciate that predicting recidivism, crimes, financial frauds, and tax evasion are not only of interest to the law enforcement agency – they are also relevant for the policy formation segments of the state. Similarly, anticipating environmental, sanitary, or financial difficulties; reviewing purchases or other contractual arrangements; predicting the flow of traffic or the consumption of energy are relevant not only for real-time response, but are also valuable in the policy formation process, including for optimising the logistics in civil and military domains.
In conclusion of this section, machine learning holds the potential of going beyond what we currently identify as legally relevant criteria. To the extent the design of the algorithmic ‘production line’ includes access to big data, not classified according to any legally relevant criteria, the algorithm may come up with alternative criteria, which are based on statistical probabilities of certain correlated facts in a given instance. In this sense, the learning algorithm is not merely an ‘application algorithm’, which contends itself with the technical application of a predetermined set of instructions. Rather, a learning algorithm can be understood as a ‘discretionary algorithm’, since it may devise the criteria upon which a state decision may be based. These criteria are those embedded in the predictive model constructed by the learning algorithm of the system, regardless of whether such criteria have a linguistic form (as in system based on inferred rules or decision trees), or whether they are coded at the sub-symbolic level (as in the weighted connections within a neural network). This holds the potential to expand the ability of the state agency (or agencies, to the extent a regulatory regime involves multiple organs). It comes, however, with its own set of legal difficulties.
It is worthwhile to note AI is not a single technology, but rather a vast bundle of diverse methods, approaches, and technologies. Within that bundle, there are learning algorithms that may be designed to generate cognitive responses (rational and emotional) that nudge people – whether they are conscious of the manipulation or not – to behave or react in a certain way. This feature may be combined with algorithms that seek, upon mining big data, to ascertain what achieves a preferred outcome without necessarily following pre-ordained legal criteria.Footnote 44 Nudging algorithms, are relevant as a regulatory measure, precisely because of their ability to nudge people to react, form opinions/emotions, and invest their attention one way (or not invest it in another), and therefore they offer regulators the ability to channel the behaviour of an unspecified public by micro-targeting segments thereof. Their deployment also clearly raises considerable ethical and right-based questions. And we should also realise that automated nudging may be deployed by the regulated industry so as to prompt a certain reaction from the agency (and the decision makers therein).
8.9 The Algorithmic State – Some Concerns
With all their promise, algorithms – application algorithms, discretionary algorithms, and policy-analysis (or policy-formation) algorithms – challenge our understanding of regulation in two dimensions, both rather obvious. The first is that the integration of algorithms into the regulatory process comes with some serious drawbacks. The second is that algorithms are not only (or primarily) integrated into the regulatory process; they emerge as the backbone of the modern, data-driven industries, and as such call for regulation by the (algorithmic) state. As noted previously, they are the subject of regulation, and hence a tension may arise.
On a fundamental level, and in reference to the analysis of the different functions of government, we may observe that AI systems could be deployed to enhance the influence of government over information flows (nodality). AI systems have indeed been used to filter the information that is available to citizens (as happens most often in repressive regimes), to analyse the information generated by citizens (and not necessarily reveal such analysis to the general public), and to provide personalised answers to citizen’s queries, or otherwise target individuals, in a manner that may be manipulative. Furthermore, as has been identified by many, AI may be used by for-profit or not-for-profit entities to further enhance existing socio-political cleavages. By nudging activities within echo-chambers in a manner that alters priorities, perceptions, and attitudes, a degree of social control may be obtained in a manner that is inconsistent with underlying presumptions regarding deliberative discourse and the ongoing formation of values. To the extent the state fails to regulate such deployment of AI by for-profit or not-for-profit organisations, AI can be used to undermine democratic values.
8.10 Algorithms and Legal (Performative) Language
The drawbacks of algorithmic regulation have been noted by many. But before we outline some such key concerns, any serious discussion between jurists and computer scientists on algorithms (or machine learning or AI) reaches the issue of language and rules. Recall that algorithms are a form of prescriptive language, and as such share this feature with law. Yet as philosophers of law teach us, ‘the law’ – itself a rather complex term – is greater than the sum of its rules. The legal universe is also comprised of standards, principles, and values – which by definition are not ‘finite’ and as such evade codification into an algorithm. Moreover, the relationship between the rule (as a general norm) and the application of the rule (to one particular case) is not trivial. It would appear that by definition a rule must include a set of cases greater than one for it to be a rule of general application. Yet as soon as we shift our focus from the rule to the particular case, at least two things happen. The first is that we have to inquire whether other legal norms may be applicable, and since as noted the legal system includes standards and values, with relatively far-reaching application, the answer is almost always yes. This creates a built-in tension, as there are no easily available rules to solve the potential clash between a rule of general application and the more general standard or value. The second, more nuanced issue that arises relates to the very notion of ‘application’, which requires a certain form of judgement which cannot be reduced, in law, to a cut and dry, mechanical syllogism. This is because conceptually, language does not apply itself, and normatively built into the rule is its purpose, which may call, in the particular case, for generating an exception to the rule or otherwise refresh the understading of the rule to address its particular ‘application’ in a manner consistent with the purpose of the rule.
In other words, in law the relationship between the rule and its application is dialectic: the very essence of the rule is that it will be ‘binding’ and apply to the particular cases captured by the language of the rules, yet at the same time part of the DNA of the language of the rules is that the application in the particular case, while fitting a certain analytic structure is also consonant with the underlying purpose and function the rule is there to fulfil. Because in law rules do not self-apply, some form of judgment is inherent. Viewed slightly differently, there is always, again, because of the nature of human language, an ingredient of interpretation regarding the meaning of the words that construct the rule. Such interpretation may be informed by the core (conventional) meaning of a certain phrase, but it may also be informed by the penumbra, where the meaning is more vague. The line between the core and the penumbra is itself open to interpretation. Some even question the clear distinction between the core and the penumbra, suggesting that drawing such a line reflects normative considerations of purpose and aesthetic considerations of fit.
Be it as it may, normatively, we do not want to erase the tension between the rule and the exception because discretion, even when highly restricted, is nonetheless an integral part of what makes law worthy of our moral respect; it connects the operative words to their (otherwise morally appropriate) purpose. At least some leading jurists suggest that law provides a distinct reason for abiding by its prescriptions, and that reason at least at some level ties back to the notion of the moral legitimacy of the rule as part of a legitimate set of rules, and ultimately of a legal system and its processes.
