1. Introduction
‘Design cognition’ refers to the mental processes and representations involved in designing (Hay et al. Reference Hay, Duffy, McTeague, Pidgeon, Vuletic and Grealy2017a). Since the emergence of design research in the 1960s, it has been recognized that a comprehensive understanding of design requires robust knowledge of its underlying cognitive processes. Analysis of design cognition informs understanding of the designer and the design team, the design process, the evolving design artefact, and ultimately, the design artefact’s users. Over the last 60 years, there have been extensive efforts to map these processes and their interactions. Design cognition analysis is now a major research area spanning multiple domains.
Despite its importance, design cognition analysis faces significant challenges moving into the future. Whilst cognitive psychology has successfully established a coherent body of scientific knowledge through a theory-driven approach, that is, abstracting knowledge ‘from the specifics of context, field and subject’ (Cash Reference Cash2018, p. 86), design cognition remains relatively immature as a scientific discipline. Specifically, while there has been extensive exploration of various phenomena using qualitative or mixed methods such as protocol analysis (Hay et al. Reference Hay, Duffy, McTeague, Pidgeon, Vuletic and Grealy2017a) and case studies (e.g., Christensen & Schunn Reference Christensen and Schunn2007; Yilmaz & Seifert Reference Yilmaz and Seifert2011; Yilmaz et al. Reference Yilmaz, Daly, Christian, Seifert and Gonzalez2014), there has been little movement towards prediction, testing and theory development (Cash Reference Cash2018). Thus, there is a general acknowledgment that major change is needed to overcome stagnation in design cognition research topics and methodologies (Dinar et al. Reference Dinar, Shah, Cagan, Leifer, Linsey, Smith and Hernandez2015; Sio et al. Reference Sio, Kotovsky and Cagan2015; Crilly & Cardoso Reference Crilly and Cardoso2017; Hay et al. Reference Hay, Duffy, McTeague, Pidgeon, Vuletic and Grealy2017a, Reference Hay, Duffy, McTeague, Pidgeon, Vuletic and Grealy2017b; Cash Reference Cash2018).
In terms of what kind of changes may be required, Dinar et al. (Reference Dinar, Shah, Cagan, Leifer, Linsey, Smith and Hernandez2015) and Hay et al. (Reference Hay, Duffy, McTeague, Pidgeon, Vuletic and Grealy2017a) highlight the need to expand the repertoire of research methods to include quantitative approaches suited to robust hypothesis testing and the study of larger samples. Hay et al. (Reference Hay, Duffy, McTeague, Pidgeon, Vuletic and Grealy2017b) emphasize the need to address ontological fragmentation preventing comparison, meta-analysis and generalization. Further, Cash, Škec & Štorga (Reference Cash, Škec and Štorga2019b) stress the need to consider how existing psychological and neuropsychological theories may be applied to designing. Addressing these challenges requires us to better understand the history and current status of design cognition research, as well as open fresh discussions regarding its potential near- and long-term future. It is this need that we aim to address in this editorial and thematic collection.
The thematic collection takes stock of current research, explores emerging topics and methodologies, and identifies future directions for inquiry. Against this backdrop, this editorial examines key issues regarding both what we investigate and how we conduct this research. We present a vision formed from a structured literature review, the work of authors in the collection, and the views of a broad cross-section of the design cognition community. Although predictions of the future are inherently uncertain (Miller & Feigh Reference Miller and Feigh2019), we challenge the status quo and provide a concrete foundation for future research.
2. A brief history
Design cognition research can be traced back to protocol studies by Charles Eastman in the 1960s (Eastman Reference Eastman1969, Reference Eastman and Moore1970). These, and subsequent studies, gave rise to two distinct research themes: one associated with understanding design itself, and one associated with the methodological and analytical means for evaluating cognition.
In terms of understanding design, the last 60 years have seen three major paradigms: the Simon-based problem solving paradigm; the Schon-based reflective paradigm; and the situated cognition paradigm (Newell & Simon Reference Newell and Simon1972; Schön Reference Schön1983; Gero & Kannengiesser Reference Gero and Kannengiesser2004). The problem solving paradigm was influenced by information processing models of cognition established in the 1970s–1980s. Here, Herbert Simon and Allan Newell’s work on human problem solving is considered foundational (Newell & Simon Reference Newell and Simon1956, Reference Newell and Simon1972; Simon Reference Simon1996). Following this, the 1980s–1990s saw a paradigm shift towards an understanding of designing rooted in Donald Schon’s conceptualization of professional practice as ‘reflection in action’ (Schön Reference Schön1983; Schon & Wiggins Reference Schon and Wiggins1992). Whilst proponents of problem solving view designing as a search process transforming knowledge to address a fixed problem, the reflective paradigm offers a different perspective. Here, design problems and solutions are subject to reinterpretation and reformulation in a process shaped by the design context (Gero Reference Gero1990; Lloyd & Scott Reference Lloyd and Scott1994; Dorst & Dijkhuis Reference Dorst and Dijkhuis1995; Murray et al. Reference Murray, Studer, Daly, McKilligan and Seifert2019). Most recently, the 1990s–2000s have seen designing formalized as a situated, exploratory, and evolutionary process by Goldschmidt (Reference Goldschmidt1991), Maher et al. (Reference Maher, Poon, Boulanger, Gero and Sudweeks1996), Gero & Kannengiesser (Reference Gero and Kannengiesser2004) and others. This has prompted a revitalization of the link to cognitive science and conceptualizations of design founded in cognition (e.g., Jin & Chusilp Reference Jin and Chusilp2006; Park & Kim Reference Park and Kim2007; Jin & Benami Reference Jin and Benami2010), with several moves towards the application of modern psychological theory in design research (e.g., Kannengiesser & Gero Reference Kannengiesser and Gero2019; Perišić, Štorga & Gero Reference Perišić, Štorga and Gero2019a).
