Some people say they vote with their gut. Other people say that they follow their heart. These metaphors are meant to reveal the affective nature of political decision making. This affective nature is also central to key political decision-making models such as motivated reasoning (Lodge and Taber Reference Lodge and Taber2013), affective intelligence (Marcus, Neuman, and MacKeun Reference Marcus, Neuman and Mackuen2000), receive-accept-sample (Zaller Reference Zaller1992), and directional voting (Rabinowitz and Macdonald Reference Rabinowitz and Macdonald1989). These very different models link affect—or allied terms such as feelings or emotions—to biases in information processing, which in turn motivate different attitudinal and/or behavioral outcomes. However, affect remains underdeveloped in these models. We observe that researchers (a) only theorize about affect while admitting that they “lack direct measures of somatic response” (Lodge and Taber Reference Lodge and Taber2013, 208), (b) study only one dimension of core affect, specifically, arousal (Mutz Reference Mutz2007; Mutz and Reeves Reference Mutz and Reeves2005; Soroka, Fournier, and Nir Reference Soroka, Fournier and Nir2019; Soroka and McAdams Reference Soroka and Mcadams2015), or (c) study the cognitive corollaries of affect, such as discrete emotions like anger and anxiety (Aarøe Reference Aarøe2011; Delton, Petersen, and Robertson Reference Delton, Petersen and Robertson2018; Huddy, Mason, and Aarøe Reference Huddy, Mason and Aarøe2015; Klar Reference Klar2013; Marcus, Neuman, and MacKeun Reference Marcus, Neuman and Mackuen2000).
We tackle these issues by establishing the circumplex model in political science as a theory of core affect. This theory distinguishes between two dimensions: the intensity of affective responses—arousal—and the direction of affective responses—valence (Russell Reference Russell1980). This enables us to theorize about the structure of affective responses to political rhetoric and to examine this empirically by using physiological measures. We capture changes in arousal using skin conductance and are the first in the discipline to capture valence in response to political rhetoric by means of facial electromyography (EMG). In addition, we assess attention with heart rate variability.
Why study affective responses? According to the “hot cognition hypothesis,” all sociopolitical concepts are affectively charged in the brain (Abelson Reference Abelson, Tomkins and Messick1963). Political issues—but also leaders and parties—are affectively tagged in our long-term memory. This means that when we retrieve information about an issue from our memory, positive or negative affect is activated automatically. This colors subsequent cognitive evaluations of the situation (Lodge and Taber Reference Lodge and Taber2013), and it may motivate expressions of specific discrete emotions such as anger, anxiety, or enthusiasm (Brader Reference Brader2006).
Existing theories predict variation in affective responses to political rhetoric but assess such affective responses only indirectly. We assess three possible sources of variation in affective responses and the consequences of these affective responses to political rhetoric. First, people with more political knowledge have stronger affective links to political objects (Lodge and Taber Reference Lodge and Taber2005) and might, therefore, experience more arousal when exposed to political rhetoric. Second, according to the directional model of voting, the more extreme an attitude is, the more intense the arousal attached to the attitude will be (Rabinowitz and Macdonald Reference Rabinowitz and Macdonald1989). Third, from the motivated reasoning framework we derive that people experience negative affect in response to incongruent information and positive affect in response to congruent information (Lodge and Taber Reference Lodge and Taber2013; Suhay and Erisen Reference Suhay and Erisen2018; Taber and Lodge Reference Taber and Lodge2006).
To investigate the variation in affective responses to political rhetoric, we report an unusually large lab experiment (N = 397) with physiological measures. To maximize variation on our independent variables of interest—political knowledge, attitude extremity, and attitude position—our experiment was conducted at a university lab and at several other public locations. Participants were randomly exposed to short video clips on with left-wing or right-wing rhetoric on different political issues.
We find that people with extreme prior attitudes on an issue are more aroused than people with less extreme attitudes. We find no statistically significant association between political knowledge and arousal. Also, we report that incongruent rhetoric produces negative affect, whereas congruent rhetoric does not produce positive affect. Finally, we present evidence that arousal predicts issue position change, independent of discrete emotions.
In the conclusion, we set out to integrate our findings regarding the affective component of emotions within the framework that studies the cognitive component of emotions—that is, discrete emotions. We outline a research agenda to theorize the interplay between these different components of emotions and their consequences for politics.
What Are Affective Responses to Politics?
Affect is a part of the larger concept of emotion. One can define emotions as “episodic, relatively short-term, biologically based patterns of perception, experience, physiology, action, and communication that occur in response to specific physical and social challenges and opportunities” (Keltner and Gross Reference Keltner and Gross1999, 468). Affective responses are the immediate physiological responses to stimuli. These responses are uncontrollable and occur between 50 and 100 milliseconds after receiving a stimulus. This is prior to cognitive evaluations, which commence after 500 milliseconds (Lodge and Taber Reference Lodge and Taber2013).
We define affective responses following the circumplex model of affect (Russell Reference Russell1980). This distinguishes between two affective dimensions: arousal and valence. Arousal captures the intensity of affect and is measured with skin conductance. Valence captures the direction (positive or negative) of affect and is measured with facial EMG. These measures are rare in political science. Some studies analyze differences between liberals and conservatives in arousal to negative stimuli (Arceneaux, Dunaway, and Soroka Reference Arceneaux, Dunaway and Soroka2018; Oxley et al. Reference Oxley, Smith, Alford, Hibbing, Miller, Scalora, Hatemi and Hibbing2008). In these studies, arousal is an independent variable and considered a trait (for a critique of this approach, see Bakker et al. Reference Bakker, Schumacher, Gothreau and Arceneaux2020). Our paper is more in line with work that studies arousal as a response to political communication (Mutz Reference Mutz2007; Mutz and Reeves Reference Mutz and Reeves2005; Soroka, Fournier, and Nir Reference Soroka, Fournier and Nir2019; Soroka and McAdams, Reference Soroka and Mcadams2015).
We move beyond arousal by turning to valence as well. This dimension of affect is largely absent in political science. This is remarkable because there are various canonical theories within the field that hint that political rhetoric can evoke both positive and negative affect (Soroka Reference Soroka2014; Taber and Lodge Reference Taber and Lodge2006). The core contribution of our study is that by introducing the circumplex model of affect we can theorize about arousal and valence and at the same time offer ways to measure this by using facial EMG.