Moreover, central to the notion of law in a liberal democracy is its human nature: it is a product of human agency, its values and goals should reflect care for human agency, and its application should ultimately be at the hands of humans exercising agency. The aforementioned legitimacy therefore is enhanced with the exercise of judgment as a matter of moral agency (and seeing right from wrong) by the person who applies the law. Some jurists suggest that the question of legal validity, scope, and operative meaning of a particular rule as considered for application in a given set of facts cannot be fully separated from the underlying values embedded in the rule (as part of a set of rules and principles, governing a given field of human interaction). If this is indeed the case, discretion is a feature, not a bug. It is not clear that we can fully separate the question of ‘what is the operative meaning of the rule with respect to a particular set of facts’ from the question ‘should we enforce that meaning in the given set of facts’.
In that respect, would we rather have bureaucrats fully automated, without seeing the unique circumstances before them – the human being (not only the case number), applying for the exercise of state power (or its withdrawal) in a particular case? Certainly, there is a risk that relaxing the technical commitment to the conventional meaning of rules will result in biases or favouritisms, as may be the case when human judgment is exercised. But the alternative, namely removing all ambiguity from the system, may result in detaching law from its human nature, by removing agency and by supposing that codes can adequately cover all circumstances, and that human language is capable of capturing ‘the reality’ in a transparent, technical manner. The latter assumption is difficult to support.
On some abstract level, the law is ‘quantic’. Contrary to our everyday understanding, in the marginal cases it evades being reduced to yes-no answers, and we may never know what the rule is until past its application (and then we know what the application has been, not necessarily how the rule will be applied in the next marginal case). The presence of the marginal cases radiates back to the core cases, such that even in some core cases irregular application may ensue, and thus an internal tension always exists between the rule and its application.
Algorithms it would seem, have a different logic: as a general matter, a clear binary answer is what makes an algorithm sound. In cases where such a binary answer is unavailable, it is replaced with an approximation, and then this approximation is reduced to a yes-no complex flow chart.
Even though AI system may be able to learn from past examples and from feedback to randomly select and test new solutions, and to model competing arguments and criteria for choosing between these solutions, it is still difficult to conceive – at least accordingly to the present state of the art – of a dialectic algorithm which adequately captures the internal tension between rule and exception, or the general rule and the particular case, built into law. As noted previously, even the most advanced predictive systems do not have an understanding of language; they can only harness ‘blind thought’ (i.e., in unreflected data manipulation), lacking the capacity to link language to reality, and in particular link legal provisions to the social and human issues that such provisions are meant to regulate. Consequently, delegating the application of the law to an automated system in a manner that eliminates human discretion (and fully removes the human from the loop, including from above the loop) entails, fundamentally, the displacement of a certain question from the legal realm to the technical/bureaucratic realm. This does not mean that certain matters cannot be so displaced, but it does mean that such displacement, to the extent it involves the exercise of state power, generates a call for a process of legal contestation, for reasons related to the rule of law. Hence, the law is reintroduced and the potential for human intervention is brought back.
An interesting, albeit highly speculative development in this domain suggests that we should reconfigure our understanding of general rules by introducing the concept of personalised law.Footnote 45 The idea is there to use AI to predict the relevant features of individual citizens, and to select accordingly the law that applies to them. For instance, if it is possible to distinguish automatically between skilful or incapable drivers, or between vulnerable or knowledgeable, consumers, each individual should be applied the law that fits his or her features, with regard to the achievement of the required level of care (e.g., speed limits), advertising messages, or privacy notices. Similarly, with regard to default rules (e.g., in matters of inheritance,), each one may be subject, by default, to the legal rule that fits his or her predicted preferences.Footnote 46 It remains to be seen not only whether this would indeed be technically feasible, but also whether it may challenge our understanding of the relationship between general norms and their application, including the relationship between rules and standards on the one hand, and rules and particular exceptions on the other.
8.11 Rule of Law
Moving to a less abstract level, resorting to algorithms as a regulatory tool may generate conflicts with the demands of the rule of law, to the extent the recourse to algorithms amounts to delegation of legal authority either to the state-run algorithm, or to private companies that own the data or the algorithm (or both). Clearly, to the extent that private entities play a key role in algorithmic regulation (of others), the issue of delegation of state power is a serious concern.Footnote 47 Considerable attention has been devoted to the public-private interface, sometimes referred as a ‘partnership’, although such partnership already assumes a model of co-regulation, which then raises concerns of self-dealing or the potential capture of either the policy formation or the enforcement processes, or both. But even if the private entities only play a supportive role (or play no role at all), the rule-of-law problem remains.
As noted previously, under the analysis of legal language, the rule of law, as a concept, is not the rule of machines. This is not a mere matter of legal technicality: the idea of the rule of law is premised on the conscious and intentional articulation and deployment of legal categories and concepts, reflecting certain values, to address specific and more general distributive and corrective decisions. Such premise holds at the level of norm-setting (thereby is relevant to policy-analysis algorithms) but also at the level of implementation (and is thereby relevant to implementation and discretionary algorithms). Contrary to a simplified meaning, according to which the rule of law is posited as the opposite of the rule of men, the rule of law is not a rule detached from humans. Rather, it is a rule formed through human interaction, governing human interaction, for the benefit of humans. The rule of law therefore is a mechanism to counter the rule of whim, desire, arbitrariness, or corrupted self-interest, which may follow from constructing the state as if it can do no wrong, and the rulers as if they are entitled to pursue whatever they deem through whatever means they chose.Footnote 48 It is not a mechanism designed to replace moral agency with automated decision-making, even if such automated decision-making may reduce negative outcomes.