In terms of the means for evaluating design cognition, protocol analysis has dominated the field since its inception, based on the seminal work of Ericsson & Simon (Reference Ericsson and Simon1984). Whilst protocol analysis emerged from cognitive science in the 1970–1980s (Ericsson & Crutcher Reference Ericsson and Crutcher1991), cognitive scientists themselves have developed a much broader range of approaches, including, for example, behavioural experiments, psychometrics, neuroimaging and computational modelling, to highlight a select few. These methods have all been applied to study generic creative thinking tasks in cognitive science (e.g., Torrance Reference Torrance1972; Ward et al. Reference Ward, Patterson and Sifonis2004; Wiggins & Bhattacharya Reference Wiggins and Bhattacharya2014; Benedek et al. Reference Benedek, Schües, Beaty, Jauk, Koschutnig, Fink and Neubauer2018), but have yet to be extensively applied in design cognition research. However, there is evidence that change is afoot. For instance, there have been efforts to develop standardized psychological tests of design ability (Shah et al. Reference Shah, Millsap, Woodward and Smith2012, Reference Shah, Millsap, Woodward and Smith2013; Khorshidi et al. Reference Khorshidi, Shah and Woodward2014), and recent years have seen a small but growing number of neuroimaging studies of design employing EEG (Liu et al. Reference Liu, Li, Xiong, Cao and Yuan2018; Nguyen et al. Reference Nguyen, Nguyen and Zeng2018; Vieira et al. Reference Vieira, Gero, Delmoral, Gattol, Fernandes and Fernandes2019), fMRI (Alexiou et al. Reference Alexiou, Zamenopoulos, Johnson and Gilbert2009; Goucher-Lambert et al. Reference Goucher-Lambert, Moss and Cagan2019; Hay et al. Reference Hay, Duffy, Gilbert, Lyall, Campbell, Coyle and Grealy2019) and fNIRS (Shealy & Gero Reference Shealy and Gero2019). As such, it seems likely that design cognition research is on the cusp of a major methodological paradigm shift, bringing new perspectives in addition to classical protocol analysis approaches.
Together, these themes highlight the dynamic evolution of design cognition research, and the need for both retrospection and foresight in moving the field forward.
3. Approach
In writing this editorial, we have derived insights from three key data sources: (i) a structured review of existing literature in major design journals publishing design cognition research; (ii) a structured elicitation and thematic analysis of views across the research community; and (iii) manuscripts in this thematic collection. Our approach entailed the following:
3.1. Structured literature review
Our analysis consisted of the following steps: (i) download all papers in Design Studies, Design Science, International Journal of Design, Journal of Engineering Design and Research In Engineering Design between January 2004 and February 2019 (1522 articles in total); (ii) identify those related to cognition via the keyword ‘cognition’ in title abstract or keywords (207 articles); and (iii) iterative analysis of the text used in the title, abstract and keywords in order to distil key terms describing the topics addressed in the field. This resulted in 11 high level topics, further divided into 31 subtopics. Importantly, many papers contribute to multiple topics, so this should not be considered an exclusive classification, rather it reflects an iterative analysis of the keywords, contribution claims and self-identified research foci within the articles themselves.
3.2. Concept mapping and thematic analysis
We collected concept maps (Johnson et al. Reference Johnson, Lee, Lee, O’Connor, Khalil and Huang2007; Johnson & O’Connor Reference Johnson and O’Connor2008) from nine early career, six mid-career and seven senior design cognition researchers (see Appendix Table A), outlining their views on the state of the art, key challenges for design cognition analysis in the next 10–20 years, and their far future vision for the field in the next 50 years and beyond. Participants were instructed to draw maps highlighting what they viewed as the key concepts/issues involved and the connections among them. The maps were produced in a workshop at the 8th International Conference on Design Computing and Cognition 2018, as well as individually by several other scholars. We conducted a thematic analysis on the maps to identify salient concepts/issues across the sample.
3.3. Papers in the collection
Around 40 authors/groups of authors were initially contacted or expressed interest in the collection. A total of 13 submissions were received, with 5 accepted. Over 30 peer reviewers were involved in evaluating the submissions. The accepted papers cover a range of topics. Sosa (Reference Sosa2019) focuses on theory development, applying the concept of accretion to propose a model of creative design ideation. Lapp et al. (Reference Lapp, Jablokow and McComb2019) present a new approach using agent-based simulation to study cognitive style in design teams. Miller & Feigh (Reference Miller and Feigh2019) present a novel application of an existing method to study design cognition – the former applying Cognitive Work Analysis in the context of the envisioned world problem, and the latter exploring the use of virtual reality to study ideation. Gero & Milovanovic (Reference Gero and Milovanovic2020) provide a detailed review of the cognitive, physiological and neurophysiological measures now available to design cognition researchers, and propose a roadmap for work in this area. Finally, Carbon (Reference Carbon2019) shifts focus from the designer’s cognition to that of the user, with a proposition for applications of cognitive theory to product design practice.
4. State of the art
Papers self-identified by the authors as design cognition research account for ~14% of all publications in the reviewed journals between 2004 and 2019. Collectively these showed distinct trends in both what and how we study design cognition.
Our analysis revealed six major topic areas, illustrated in Figure 1: designer; design process; design artefact; user; cognitive processes; and research methods. Decomposing these reveals a number of topics that dominate what we study. For example, in terms of design process, 71% of articles deal with some aspect of conceptual and creative design, reflecting the fundamental importance of creativity in design theory and practice. Further, aspects of design process, strategies and methods are discussed in 79% of the sample. Shifting focus, 81% of papers deal with some aspect of the artefact and artefact attributes, particularly the pervasive notions of function, behaviour and structure. In terms of cognitive processes, problem solving continues to be a central focus – discussed in 70% of papers. Although less extensive, there is also substantial work spanning a wide range of lower-order cognitive processes, for example, semantic processing (42%), perception and mental imagery (34%), spatial processing (17%), conceptual combination (11%) and attention (6%). Finally, there are a number of topics that serve to distinguish us from other fields concerned with human cognition: use of temporally sensitive research methods to reveal design dynamics (19%); cognition in collaborative/group design settings (31%); and the broader socio/cultural context of design (9%).