How do affective responses align with the more cognitive discrete emotions? This is a big unsettled question in neuroscience (Barrett and Satpute Reference Barrett and Satpute2019; LeDoux and Pine Reference Ledoux and Pine2016). Subjective appraisals of affect are typically, but not always, translated into concrete discrete emotions such as anxiety, anger, or enthusiasm. Affect—sometimes labeled “core affect”—is thus causally prior to the appraisal of (discrete) emotions (Barrett and Satpute Reference Barrett and Satpute2019), and it requires a different methodology to measure. This means that our work is different from seminal work in political science research on emotions, which uses self-reported discrete emotions (e.g., Aarøe Reference Aarøe2011; Delton, Petersen, and Robertson Reference Delton, Petersen and Robertson2018; Huddy, Mason, and Aarøe Reference Huddy, Mason and Aarøe2015; Klar Reference Klar2013; Marcus, Neuman, and MacKeun Reference Marcus, Neuman and Mackuen2000).
Self-reported emotions can align with affect (Bradley et al. Reference Bradley, Codispoti, Cuthbert and Lang2001). Within the circumplex model, anger is, for instance, seen as a discrete emotion that is characterized by high arousal and negative valence (Russell, Reference Russell1980). Yet the correlation between (physiological) affect and self-reported emotions is typically very low (LeDoux and Pine Reference Ledoux and Pine2016). This might be because they are produced by different brain systems. That said, physiology and cognitive-emotional responses are both part of the broader concept of emotion. It is not wrong to study one or the other; they are simply different, and they may have different consequences. We investigate these consequences in this paper by also analyzing whether self-reported discrete emotions and physiological responses can explain attitude change. In addition to that, in our conclusion we set out a research agenda that can further explore the relevance of studying emotions as both unconscious, physiological responses and conscious, self-reported emotions. Before we continue to specify our expectations about physiological affective responses, below we discuss each measure in more detail.
Arousal
Skin conductance captures activity of the sympathetic nervous system (Dawson, Schell, and Filion Reference Dawson, Schell, Filion, Cacioppo, Tassinary and Berntson2007). It measures the varying electrical properties of the skin in response to the increase of sweat secretion in the eccrine glands. With more sweat secretion, the conductance of electricity improves and skin conductance levels rise. This is interpreted as an increase in arousal. Increases in skin conductance have been reported in response to negative images (Lang et al. Reference Lang, Greenwald, Bradley and Hamm1993), negative news (Soroka, Fournier, and Nir Reference Soroka, Fournier and Nir2019; Soroka and McAdams Reference Soroka and Mcadams2015), and negative political ads (Daignault, Soroka, and Giasson Reference Daignault, Soroka and Giasson2013; Wang, Morey, and Srivastava Reference Wang, Morey and Srivastava2014), but such increases are also observed in response to positive stimuli such as a exposure to a preferred football team (Potter and Keene Reference Potter and Keene2012), political party (Petersen, Giessing, and Nielsen Reference Petersen, Giessing and Nielsen2015), or politician (Wagner et al. Reference Wagner, Deppe, Jacobs, Friesen, Smith and Hibbing2014).
Valence
The valence of affect can be measured by recording electromyographic (EMG) signals of specific muscles in the face (Tassinary, Cacioppo, and Vanman Reference Tassinary, Cacioppo, Vanman, Cacioppo, Tassinary and Berntson2007). To disentangle the experience of negative and positive affect, the activity of the corrugator and zygomaticus muscle groups, respectively, are recorded (Tassinary, Cacioppo, and Vanman Reference Tassinary, Cacioppo, Vanman, Cacioppo, Tassinary and Berntson2007). The corrugator ciculii is a muscle above the eyebrow that draws the brow down and pulls the brows together. Corrugator activity has been recorded in response to negative images (Lang et al. Reference Lang, Greenwald, Bradley and Hamm1993), negative words (Wexler et al. Reference Wexler, Warrenburg, Schwartz and Janer1992), and negative affective cues in language (Hietanen, Surakka, and Linnankoski Reference Hietanen, Surakka and Linnankoski1998). The zygomaticus major pulls the corners of the mouth up and back into a smile (Larsen, Norris, and Cacioppo Reference Larsen, Norris and Cacioppo2003). Zygomaticus activity increases in response to positively valenced images (Van Oyen Witvliet, and Vrana Reference Van Oyen Witvliet and Vrana1995) and videos (Cacioppo et al. Reference Cacioppo, Petty, Losch and Kim1986).
Attention
A fourth physiological measure that is sometimes used is heart rate variability. In some cases it is used to measure arousal, and in other cases it is used to measure (cognitive) attention (Lang, Newhagen, and Reeves Reference Lang, Newhagen and Reeves1996; Soroka, Fournier, and Nir Reference Soroka, Fournier and Nir2019; Soroka and McAdams Reference Soroka and Mcadams2015). However, any acceleration in heart rate that comes from arousal will be overwhelmed by the deceleration that comes with attention (Potter and Bolls Reference Potter and Bolls2012). We follow Soroka and colleagues (Soroka, Fournier, and Nir Reference Soroka, Fournier and Nir2019; Soroka and McAdams Reference Soroka and Mcadams2015) and employ heart rate variability as a measure of attention.
Who Have Stronger Affective Responses to Politics?
It seems unlikely that all political issues are equally “hot” for all citizens. Brader (Reference Brader2006, 187) pointed out that the next generation of studies “must consider” how “individual variation” conditions the extent to which people experience affect towards politics. As such, we move this literature one step further and distinguish three sources of variation: knowledge, attitude extremity, and (in)congruence with political rhetoric.
According to the “hot cognition hypothesis,” all sociopolitical concepts are affectively charged in the brain (Abelson Reference Abelson, Tomkins and Messick1963). In our long-term memory we have stored associations between an object and a good, bad, or ambivalent evaluation. Leaders, parties, and even issues can be such objects, and when exposed to them, we quickly and unconsciously retrieve the relevant affective tags from memory (Lodge and Taber Reference Lodge and Taber2013). Experimental priming tasks indeed demonstrate the affective nature of the retrieval of political objects from long-term memory (Erisen, Lodge, and Taber Reference Erisen, Lodge and Taber2014; Lodge and Taber Reference Lodge and Taber2005). Yet, people differ in the chronic accessibility of evaluations (Lau Reference Lau1989) because the strength of the associations between objects and their evaluation differs. Repeated evaluations are likely to strengthen these associations; therefore, they make affective tags more chronically accessible. People with more political knowledge are more likely to have “thought about and repeatedly evaluated” political concepts than are people with little to no political knowledge (Lodge and Taber Reference Lodge and Taber2005, 471). The more knowledgeable “have formed crystallized attitudes to a fuller set of political issues” because of their “interest in politics” (Lodge and Taber Reference Lodge and Taber2005, 473). Therefore, it is plausible that people with more political knowledge have formed stronger affective links to political objects (Lodge and Taber Reference Lodge and Taber2005). Indeed, more politically sophisticated citizens self-report stronger discrete emotions towards politicians in surveys (Brader Reference Brader2006). Hence, for people with high levels of political knowledge, political rhetoric is more likely to be arousing because the concepts activated by this rhetoric are more accessible than is the case with people with little political knowledge. This leads us to expect that the more political knowledge someone has, the more s/he experiences arousal in response to political rhetoric (H1).