Since the rule of law is an expression of autonomy and agency, and since agency is a rather complex term which includes the exercise of empathy, it appears that the rule of law demands a rule laid down and then implemented by moral agents, at least so long as an algorithmic rule (and application) will result in some errors (defined as rules or applications which fail to optimise the fit between legitimate regulatory purposes and the means used, or fail to be consistent with underlying values and the analytic logic of technical legal concepts). Granted that algorithms may reduce such errors, compared to human-made rules and applications, the errors caused by machines are more difficult to justify for those who suffer from their consequence than errors caused as a product of processes through which deliberative moral agency is exercised. A human error can be accepted, or tolerated, because collective decision-making – and legal rules and their implementations are examples of decisions made by some and then applied to others – is premised on a certain degree of solidarity, which stems from a shared notion of what it feels like to suffer from the harm errors cause. Such solidarity, and the premise of empathy, are not present when decisions are made by machines, even if machines may reach fewer decisions that cause such errors. In other words, the concept of the rule of law requires a human in or over the loop, even if we reach a position that AI is fully developed to pass a legal Turing Test (i.e., be indistinguishable from a competent human decision maker) for its ability to integrate purposes and the means achieve consistency between underlying values, on the one hand, and technical legal concepts, on the other. To date, we should be reminded, we are still some ways away from that demanding benchmark. In the law as in other domains, at least in the foreseeable future, it is most likely (and normatively appropriate) that complex tasks, including those requiring creativity and insight, are approached through a hybrid or symbiotic approach that combines the capacities of humans and machines.Footnote 49
Moreover, the issues of legal competence (who gets to call the shots?), of process (how is the decision reached?), and of discretion (what are the relevant considerations, and their respective weight?) are central because they reflect social experience regarding the use of power (and law is a form of power). A rather intricate system of checks and controls is usually in place to ensure the four heads of legal competence (over the matter, the person exercising power, the territory, and the time frame) are checked and often distributed to different entities. What would it mean for algorithms to reflect the need to distribute power when rules are promulgated and applied? Algorithms are designed to integrate and optimise. Should we rather design algorithms so as to check on other algorithms? Similarly, the process that produces legal norms and particular legal decisions is itself regulated, with principles of due process in mind. How would a due-process algorithm be designed?
And, lastly, the modern public law has developed rather extensive structures for managing executive discretion (at the policy-formation, norm-giving and then implementing stages), based upon a paradigm which stipulates that (a) certain considerations are ‘irrelevant’ to the statutory purpose or even ‘illegitimate’ to any purpose, and (b) the relevant or legitimate considerations are to be given a certain weight. The latter is premised on the language of balancing and proportionality, central to which is the structured duty for justifying the relationship between means to achieve the chosen goal, the lack of a less restrictive mean, and the overall assessment that the benefit (to the protection of rights and public interests) expected to be gained by the application of measure is not clearly outweighed by the harm the measure will cause (to protected rights and public interests). This language of proportionality, notwithstanding its rational structure, is rather difficult to code, given the absence of reliable data, unclear causal lines, and the lack of agreed-upon numbers with which to determine when something is clearly outweighed by something else.
This is not to say that algorithms cannot assist in determining where data is missing (or otherwise diluted or corrupt), whether less restrictive means may be available, and what may be the overall cost-benefit analysis. Banning access to proportionality-related algorithms is not warranted, it seems, by the principles of the rule of law, nor would it be a sound policy decision. But fully relying on such algorithms as if their scientific aura places them as a superior tool of governance is neither warranted nor reconcilable with the underlying premise of proportionality, namely that the judgement call will be made by a moral agent capable of empathy.
To sum up this point, a robust delegation of authority is, to date, compatible with the principles of the rule of law only in the most technical applications of clearly defined norms, where the matter under consideration is of relatively minor importance, the data in the particular case is relatively straightforward and verifiable, and an appeal processes (to other machines and ultimately to humans) is available. In such cases, machine learning (and AI more generally) may be relevant in the periphery, as a tool for identifying regimes where decisions are processed by the administration as technical decisions, and therefore as candidates for ‘simple’ algorithmic processing. Learning, in the sense that the algorithm will devise the predictive model to be applied, will be relevant to the technical, binary decisions discussed in this paragraph, mostly with regard to the assessment of relevant facts (e.g., recognising images and people in the context of traffic fines, identifying potential frauds or risks of violation in the tax of financial domain).
8.12 Responsive Law
As machines cannot be expected to understand the values and interests at stake in administrative and judicial decisions, we can conclude that, left alone, they would not be able to make improvements over the law, but just reproduce the existing practice, leading to the ‘petrification’ about which Roscoe Pound complained, as we observed previously, and about which modern scholars have expressed concerns.Footnote 50 Some aspects of this critique attracted possible rebuttals, suggesting that the force of the concern may depend on the manner in which the AI system is designed, and the manner in which it is used.Footnote 51 Researchers in AI and law have suggested that there may be computational models of legal reasoning going beyond deduction that involve the generation of multiple defeasible arguments,Footnote 52 possibly concerning alternative interpretations, on the basis of cases and analogies.Footnote 53 The advent of machine learning may advance these, or similar approaches by overcoming the technical difficulty of formalising such models, but at the same time, the opacity of machine learning systems proves counterproductive for generating meaningful debate regarding alternative norms.
A possible example on point may be what has been called predictive justice (but the same idea can also be applied both to the judiciary and to administration). The key idea is that systems can be trained on previous judicial or administrative decisions (on the relation between the features of such cases and the corresponding decisions), in such a way that such systems predict what a new decision may be, on the basis of the features of the case to be decided. The results so far obtained have limited significance, as accuracy is low. Some systems base their predictions on extra-legal features (e.g., identity of the parties, lawyers, and judges),Footnote 54 others on the text of case documents. Some of the experiments made no real prediction of the outcome of future cases, but rather the decision of an already decided case is predicted based on sections of the opinion on that case.Footnote 55 Moreover, it can be argued that the task of judicial or administrative decision makers does not consist of predicting what they would do, nor what their colleagues would do (though this may be relevant for the sake of coherence), but in providing an appropriate decision based on facts and laws, supported by an appropriate justification.Footnote 56 However, looking into the future, we may consider the possibility that outcomes of decisions may be reliably forecasted and we may wonder how this would affect the behaviour of the parties, officers, and judges. We may wonder whether this would reduce litigation and induce more conformism in the behaviour of officers and judges, so contributing to legal certainty, but also favouring the ‘petrification ‘of law.
8.13 Human Rights
Beyond rule of law and responsive law concerns, recourse to algorithmic regulation may infringe protected rights, primarily human dignity, due process, privacy, and equality. Human dignity can be infringed upon to the extent a person is reduced to being a data object rather than a fully embodied moral agent, deserving meaningful choice, reasoning, and a decision by another moral agent. We will expand on a variant of this argument below. Due process can be infringed upon to the extent the decision cannot be meaningfully contested as either the data or the explanation behind the decision are opaque.Footnote 57 Privacy can be infringed upon to the extent the algorithm relied on data mined without full and free consent (including consent for the application of the particular data for the purpose it was used) or to the extent the algorithm was used in a manner that inhibited decisional autonomy by nudging a person without full disclosure.Footnote 58 Finally, equality can be infringed upon to the extent the algorithm relies on what turns out to be discriminatory factors, reflects existing discriminatory practices in society, or generates discriminatory impact. As noted, the proportionality analysis, which is designed to check whether the infringement on individual rights may nonetheless be justified, with regard to other rights or social values, is difficult to run, in part because the key aspects of a proportionality assessment – the availability of alternative means, the overall benefit generated by recourse to algorithmic machine learning, and the extent to which the benefit outweighs the harm – are neither easy to concretise nor reasonably assess.