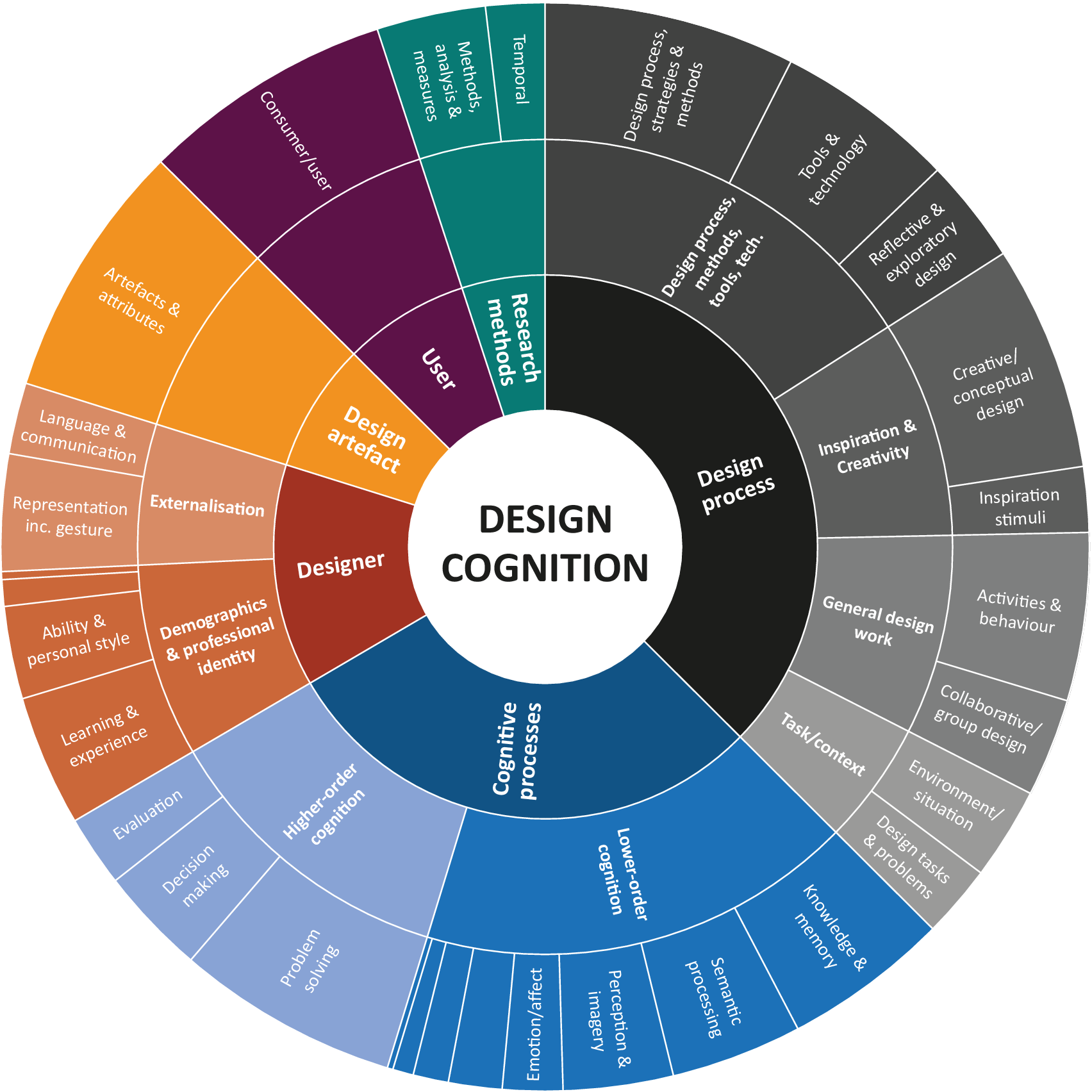
Figure 1. The design cognition landscape – distribution of focus across design cognition topics in the design literature between 2004 and 2019. See Appendix Table B for full breakdown and examples.
In terms of how we study the above topics, thematic analysis of the concept maps coupled with analysis of the literature highlighted three salient and interconnected methodological themes: (i) sampling, that is, how and who we select as designers to participate in our studies; (ii) how we measure cognition; and (iii) the approaches we use to study design. The range of themes here was significantly less diverse than the topics highlighted in Figure 1.
4.1. Sampling
Sampling decisions are largely driven by factors such as: the need to understand both individual and team cognition in design; cross-domain comparisons and the impacts of domain differences; and the effects of design expertise. There is a tendency to focus on small samples, which afford rich in-depth data. In this respect, there has been little discussion in design cognition research on the key drivers of rigorous sampling decisions. There is also little consensus on when variation in designer attributes such as expertise, education level and design background may have undesirable effects on study results, and how this should be addressed by design cognition sampling (Sjoberg et al. Reference Sjoberg, Anda, Arisholm, Dyba, Jorgensen, Karahasanovic, Koren and Vokac2002; Stevens Reference Stevens2011).