A second factor that might evoke affective responses is attitude extremity. The theory of directional voting (Rabinowitz and Macdonald Reference Rabinowitz and Macdonald1989) attributes a central role to affect because political issues are affectively charged. Thus, “for issues (or other political cues) to have impact, they must evoke emotions” (Rabinowitz and Macdonald Reference Rabinowitz and Macdonald1989, 94). Because people differ in their attitudes to these issues, the intensity of affective responses will differ per individual. Neutral (indifferent) attitudes are cold (no arousal), while the more extreme an attitude becomes, the more intense the affect (arousal) attached to the attitude. Such thinking is in line with the motivated reasoning framework. Stronger (more extreme) attitudes on an issue produce stronger biases in information processing (Lodge and Taber Reference Lodge and Taber2013). Affective primes also have stronger effects on people with stronger attitudes on an issue (Lodge and Taber Reference Lodge and Taber2005). The underlying rationale is similar to that of our previous hypothesis concerning political knowledge. People with strong attitudes are more invested in an issue, and they have given it more thought. Therefore, these concepts are more chronically accessible. Also, the affective tags that are activated are more likely to be exclusively positive or negative for people with strong opinions on an issue. In sum, we expect that the more extreme someone’s attitude on an issue is, the more s/he experiences arousal in response to political rhetoric on that issue (H2).
A third possibility is that not issue extremity but the extent to which someone’s issue position is congruent with the political message s/he is exposed to conditions affective responses. Here we move from a focus on “arousal” to “valence” because Taber and Lodge (Reference Taber and Lodge2006) theorized that citizens respond with negative affect to information they disagree with. As a reaction, counterarguments are formulated to reject the attitudinally incongruent message and to reduce the negative affect (Taber and Lodge Reference Taber and Lodge2006). There is some indirect evidence that people indeed experience affect in response to incongruent messages. Using a priming paradigm, Lodge and Taber (Reference Lodge and Taber2005) show that participants produced faster evaluations when the prime was congruent with the target word than when the prime was incongruent with the target word. For a participant identifying as a Democrat, a congruent prime is, for example, Clinton combined with a positive word. An incongruent prime would be Clinton combined with a negative word. These studies led Lodge and Taber (Reference Lodge and Taber2005, 467) to conclude that affect is “triggered automatically on the mere presentation of the concept.” Along these lines, preliminary work in neuroscience suggests that when a person disagrees with a message, a strong brain response is evoked (Morris et al. Reference Morris, Squires, Taber and Lodge2003) and brain regions associated with the experience of negative affect appear to be more active (Kaplan, Gimbel, and Harris Reference Kaplan, Gimbel and Harris2016). We therefore expect that the more incongruent someone’s attitude is with the rhetoric s/he is exposed to, the more s/he experiences negative affect (H3). Rhetoric can, of course, also be congruent with prior attitudes. If this is the case, we expect that the more congruent someone’s attitude is with the rhetoric s/he is exposed to, the more s/he experiences positive affect (H4). Note that we do not formulate directional expectations about the relationship between incongruence and arousal or congruence and arousal because exposure to incongruent messages and congruent messages could be equally arousing.
Following the argument that incongruent rhetoric motivates people to look for counter arguments (Lodge and Taber Reference Lodge and Taber2013), we also expect that the more incongruent someone’s attitude is with the rhetoric s/he is exposed to, the more attentive s/he will be (H5). As discussed in the previous section, heart rate variability is a physiological measure of attention, which we use in our study.
Finally, we ask the question of whether affect is associated with subsequent opinion change. Taber and Lodge (Reference Taber and Lodge2006) demonstrated that people with extreme attitudes and high knowledge were not only reasoning in biased ways, their attitudes also polarized at the end of the study. We have theorized that such biased reasoning is triggered by affect. This raises the question of whether affective responses then also lead to attitude changes. There is, to our best knowledge, no earlier work that suggests how exactly affect and attitude change would be connected. Increased affect could lead to changes in attitudes in different directions: one could move either towards or away from the message one receives. Hence, although we expect that increased affect in response to political rhetoric is associated with attitudinal changes, we do not have clear expectations regarding the specific direction of such changes.
Research Design
Sample
Our study was conducted at multiple locations in the Netherlands between August 2016 and June 2017. We used two different protocols. Table 1 describes the locations, specifies the protocols used, and notes the number of participants collected at each location. In all we have collected data for 397 Dutch adults. No statistical methods were used to predetermine sample size, but our sample size is more than three times larger than the median sample size reported in previous publications (e.g., Arceneaux, Dunaway, and Soroka Reference Arceneaux, Dunaway and Soroka2018; Mutz Reference Mutz2007; Mutz and Reeves Reference Mutz and Reeves2005; Renshon, Lee, and Tingley Reference Renshon, Lee and Tingley2015; Soroka, Fournier, and Nir Reference Soroka, Fournier and Nir2019; Soroka and McAdams Reference Soroka and Mcadams2015)—see Appendix A.1 for more details. Our study thus has an unusually large sample size for a study of its kind.