More specifically, the very increase in predictive capacity provided by machines can contribute to fixing and solidifying or even increasing inequalities and hardship embedded in social relations, rather than enabling solutions designed to overcome such inequalities. This is likely to happen when an unfavourable prediction concerning an individual – the prediction that the person is likely to have a health problem, to commit crime, to have inferior performance in education, and so forth – leads to a further disadvantage for the concerned individuals (increased insurance costs, heavier sentences, exclusion from education), rather than to a remedial action to mitigate the social causes of the predicted unfavourable outcome. For this to be avoided, prediction has to be complemented with the individuation of socially influenceable causes and with the creative identification of ways to address them or spread risks.
It should be noted that supporters of the use of predictive systems argue that the baseline for assessing the performance of automated predictors should be human performance rather than perfection: biased computer systems still contribute to fairness when their biases are inferior to those of human decision makers. They argue that automated decision-making can be controlled and adjusted much more accurately than human decision-making: automated prediction opens the way not only for more accuracy but also for more fairness,Footnote 59 since such systems can be ‘calibrated’ so that their functioning optimises, or at least recognises, the idea of fairness that is desired by the community.Footnote 60
A more general issue pertains to the fact that the possibility to use AI to make accurate predictions on social dynamics pertaining to groups and or individuals, based on vast sets of data sets, provides a powerful incentive toward the massive collection of personal data. This contributes to lead toward what has been called the ‘surveillance state’, or the ‘information state’, namely a societal arrangement in which ‘the government uses surveillance, data collection, collation, and analysis to identify problems, to head off potential threats, to govern populations, and to deliver valuable social services’.Footnote 61 The availability of vast data set presents risks in itself, as it opens the possibility that such data are abused for purposes pertaining to political control and discrimination.
In the context of the availability of massive amounts of data, AI enables new kinds of algorithmic mediated differentiations between individuals, which need to be strictly scrutinised. While in the pre-AI era differential treatments could be only based on the information extracted through individual interactions (interviews, interrogation, observation) and human assessments, or on few data points whose meaning was predetermined, in the AI era differential treatments can be based on vast amounts of data enabling probabilistic predictions, which may trigger algorithmically predetermined responses. In many cases, such differential treatment can be beneficial for the concerned individuals (consider for instance how patients may benefit from personalised health care, or how individuals in situations of social hardship can profit from the early detection of their issues and the provision of adequate help). However, such a differential treatment may on the contrary exacerbate the difficulties and inequalities that it detects. The impacts of such practices can go beyond the individuals concerned, and affect important social institutions, in the economical as well as in the political sphere. An example on point is the recourse to AI for generating grades based on past performance for students in the UK, given the inability to examine students on the relevant materials they should have learned during the COVID-19 crisis. Students reacted negatively to the decision, in part because the very idea of an exam is based on individual performance at the exam itself and substituting this data point by going to past practices, reproduces past group-based inequalities.Footnote 62
8.14 Opaqueness and Explainability (Due Process and Fairness)
A key issue concerning the use of machine learning in the public sector also concerns the fact that some of the most effective technologies for learning (in a particular neural network) tend to be opaque – that is, it is very difficult to explain, according to human-understandable reasons, their predictions in individual cases (e.g., why the machine says that an application should be rejected or that a person is likely to escape from parole). So not only can such machines fail to provide adequate justifications to the individuals involved, but their opacity may also be an obstacle to the identification of their failures and the implementation of improvements.Footnote 63
An example for this conflict is the discussion concerning ‘COMPAS’ (Correctional Offender Management Profiling for Alternative Sanctions) – a software used by several US courts, in which an algorithm is used to assess how and whether a defendant is likely to become a recidivist. Critics have pointed both to the inaccuracy of the system (claiming that in a large proportion of cases, the predictions that released individuals would or would not engage in criminal activities were proved to be mistaken) and on its unfairness.Footnote 64 On the latter point, it was observed that the proportion of black people mistakenly predicted to reoffend (relative to all black people) was much higher than the corresponding proportion of white people. Thus it was shown that black people have a higher chance of being mistakenly predicted to reoffend and be subject to the harsh consequences of this prediction. Consequently, detractors of the system accused it of being racially biased. Supporters of the system replied by pointing out that the accuracy of the system had to be matched against the accuracy of human judgments, which was apparently inferior. On the point of fairness, they responded that the system was fair, from their perspective: it treated equally blacks and whites in the sense that its indication that a particular individual would reoffend was equally related, for both blacks and whites, to the probability that the person would in reality reoffend: the proportion of black people which were correctly predicted to reoffend (relative to all black people who were predicted, correctly or incorrectly to reoffend) were similar to the same proportions for white people. The same was the case with regard to those who were predicted not to reoffend.Footnote 65
The use of COMPAS was the object of a judicial decision, in the Loomis v. Wisconsin case, where it was claimed that the opacity of the system involved a violation of due process, and that the system might have been racially biased. The Court, however, concluded that the use of the algorithm did not violate due process, since it was up to the judge, as part of his or her judicial discretion, to determine what use to make of the recidivism assessment, and what weight to accord to other data. The Court also stated that the judges should be informed of the doubts being raised about the racial fairness of the system.
As noted, COMPAS presented the problem of the opacity of the algorithm, since defendants faced considerable hurdles in understanding the basis upon which the assessment in their case has been reached. This issue is compounded by an additional problem – the IP rights of the private companies that developed the system. Invoking IP rights proved to be an obstacle in obtaining the code, which may be necessary for providing a meaningful opportunity for challenging the outcomes of the system.Footnote 66
Further issues concerning automated decisions in the justice domain pertain not so much to the accuracy and fairness of automated predictions, but rather to the use of such predictions. It has been argued that predictions of recidivism should be integrated by causal analyses of modifiable causes of recidivism. This would open the space for interventions meant to mitigate the risk of recidivism, rather than using the predictions only for aggravating the condition of the concerned individuals.Footnote 67
The debate on automated decision-making within the justice system is part of a broader discussion of the multiple criteria for measuring the fairness of a predictive system relative to the equal treatment of individuals and groups,Footnote 68 a debate which adds a level of analytical clarity to the discussion on fairness and affirmative action, not only in connection with algorithms.Footnote 69
Some initiatives to mitigate the issues related to automated decision models, by both public and private actors, were introduced in recent years. The European General Data Protection Regulations (GDPR)Footnote 70 – the goal of which is to ‘supervise’ the movement of data in the European Union, and mostly to protect the ‘fundamental rights and freedoms of natural persons and in particular their right to the protection of personal data – addresses automated decision-making at Article 22. It establishes the right ‘not to be subject to a decision based solely on automated processing, including profiling, which produces legal effects concerning him or her or similarly significantly affects him or her’. However, automated decision-making is permissible when explicitly consented by the data subject, when needed for entering into or performing a contract, or when ‘authorised by Union or Member State law to which the controller is subject’. The laws that authorise automated decision-making must lay down ‘suitable measures to safeguard the data subject’s rights and freedoms and legitimate interests’. Thus a legality requirement for the use of automated decision-making by state authorities is established.
