Introduction
The consequence of rapid technological change, i.e. digitalization and the automation of work, is a hotly debated topic in both public and academic debates. Some expect large-scale transformative changes on the labor market that might lead to mass unemployment and increasing job polarization (Frey and Osborne, Reference Frey and Osborne2017; McAfee and Brynjolfsson, Reference McAfee and Brynjolfsson2016), whereas others posit that the current wave of technological change is not fundamentally different from previous waves (Mokyr et al., Reference Mokyr, Vickers and Ziebarth2015) and will therefore have a more limited impact on the world of work and welfare. The bulk of existing work on the implications of digitalization and automation for work and welfare has so far focused on labor market outcomes such as employment and wage levels. Instead, and building on a growing literature that explores the association between general labor market risks and social policy preferences (Rehm, Reference Rehm2009) as well as the pioneering studies of Thewissen and Rueda (Reference Thewissen and Rueda2019), Dermont and Weisstanner (Reference Dermont and Weisstanner2020), Sacchi et al. (Reference Sacchi, Guarascio, Vannutelli, Careja, Emmenegger and Giger2020) and Im (Reference Im2020), this paper addresses the question of how automation risk is related to individual attitudes towards the welfare state. Different from the few previous studies, we adopt a broader perspective on the dependent variable, moving beyond redistribution to include support for basic income as well as social investment employment policies, and we take into account to what extent the welfare state context influences the micro-level association between risk and social policy preferences (cross-level interaction effects).
To briefly foreshadow our main findings: employing cross-national survey data from the 2016 wave of the European Social Survey (ESS) for 21 countries, our analysis confirms that automation risk – operationalized via the automation risk of individual occupations – is positively associated with support for redistribution. We do not find any statistically significant association with support for basic income. Further, we find that automation risk is negatively related to support for social investment policies, i.e. expanding active labor market policies and training, although the statistical significance of this finding depends on the specific measure of automation risk. This is surprising and – from a policy-makers’ perspective – worrying since the expansion of further training opportunities is a frequently heard policy recommendation to deal with technological change. Finally, we also find that welfare state contexts matter: in residual welfare states, the association between automation risk and support for any kind of social policy tends to be more positive than in generous welfare states, probably because, in the latter case, individuals can more easily rely on existing social safety nets.
Literature Review
Existing literature on the implications of the recent wave of technological change has mainly focused on its effects on labor market outcomes such as wages and employment opportunities. Of course, technological change has been identified as a driving force of labor market inequalities for previous periods as well (OECD, 2011). Hence, some scholars argue that the current wave of technological change is not inherently different from past waves (Mokyr et al., Reference Mokyr, Vickers and Ziebarth2015). However, others believe that the changes of employment observed in recent years might in fact be interpreted as evidence that ‘this time might be different’, because digitalization and automation in the current period will likely replace “not only muscle but also brain power” (Bührer and Hagist, Reference Bührer, Hagist, Ellermann, Kreutter and Messner2016: 115). Furthermore, even though technological advancements may lead to more employment because of increased productivity in the long term (Acemoglu and Restrepo, Reference Acemoglu and Restrepo2019), technological change is likely to create significant displacement effects in the short to medium term, as the skill profiles of current-day workers no longer match the requirements of the developing knowledge economy. The aftershock of the Corona crisis is likely to reinforce these trends.
In line with this argument, much scholarship in recent years has focused on the connection between technological change and labor market polarization. Inspired by the work of Autor et al. (Reference Autor, Levy and Murnane2003) on routine-biased technological change (RBTC), which in turn is very much related and inspired by previous work on skill-biased technological change (Acemoglu, Reference Acemoglu2002), the main thrust of this literature has been to assess whether and to what extent RBTC is associated with a polarization of labor markets in terms of wages and employment opportunities. Empirically, a significant body of work has demonstrated that such a “hollowing out of the middle” and the implied increase in inequality is occurring in OECD countries, albeit to different degrees depending on the national context (Autor and Dorn, Reference Autor and Dorn2013; Goos and Manning, Reference Goos and Manning2007; Goos et al., Reference Goos, Manning and Salomons2014; Graetz and Michaels, Reference Graetz and Michaels2018; Michaels et al., Reference Michaels, Natraj and van Reenen2014).