Table 1. Description of the Locations
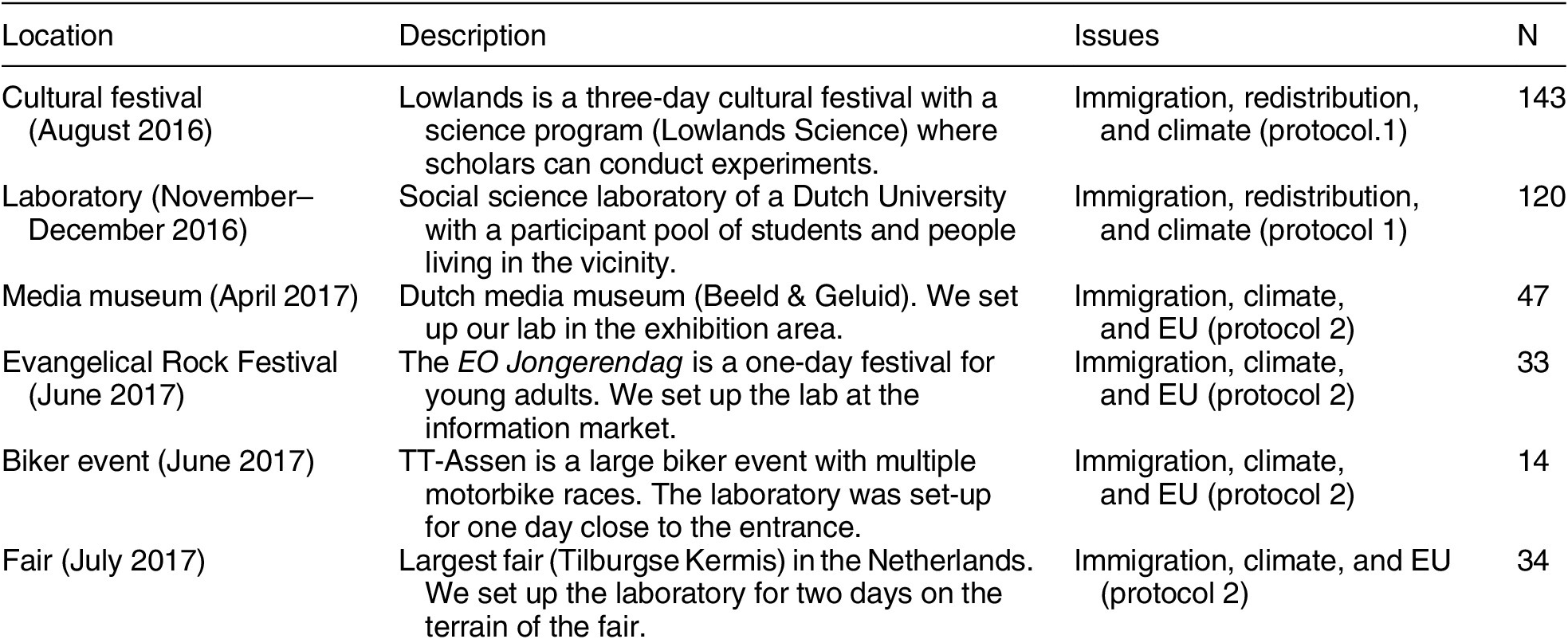
To obtain sufficient variation on the theoretically relevant independent variables (e.g., political knowledge and political attitudes), we set up our lab at different lab-in-the-field locations. In Appendix A.4, we demonstrate that we have succeeded in obtaining sufficient variance on our key independent variables.
Design of the Study
Upon signing the informed consent form, participants completed a survey on a desktop computer (laboratory) or iPad 2 (lab-in-the-field). We asked about their political attitudes and socioeconomic background (see Appendix A.2 for details on rewards and procedures during the survey as well as item wording of the survey). Next, participants were connected to physiological measurement equipment by trained research assistants. Participants were also given noise-canceling headphones (Bose). The experiment started with a baseline measure of 30 seconds (Soroka and McAdams Reference Soroka and Mcadams2015). Afterwards, participants were randomly assigned to a treatment in the form of a video clip (between 50 and 60 seconds) about a specific political issue—namely, immigration (protocol 1 and 2)Footnote 1, climate change (protocol 1), redistribution (protocol 1), and the European Union (protocol 2)—see Table 1 for an overview.Footnote 2 We chose these issues because they differ in their salience, with immigration the most salient issue at the time of the study and climate, redistribution, and Europe considerably less salient (den Ridder et al. Reference Den Ridder, Dekker, Van Houwelingen and Schrijver2016).
The video clips were created by a professional editor, using a selection of clips from news broadcasts that illustrated these issues, without showing politicians, political parties, or other prominent persons. Per issue, we constructed two treatments: a “left-wing” message (liberal) and a “right-wing” message (conservative). To do this, a professional speech actor recorded a voice-over loosely based on real speeches from left-wing or right-wing parliamentarians. By using real speeches, we can guarantee a degree of external validity, without mentioning the source to the participant, which could bias our treatment effects. Table 2 provides snippets of all messages (see Appendix A.6 for transcripts, links to the videos, and other details of the treatments). In a pretest (N = 23), we confirmed the ideological slant of the messages (Appendix A.6).
Table 2. Treatments: Snippets of the Message

Note: Complete text of treatments and links to videos can be found in Appendix A.6.
We employ block-randomization whereby the order of the four issues was randomized and for each issue participants were randomly exposed to left-wing or right-wing rhetoric. This means that participants saw a total of four clips. The interstimulus intervals between the different messages were roughly 30–60 seconds, during which participants answered a set of filler questions. Note that we refrain from treating these periods as interstimulus intervals in the analyses because participants also answered questions during this period. As a consequence, their hands moved a lot, causing all sorts of unknown distortions in the measure of the physiological responses.
Survey Measures
In the survey before the treatment, we measured attitudes towards four issues: (1) immigration (three items, e.g., “The Dutch culture is threatened by refugees” [reverse coded]), (2) redistribution (two items, e.g., “Income inequality is too big in the Netherlands. People with the lowest incomes should get the biggest salary increase”), (3) the EU (three items, e.g., “I am in favor of more decision-making at the European level”), and (4) climate (three items, e.g., “There are more important things in life than protecting the environment” [reverse coded]). All variables were measured on a 9-point scale ranging from “totally disagree” (1) to “totally agree” (9)—see Appendix A.7 for item wording. Descriptive statistics for these and all other measures in this study are provided in Appendix A.3. After each treatment, respondents were asked again to fill out these questions. For our final analysis of the consequences of affective responses, we use the absolute difference between the pretreatment and posttreatment measure as the dependent variable. Attitude extremity on an issue was measured by taking the absolute distance between the participant’s placement on the scale from the scale’s middle point. We measured political knowledge using three multiple choice items, such as “How long is one term for a member of the Lower House” (correct answer = 4 years; see Appendix A.7 for other items and psychometric properties). We created an index by summing the correct answers.
For all four issues, we created an index of incongruence by contrasting the pretreatment attitudes with the video clip participants received. Consider immigration attitudes. A score from >0 to +4 means that the video clip participants received was incongruent with their immigration attitudes (left-wing attitude with right-wing clip or right-wing attitude with left-wing clip). The higher the score, the more incongruent the clip was with the attitude. Scores <0 to -4 indicate congruence between the video clip and the attitude (left-wing attitude and left-wing clip, etc.). Again, we assume that the message is more congruent for participants with more extreme attitudes. A score of “0” indicates that participants have a neutral immigration attitude, and so neither the left-wing nor the right-wing video clip is incongruent with their attitude.
Physiological Measures
We recorded physiological data using the Versatile Stimulus Response Registration Program 1998 (Vsrrp98) software on laptops (lab-in-the-field data collection) or stationary computers running Windows 7 (laboratory data collection). We assess arousal using skin conductance levels (SCL). These are measured by passing a small current through two electrodes placed on the skin (see panel A of Figure 1). By keeping the current constant, it is possible to measure the flow of the current—what we call skin conductance expressed in micro-Siemens (Dawson, Schell, and Filion Reference Dawson, Schell, Filion, Cacioppo, Tassinary and Berntson2007). For a description of the measure, see Appendix A.8.