4.2. Design cognition measures
As discussed in this collection by Gero & Milovanovic (Reference Gero and Milovanovic2020), currently applied measures include: (i) those based on conventional analysis of design processes and outcomes, for example, the protocol-based P–S index applied by Milovanovic & Gero (Reference Milovanovic and Gero2018) and the outcome-based ideation metrics proposed by Shah et al. (2009); (ii) a growing body of physiological measures that can be related to various aspects of affective and mental processing, for example, eye tracking, galvanic skin resistance, and heart rate; and (iii) neurophysiological measures based on cerebral blood flow (in fMRI and fNIRS) and electrical activity (in EEG), which can provide information on the brain activation underpinning cognitive processing. These measures can provide different perspectives on design cognition, but none of them measure cognition directly. Thus, a key issue is how we interpret and link them to cognitive processing. The use of physiological and neurophysiological measures brings new interpretative challenges for design cognition researchers, including the dangers of reverse inference (Poldrack Reference Poldrack2011) and the lack of a common ontological framework (Duffy et al. Reference Duffy, Hay, Grealy and Vuletic2019).
4.3. Approaches for studying design cognition
Current approaches can be characterized in a number of dimensions: the degree of artificiality, from controlled experiment to design in the wild; the temporal scope, from microscale to macroscale; data collection strategy, from case to experimental; and analytical strategy, from qualitative thematic to quantitative or mixed methodologies. Despite the diverse range of approaches available to design cognition researchers, there remains a significant focus on empirical protocol studies – involving the collection of verbal, video and/or sketch-based protocol data under controlled conditions, and typically some form of qualitative or mixed analysis strategy. Whilst this approach can be effective and has considerably advanced our understanding of design cognition to date, it is limiting the scope of methodological discussions in the field – for example, as highlighted by Crilly (Reference Crilly2019) in their call for more effective use of case research, and by Ball & Christensen (Reference Ball and Christensen2018) in their recent Design Studies special issue on Designing in the Wild. Further, there is little discussion of the capabilities and limitations of critical newer approaches (e.g., neuroimaging). Ultimately, few papers focus on methodology, which presents an opportunity to explore new and mixed method research designs that could potentially disrupt current design cognition understanding.
Overall, design cognition research is richly diverse in terms of both what is studied and how. This diversity simultaneously presents opportunities and challenges for future work in the area.
5. The near future
Looking forward from the state of the art outlined in Section 4, we suggest a number of growing or emerging topics that may shape research focus over the next 10–20 years. These are summarized in Figure 2, where we highlight both what and how we study design cognition.
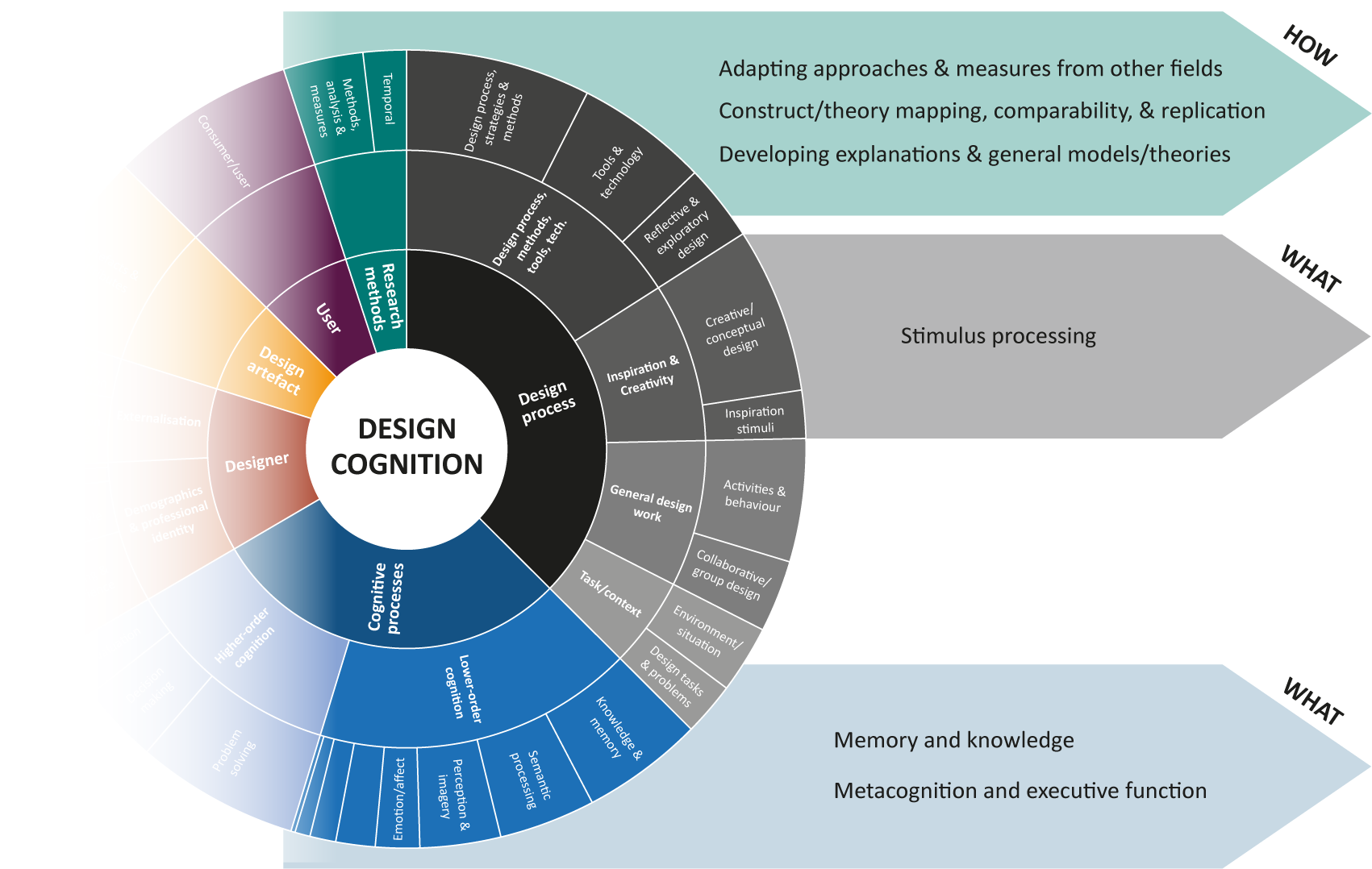
Figure 2. The near future of design cognition research – growing and emergent topics for the next 10–20 years.