The literature cited in the previous paragraph adopts a backward-looking perspective, i.e. studying how technological change has affected labor markets in the past. A different approach is pursued by studies that aim at deriving predictions about the future automation potential of jobs and occupations. The influential, but also controversial study of Frey and Osborne (Reference Frey and Osborne2017), for instance, develops an index measuring the automation potential of occupations based on expert assessments of current and expected engineering bottlenecks in technological development. Adopting this approach, the study posits that 47 percent of employment opportunities in the United States are at high risk of automation in the near future (ibid. 265). Other studies with a similar approach have come up with lower estimates of jobs at high risk of automation (Nedelkoska and Quintini, Reference Nedelkoska and Quintini2018). The approach pursued by Frey and Osborne (Reference Frey and Osborne2017) has been criticized for a number of reasons. One issue is that Frey and Osborne (Reference Frey and Osborne2017) focus on the automation potential of whole occupations, whereas a more fine-grained perspective would look at the automation potential of tasks that make up different occupations. Adopting such a task-based rather than occupation-based approach, Arntz et al. (Reference Arntz, Gregory and Zierahn2016) find that merely 9 percent of jobs across 21 OECD countries are at risk of being automated in the coming years.
All of the previously mentioned studies are concerned with the labor market outcomes related to technological change. So far, little work has been done on the question of how these labor market outcomes in turn affect welfare state attitudes and preferences. One research topic in this field is the question of how citizens perceive and evaluate technological change and how these perceptions might be influenced by the welfare state: a more generous welfare state could increase citizens’ tolerance for more rapid technological change since the welfare state provides social protection (Dekker et al., Reference Dekker, Salomons and van der Waal2017; Lim, Reference Lim2020). Other work explores the implications of the rise of the platform economy for labor markets and the welfare state (Culpepper and Thelen, Reference Culpepper and Thelen2020; Eichhorst et al., Reference Eichhorst, Hinte, Rinne and Tobsch2016; Thelen, Reference Thelen2018).
Of greater relevance for this paper are studies that look at the association between individual labor market risk related to technological change and welfare state attitudes (Thewissen and Rueda, Reference Thewissen and Rueda2019; Dermont and Weisstanner, Reference Dermont and Weisstanner2020; Im, Reference Im2020; Sacchi et al., Reference Sacchi, Guarascio, Vannutelli, Careja, Emmenegger and Giger2020). In line with existing work on the association between labor market risk and welfare state policies (Rehm, Reference Rehm2009; Rehm et al., Reference Rehm, Hacker and Schlesinger2012), Thewissen and Rueda (Reference Thewissen and Rueda2019) find that automation risk, as measured as routine task intensity, is positively associated with support for redistributive policies as workers whose jobs are at risk demand protection from the welfare state. Dermont and Weisstanner (Reference Dermont and Weisstanner2020), in contrast, do not find a statistically significant association between automation risk and support for basic income, whereas Sacchi et al. (Reference Sacchi, Guarascio, Vannutelli, Careja, Emmenegger and Giger2020) provide more mixed findings on this issue. Im (Reference Im2020) shows that automation risk is positively associated with support for active labor market policies.
Theory
This paper follows in the footsteps of Thewissen and Rueda (Reference Thewissen and Rueda2019) but adopts a much broader perspective. As is long known in welfare state scholarship, the welfare state is not only (or maybe not even primarily) about redistribution, but also about providing social insurance against a range of social risks, from unemployment, old age and illness to new social risks such as single parenthood or a lack of skills. Furthermore, recent work on social investment argues that welfare states should “create, mobilise, and preserve skills/human capital/capabilities” (Garritzmann et al., Reference Garritzmann, Häusermann, Palier and Zollinger2017: 37) in order to “prepare support, and equip individuals in a way that increases their chance to participate in the knowledge-based economy and reduces their future risk of income loss and poverty” (ibid. 36, see also Morel et al., 2012, Bonoli, Reference Bonoli2013, Hemerijck, Reference Hemerijck2018; Ronchi, Reference Ronchi2018). In a similar vein, pundits and academic experts studying the implication of digitalization for the world of work regularly point to the crucial role of policies supporting education and employment (Colin and Palier, Reference Colin and Palier2015; McAfee and Brynjolfsson, Reference McAfee and Brynjolfsson2016).