Figure 1. Physiological Measures: SCL and EMG
We constructed our measure of change in skin conductance response
$$ (\Delta \mathrm{SC}{L}_i) $$
by taking the difference between the logged mean SCL recorded while participants were exposed to treatment T and the logged mean SCL recorded during the baseline. To minimize the effects of extreme values, we follow Oxley et al. (Reference Oxley, Smith, Alford, Hibbing, Miller, Scalora, Hatemi and Hibbing2008) and take the average of the natural log of SCL during the image and the ISI (Equation 1 summarizes this approach). As such, we create a change-in-skin-conductance-level variable whereby higher values indicate that skin conductance levels increase during the exposure to the message.

The valence of emotions is measured using a facial electromyagram (EMG) (Tassinary, Cacioppo, and Vanman Reference Tassinary, Cacioppo, Vanman, Cacioppo, Tassinary and Berntson2007; see Appendix A.10 for more details).Footnote 3 To measure negative affect, we measure the activity of the corrugator supercilii muscle—above the eyebrow (see panel B of Figure 1). The corrugator has no overlapping muscle groups and has a very limited representation in the motor cortex and “tends to be bilateral innervated” (Larsen, Norris, and Cacioppo Reference Larsen, Norris and Cacioppo2003, 776–777). The measurement of the corrugator is therefore less subject to disruptions from the (voluntary) movement of other muscles.
To measure positive affect, we measure the activity of the zygomaticus major (Larsen, Norris, and Cacioppo Reference Larsen, Norris and Cacioppo2003). It is a difficult muscle to measure because it has greater contralateral innervation (Larsen, Norris, and Cacioppo Reference Larsen, Norris and Cacioppo2003), and the cheek—where the zygomaticus is located (see panel B of Figure 1)—is a particularly crowded area of the face with lots of muscles (Tassinary, Cacioppo, and Vanman Reference Tassinary, Cacioppo, Vanman, Cacioppo, Tassinary and Berntson2007). This makes measures of the zygomaticus susceptible to “cross talk” (Larsen, Norris, and Cacioppo Reference Larsen, Norris and Cacioppo2003, 777). Irrespective of the difficulties of measuring the zygomaticus, it is a unique measure to capture positive affect. Zygomaticus activity was only recorded in protocol 1 and not in protocol 2.
People do not constantly frown or laugh, at least not in response to the type of political messages we used during the experiment. The object of interest of EMG analyses is whether people frowned or laughed. To evaluate this, we first cleaned the data as follows: we extracted EMG per second and calculated the change between t and t − 1. There was a time trend in that data. We extracted the time trend by regressing change on time. The subsequent model predictions were subtracted from the transformed data. We subsequently decided on a cutoff value of 10 microvolts as a peak. We kept all increases of 10 microvolts and larger, and for each individual we summed these peaks and logged it to procure a normally distributed variable. We have experimented with several alternatives: using individual cutoff values based on within-individual deviations, sums of all changes, and the number of peaks. In most cases, correlations between the 10-microvolt cutoff point and the other variables were high, and the alternative operationalizations led to similar regression output (see Appendix C.2).Footnote 4
Like all other physiological measures, heart rate variability was registered with the VSRRP98 program (see Appendix A.9). The program contains an algorithm to extract peaks from the raw heartbeat data. We manually checked and corrected the algorithm where necessary. The program also calculates the root mean square of successive differences (RMSSD), which is a standard measure of heart rate variability—that is also used by Soroka, Fournier, and Nir (Reference Soroka, Fournier and Nir2019)—and is based on the standard deviation of the interbeat intervals (Blascovich et al. Reference Blascovich, Vanman, Mendes and Dickerson2014). As we do for skin conductance, we compare the mean heart rate variability during exposure to the message with the baseline. A positive value indicates an increase in heart rate variability compared with the baseline, while a negative value indicates a decrease in heart rate variability.
All physiological measures were visually inspected by the researchers as well as a student assistant blind to the expectations of our study. We cross-checked anomalies with the lab log. In the case of EMG, sometimes electrodes fell off during the experiment, and these data points were removed. In the case of heart rate there were some anomalies due to movement of the participant or improper placement of the measurement equipment. We also removed these data points. As a consequence, the number of observations will differ per analysis of physiological measures.
In a subset of protocol 1—during the cultural festival (see Table 1)—we asked respondents (N = 143) to self-report their levels of enthusiasm, anger, and anxiety on a 0–100 thermometer in response to the immigration treatment.Footnote 5 In this subset of our data, these measures do not correlate with the physiological measures.Footnote 6 We use these self-reported emotions in the final exploratory analysis concerning the effects of physiological responses (see Appendix A.11 for all correlations between physiology and self-reported emotions).
Method of Analysis
To evaluate our hypotheses, we pool the data across the different clips (N = 961 for arousal, N = 1,032 for negative affect, N = 1,012 for heart rate variability, and N = 429 for positive affect). We pool because of power reasons. Similar to earlier psychophysiological studies (e.g., Mutz Reference Mutz2007; Mutz and Reeves Reference Mutz and Reeves2005; Soroka, Fournier, and Nir Reference Soroka, Fournier and Nir2019; Soroka and McAdams Reference Soroka and Mcadams2015), we expected small-sized effects. A power analysis conducted after the data was collected and based on the regression procedure described below indicates that our achieved power in the pooled analysis of corrugator activity is 0.96. However, once we subset to specific issues, specific clips, or on independent variables, power drops dramatically to values below 0.8 (see Appendix A.1 for calculations). Therefore, we are reluctant to disaggregate our analyses to the individual clips or other subsets of the data.
For Hypotheses 1 and 2, we run one OLS regression analysis: our dependent variable is change in skin conductance and our main independent variables are attitude extremity and political knowledge. In this analysis, we control for the computer the respondent used, the temperature during the experiment (Venables and Mitchell Reference Venables and Mitchell1996), the order in which the treatment was received, the salience of the issue (for the respondent), gender, age, educational level, the percentage level of alcohol in blood, and dummy variables to pick up differences between treatments. We provide detailed information about each of the covariates in Appendix A.7. By using these covariates, we pick up any differences in the protocols as well as possible distortions due to the time and place of the specific data collection. We cluster our standard errors by individual to account for the interdependence in our observations. Formula 2 is used to evaluate hypotheses 1 and 2, with k reflecting the issue and Right-wing reflecting whether participant i was assigned to the right-wing treatment on issue k (1) or the left-wing treatment on issue k (0).Footnote 7

For the hypotheses 3–5, we add the incongruence variable and omit the treatment direction variable. This leads to formula 3. We use the change in corrugator activity, the zygomaticus activity, and heart-rate variability (RMSSD) as dependent variables.

For the purposes of interpretation we have standardized all variables, except for the dichotomous variables.
