Health-related quality of life (HRQoL) is an increasingly important outcome measure in healthcare, reflecting the transition of disease burden from infectious diseases to chronic conditions (Reference Murray and LopezMurray & Lopez, 1996) and the change from a paternalistic attitude to increased respect for autonomy and the subjective valuations of patients. Its measurement is important for rational public health policy, as it allows direct comparison between different conditions and interventions using quality-adjusted life-years (QALYs). Clinical psychiatry often concentrates on treating acute exacerbations of episodic disorders. The characteristics of patients seeking treatment are well known, but knowledge of the average severity of disorders at the population level is necessary for comparisons of the true burden of disorders. We investigated the HRQoL and QALY losses associated with pure and comorbid forms of DSM–IV (American Psychiatric Association, 1994) depressive and anxiety disorders and alcohol dependence in the general population of Finland.
METHOD
Health-related quality of life is the part of people's quality of life that health and healthcare can potentially influence. The results generated by some HRQoL instruments, such as the 15D measure (Reference SintonenSintonen, 1994) and the EQ–5D (EuroQol Group, 1990), can be summarised as a single score using utility theory and preferences elicited from the general population. The resulting score represents ‘health utilities’ and is a quantitative measure of the severity of health states, based on people's own preferences. Health utilities are anchored at 0 (equal to death) and 1 (perfect health), and are the ‘quality’ component of QALYs, which combine the length and quality of life into a single metric (Reference Dolan, Culver and NewhouseDolan, 2000) that is increasingly used for cost-effectiveness studies and rationing decisions. Among others, the National Institute for Health and Clinical Excellence uses QALYs as the preferred outcome measure in cost-effectiveness analysis (Reference Rawlins and CulyerRawlins & Culyer, 2004).
Survey design
This study was based on the Health 2000 survey (http://www.ktl.fi/health2000), which comprehensively represented the Finnish population aged 30 years and over. The survey had a two-stage, stratified cluster sampling design. The 15 largest towns and 65 healthcare districts were sampled as clusters, and a random sample of 8028 individuals was drawn from these areas, with double sampling of people over 80 years old. Data were collected between August 2000 and July 2001. The survey consisted of a health interview, a thorough health examination and self-report questionnaires (Reference Aromaa and KoskinenAromaa & Koskinen, 2004).
Socio-economic factors, chronic conditions and psychiatric diagnostics
Data on socio-economic factors and somatic diseases were collected using structured interviews at the participant's home or institution. Education was classified as basic, secondary or higher (Reference Aromaa and KoskinenAromaa & Koskinen, 2004). Family income, adjusted for family size (Organisation for Economic Cooperation and Development, 1982), was divided into quintiles. Participants were asked (separately for each condition) whether they had ever been diagnosed by a physician as having heart failure, coronary heart disease, hypertension, asthma, chronic obstructive pulmonary disease, unoperated cataract, glaucoma, macular degeneration, rheumatoid arthritis, arthrosis of hip or knee, other arthrosis, hearing loss, disturbing tinnitus, stroke, migraine, Parkinson's disease, permanent disability from accident, diabetes, psoriasis, inflammatory bowel disease, cancer or urinary incontinence. Problems of back or neck and disturbing allergy were included only if they had necessitated a visit to a physician in the preceding 12 months.
After the interview, participants were invited to a health examination. This included the Munich version of the Composite International Diagnostic Interview (M–CIDI; Reference Wittchen, Lachner and WunderlichWittchen et al, 1998), which was used to assess 12-month prevalence of depressive, anxiety or alcohol use disorders (Reference Pirkola, Isometsä and SuvisaariPirkola et al, 2005) defined by DSM–IV criteria. Conditions included in our study were major depressive disorder, dysthymia, alcohol dependence, agoraphobia, generalised anxiety disorder, panic disorder and social phobia. We controlled for self-reported psychosis or probable psychotic disorder identified by physician at the health examination in the regression analyses.