5.1. Topics (what?)
In terms of the topics potentially dominating the next era of design cognition research, analysis of the literature, concept maps, and thematic collection papers point to three main foci: (i) memory and knowledge; (ii) stimulus processing; and (iii) metacognition and executive function.
5.1.1. Memory and knowledge
In cognitive science, memory processes have been shown to play a key role in creative tasks. They are an important element of dual-process models of creativity, where creative thinking involves both the spontaneous, unconscious retrieval and association of representations from memory and more deliberate, conscious evaluation and modification of ideas to meet task goals and constraints (Beaty et al. Reference Beaty, Kenett, Christensen, Rosenberg, Benedek, Chen, Fink, Qiu, Kwapil, Kane and Silvia2018; Kleinmintz et al. Reference Kleinmintz, Ivancovsky and Shamay-Tsoory2019). Memory and knowledge emerge from our literature analysis as one of the fastest growing topics in design cognition research, with growth from ~30% in 2005 to ~60% in 2017 and 2018.
Key opportunities in this area surround mapping and applying established psychological models and theories to design. For example, prominent concepts that have yet to be explored in design research include multicomponent working memory (Baddeley Reference Baddeley1983, Reference Baddeley2003), long term episodic (contextual and experiential) and semantic (conceptual) memory (Tulving Reference Tulving1983; Squire & Zola Reference Squire and Zola1998), and fuzzy trace theory (Reyna & Brainerd Reference Reyna and Brainerd1995; Corbin et al. Reference Corbin, Reyna, Weldon and Brainerd2015) These could, for instance, provide insights into major areas that are unresolved in current design research –for example, how knowledge about problems/solutions is structured and prioritized from moment to moment, how more general representations are constructed over time, and how different types of memory and knowledge are used in design tasks.
5.1.2. Stimulus processing
Considerable work has been conducted on the use of stimuli as sources of inspiration in creative design, with major growth from ~10% in 2004/2005 to ~30% in 2017/2018. This includes research on fixation (e.g., Sio et al. Reference Sio, Kotovsky and Cagan2015; Crilly & Cardoso Reference Crilly and Cardoso2017; Crilly Reference Crilly2019), the general effects of stimuli on creativity (e.g., Chan et al. Reference Chan, Fu, Schunn, Cagan, Wood and Kotovsky2011; Goucher-Lambert & Cagan Reference Goucher-Lambert and Cagan2019), and neuroimaging studies of the brain regions involved in stimulus processing during ideation (Goucher-Lambert et al. Reference Goucher-Lambert, Moss and Cagan2019). Interpreting stimuli during creative tasks involves numerous interacting cognitive processes, including perception (the processing of sensory information), semantic processing (the processing of meaning) and attention (the processes involved in selecting, focusing on and ignoring information streams). All of these processes were studied in our literature sample, but not to a great extent and generally not with consideration of the most recent psychological research in these areas. This kind of processing is not only important during designing, but also in the use of designed products. In this thematic collection, Carbon (Reference Carbon2019) calls for user-centred design approaches with a more robust grounding in scientific knowledge about how users perceive and interpret products.
Key opportunities in this area again centre on bringing constructs and models from broader cognitive science into design cognition. For instance, cognitive and neurocognitive work on visual perception and visual mental imagery (e.g., Kosslyn Reference Kosslyn, Kosslyn and Osherson1995; Bywaters et al. Reference Bywaters, Andrade and Turpin2004; Borst & Kosslyn Reference Borst and Kosslyn2010; Singh & Pande Reference Singh and Pande2012; Ganis Reference Ganis, Lacey and Lawson2013; Bergmann et al. Reference Bergmann, Genç, Kohler, Singer and Pearson2016) could provide insights on the interplay between seeing and imagining during designing. Semantic processing constructs (e.g., semantic reinterpretation) can be mapped to related design phenomena (e.g., ‘seeing as’ (Goldschmidt Reference Goldschmidt1991) and ‘unexpected discovery’ (Suwa et al. Reference Suwa, Gero and Purcell2000)), providing a basis for further investigation. We can also move beyond visual attention studies (Yu & Gero Reference Yu and Gero2018) by identifying which of the numerous attentional constructs are relevant and applicable in design – for example, see Chun et al. (Reference Chun, Golomb and Turk-Browne2011) for a review.
5.1.3. Metacognition (MC) and executive functions (EF)
Finally, MC and EF are two models of higher-order cognition involved in monitoring, understanding, and regulating one’s own thought processes and behaviours (Roebers Reference Roebers2017). This kind of processing is believed to drive task-oriented evaluation and modification in the dual-process creativity models mentioned above. Our literature analysis suggests that MC is only slowly emerging in design cognition research, and EF appears to be largely unknown, collectively accounting for only 1% of papers (Appendix Table B). However, these reflective and task-oriented processes are critical in design, particularly in exploratory design processes. The designer continually monitors what is uncertain or unknown, initiating processes and/or behaviours to address these limitations and update the problem/solution state (Cash & Kreye Reference Cash and Kreye2018). Thus, the lack of discussion on MC and EF is in stark contrast to the major growth in focus on reflective & exploratory design (Figure 1) from ~20% in 2004/2005 to ~40% in 2017/2018.
Key opportunities in this area include generating insights into the higher-order cognition that likely operates alongside basic memory processes (above) to drive problem–solution coevolution. Research in this area could also advance dual-process conceptualizations of creative design ideation – for example, building upon applications of the Geneplore model (Finke et al. Reference Finke, Ward and Smith1992), where there is an interplay between intuitive generative processes and task-oriented evaluative processes.