Results
To get a feel for the data, we have first plotted the means and 95% confidence intervals of the four physiological measures per clip (see Figure 2). There is considerable variation across issues regarding the level of arousal (see upper left panel). In particular, the Immigration (protocol 1) and the left-wing Climate clips stand out as having lower than baseline levels of arousal. This means that fewer people were aroused when exposed to these clips. Specifically, about 70% of the participants were aroused by the Immigration protocol 2 clips, and only 50% of the participants were aroused by the Immigration protocol 1 clips. The two valence measures (bottom row Figure 2) have relatively similar values across issues and left-wing and right-wing clips. There is, on average, a bit more corrugator and zygomaticus activity with right-wing clips than with left-wing clips. For RMSSD—the attention measure (see upper right panel)—the mean values are negative. This indicates that heart-rate variability slows down when people concentrate. In most cases the mean is rather similar, but for the left-wing EU and Immigration (protocol 2) clips, the heart-rate variability is somewhat higher (therefore less attention).

Figure 2. Mean Levels of Arousal and Valence Per Clip
Attitude Extremity, Knowledge, and Arousal
Now we evaluate hypotheses 1 and 2. Arousal is the dependent variable, and it is measured as the difference in skin conductance between the treatment and the baseline. Figure 3 plots the effects of attitude extremity, political knowledge, and the control variables. The effect of political knowledge (H1) is positive but not statistically significant (b = 0.034, SE = 0.047, p = 0.466). The effect of attitude extremity (H2) is positive and statistically significant (b = 0.073, SE = 0.034, p = 0.031).Footnote 8 Therefore, we reject H1 while accepting H2. The effect of extremity is small—but with the exception of the gender and education covariates—it is the only significant individual-level variable. The plots on the right side of Figure 3 demonstrate that the effect of extremity is similar when analyzing the full exposure to the treatment or just the first 10 to 20 seconds of exposure. We do find significant differences in arousal between issues. Compared with the climate issue, the EU issue produces more arousal. Interestingly, immigration is more arousing than climate in the second protocol and less arousing than climate in the first protocol. If we split our analyses per issue (see Appendix C.1), the effects of extremity remain positive, but they are not always significant due to low power. The effects of political knowledge do differ in direction depending on the issue. For example, political knowledge has a positive effect for the inequality issue but a negative effect for the immigration issue (protocol 2). We have no strong explanation of why these differences in arousal per issue occur, but we discuss various possible reasons in the discussion section. The “right-wing” variable is insignificant in all our analyses, so there are no differences in arousal between left-wing and right-wing video clips.

Figure 3. Effects of Attitude Extremity and Political Knowledge on Change in Arousal in Response to Political Rhetoric about Immigration, Redistribution, EU, and Climate
Physiological Responses to Incongruent and Congruent Political Rhetoric
To test H3–H5, we ran a new series of OLS regression models. Here negative valence (H3, corrugator), positive valence (H4, zygomaticus), and attention (H5, heart rate variability) are the dependent variables. We again pool the data and analyze each physiological dependent variable (corrugator, zygomaticus, and heart rate variability) independently. Figure 4 reports the effects of the independent variables in these models (see tables in Appendix B for the regression output). Note that all variables, except for the dichotomous ones, are standardized to facilitate interpretation.

Figure 4. Physiological Responses to Attitudinal Incongruent Political Rhetoric
Do incongruent messages evoke negative affect (H3)? We find a positive association between the incongruence of the treatment and corrugator activity (b = 0.109, SE = 0.045, p < 0.001). This means that as incongruence increases, the activity of the corrugator becomes stronger. None of the other individual-level variables matter. Increases in temperature reduce corrugator activity somewhat. Also, corrugator activity in response to the inequality issue is somewhat lower than with the climate treatment. If we split this analysis per issue, we find that the effect of incongruence is driven by corrugator responses to the inequality and immigration (protocol 1) issues (see Appendix C.1).
Do congruent messages evoke positive affect (H4)? For this we look at the middle panel of Figure 4 and expect a negative sign of the incongruence variable. However, the sign is positive and insignificant. We therefore reject H4. This null-effect is not driven by a particular issue; all effects are clearly non-negative (see Appendix C.1).
Do incongruent messages evoke lower heart rate variability (H5)? Again, we expect a negative sign of the incongruence variable. However, the sign of the incongruence variable is positive and insignificant (Figure 4 right panel).Footnote 9 Furthermore, we find positive effects for alcohol and temperature and no level differences between issues. Splitting the analyses per issue does not change our conclusion (see Appendix C.1). In sum, we reject H5.
Consequences of Physiological Responses
Do these physiological responses have consequences regarding how people position themselves on these issues? We now use the posttreatment issue attitude questions and calculate the absolute difference between pretreatment and posttreatment position per issue. Comparing these positions, approximately 75% stays very close (less than one point change) to the original position.
Figure 5 presents three OLS regression analyses where absolute position change is the dependent variable. In these models, we have omitted heart rate variability and zygomaticus because we could not explain variation in these variables in our analyses in the previous section. First, we analyze absolute position change for the immigration film (protocol 1 during the cultural festival) only (left panel). We focus on the immigration issue (protocol 1) because we have only asked about self-reported emotions regarding this treatment (see left-hand panel of Figure 5). Attitude extremity reduces absolute position change, which is probably a ceiling effect. Interestingly, both arousal and negative valence have a positive effect on attitude change and are marginally significant (p < 0.10). Second, regarding the self-reported emotions, we asked respondents whether they felt enthusiasm, anxiety, or anger during the treatment. Introducing these variables changes the model substantially (see middle panel of Figure 5). The effects of anger and enthusiasm are positive but insignificant, while anxiety has a marginally significant (p < 0.10) positive effect. The effect of arousal remains the same, and the effect of negative valence increases in precision (p < 0.05). Third, we perform the same analysis for the whole sample (see right-hand panel of Figure 5). Now the self-reported emotions drop out because they were not asked for all issues. The effects of arousal and negative valence become somewhat weaker for the whole sample. Arousal remains positive and significant, whereas the effect of negative valence becomes insignificant. The effect of arousal is similar to Renshon, Lee, and Tingley (Reference Renshon, Lee and Tingley2015), who link physiological arousal to stronger anti-immigrant attitudes.

Figure 5. Estimates of Absolute Position Change
We have also evaluated the presence of mediation effects. We have considered physiological responses as mediators of attitudes (using the full sample) and physiological responses as mediators of self-reported emotions (using the analysis in the middle panel). We have followed the recommendations regarding mediation analysis set out by Imai, Keele, and Tingley (Reference Imai, Keele and Tingley2010). The findings give no indication of mediation effects (see Appendix C.3 for results). Part of this result is also visible when comparing the left and middle panel of Figure 5: the effects of SCL and corrugator barely change after controlling for the self-reported emotions.Footnote 10 As such, the effects of physiological responses seem to be operating independently of self-reported emotions and attitudes.