HRQoL measurement: EQ–5D and 15D
Participants were given a questionnaire including the EQ–5D at the home interview; only respondents fully completing the questionnaire were included in the analysis. The EQ–5D (EuroQoL Group, 1990; Reference BrooksBrooks, 1996) is among the most evaluated HRQoL measures (Reference Garratt, Schmidt and MackintoshGarratt et al, 2002) and is available from the EuroQol website (http://www.euroqol.org). The EQ–5D includes five dimensions: mobility, self-care, usual activities, pain or discomfort, and anxiety or depression. Each dimension has three grades of severity corresponding to no, moderate or extreme problems, so the EQ–5D can capture 243 different health states. We used the most common tariff, the UK time trade-off values (Reference Kind, Hardman and MacranKind et al, 1999) to convert these HRQoL states to health utility scores. Finnish and UK valuations of health states have been shown to be comparable (Reference Sintonen, Weijnen, Nieuwenhuizen, Brooks, Rabin and de CharroSintonen et al, 2003). The time trade-off method measures how much of their remaining life expectancy the respondents would be willing to trade off in order to be in perfect health. The EQ–5D time trade-off scores range from 1 (full health) to –0.59 (0, being dead).
Participants were given a questionnaire including the 15D at the health examination and asked to return it later by mail. Questionnaires with 12 or more completed 15D dimensions were included, and missing values were imputed (Reference SintonenSintonen, 1994). The 15D (available at http://www.15d-instrument.net) includes 15 dimensions: mobility, vision, hearing, breathing, sleeping, eating, speech, elimination, usual activities, mental function, discomfort and symptoms, depression, distress, vitality and sexual activity (Sintonen, Reference Sintonen1994, Reference Sintonen1995). Each dimension has five grades of severity, so the 15D is able to capture a vast number of health states. In calculating the 15D score, valuations elicited from the Finnish population using the multi-attribute utility method were used (Reference SintonenSintonen, 1995). Values range between 1 (full health) and 0 (dead).
Eighty-three per cent (n=6681) of participants completed either the 15D or EQ–5D; 77% completed the 15D (mean age 52.5 years), 77% completed the EQ–5D (mean age 52.3 years) and 70% completed both. The EQ–5D was completed approximately 1 month before the 15D. The M–CIDI was reliably completed by 75% of participants. All information needed for regression analyses was available for 68% (n=5422) of the sample for 15D and 65% (n=5219) of the sample for EQ–5D.
Statistical analyses
Both HRQoL measures had a ceiling effect: 47% of respondents (30% of those with psychiatric disorders) scored full health on the EQ–5D and 15% (5% of those with psychiatric disorders) did so on the 15D. The true variation in HRQoL among those scoring full health is not captured by the measures, i.e. the scores on these measures (especially EQ–5D) are censored. Because of this, we used the Tobit model (multiple regression for censored data) to estimate the impact of each of the reported disorders on HRQoL (Reference TobinTobin, 1958; Reference Austin, Escobar and KopecAustin et al, 2000). If the proportion of censoring is small, as it was for the 15D, the results of Tobit modelling approach those of ordinary linear regression. We report the marginal effects of the different disorders for the unconditional expected value of the HRQoL score, evaluated at the means of the explanatory variables (Reference CongCong, 2000). These marginal effects are interpreted as the change in HRQoL score associated with the disorder in question.
To estimate the modifying effect of socio-economic factors and somatic conditions on the HRQoL impact of psychiatric disorders, we created two different sets of regression models. The first controlled for age (six groups), gender, education (three categories), income (quintiles) and marital status. The second added the 25 chronic somatic conditions. Both models were done separately for each of the M–CIDI diagnoses. To estimate the impact of psychiatric comorbidity, we created two additional sets of regression models. The third models included all background variables, all M–CIDI diagnoses and psychosis in the same model. The fourth set of models estimated the impact of each pure psychiatric disorder and controlled for socio-economic factors, somatic conditions and psychosis. All models were done for 15D and EQ–5D separately. To investigate which dimensions of HRQoL were affected by the disorders, we used linear regression to adjust the losses on each 15D dimension for age and gender. This was done separately for alcohol dependence, anxiety disorders and depressive disorders. The 15D preference-based scoring system scales all dimensions between 0 and 1, making the losses comparable.
The HRQoL loss in the population associated with disorders was estimated by multiplying the marginal effect by the prevalence of the disorder. This is interpreted as the annual loss in QALYs resulting from the disorder, without considering mortality. The standard errors and confidence intervals were calculated using the delta method (Reference Migon and GamermanMigon & Gamerman, 1999). The results are reported as annual QALY loss per 100 000 persons. Analyses were conducted on the largest possible number of participants. A weighting adjustment was used to take into account the sampling design and non-participation (Reference Aromaa and KoskinenAromaa & Koskinen, 2004). Analyses were performed using Stata version 8.2 for Windows.