5.2. Methodological and theoretical challenges (how?)
In terms of how we study, a number of methodological and theoretical challenges are on the horizon, with research methods growing in importance from ~25% in 2004/2005 to ~45% in 2017/2018. Here, three areas are highlighted: (i) methodological adaption from other fields; (ii) construct/theory mapping; and (iii) theory development.
5.2.1. Adapting approaches and measures from other fields
Several approaches from cognitive science and other fields are relevant for design cognition research, including behavioural experiments, psychological tests, big data and neurophysiological techniques. In this thematic collection, for instance, Miller & Feigh (Reference Miller and Feigh2019) focus on a novel adaptation of Cognitive Work Analysis from cognitive systems engineering. There are numerous challenges involved in adapting and applying methods from outside the design domain. Designing is a temporal activity that may unfold over minutes, hours, days, weeks and months. Thus, issues of granularity are important. Further, this multilevel conceptualization leads to an understanding of design as a complex activity that likely involves multiple interacting processes, and can be highly variable between contexts (Cash & Kreye Reference Cash and Kreye2017).
Key opportunities in this area centre on answering major questions such as, How can we ensure the reliability of measurement when dealing with complex multilevel activity and cognition? and How do we separate signal from noise in emerging approaches involving large volumes of data (e.g., big data and neuroimaging)? Furthermore, numerous issues surround validity: how to minimize internal validity threats, how to ensure the validity of statistical tests, and how to overcome the trade-off that often exists between ecological validity and empirical control.
5.2.2. Construct/theory mapping, comparability and replication
Constructs in design cognition research are often not clearly defined and differentiated. It is generally unclear whether they map to those studied in psychology, and thus to what extent psychological theories can be applied to designing. Similarly, there is a lack of comparability of studies and a lack of consistency in the experimental designs and analysis methods used to study design cognition. Together, these issues make comparison and research synthesis difficult (Sio et al. Reference Sio, Kotovsky and Cagan2015; Vasconcelos & Crilly Reference Vasconcelos and Crilly2016; Hay et al. Reference Hay, Duffy, McTeague, Pidgeon, Vuletic and Grealy2017b; Cash Reference Cash2018) and impede efforts to connect to theory in other relevant domains (e.g., see the discussions of dual-process theory by Badke-Schaub & Eris (Reference Badke-Schaub and Eris2014) or Cash et al. (Reference Cash, Daalhuizen, Valgeirsdottir and Van Oorschot2019a)).
Key opportunities centre on means to facilitate the systematic adoption of methods and knowledge from other fields, including the identification and mapping of relevant constructs and theory. There is also a need for more wide-ranging methodological standards that can foster consistency without detracting from the diversity of research approaches in current literature. However, potential templates for such efforts do exist in other fields – for example, the Cognitive Atlas created by Poldrack et al. (Reference Poldrack, Kittur, Kalar, Miller, Seppa, Gil, Parker, Sabb and Bilder2011), an online knowledge base of construct definitions and measures in cognitive neuroscience, or the standards developed by Kitchenham et al. (Reference Kitchenham, Pfleeger, Pickard, Jones, Hoaglin, El Emam and Rosenberg2002) in software engineering.
5.2.3. Developing explanations and general models/theories
Finally, there is a generally acknowledged need to move beyond descriptive, exploratory studies towards general model and theory development (Dinar et al. Reference Dinar, Shah, Cagan, Leifer, Linsey, Smith and Hernandez2015; Hay et al. Reference Hay, Duffy, McTeague, Pidgeon, Vuletic and Grealy2017a; Cash Reference Cash2018). Whilst this does not mean that every study must build a full ‘theory’, there is a need to discuss what theory construction means in design cognition research, particularly because of its close link to the established body of formal cognitive theory. Here efforts have examined how to generally build knowledge (Hevner Reference Hevner2007; Blessing & Chakrabarti Reference Blessing and Chakrabarti2009; Cash Reference Cash2018), the types of knowledge contributions possible in a theory-driven paradigm (Colquitt & Zapata-Phelan Reference Colquitt and Zapata-Phelan2007), best practices for knowledge and theory development (Wacker Reference Wacker2008), and specific guidance on adapting theory from other fields (Amundson Reference Amundson1998).
Key opportunities in this area include mapping and identifying relationships between major variables, formulating and testing predictions about phenomena through repeated studies, and refining and evolving scientific formalisms. Building on the extensive findings of the rich exploratory work conducted to date, there are numerous opportunities to apply psychological models and theories to support the theory development process as outlined above.
6. The far future
Finally, our analysis provides a potential window on what the far future (next 50 years and beyond) might look like for design cognition research. Here, themes combine what and how, as illustrated in Figure 3: ecological measurement; artificial intelligence (AI); and theory construction and research maturation.

Figure 3. The far future of design cognition research – possible topics for the next 50 years and beyond.
6.1. Ecological measurement
As noted above, design is a complex and temporal activity that is fundamentally affected by context. This raises the question of whether we can develop meaningful cognitive models and theories through controlled, time-limited and artificial experiments in the lab. In this respect, there are likely to be considerable advances in sensing and measurement technology over the coming decades that could enable us to measure thousands of variables unobtrusively during designing. Prior research has already addressed steps towards for example, work sampling (Cash et al. Reference Cash, Škec and Štorga2019b; Robinson Reference Robinson2009) and smart logbooks (McAlpine et al. Reference McAlpine, Cash and Hicks2017), but researchers are currently working towards new paradigms in areas such as advanced brain–computer interface technology (Liu et al. Reference Liu, Fu, Cheng, Hong, Zhou, Jin, Duvvuri, Jiang, Kruskal, Xie, Suo, Fang and Lieber2015; Musk & Neuralink Reference Musk2019) and smart clothing (Chen et al. Reference Chen, Ma, Song, Lai and Hu2016). Using such technologies, we could obtain a rich and detailed view on design cognition as it naturally unfolds ‘in the wild’ (Ball & Christensen Reference Ball and Christensen2018).