Another idea is Explainable Artificial Intelligence (xAI): this concept seeks to alleviate the ‘black box’ problem,Footnote 71 at least to an extent, by providing some human-understandable meaning to the process of decision-making and data analysis. Thus it may contribute to reducing the due-process problem. This approach may also address problems of machine-learning biasFootnote 72, as in the COMPAS example noted previously, and answer questions of fairness in an algorithm’s decision-making process. This approach is not free from difficulties, in particular relating to the question of the scope of the desired explanations. Do we wish, for example, for the explanation to be an ‘everyday’ explanation, that would lack in scientific, professional details, but provide an accessible explanation any individual would be able to understand? Or would we rather have a ‘scientific’ explanation, only meaningful to certain proficient and sufficiently educated individuals, though by far more reflective of the process? Related, is the question of process: do we need to know the exact process that led to the decision, or are we satisfied that an ex-post-facto explanation is available, namely that a backward-looking analysis can find a match between the decision reached and demonstrable salient factors, that can be understood by the data subject as relevant? There is also a question whether an explanation should be provided regarding the entire model or only to the specific decision or prediction. Furthermore, some explanations have the potential of being misleading, manipulative, or incoherent.Footnote 73
Others also argue that human decision-making itself suffers from a lack of explainability, in the sense that one never fully knows what a human truly thought about while making a decision. Yet explanation and reason-giving are required especially because humans tend to suffer from bias and errors; the prior knowledge that they are required to provide an explanation for their decision offers a path for accountability, as it generates awareness and focuses the attention of the decision-maker, at the very least, on the need to reach a decision that fits criteria that can be explained, given the facts of the case.Footnote 74 Another argument stipulates that the main importance of the duty to provide reasoning is not having a ‘casual’ or a ‘scientific’ explanation – but having a legal explanation – claiming that an algorithm should be able to explain the rationale behind its decision and fulfil the legal requirements for an explanation, due process and other obligations set by administrative law or any other law.Footnote 75 Therefore, a fully detailed explanation does not necessarily provide a legal explanation. Attention should be paid also to the specific justification requirements for administrative and judicial decision-making (i.e., in particular, that a decision is grounded in legally acceptable rationales, based on legal sources).
Lastly, some argue that too much transparency may be bad. From an explainability perspective, more transparency does not mean better explainability. Informing an individual about every minor calculation does the exact opposite of what the idea of explainable AI is seeking to achieve: it saturates and ends up obfuscating. Moreover, too much transparency could reveal private data collected by the machine-learning software, hence infringing the right to privacy for many individuals and in this sense doing more harm than good. Some also claim that increased transparency will reduce private incentives and delay progress by forcing the exposure of certain key elements of a developer’s intellectual property.Footnote 76 These problems are important to keep in mind when thinking about xAI.
8.15 The Specific Problems of ‘Zero Price’ and ‘The Score’ (or ‘The Profile’)
Of particular concern is the lack of valuation of data for citizens/users/consumers, given that the collection of data is not attached to any tangible price. In most services online, whether offered by the industry or the state, there is no option to obtain the service while paying for the data not to be collected and analysed or rather to obtain the service without those aspects of it (e.g., personalised recommendations) that require our personal data.Footnote 77 The sense that we are getting an optimised service by giving up our private data and by subjecting ourselves to personalised information that would be fed back to us seems like a good deal in part because we have no way of fully understanding the value, in monetary terms, of the data we provide the system, and the value, in monetary terms, of the nudging that may be associated with the manner in which the personalised information is presented to us. We may be aware, of course, that the data lumps us with "people like us", thereby creating a filter buble, but it is almost impossible to figure out how much are would we be willing to pay in order to ascertain better control over this batching process. In other words, our consent is given in a highly suboptimal context: we lack important anchors for making an informed decision. Moreover, some may even argue that since we are already immersed in a saturated environment premised on surveillance capitalism, it is not easy to ensure that we have not been nudged to accept the loss of privacy (in both senses, the collection of data and the feedback of analysed data) as inevitable.
Furthermore, as noted, the logic of the algorithmic eco-system is that providing the data enhances the service. Each data point provided by users/citizens assists both the data subject and other data subjects. In our daily lives, we seem to acquiesce by participating in the AI ecosystem, which is premised on constant surveillance, data collection, and data analysis, which is then fed back to us in correlation with the filter bubble to which we belong, thereby further shaping, nudging, and sculpting our outlook, attitude, mood, and preferences.
But with it comes a hidden price related to the accumulation of data and, more importantly, to the construction of a profile (often including a ‘score’). The matter of the ‘score’ is pernicious. Attaching a certain number to a person, indicating the extent to which that person is predicted to have a certain desired or undesired feature, attitude, or capacity, brings to the fore a clash between the underlying feature of a liberal democracy – premised on the unrestricted authorship of any individual to write her or his own life story, express, experience, and expand their agency by interacting with others in a meaningful manner – with the bureaucratic logic of the regulatory state, even when this logic aims at achieving certain liberal goals (particularly the promotion of collective goods and the protection of human rights, including, ironically, the optimisation of human dignity). As soon as such goal-driven attitude is translated into a ‘score’, broadly understood (a classification as a good or bad customer or contractor, a quantification of a probability or propensity, such as the likelihood of recidivism, or a grade, as is the assessment of the merit of citizens or officers), and as soon such a score is attached to any move within the social matrix or to any individual interacting with another or with a state agency, then a dignitary component is lost. Yet without that score (or classification, or grade), we may be worse off, in the sense that the value brought about by the AI revolution may be sub-optimal, or lost altogether. Given this tension, greater attention needs to be given not only to the processes through which these ‘scores’ (or classifications, or grades, or profiles) are generated, including the power to understand and contest, and not only to the spheres and contexts in which such scores may be used, but also to the social meaning of the score, so that it is clear that we are not governed by a profile, but are seeking ways to constantly write, change, and challenge it.