RESULTS
Demographic characteristics, prevalence of disorders, proportions of associated somatic and psychiatric comorbidity, mean unadjusted HRQoL scores and proportion of participants reporting full HRQoL are given in Table 1. Psychiatric comorbidity was common and varied widely between disorders.
Table 1 Prevalence of psychiatric disorders, age, somatic and psychiatric comorbidity and HRQoL scores of respondents
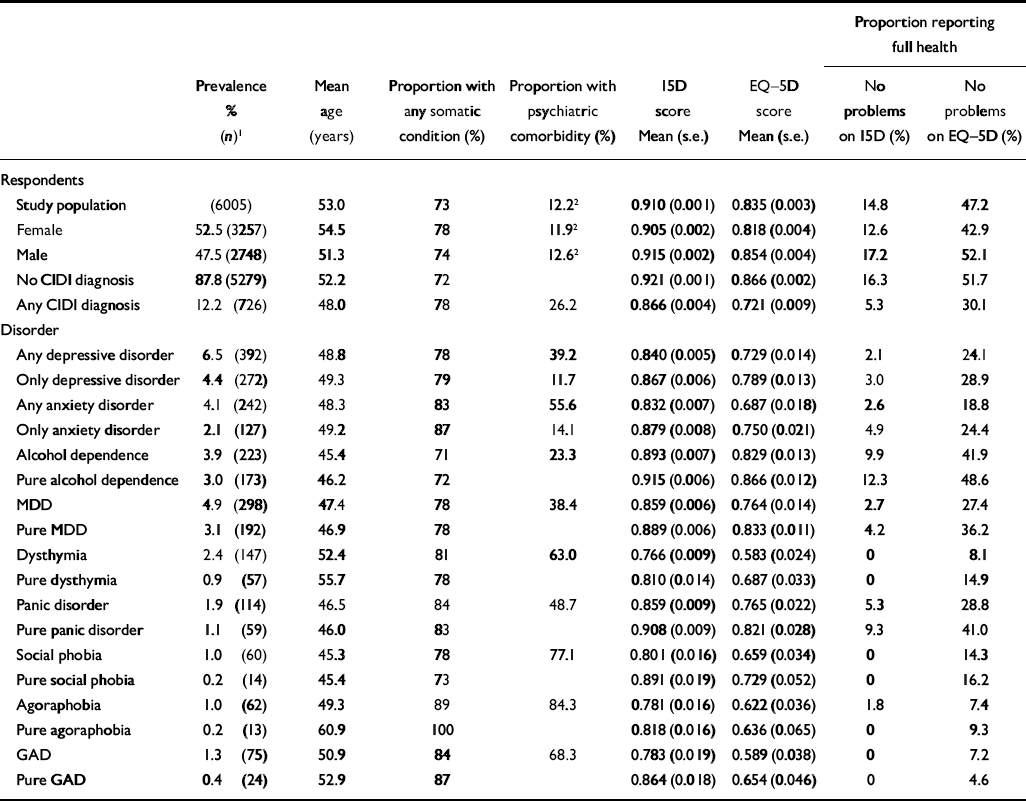
Proportion reporting full health | ||||||||
---|---|---|---|---|---|---|---|---|
Prevalence % (n)1 | Mean age (years) | Proportion with any somatic condition (%) | Proportion with psychiatric comorbidity (%) | 15D score Mean (s.e.) | EQ—5D score Mean (s.e.) | No problems on 15D (%) | No problems on EQ—5D (%) | |
Respondents | ||||||||
Study population | (6005) | 53.0 | 73 | 12.22 | 0.910 (0.001) | 0.835 (0.003) | 14.8 | 47.2 |
Female | 52.5 (3257) | 54.5 | 78 | 11.92 | 0.905 (0.002) | 0.818 (0.004) | 12.6 | 42.9 |
Male | 47.5 (2748) | 51.3 | 74 | 12.62 | 0.915 (0.002) | 0.854 (0.004) | 17.2 | 52.1 |
No CIDI diagnosis | 87.8 (5279) | 52.2 | 72 | 0.921 (0.001) | 0.866 (0.002) | 16.3 | 51.7 | |
Any CIDI diagnosis | 12.2 (726) | 48.0 | 78 | 26.2 | 0.866 (0.004) | 0.721 (0.009) | 5.3 | 30.1 |
Disorder | ||||||||
Any depressive disorder | 6.5 (392) | 48.8 | 78 | 39.2 | 0.840 (0.005) | 0.729 (0.014) | 2.1 | 24.1 |
Only depressive disorder | 4.4 (272) | 49.3 | 79 | 11.7 | 0.867 (0.006) | 0.789 (0.013) | 3.0 | 28.9 |
Any anxiety disorder | 4.1 (242) | 48.3 | 83 | 55.6 | 0.832 (0.007) | 0.687 (0.018) | 2.6 | 18.8 |
Only anxiety disorder | 2.1 (127) | 49.2 | 87 | 14.1 | 0.879 (0.008) | 0.750 (0.021) | 4.9 | 24.4 |
Alcohol dependence | 3.9 (223) | 45.4 | 71 | 23.3 | 0.893 (0.007) | 0.829 (0.013) | 9.9 | 41.9 |
Pure alcohol dependence | 3.0 (173) | 46.2 | 72 | 0.915 (0.006) | 0.866 (0.012) | 12.3 | 48.6 | |
MDD | 4.9 (298) | 47.4 | 78 | 38.4 | 0.859 (0.006) | 0.764 (0.014) | 2.7 | 27.4 |
Pure MDD | 3.1 (192) | 46.9 | 78 | 0.889 (0.006) | 0.833 (0.011) | 4.2 | 36.2 | |