Key opportunities could include the development of techniques for processing big data and generating feedback in real time, for both researchers and designers (e.g., ‘neurofeedback’ systems to facilitate more creative neural and cognitive processing (Gruzelier Reference Gruzelier2018)). Furthermore, it is likely that this will necessitate advanced AI techniques for data analysis. Another opportunity lies in understanding and finding ways to identify systematic biases in data and analysis, as well as other methodological confounds, the negative effects of which will be amplified as the size of datasets increases.
6.2. Artificial intelligence (AI)
AI has been studied in design since the 1980s (MacCallum Reference MacCallum1990). However, research in this area has evolved significantly since then, with AI being widely acknowledged as a key element shaping long-term development in design cognition analysis:
(i) As big data continues to develop and grow in popularity, AI will be needed to support dynamic analysis of large volumes of complex data. This is necessary if we are to implement the concept of ecological measurement as outlined above.
(ii) AI agents may be used to directly simulate cognition for study, enabling research using very large samples, and investigations of phenomena, contexts and populations that are difficult to study empirically in practice. Work in this area is already emerging. In this thematic collection, Lapp et al. (Reference Lapp, Jablokow and McComb2019) present a novel agent-based model for studying cognitive style in team problem solving, providing a foundation for more advanced simulations reflecting diversity in problem-solving styles. In the broader literature, Perišić, Štorga & Gero (Reference Perišić, Štorga and Gero2019b) apply agent-based simulation to explore the perceived novelty of design solutions, observing that perceptions can change over the design process and in different situations.
(iii) AI could support augmented humans carrying out design, and/or greater symbiosis between human designers and computer systems. Significant advances will be required in augmented/virtual reality, sensing technology and human–computer interfaces to realise this vision, as well as foundational scientific knowledge about designer behaviour and cognition (Duffy et al. Reference Duffy, Hay, Grealy and Vuletic2019).
Key opportunities here include defining possible interactions between big data, advanced AI and human–computer interaction (HCI) technologies. Advanced intelligent interfaces between human designers and technology could fundamentally change designing (Duffy et al. Reference Duffy, Hay, Grealy and Vuletic2019), leading to significant evolution in design cognition models and theories. Expanding the role of AI agents in design research (above) also leads to questions about how the performance of human and nonhuman designers compares, particularly in creative tasks. A reexamination of the roles of human and nonhuman agents in the design process may be needed, and the relationship between humans and computer-based systems may be radically redefined. It is even possible that as AI designers emerge, human cognition-based models and theories of design may need to be extended, complemented or replaced to account for nonhuman agents that function in fundamentally different ways.
6.3. Theory construction and research maturation
As discussed in Section 5, a major challenge is the development of design cognition methodology and theory. This is critical for the long-term maturation of the field as a discipline. From a methodological perspective, research is currently dominated by qualitative approaches and we have yet to see widespread application of the quantitative approaches used for hypothesis testing and generalization in other scientific domains. Perhaps more fundamentally, design cognition research does not have a holistic and systematic approach to the theory building cycle that underpins most mature scientific fields (Cash Reference Cash2018). Design cognition research often focuses on description, without progressing to theory testing and refinement. Sosa’s paper (Reference Sosa2019) in this thematic collection presents an effort to more robustly characterize the variables and relationships involved in design ideation, linking to theories in other fields and discussing the steps required to progress through the next phase of the theory development cycle. If these methodological and theoretical issues are not addressed, the field will be ill-prepared for using complex big data and machine learning/AI analytics. Collecting and analysing large amounts of complex data will not advance the field unless we have a rigorous framework for interpreting and using it to evolve theory.
A key opportunity here is the development of a mature scientific paradigm for design cognition research, focusing on the diversity, complexity and dynamic evolution that is characteristic of the field and often absent in more narrowly-focused disciplines. Further, the development of more robust processes for building and testing design cognition theory could encourage its application beyond our field, and thus broaden the scientific influence and contribution of our community.
7. A roadmap for design cognition analysis
In Sections 4–6, we have outlined a view on the state of the art, near future (10–20 years), and possible far future (50 years and beyond) of design cognition analysis. We have examined current, emerging and envisioned topics regarding both what and how we study, and highlighted key opportunities for developing and advancing the field. These insights are summarized in Table 1 and Figure 4, which provides design researchers with a proposed roadmap for the development of the field, as well as critical research questions to be answered in each area.