A contrasting approach is taken by supporters of a universal basic income (van Parijs, Reference van Parijs2004; Martinelli, Reference Martinelli2020). The debate about the introduction of an universal basic income (UBI) has been going on for some time, but it clearly gained in prominence in the context of the digital transformation of work (Roosma and van Oorschot, Reference Roosma and van Oorschot2020; Vlandas, Reference Vlandas2019). The apparent connection between the UBI and the labor market consequences of technological change is that the basic income could safeguard the potential losers of this change against income loss and precarious employment, encouraging them to become more entrepreneurial and risk-taking. Critics of the UBI, however, fear that it might lead to a new cleavage between those living on a basic income on the one hand and those in classical types of formal employment on the other (Colin and Palier, Reference Colin and Palier2015).
So far, these debates about which kinds of policies should be adopted have mainly played out on the level of policy elites and experts. Little attention has been paid to the question of what policies those who are actually most likely to be affected by digitalization and automation in fact demand themselves. Even though there is little research on this issue, we can derive plausible expectations from existing findings.
Regarding support for redistribution, a straightforward expectation in line with Thewissen and Rueda (Reference Thewissen and Rueda2019) is that individuals in occupations at high risk of automation will express significantly higher levels of support for redistribution in order to compensate for expected income losses due to unemployment. Likewise, individuals facing a high automation risk should also be more likely to support more generous social transfer programs – in particular, more generous unemployment insurance programs. Even though experts might regard a heavy focus on social transfers as detrimental to economic growth and technological development, the individuals directly affected by potential rationalization are likely to demand these types of policies from their government since they are directly related to perceived risks in the short term.
In contrast, support for social investment policies, such as expanding opportunities for lifelong learning and labor market training, may be more contingent. Admittedly, previous research has shown that, in general, social investment policies are supported by large majorities across European countries (Busemeyer et al., Reference Busemeyer, Garritzmann, Neimanns and Nezi2018; Garritzmann et al., Reference Garritzmann, Busemeyer and Neimanns2018). Nevertheless, many of the supposed and expected benefits of social investment policies materialize in the long term rather than the short term. Regarding employment policies, therefore, individuals could be reluctant to support active labor market policies with a stronger long-term outlook such as training and lifelong learning if they are facing a concrete threat of unemployment in the near future. Overall, we still expect a positive association between automation risk and support for social investment type employment policies (similar to Im, Reference Im2020), albeit a weaker one compared to the association between risk and redistribution support.
Finally, it is an open question whether tech-related automation risk is related to support for an UBI (Dermont and Weisstanner, Reference Dermont and Weisstanner2020). From a political point of view, support for – or opposition against – the notion of UBI stems from different sources (Vlandas, Reference Vlandas2019), depending on the details of policy design such as the level of the basic income, its conditionality, whether it is supposed to replace existing social benefits or not, etc. This is why the wording of the survey question in the ESS (see below) includes a rather detailed description of a more generous and unconditional implementation of a UBI. On the one hand, it is plausible to assume that individuals in jobs at high risk of automation are more likely to support the introduction of a UBI as a basic form of social protection. On the other hand, in contrast, those in occupations at high risk of automation could be more likely to prefer continued employment in their current job or rather demand a more generous and income-related unemployment insurance scheme. Taken together, these countervailing forces could balance each other out in the aggregate, leading us to expect a weak or no association between tech-related automation risk and support for the UBI.
In sum, our hypotheses are:
Hypothesis 1: There is a positive association between individual automation risk and support for redistributive policies and a more generous welfare state broadly defined.
Hypothesis 2: There is a positive, although less strong association between individual automation risk and support for social investment employment policies.
Hypothesis 3: There is no strong association between individual automation risk and support for the introduction of a UBI.