Dysthymia | 2.4 (147) | 52.4 | 81 | 63.0 | 0.766 (0.009) | 0.583 (0.024) | 0 | 8.1 |
Pure dysthymia | 0.9 (57) | 55.7 | 78 | 0.810 (0.014) | 0.687 (0.033) | 0 | 14.9 | |
Panic disorder | 1.9 (114) | 46.5 | 84 | 48.7 | 0.859 (0.009) | 0.765 (0.022) | 5.3 | 28.8 |
Pure panic disorder | 1.1 (59) | 46.0 | 83 | 0.908 (0.009) | 0.821 (0.028) | 9.3 | 41.0 | |
Social phobia | 1.0 (60) | 45.3 | 78 | 77.1 | 0.801 (0.016) | 0.659 (0.034) | 0 | 14.3 |
Pure social phobia | 0.2 (14) | 45.4 | 73 | 0.891 (0.019) | 0.729 (0.052) | 0 | 16.2 | |
Agoraphobia | 1.0 (62) | 49.3 | 89 | 84.3 | 0.781 (0.016) | 0.622 (0.036) | 1.8 | 7.4 |
Pure agoraphobia | 0.2 (13) | 60.9 | 100 | 0.818 (0.016) | 0.636 (0.065) | 0 | 9.3 | |
GAD | 1.3 (75) | 50.9 | 84 | 68.3 | 0.783 (0.019) | 0.589 (0.038) | 0 | 7.2 |
Pure GAD | 0.4 (24) | 52.9 | 87 | 0.864 (0.018) | 0.654 (0.046) | 0 | 4.6 |
HRQoL scores
The average unadjusted 15D score for the population was 0.91 and for people with any psychiatric diagnosis it was 0.87. On the EQ–5D the respective scores were 0.83 and 0.72. The lowest scores were reported by people with dysthymia, agoraphobia, generalised anxiety disorder and social phobia.
Socio-economic factors and somatic conditions
The adjusted HRQoL scores are reported in Figs 1 and 2 (further information is available in the data supplement to the online version of this paper). After socio-economic variables are controlled for the largest HRQoL impacts on both the 15D (0.13–0.14) and the EQ–5D (0.24–0.27) are associated with dysthymia, agoraphobia, generalised anxiety disorder and social phobia. Alcohol dependence had the lowest impact (0.04 on 15D and 0.07 on EQ–5D). The inclusion of somatic conditions resulted in small decreases of the HRQoL impacts associated with M–CIDI diagnoses.

Fig. 1 Health utility loss measured with the 15D, controlling for socio-economic status (SES), somatic comorbidity, psychiatric comorbidity and pure forms of conditions, marginal effects and 95% confidence intervals (GAD, generalised anxiety disorder; MDD, major depressive disorder).