Table 1. A design cognition research road map from current foci to the far future, highlighting topics and example research questions

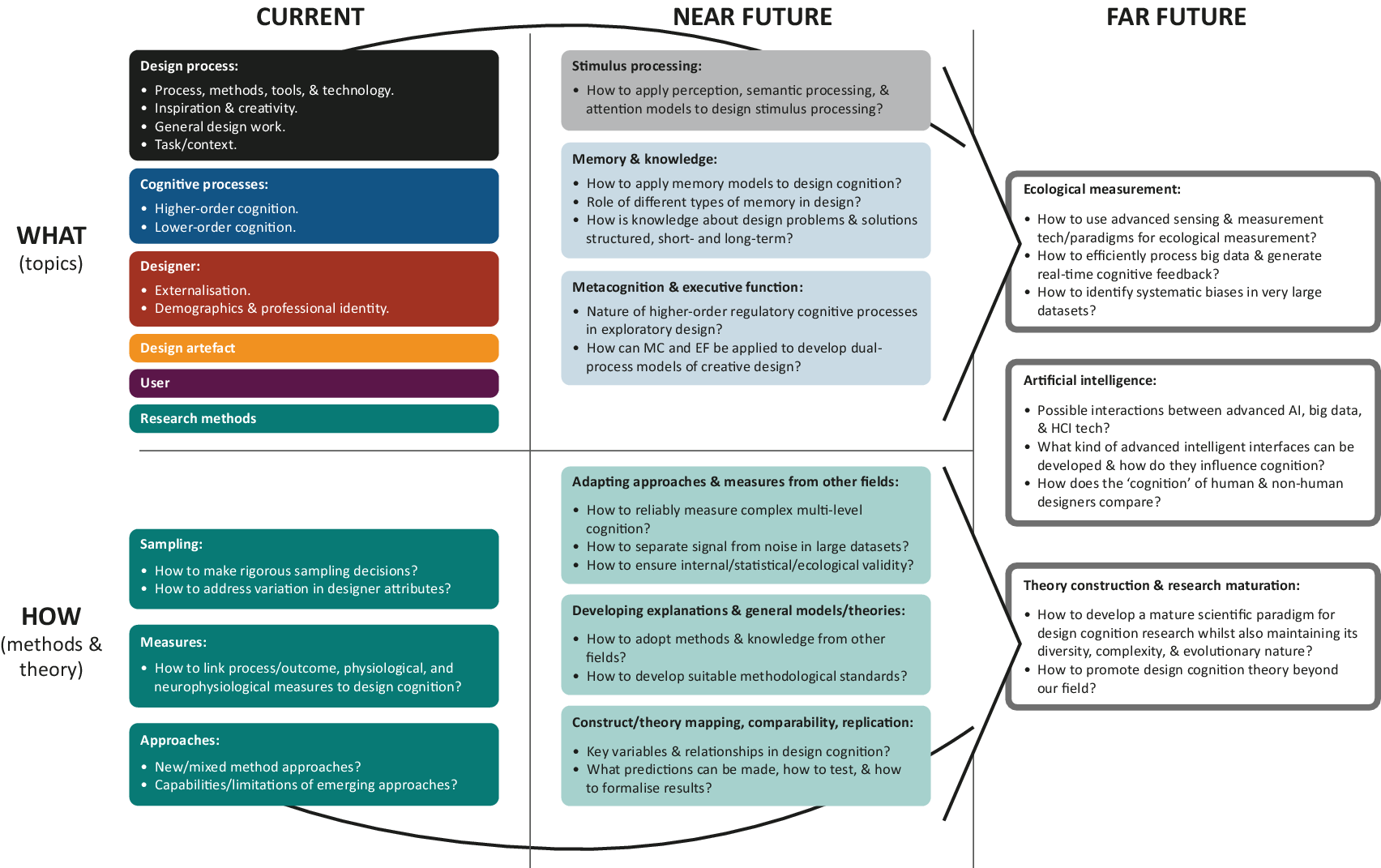
Figure 4. Visualization of the proposed design cognition roadmap (Table 1).
8. Conclusion
The analysis of design cognition, that is, the mental processes and representations involved in design, is now a major research area spanning multiple domains. Design cognition research informs understanding of the designer and design team, the design process, the evolving design artefact, and the artefact’s users. Recent years have seen growing acknowledgment that significant change is needed to overcome stagnation in design cognition topics and methodologies. Realising this change requires us to understand the history and current status of the field, as well as open discussions on its possible near- and long-term future. This has been the focus of this thematic collection and editorial.
The papers in the thematic collection cover varied topics, including theory development (Sosa Reference Sosa2018), new research approaches (Lapp et al. Reference Lapp, Jablokow and McComb2019), novel applications of known methods (Miller & Feigh Reference Miller and Feigh2019), measures (Gero & Milovanovic Reference Gero and Milovanovic2020), and the application of cognitive theory to product design practice (Carbon Reference Carbon2019). However, we have sought to do more in this editorial than simply summarize these papers. In the spirit of the collection, we have attempted to challenge the status quo, examining key issues regarding both what is investigated in design cognition and how research is conducted. Our examination was informed by a structured review of major design journals over the past 15 years, as well as the papers in the collection and the views of a broad cross-section of the design cognition community. Through this analysis, we have provided insights into the current state of the art, near future (10–20 years), and far future (50 years and beyond) of the field, highlighting salient directions and key research opportunities, which we bring together in a proposed research roadmap (Table 1, Figure 4). Whilst we make no claims regarding the completeness of this roadmap, we hope that it provides the foundation for a shared future agenda and a basis for continued discussion and debate.
It has become clear to us during the development of this collection that whilst major evolution is required in topics, methodologies, and scientific paradigm, design cognition research has positive and unique characteristics that differentiate it from other fields concerned with human cognition. These include methodological diversity, a focus on complexity and temporal dynamics, and a propensity for evolution and change. Preserving these characteristics while we address the challenges facing us is key to the maturation and flourishing of our community as a scientific field. We would like to thank all of the authors in the collection, as well as the design cognition researchers we have more broadly engaged with, for contributing their work and viewpoints to this vision for the future of design cognition analysis.
Data availability statement
The workshop data underpinning this paper are openly available from the University of Strathclyde KnowledgeBase at https://doi.org/10.15129/02b36f5c-f51f-4be4-9219-0d799ba9fb03.
Acknowledgement
The concept mapping workshop (‘Measuring design cognition’) at the 8th International Conference on Design Computing and Cognition 2018 was supported by the UK’s Engineering and Physical Sciences Research Council, grant number EP/M012123/1. We are grateful to the numerous colleagues and researchers who have contributed to the views presented in this paper. In particular, we would like to thank our co-chair at the above workshop, Alex Duffy, and the programme committee: Jonathan Cagan, Carlos Cardoso, Yukari Nagai, and Maria Adriana Neroni.
Appendix
Table A. Researchers contributing to the concept mapping study

Table B. Full list of topics with coverage and percentage mentions between 2004 and 2019