An extreme example of the usage of ‘score’ would be the Chinese Social Credit System (SCS).Footnote 78 This system, used by the Chinese government, creates an extremely extensive database of personal data for every citizen, regarding most aspects of one’s life. This data is then used to create a social credit score, which rates an individual’s ‘trustworthiness’ and is then used by both authorities and business entities for their benefit.Footnote 79 A lower social credit score may lead to legal, economic, and reputational sanctions, while a higher social credit score would allegedly provide an individual with more opportunities and a better life.Footnote 80 This almost dystopian reality may seem to be a distant problem, relevant only to non-democratic societies such as China, but many believe that these ideas and especially technologies are not very unlikely to ‘leak’ into democratic Western societies as well.Footnote 81 The credit score systems used in most Western democracies for assessing the reliability of prospective borrowers, although less invasive and thorough, may not be as different from the SCS as one might think: In both systems, credit score is an index for an individual’s reputation or trustworthiness. Also, both credit systems have many similar implications on an individual’s life for good or for bad.Footnote 82
8.16 The Data (and AI Derivatives)
On a more basic level, to date, it is not clear that the data sets used for training and calibrating the algorithms are sufficiently sound, in the sense that the data is not corrupt by either being inaccurate or reflecting past or pre-existing wrongs which, normatively, should be discounted and not reinforced.Footnote 83 For example, as noted previously, criticisms were raised against the extent to which predictive systems might reproduce and expand social bias. Various critics observed that systems trained on human decisions affected by prejudice (e.g., officers treating with harshness the member of certain groups), or on data sets that reflected different attitudes relative to different groups (e.g., data sets of past convictions, given different level of control over subpopulations), or on variables that disregarded the achievements of certain groups (e.g., results obtained in less selective educational environments) could lead to replicate iniquities and prejudice.
The state of the data, therefore, casts serious doubts regarding the reasonableness of relying on the assumption that the algorithm is capable of achieving the preferred result, taking into consideration broader concerns of overall dignity and equal, meaningful membership in a society of moral agents. It then becomes a policy question of comparing the propensity of the algorithm to get it wrong because of corrupt data, to the propensity of humans to get it wrong on account of other errors, including bias, as well as our ability to generate social change so as to recognise past wrongs and work towards their remedy, rather than reification.
Data-related issues do not end here. The data may be problematic if it is not sufficiently reflective (or representative). This may be a product of a data market in which the data-collecting pipelines (and sensors) are owned or otherwise controlled by entities that erect barriers for economic reasons. The logic of surveillance capitalism tends to lead to the amalgamation of collection lines up to a point, precisely because the value of the data is related to it being reflective and therefore useful. But when a data-giant emerges – an entity the data it owns is sufficiently ‘big’ to allow for refined mining and analysis – the incentive to further collaborate and share data decreases. To the extent that data giants (or ‘super-users’) already have sufficient control over a certain data market, they may have an incentive to freeze competition out. Such access barriers may hamper optimised regulation.Footnote 84
The dependence on data raises further concerns: data has to be continuously updated (for the algorithms to keep ‘learning’). While with respect to some algorithms, the marginal utility of more data may be negligible (and in that sense, the algorithm has already ‘learned’ enough), the dynamic changes in technology, and more importantly, the changes in society as it interacts with technological developments and with new applications – many of which are designed to ‘nudge’ or otherwise affect people and thus generate further change – suggest that the demand for data (and updated AI based on that data) is un likely to diminish, at least in some contexts. This leads to accelerating pressures towards surveillance capitalism and the surveillance state. It also raises data-security concerns: data stored (for the purposes of ‘learning’) attracts hackers, and the risk for data breaches is ever-present. The state then has to decide on a data collection and retention policy: would it be agency specific? Or may agencies share data? The answer is far from easy. On the one hand, generating one database from which all state agencies draw data (and use these data for algorithmic purposes) is more efficient. It is easier to protect and to ensure all access to the data is logged and monitored. It also saves contradictions among different state agencies and, one may assume, reduces the rate of erroneous data, as it increases the chances of wrong data being corrected by citizens or by a state agency. To the extent that the data are indeed ‘cleaner’, the data analysis will be less prone to error. On the other hand, consolidating all data in one place (or allowing access to many or all agencies to data collected and stored by co-agencies) increases the allure for hackers, as the prize for breach is greater. Moreover, the consolidation of data raises separation-of-powers concerns. Access to data is power, which may be abused. The algorithmic state can be captured by special interests and/or illiberal forces, which may use algorithms for retaining control. Algorithms may assist such forces in governance as they may be used to manage public perception and nudge groups in an illiberal fashion. In other words, algorithms make social control easier. They may also be used to provide preferential treatment to some or discriminate against others. And they may infringe fundamental rights. Consequently, concentrating data in one central pot increases the risk of capture. Reasons underlying separation of powers – between the three branches, between state and federal powers, and within the various executive agencies – call for ‘data federalism’ whereby checking mechanisms are applied prior to data-sharing within various state agencies. Such sharing requires justification and should allow for real-time monitoring and ex-post review. The price is clear: access to data will be more cumbersome, and monitoring costs will increase. More importantly, the technical protocols will have to support effective review, so as to increase the likelihood of detecting, at least ex post, illegitimate use. To the best of our knowledge, the regulatory incentives are such that to date this field is under-developed.
8.17 Predicting Predictions
Rule of law concerns, fundamental rights infringements, and data-regulation questions are not the only issues facing the state. At this point in time, as the ‘predictive’ agencies are beginning to flex their algorithmic muscles, another use for predictive machine learning emerges: one that predicts how agencies make decisions. This approach can be deployed by the industry – as will be discussed later – but also by the state agencies themselves. In order to better manage their regulatory resources, and ease regulatory burden, agencies are seeking ways to separate the wheat from the chaff by knowing which regulatory problems deserve regulatory attention, versus other tasks that can be managed as a matter of routine. ‘97% of cases like that are decided in this or that way’ is a message that is attached now to certain agency procedures, a product of an algorithm that follows state practice and designed to assist bureaucrats in deciding on which issues or decisions to focus, and which can be summarily decided one way or another. This approach is premised on the importance of having a human in the loop, or over the loop, so that decisions are not fully made by machines, but algorithms may nonetheless reflect useful information to the decision makers.