Fig. 2 Health utility loss measured with the EQ–5D, controlling for socio-economic status (SES), somatic comorbidity, psychiatric comorbidity and pure forms of conditions, marginal effects and 95% confidence intervals (GAD, generalised anxiety disorder; MDD, major depressive disorder).
Psychiatric comorbidity
The inclusion of all psychiatric disorders in the same regression model decreased the impact of individual disorders clearly. The relative decrease was largest for anxiety disorders and smallest for alcohol dependence. On 15D the largest loss of HRQoL was associated with dysthymia 0.08 (95% CI 0.07–0.10), social phobia 0.06 (95% CI 0.03–0.08) and generalised anxiety disorder 0.05 (95% CI 0.03–0.07). A smaller but still significant effect was associated with major depressive disorder (0.03, 95% CI 0.03–0.04) and agoraphobia (0.03, 95% CI 0.01–0.05), followed by alcohol dependence (0.02, 95% CI 0.01–0.04) and panic disorder (0.01, 95% CI 0.00–0.03, P=0.012). On EQ–5D the picture was similar. When considering the pure forms of disorders, i.e. only those respondents receiving one M–CIDI diagnosis, the largest impacts on both 15D and EQ–5D were associated with dysthymia, social phobia, generalised anxiety disorder and major depressive disorder. Agoraphobia and panic disorder did not reach statistical significance on either scale. Pure forms of anxiety disorders were relatively rare, the smallest groups being pure social phobia (n=14) and pure agoraphobia (n=13).
Dimensions of HRQoL affected
The HRQoL profiles of alcohol dependence, anxiety disorders and depressive disorders are strikingly similar, although the effect of alcohol dependence is smaller on all dimensions (Fig. 3). The domains of HRQoL most affected are the same for all disorders: depression, distress, vitality and sleeping. A statistically significant decrease in quality of life was found on almost all dimensions of HRQoL.
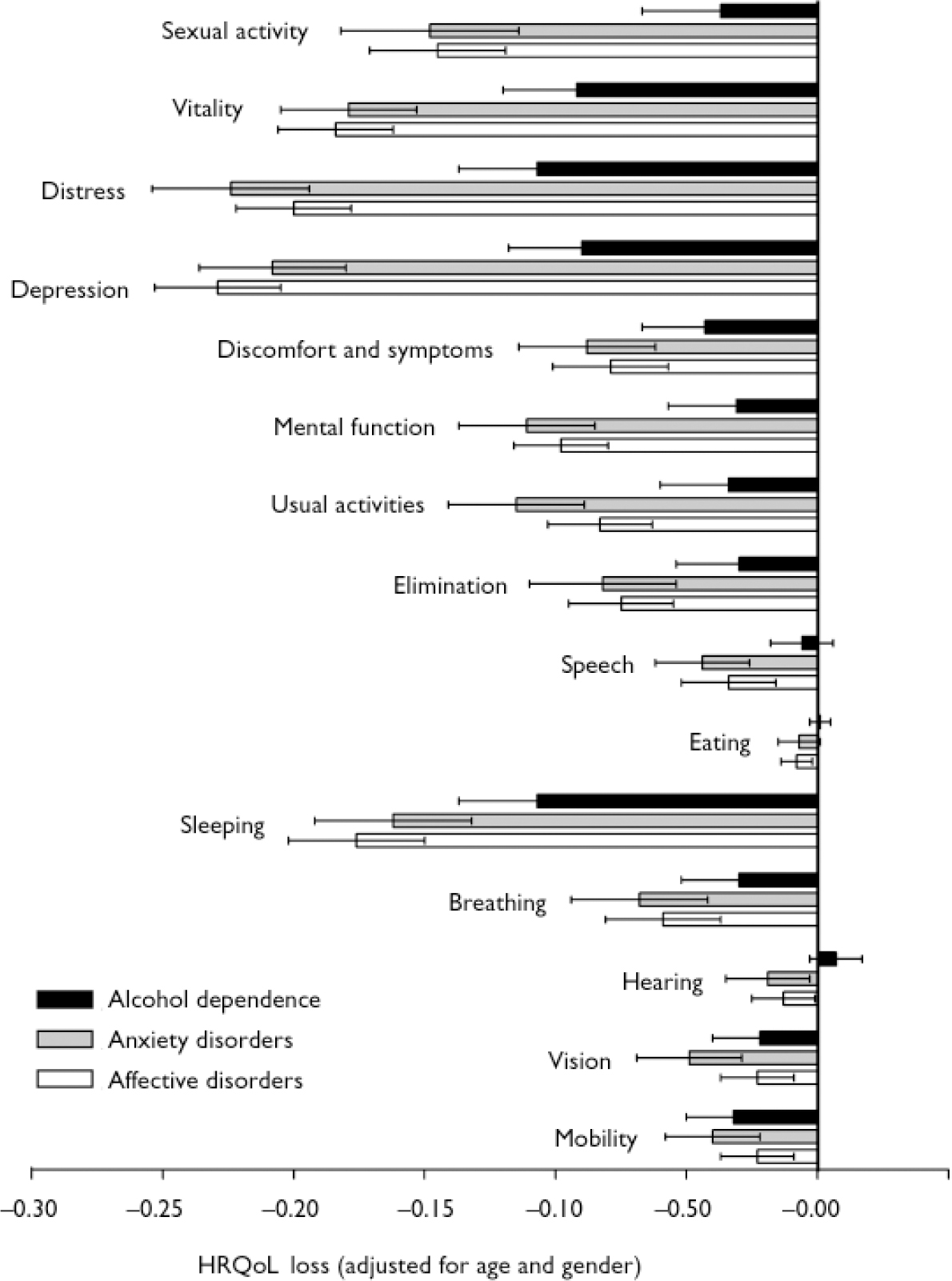
Fig. 3 The 15D dimensions of health-related quality of life (HRQoL) affected by alcohol dependence, anxiety and affective disorders, controlling for age and gender, and 95% confidence intervals.
Population-level QALY losses
Annual QALY losses per 100 000 persons are shown in Table 2. Dysthymia and major depressive disorder were associated with the largest loss of QALYs. Alcohol dependence had a smaller impact, followed by anxiety disorders, of which generalised anxiety disorder and social phobia were associated with the largest losses of QALYs. Combined as groups, depressive disorders were associated with approximately 55%, anxiety disorders with 30% and alcohol dependence with 15% of the QALY losses associated with the disorders included in this study.