To conclude the results section, we would like to reflect on the effect sizes we reported. They are small but comparable to existing physiology work. Studies with similar designs and stimulus materials as our study have reported effect sizes between 0.028 and 0.085 (Soroka, Fournier, and Nir Reference Soroka, Fournier and Nir2019; Soroka and McAdams Reference Soroka and Mcadams2015; Wagner et al. Reference Wagner, Deppe, Jacobs, Friesen, Smith and Hibbing2014) and skin conductance responses to a series of validated threatening images between 0.029 and 0.048 (Arceneaux, Dunaway, and Soroka Reference Arceneaux, Dunaway and Soroka2018; Bakker et al. Reference Bakker, Schumacher, Gothreau and Arceneaux2020). Moreover, small effect sizes are common in political science (e.g., Claassen Reference Claassen2020; Kalla and Broockman Reference Kalla and Broockman2020; Shepherd and You Reference Shepherd and You2020) and the social sciences in general (Camerer et al. Reference Camerer, Dreber, Holzmeister, Ho, Huber, Johannesson and Kirchler2018). Finally, small effect sizes are not problematic as long as a study is sufficiently powered to reliably detect small effects—as is the case here.
Discussion
In this section, we first summarize our findings and core contribution. We continue with a reflection on the unexpected findings. After that we seek to further integrate the affective and cognitive components of emotions in a single theoretical model and set out a research agenda along these lines.
We find that people with more extreme attitudes on an issue experience more arousal when exposed to political rhetoric (H1). Political knowledge, however, does not explain variation in arousal (H2). We find that rhetoric that is incongruent with someone’s attitudes produces negative affect (H3), whereas congruent rhetoric does not produce positive affect (H4). Regarding our last hypothesis, we find no evidence that incongruence increases attentiveness (H5). Finally, we present evidence that arousal and negative affect predict issue position change, independent of self-reported discrete emotions.
The main contribution of this paper is that we establish the two-dimensional circumplex model in political science as a theory of core affect. We connect this model to existing theories in political science and formulate and test how some factors predict arousal in response to political rhetoric while others predict valence. In our study, arousal is stronger as attitudes become more extreme, which aligns with the suggestions from the directional model of voting (Rabinowitz and Macdonald Reference Rabinowitz and Macdonald1989) and motivated reasoning (Lodge and Taber Reference Lodge and Taber2013). Negative valence increases as disagreement with rhetoric increases, which provides evidence for the importance of the valence in motivated reasoning (Lodge and Taber Reference Lodge and Taber2013). Thereby we also move beyond existing work that studies only one dimension of core affect (Mutz Reference Mutz2007; Mutz and Reeves Reference Mutz and Reeves2005; Soroka, Fournier, and Nir Reference Soroka, Fournier and Nir2019; Soroka and McAdams Reference Soroka and Mcadams2015). Moreover, building on others who “lack[ed] direct measures of somatic response” (Lodge and Taber Reference Lodge and Taber2013, 208), we are the first to capture valence in response to political rhetoric using facial EMG. Applying such physiological measures we provide the first evidence for the affective underpinning of canonical political science theories.
Reflections on Unexpected Findings
There might be multiple reasons for why we rejected our hypothesis about political knowledge (H1). First, the failure to reject the null hypothesis might be due to measurement error in our short knowledge battery (Lord and Novick Reference Lord and Novick2008). Second, the validity of the type of knowledge questions used by us—and others (Brader Reference Brader2006; Taber and Lodge Reference Taber and Lodge2006)—has been criticized (Lupia Reference Lupia2006; Prior Reference Prior2013). Future work is therefore well advised to measure political knowledge with a longer and more diverse battery. Third, the role of knowledge as a moderator of political rhetoric is perhaps not as important as we initially thought. Close inspection of the work by Lodge and Taber (Reference Lodge and Taber2005), for instance, shows that knowledge predicts affective links in just one of their two experiments. Finally, although higher levels of political knowledge might make affective attachments to political objects stronger, careful consideration of these political objects by those with higher knowledge could also weaken these affective attachments again.
There are four possible explanations for why we rejected our hypothesis regarding positive affect (H4, zygomaticus activity). First, the analyses for the zygomaticus were run on a subset of the data (N = 141), so the analyses are likely underpowered. Second, even though previous studies have shown that the zygomaticus is activated in response to positive stimuli (Lang et al. Reference Lang, Greenwald, Bradley and Hamm1993), there is a high degree of measurement error in the zygomaticus (Larsen, Norris, and Cacioppo Reference Larsen, Norris and Cacioppo2003; Tassinary, Cacioppo, and Vanman Reference Tassinary, Cacioppo, Vanman, Cacioppo, Tassinary and Berntson2007).Footnote 11 Third, our treatments were not particularly positive in tone. Fourth, given research on the negativity bias (Soroka, Fournier, and Nir Reference Soroka, Fournier and Nir2019; Soroka and McAdams Reference Soroka and Mcadams2015), we should not expect a strong response from giving participants information they agree with.
We also rejected our hypothesis concerning attention (H5). Here, the problem may be that heart rate is regulated by the sympathetic and parasympathetic nervous systems and may therefore indicate both arousal and attention (Blascovich et al. Reference Blascovich, Vanman, Mendes and Dickerson2014). One way to strictly measure attention is to analyze high-frequency heart variability only, with the respiratory sinus arrhythmia measure (Blascovich et al. Reference Blascovich, Vanman, Mendes and Dickerson2014). Unfortunately our setup did not allow for the recording of this measure.
Our initial expectation was that issue salience should matter. However, at the individual level, issue salience consistently has zero effect. At the macro level, immigration was most salient at the time of data collection (den Ridder et al. Reference Den Ridder, Dekker, Van Houwelingen and Schrijver2016), but this is not reflected in the analyses. In fact, levels of arousal were systematically lower for the immigration issue in protocol 1. Another expectation we had was that there would be more arousal for easy issues than for hard issues. Easy issues, such as immigration and European integration, are highly symbolic, and they have been on the agenda for a long time (Carmines and Stimson Reference Carmines and Stimson1980). Hard issues, such as climate change and inequality, are more complex issues “for which symbolic referents are not readily available” (Johnston and Wronski Reference Johnston and Wronski2015, 3). By consequence, one could expect that “easy” issues evoke more arousal than “hard” issues. We find some evidence for this pattern (stronger arousal in EU and immigration protocol 2; see Figure 3) and some evidence against this pattern (less arousal in immigration protocol 1). We welcome well-powered studies that develop and test ideas about the extent to which issue characteristics condition the affective responses of citizens.