Such predictive algorithms, often designed and run by private entities, raise not only the familiar ‘private-public-interface’ conundrum, as the agencies partner with the industry, but also pose an interesting problem of path dependency: to the extent that the algorithm captures the bureaucratic practice as it is currently administered, and to the extent the predictive information it provides is indeed followed by state officials, the normative power of the actual is solidified, not necessarily as a product of thorough consideration, and in any event, in a manner that affects the path of future developments. As noted previously with regard to predictive justice (the use of algorithms to predict judicial decisions based on past cases), it remains to be seen how such algorithms will influence the expectations of those interacting with officers, as well as the behaviour of officers themselves. Algorithms developed by private entities and used by governments can create an accountability gap regarding the government’s lack of ability to understand or explain the decision that has been made.Footnote 85
As noted previously, the second main concern with the rise of the algorithmic society is that algorithms are (also, if not mainly) used by the regulated industry. Therefore, their use must also be regulated by checking the degree to which it conflicts with the regulatory regime (including statutory or constitutional rights). In that respect, the algorithmic state is facing the challenge to regulate the algorithmic industry, and determine the appropriate way to go about this task, given regulatory challenges related to informational asymmetries, intellectual property rights of the regulated industry, and privacy rights of its customers.
8.18 Regulating the Industry, Regulating the Executive – Regulating Algorithms with Algorithms?
The challenge of regulating the algorithmic market by the algorithmic state is technological, administrative, and legal. Technological, in the sense that the development of auditing tools for algorithms, or of statistical equivalents thereof, becomes an integral part of the evidence-gather process upon which any auditing regulatory scheme is premised. Recall that the state currently develops algorithms to audit the non-algorithmic activities of industries – for example, it develops auditing tools to monitor health-related records, or pollution-related records, or, for that matter, any record that is relevant for its auditing capacity. It now faces a challenge to develop auditing tools (algorithmic or non-algorithmic) in order to audit algorithmically developed records. The challenge is to have the technological tools to uncover illegal algorithms, namely algorithms that deploy processes or criteria that violate the law or algorithms that are used to pursue outcomes that violate the law. Technologically, this is a complex task, but it may be feasible. Put differently, if algorithms are the problem, they may also be the solution, provided the relevant ecosystem is developed and nurtured. It is also administratively challenging, because it requires qualified personnel, person-hours, and other resources, as well as the awareness and institutional incentives to follow through. Legally, it is challenging both institutionally and normatively. Institutionally, it may be the case that some procedures may need to be tweaked or modified in order to provide judicial or quasi-judicial bodies with the necessary procedural infra-structure with which inquiries into the misuses of algorithms can be conducted. This is not to suggest that a wholesale revolution is necessary, but neither is it to say that the current procedural tools are necessarily optimal. Normatively, it may be the case that some new rules may need to be introduced, in order to align the modalities of regulation with the challenges of regulating the algorithmic industry.Footnote 86
More specifically, the risks – some of which identified in this chapter – need to be defined in a manner precise enough to enable the regulators to design appropriate regulatory means. This may be taxing, given the polycentric nature of the problem, as various goals – sometimes conflicting – may be at play. For example, as has been stated, data-driven AI markets tend to concentrate, and hence generate competition-related harms, but also democracy-related risks. Such concentration, and the potential to ‘nudge’ people within certain echo-chambers by deploying AI-driven manipulations, are certainly a challenge, to the extent we care about the integrity of the democratic process. Addressing each concern – the anti-trust challenge and the social-control worry – may point to different regulatory approaches.
Turning our attention to the available modalities, regulating information sharing seems to be important, in order to cut down disruptive information barriers by defining the relevant informational communities that should have access to certain information regarding regulated algorithms (defined as including the data pipelines that feed them and the output they produce). Some information should be shared with the state agency, while others with the customers/users. Similarly, licensing regimes may be relevant, to the extent that some algorithms require meeting some defined standards (such as privacy or accountability by design). This modality may apply also to the state regulating its own licensing agencies. Furthermore, the structure of civil and criminal liability may have to be refined, in order to match the responsibility of the relevant agents, as well as their incentives to comply. Criminal liability specifically might pose a serious problem with the further development of artificial intelligence and might require both the lawmakers and the court to find new solutions that will fit the technological changes.Footnote 87 Tax and subsidy modalities also come to mind, as the state may resort to taxing elements of the algorithmic eco-system (e.g., taxing opacity or providing subsidies for greater explainabilityFootnote 88). In that respect, it would appear that an algorithm that tracks other algorithms in order to detect the saliency of certain criteria may be useful. And, finally, insurance may be a relevant regulatory factor, both as a tool to align incentives of the industry, and because insurance itself is algorithmic (in the sense that the insurance industry itself relies on machine learning to predict individualised risks and determine corresponding insurance premiums, which may affect risk-sharing).
In short, as the regulator and an actor itself, the state may be pulled by the algorithmic logic state in different directions. As an executive, it may seek to flex its algorithmic muscles so as to optimise its executive function. As a regulator, it may seek to harness algorithms in order to tame their far-reaching and perhaps unintended consequences. This requires policy formation processes that are attuned to the different pulls, as well as to the different modalities that can be put to bear in order to align the incentives.
8.19 Regulation and the Market – The Background of Civil Liability
Before concluding, it is important to revisit regulation and policy making by situating these concepts in a larger context. According to some voices, technology at large and algorithms in particular are better off being ‘deregulated’. We want to resist these calls not only for normative reasons (namely, that regulation is a good thing) but mainly because the term ‘de-regulation’ is misleading. There is always at least one regulatory modality which covers any field of human activity. At the very least, any human interaction raises questions of civil liability. The contours of such liability are a form of regulation, from the perspective of the state. If state agencies are still debating how to proceed with a specialised regulatory regime (including modalities other than civil liability), the residual nature of property, tort, contract, and unjust enrichment is always present.
Take two examples. To the extent the state does not regulate the production, distribution, and deployment of malicious software (which detects and exploits vulnerabilities algorithmically), at the end of the day a civil lawsuit may generate the boundaries of liability. This is exemplified by the civil suit brought by Facebook against NSO, for using the Facebook platform in order to plant malicious software (worms) which allow the attackers the ability to access information on the attacked device. This, of course, is a subject matter upon which a certain regulatory agency should have a say. But to the extent it does not, regulation is still present – in the form of civil liability. Likewise, a civil lawsuit opposed the pharmaceutical company Teva against Abbot Israel (the importer and distributor of Similac, a baby-food formula) and Agam Leaders Tech, a marketing firm. The suit alleges that the defendants engaged in a ‘mendacious and covert slur campaign’ by using fake profiles to distribute false information about Teva’s product (Nutrilon) which caused Teva considerable damage. Such marketing campaigns rely on algorithms to detect relevant ‘conversations’ where either fake profiles or real people are rallied to put forward a certain position, almost always without the audience (or other participants in the conversation) being aware of the algorithmic rally being deployed (let alone being deployed for money). In such cases, a civil lawsuit will have to determine the boundaries of such algorithmic campaigns (and the potential duties to disclose their source) and the relevant regime of civil liability (including consumer protection).