Table 2 Annual losses of quality-adjusted life-years associated with different disorders, measured with 15D and EQ–5D

Disorder | Annual loss of QALYs | |
---|---|---|
15D Loss (95% CI) | EQ—5D Loss (95% CI) | |
Dysthymia | 206 (153-259) | 298 (177-419) |
MDD | 179 (119-220) | 284 (174-394) |
Alcohol dependence | 99 (58-139) | 159 (69-249) |
GAD | 66 (35-97) | 140 (70-211) |
Social phobia | 58 (30-86) | 104 (35-173) |
Agoraphobia | 41 (8-73) | 69 (7-145) |
Panic disorder | 26 (2-49) | 48 (22-119) |
DISCUSSION
We conducted a comprehensive population survey to estimate the impact of pure and comorbid forms of depressive and anxiety disorders and alcohol dependence on health-related quality of life. We used two established, generic HRQoL instruments, the 15D and EQ–5D, which enable estimation of health utilities loss associated with disorders. This is important, as health utilities are used to calculate QALYs, the measure commonly recommended and increasingly used for cost-effectiveness analysis. Estimation of the true burden that psychiatric disorders place on HRQoL is essential for rational public health policy and for setting priorities in healthcare.
We found that after controlling for socio-economic factors and somatic comorbidity, the typically chronic disorders of dysthymia, agoraphobia, generalised anxiety disorder and social phobia were associated with the largest losses in HRQoL. When considering the impact on HRQoL and prevalence together, dysthymia was associated with the largest annual loss of QALYs, followed by major depressive disorder. Generalised anxiety disorder and social phobia had the largest impact of the anxiety disorders. The lowest unadjusted HRQoL scores in this study (dysthymia, generalised anxiety disorder) were below 0.8 on 15D and below 0.6 on EQ–5D. To put these scores in perspective, they are similar to those reported by people 20 years older who had somatic conditions that decreased the HRQoL most, i.e. Parkinson's disease and heart failure. Adjusted for socio-economic factors and somatic comorbidity, the HRQoL scores for chronic psychiatric disorders were clearly lower than scores for any of the somatic conditions included in our previous study (Reference Saarni, Härkänen and SintonenSaarni et al, 2006). The large impact of psychiatric disorder is understandable considering the many dimensions of quality of life that these disorders influence, the relative importance of mental health domains to total HRQoL, and the young age at which these disorders present (Reference Katschnig, Freeman and SartoriusKatschnig et al, 1997).