Next Steps in This Area of Research
Theories in political science—such as motivated reasoning (Lodge and Taber Reference Lodge and Taber2013) and receive-accept-sample (Zaller Reference Zaller1992)—have theorized differently about affect. In Zaller (Reference Zaller1992) affect is mostly cognitive evaluation, while in Lodge and Taber (Reference Lodge and Taber2013) it is mostly unconscious. Our suggestion is to approach affect as both conscious and unconscious (see, Keltner and Gross Reference Keltner and Gross1999). The introduction of the circumplex model and the physiological measures of core affect capturing both arousal and valence allowed us to theorize and test the unconscious role of affect in political information processing. Going forward, a core question is how core affect relates to self-reported discrete emotions. The latter is used in the current state-of-the-art (Aarøe Reference Aarøe2011; Delton, Petersen, and Robertson. Reference Delton, Petersen and Robertson2018; Huddy, Mason, and Aarøe Reference Huddy, Mason and Aarøe2015; Klar Reference Klar2013; Marcus, Neuman, and MacKeun Reference Marcus, Neuman and Mackuen2000), and typically the two components are seen as competitors: mutually exclusive approaches to pin down the emotions of the people we study. In defending the choice for one, the other needs to be discredited. For example, McDermott (Reference Mcdermott, Neuman, Marcus, Crigler and Mackuan2007, 384) summarizes, “clearly such self-reports are not as reliable . . . as the emotional physiological measures that some neuroscientists use in assessing online emotional processing such as skin conductance, heart rate and other biological measures.” Similarly, physiological measures are criticized for being invalid, as they typically—and also in this study—do not correlate with self-reported emotions (Osmundsen et al. Reference Osmundsen, Hendry, Laustsen, Smith and PetersenForthcoming).
We do not share these criticisms. Keltner and Gross (Reference Keltner and Gross1999, 468) explained that emotions have affective and cognitive components. Therefore, physiological and self-reported measures are different but valid indicators of emotions. These different components are dissimilar, and they are not necessarily aligned (Barrett and Satpute Reference Barrett and Satpute2019; Evers et al. Reference Evers, Hopp, Gross, Fischer, Manstead and Mauss2014). Recent work in neuroscience, for instance, suggests that different brain systems are responsible for physiological fear responses and cognitive fear responses (LeDoux and Pine Reference Ledoux and Pine2016). In line with this, we find evidence that arousal and negative affect predict attitude change, even when controlling for self-reported emotions (see Figure 5). We interpret these findings not as evidence for the superiority of physiological measurement but rather as evidence for the different effects it has compared with self-reported emotions.
It may be particularly relevant when the affective and cognitive components of emotions align or dealign. Dealignment may be due to a lack of reflection or limited cognitive access (Lapate et al. Reference Lapate, Rokers, Li and Davidson2014). Therefore, individual differences in “emotional granularity” (Barrett Reference Barrett2004) may be an important moderator. People who are more able to characterize emotions in terms of specific discrete emotions—that is, score higher on emotional granularity—might show stronger alignment between the discrete emotion they report and their physiological response compared with those who describe their emotions in “broad, global terms”—that is, score lower on emotional granularity (Barrett Reference Barrett2004, 37). Partisanship may also condition the alignment or dealignment of physiological affect and self-reported emotions in at least two possible ways. First, partisan parrots might echo the sentiments they hear from elites and report strong self-reported emotions, without actually having any affective (unconscious) response (Bakker, Schumacher, and Homan Reference Bakker, Schumacher, Gothreau and ArceneauxForthcoming). Second, partisan ostriches have physiological responses but do not report strong self-reported emotions because they are motivated to unconsciously or consciously down-regulate their cognitive response (Bakker, Schumacher and Homan Reference Bakker, Schumacher and HomanForthcoming; Butler, Gross, and Barnard Reference Butler, Gross and Barnard2014). When affective and cognitive responses align or dealign they may amplify or hamper the effect of political rhetoric on political attitudes and behaviors. To conclude, we expect that studying alignment and dealignment and its consequences is a promising avenue for future research.
Within the context of further situating the circumplex model of affect in political science we see several other fruitful avenues of further study. For example, our findings speak to an emerging literature on the negativity bias in political communication (Soroka, Fournier, and Nir Reference Soroka, Fournier and Nir2019; Soroka and McAdams Reference Soroka and Mcadams2015). The fact that we can explain variation in negative affect—but not positive affect—could be seen as an indication of the prevalence of negative over positive information. Specifically, future work could explore whether negative campaign ads (Brader Reference Brader2005), negative news (Soroka, Fournier, and Nir Reference Soroka, Fournier and Nir2019), and elite incivility (Mutz and Reeves Reference Mutz and Reeves2005) elicit negative affect. At the same time, we also see possibilities to use the circumplex model of affect to theorize and test the role of positive affect in explaining voter turnout and campaign involvement (Brader Reference Brader2005; Valentino et al. Reference Valentino, Brader, Groenendyk, Gregorowicz and Hutchings2011). Another option is to examine other individual differences in physiological reactions to political rhetoric (Brader Reference Brader2006). Our data show, for instance, that women respond with less physiological arousal in response to political rhetoric than men do. This exploratory finding contributes to a small body of research that explores if and to what extent individual differences—such as gender and ideology—can explain differences in physiological responses (Peterson et al. Reference Peterson, Jacobs, Hibbing and Smith2018; Soroka et al. Reference Soroka, Gidengil, Fournier and Nir2016).
Going forward, we recommend that researchers consider effect sizes and the validity of physiological measures. We have benchmarked our results against, among other things, physiological responses to validated negative and positive images from the International Affective Picture System (IAPS; Arceneaux, Dunaway, and Soroka Reference Arceneaux, Dunaway and Soroka2018; Bakker et al. Reference Bakker, Schumacher and Homan2020; Lang et al. Reference Lang, Greenwald, Bradley and Hamm1993) reported elsewhere. We encourage researchers to assess the validity of the physiological measures by presenting stimuli that have been shown to evoke activity on the physiological measure of interest, such as the IAPS pictures (Lang et al. Reference Lang, Greenwald, Bradley and Hamm1993). This has two advantages: (1) researchers can assess the validity of the physiological measure and (2) researchers can use the effect sizes of the validated images to interpret the effect size of the politically relevant stimulus. This allows researchers to directly assess whether affective responses to politics are comparable, smaller, or even larger than affective responses to other stimuli—for an illustration of this approach, see Bakker, Schumacher and Homan (Reference Bakker, Schumacher and HomanForthcoming).
To conclude, after a number of surprising political upheavals, societal and academic attention for the role of emotion in politics has rebounded. Our paper addresses this by setting the agenda for a new perspective on how emotions influence politics.
Supplementary Materials
To view supplementary material for this article, please visit http://dx.doi.org/10.1017/S0003055420000519.
Replication materials can be found on Dataverse at: https://doi.org/10.7910/DVN/LCWR7G.
Comments
No Comments have been published for this article.