8.20 Conclusion
While in legal literature usually a very sceptical approach is adopted toward a mechanical application of rules, different indications come from other disciplines, which focus on the limits of intuitive judgments. For instance, the economist and psychologist Daniel Kahneman observes that in many cases, simple algorithms provide better results than human intuition, even when human capacities and attitudes have to be assessed.Footnote 89 What should be the procedures and norms according to which the state ought to regulate the adoption of AI to its own decision and assessment infrastructure? Should there be a difference between mere application AI, relevant for implementation decisions in highly codified contexts, to discretionary AI, which is designed to address regulatory decisions in a more open legal landscape, to policy-making algorithms, which are designed to assist in the policy formation level?
In the previous section, we considered in detail many issues emerging from the intermingling of AI and government, concluding that the law and its principles such as human rights and the rule of law are not averse to AI-based innovation, but that nonetheless serious concerns emerge. AI, where appropriately deployed, can contribute to more informed, efficient, and fair state action, provided some safeguards are maintained. For this purpose, human judgment must not be substituted by the ‘blind thought’ of AI systems, which process whatever kind of information is provided to them without understanding its meaning and the human goals and values at stake. Humans must be in the loop or at least over the loop in every deployment of AI in the public domain, and should be trained so as to be mindful of the potential risks associated with being influenced by scores and profiles in a manner inconsistent with what must ultimately be human judgment. The level of human involvement should therefore be correlated to the extent to which essentially human capacities are required, such as empathy, value judgments, and capacity to deal with unpredictable circumstances and exceptions.
A broader speculation concerns whether, or to what extent, the impact of AI will generate a change in the manner by which we are governed. More specifically, it concerns whether the law, as we understand it now, particularly in connection with the value of the rule of law, may be supplemented or even substituted by different ways to guiding human action, driven by the extensive deployment of AI.Footnote 90
The law, in its current form, is based on authoritative verbal messages, which are enacted in written form by legislative and administrative bodies. Such messages usually convey general instructions which order, prohibit or permit certain courses of action, and in so doing also convey a normative or moral position with respect to these actions. Interwoven within the legal apparatus are further norms that perform ancillary functions, by ascribing legal outcomes (or sanctions), qualifications to people and objects, as well as by creating institutional facts, institutions, and procedures. Legislation and administration are complemented by other written verbal messages, namely judicial or quasi-judicial decisions – which apply the law to specific cases, developing and specifying it – as well as by doctrinal writings which interpret and develop the norms, close gaps between the code and social life, and again generate expressive content pertaining to morality and identity. To become active, such verbal messages have to be understood by humans (the addressees of legal provisions), and this may require an act of interpretation. An act of human understanding is also required to comprehend and apply non-written sources of the law, such as customs and other normative practices. Once that the citizens or officers concerned have understood what the law requires from them in their circumstances, they will usually comply with the law, acting as it requires, but they may also choose to evade or violate the law (though this may entail the possibility of suffering the consequences of violation) or otherwise sidestep the legal rule by relying on a standard which may conflict with the rule or potentially mute its application. They may also approve or disapprove of the norms in question and voice their opposition. Thus, the law assumes, at least in a democratic state, that citizens are both free agents and critical reasoners.
It is unclear whether the law will preserve this form in the future, as AI systems are increasingly deployed by the state. This is problematic. In a world in which the governance of human action is primarily delegated to AI, citizens could either no longer experience genuine legal guidance (or experience it to a lesser extent), being rather nudged or manipulated to act as desired (and thus the law as such would be rendered irrelevant or much less relevant), or they would only or mainly experience the law through the mediation of AI systems.
The first option – the substitution of normativity with technology – would take place if human action were influenced in ways that prescind from the communication of norms.Footnote 91 The state might rather rely on ‘technoregulation’.Footnote 92 Such an architecture may open or preclude possibilities to act (enable or disable actions, as when access to virtual or digital facilities require automated identification), open or preclude possibilities to observe human action (enable or disable surveillance), facilitate or make more difficult, or more or less accessible certain opportunities (as it is the case for default choices which nudge users into determined options), directly perform action that impacts on the interests of concerning individuals (e.g., apply a tax or a fine, disabling the functioning of a device, such as a car, etc.), or may direct individuals through micro-targeted rewards and punishments towards purposes that may not be shared by or that are not even communicated to the concerned individuals. This is troubling, even dystopian, to the extent we care about human agency (and human dignity) as currently understood.
The second option, AI-mediated normativity, would take place if the state were to delegate to AI systems the formulation of concrete indications to citizens – on the predicted outcome of cases, or the actions to be done or avoided in a given context – without citizens having access to understandable rules and principles that support and justify such concrete indications. The citizens would just know that these concrete indications have been devised by the AI system itself, in order to optimise the achievement of the policy goals assigned to it. Citizens would be in a situation similar to that of a driver being guided step by step by a GPS system, without having access to the map showing the territory and the available routes toward the destination. Again, the implications regarding agency, meaningful participation in a community of moral agents, and human dignity are obvious (and troubling).
In summary, if these scenarios materialise, and especially if they materialise in a concentrated market (characterised by states and monopolies), we fear that humans may lose a significant component of control over the normative framework of their social action, as well as the ability to critically address such a normative framework. In this context, the state may no longer address its citizens (and lower officers as well) as fully autonomous agents, capable of grasping the law’s commands (and acting accordingly, based on such understanding, and on the reasons they have for complying).Footnote 93 This concern holds also for office-holders, who are often the direct subject of such instructions.Footnote 94 Moreover, it is unclear whether the state would still consider its citizen as agents capable of critical reflection, able to grasp the rationales of the commands (or instructions) and subject them to scrutiny, debate, and deliberation. Such a transformation entails a fundamental shift in the structure of communicationFootnote 95 underlying the legal system and thus raises significant moral legitimacy concerns.
We believe therefore that it is essential that the state continues to express its regulatory norms in human language, and that the human interpretation of such instructions, in the context of legal principles and political values, represents the reference for assessing the way in which the law is applied through AI systems, and more generally, the way in which the operation of such systems affects individual interests and social values.
In conclusion, AI puts forward significant opportunities but also a deep challenge to the state, as the latter debates the uses and misuses of AI.