Comparison with previous studies
It is well established that affective and anxiety disorders cause significant distress, lowered HRQoL and disability on several domains of life. The impact of alcohol use disorders generally appears smaller (Reference Ormel, Von Korff and UstunOrmel et al, 1994; Reference Bijl and RavelliBijl & Ravelli, 2000; Reference Alonso, Angermeyer and BernertAlonso et al, 2004b ; Reference Sareen, Stein and CampbellSareen et al, 2005). However, most HRQoL surveys have used the 36-item Short Form Health Survey (Reference Ware and SherbourneWare & Sherbourne, 1992) or related instruments, which report the impact of disorders on HRQoL in several domains but do not combine them as a single-dimensional utility score. We therefore compare our findings with three other types of studies: ‘burden of disease’ studies, direct utility valuation exercises and selected clinical studies.
Burden of disease studies
The ‘burden of disease’ studies estimate years lived with disability (YLD) and, adding mortality, disability-adjusted life-years (DALY, Reference Murray and LopezMurray & Lopez, 1996). The YLD method uses constant disability weights which are combined with prevalence estimates. Generally, our results suggest that dysthymia is more serious than the burden of disease studies estimate, and alcohol dependence less so (Reference Murray and LopezMurray & Lopez, 1996; Reference Mathers, Vos and StevensonMathers et al, 1999; Reference Melse, Essink-Bot and KramersMelse et al, 2000). We found large differences in severity between different anxiety disorders. The original Global Burden of Disease study (Reference Murray and LopezMurray & Lopez, 1996) and further World Health Organization studies, which have received great attention and emphasised the burden of depression, did not include a thorough list of anxiety disorders. Later studies with a more comprehensive list of anxiety disorders are in line with our results in showing that the burden of anxiety disorders is close to the burden of depressive disorders (Reference Mathers, Vos and StevensonMathers et al, 1999; Reference Melse, Essink-Bot and KramersMelse et al, 2000).
An Australian burden of disease study used different weights for different anxiety disorders and varying severities of disorders (Reference Mathers, Vos and StevensonMathers et al, 1999). Alcohol dependence was weighted between moderate and severe depression, and dysthymia was weighted equal to mild depression. Differences in severity between mild and severe forms of disorders were estimated to be 3- to 5-fold. Compared with this, the differences between anxiety disorders were small. This approach, consistent with results of other valuation exercises (Reference Revicki and WoodRevicki & Wood, 1998; Reference Bennett, Torrance and BoyleBennett et al, 2000), highlights how a psychiatric diagnosis as such does not determine the associated disability, but that disorder severity and longitudinal course are more important. This emphasises the importance of gathering HRQoL and diagnostic information simultaneously, as was done in our study.
Clinical studies
Most clinical studies using utility-based HRQoL instruments concern depression (Reference Foster, Powell and MarshallFoster et al, 1999; Reference Mogotsi, Kaminer and SteinMogotsi et al, 2000). A study using EQ–5D (Reference Sapin, Fantino and NowickiSapin et al, 2004) on people with major depressive disorder treated as patients in France found a baseline EQ–5D index mean value of 0.33, with 8% scoring below 0. The EQ–5D score improved in 8 weeks to 0.78. A Finnish study of patients receiving psychiatric treatment for major depressive disorder reported a baseline 15D score of 0.72, which improved to 0.86–0.89 at week 18 (Reference Lönnqvist, Sihvo and SyvälahtiLönnqvist et al, 1995). A British study using the EQ–5D to assess a clinical sample of currently drinking participants with alcohol dependency (Reference Foster, Peters and KindFoster et al, 2002) found a mean EQ–5D score of 0.45. These HRQoL scores of treatment-seeking individuals are very low compared with the population scores in our study, which again emphasises the impact of severity on HRQoL scores.
Direct valuation exercises
The health utility losses associated with different conditions can also be estimated using different direct valuation techniques, most commonly standard gamble or time trade-off techniques. A Swedish postal survey (Reference Isacson, Bingefors and von KnorringIsacson et al, 2005) investigated the time trade-off valuations of current health of people who also reported feelings of depression. After gender, age and other conditions were controlled for, the presence of depressive feelings was associated with a decrease of 0.090 health utilities and self-reported anxiety with a decrease of 0.045 health utilities. A large study of managedcare patients in the USA (Reference Wells and SherbourneWells & Sherbourne, 1999) using both standard gamble and time trade-off methods found that after adjustment for somatic conditions and socio-economic variables, probable 12-month depression was associated with loss of 0.079 health utilities on time trade-off and 0.036 on standard gamble. These results are roughly in line with our findings.
Study strengths and weaknesses
To our knowledge, this study is the first comprehensive population survey reliably diagnosing psychiatric disorders and measuring the associated loss of health utilities, using two different established HRQoL measures. The most important strength of our study is that it estimates the HRQoL burden of the major non-psychotic psychiatric disorders as they occur in the population. The use of two HRQoL measures permits more valid estimation of HRQoL and the comparison of the measures, as there is no gold standard of HRQoL measurement but rather a vast variety of different generic and condition-specific measures (Reference Garratt, Schmidt and MackintoshGarratt et al, 2002). As the EQ–5D is insensitive at the upper range of HRQoL, it would be problematic to use it alone in general population surveys.
Our study aimed to estimate the individual, additive contribution of each disorder on HRQoL. It is likely, however, that there are complex interactions between the disorders, modifying their effects. To overcome this we also investigated pure forms of disorders. However, pure disorders are rare and may thus actually represent atypical forms. Our approach of assuming individual, additive effects of DSM–IV disorders on HRQoL is supported by the mostly comparable results of these two estimations.
We controlled for the most common chronic conditions in our analysis. However, as these diagnoses were based on self-report, their reliability is not known. We did not include mortality when estimating the annual QALY loss associated with disorders. This means that our QALY estimates probably underestimated the total burden of alcohol dependence, as alcohol is associated with more excess mortality than anxiety or depressive disorders (Reference Murray and LopezMurray & Lopez, 1996; Reference Melse, Essink-Bot and KramersMelse et al, 2000).
It is important to note that because we used the 12-month prevalence of disorders, some people with typically episodic disorders (such as major depressive disorder) were in remission at the time of the HRQoL measurement. The transient impact of episodic disorders at their worst phase is thus larger than the averages needed to estimate the overall burden of disorders. However, the use of 12-month prevalence is necessary, as it enables the comparison of the total burden of chronic and episodic disorders.
Implications
We have shown how chronic disorders – dysthymia, generalised anxiety disorder and social phobia – are associated with larger losses of HRQoL, at both individual and population levels, than more episodic disorders. This is true both before and after controlling for somatic and psychiatric disorders, even though comorbidity is very common. The HRQoL scores reported by people with these disorders are low also in comparison with people with severe somatic conditions, despite the fact that our survey HRQoL results are clearly higher than those of previous clinical studies. Our method enables true comparison between chronic and episodic disorders as they appear in the population. This might explain the contrast with the burden of disease study findings, which have equated dysthymia to mild cases of major depressive disorder. The impact of alcohol dependence on HRQoL is smaller than that of depressive and anxiety disorders. This appears to be due to differences in the general severity of disorders, rather than differences in the dimensions of HRQoL affected. People with depressive and anxiety disorders have almost identical HRQoL profiles; this is an interesting finding from the point of view of diagnostic systems, and requires further study.
On the population level, the impact of dysthymia on quality-adjusted life-years is comparable with that of major depressive disorder. This is an important finding, as dysthymia might require different treatment and recognition strategies from the latter disorder. Anxiety disorders can have a more serious effect on HRQoL than major depressive disorder, and a public health impact close to that of depressive disorders. This is important, as it appears that chronic anxiety disorders, especially agoraphobia and social phobia, receive treatment even more rarely and with longer delay than depressive disorders (Reference Alonso, Angermeyer and BernertAlonso et al, 2004a ; Reference Wang, Berglund and OlfsonWang et al, 2005). Anxiety disorders and dysthymia should be recognised as major public health concerns and treated accordingly.
Acknowledgement
S.I.S. received a grant from the Signe and Ane Gyllenberg Foundation.
eLetters
No eLetters have been published for this article.