Next, we study to what extent the welfare state contexts matter for preferences, building on the insights of the large literature on policy feedback (Busemeyer et al., Reference Busemeyer, Abrassart and Nezi2019). In a first step, we focus on the overall size of the welfare state and how it might mediate the association between automation risk and social policy preference. We expect that individuals in residual welfare states should be more concerned about the negative side effects of technological change since there are fewer social safety nets to rely on. Hence, the association between automation risk and support for expanding social policy (in different dimensions) should be more positive in these countries, i.e. individuals facing higher risk demand more social policy. In contrast, automation risk should be a less important determinant of social policy preferences in generous welfare states, since these provide a more robust safety net against new emerging labor market risks.
In a second step, we distinguish between different institutional dimensions of the welfare state, focusing on the distinction between active and passive labor market spending since this is most directly connected to our core research question. The analogous reasoning to the above leads us to expect a more positive effect of automation risk on support for passive transfers in countries with lower levels of PLMP spending and a more positive effect of automation risk on support for ALMP in countries in which spending on these policies is below average. In short, this reasoning amounts to a logic of compensation as individuals facing high automation risk demand more spending on that type of policy which is currently less well developed in their particular country.
Taken together, our fourth hypothesis is:
Hypothesis 4: The association between automation risk and support for expansive social policies is more positive (negative) in more residual (generous) welfare states, i.e. we expect a negative cross-level interaction effect between automation risk and the size of the welfare state on support for social policy. Likewise, we expect high-risk individuals to demand more spending on transfers (i.e. redistributive policies) in countries with a low level of PLMP spending and to demand more spending on ALMP in countries with a low level of ALMP spending.
Data and methods
For our analysis we make use of the European Social Survey (ESS), Wave 8 (ESS, 2016). The ESS is a repeated comparative survey of individuals living in European countries, that is well-known for its high standards in survey sampling and data quality. In 2016 more than 40,000 individuals were representatively sampled from 21 countriesFootnote 1 and asked for a wide range of social policy attitudes as part of a special module on the welfare state.
We select three questions as dependent variables, tapping into support for social investment, basic income and redistribution, respectively. We add a fourth dependent variable that is an aggregate measure of respondents’ general support for the welfare state (see below).
Support for social investment policies is measured by the following question:
Now imagine there is a fixed amount of money that can be spent on tackling unemployment. Would you be against or in favour of the government spending more on education and training programs for the unemployed at the cost of reducing unemployment benefit?
Admittedly, this question only captures only a particular aspect of the social investment model of the welfare state. Also, somewhat different from the other questions used below, it confronts respondents with a trade-off scenario within a constrained budget, effectively forcing respondents to prioritize between ALMP and PLMP. To some extent, this delimits the comparability between the questions used in this analysis, but unfortunately the ESS does not offer alternative measures of support for ALMP.Footnote 2 Thus, when interpreting the results, it should be taken into account that the specific measure of ALMP support might underestimate the support for this type of policy to some extent. As Busemeyer and Garritzmann (Reference Busemeyer and Garritzmann2017) have shown, support for social investment policies declines significantly once budget trade-offs are acknowledged and taken into account (see also Busemeyer and Lober, Reference Busemeyer and Lober2020). However, since the above measure nicely brings out the difference between a social investment oriented approach that prioritizes investments in training and skill acquisition on the one hand and a more traditional, compensatory approach focusing on passive transfers on the other, we regard the question as a valid measure of support for social investment (in employment policy), while being aware of its limitations.
To operationalize support for basic income, we use the following question:
Some countries are currently talking about introducing a basic income scheme. […] A basic income scheme includes all of the following: The government pays everyone a monthly income to cover essential living costs. It replaces many other social benefits. The purpose is to guarantee everyone a minimum standard of living. Everyone receives the same amount regardless of whether or not they are working. People also keep the money they earn from work or other sources. This scheme is paid for by taxes. Overall, would you be against or in favour of having this scheme in [your country]?
As briefly mentioned above, the wording of this question necessarily contains a rather detailed definition of the policy design of the hypothetical UBI scheme. The specific wording has some limitations, i.e. it does not specify the level of the basic income, which other social benefits might be replaced and which taxes will be used or raised in order to finance it. Still, the wording of the question suggests a rather generous and unconditional basic income scheme, which may explain why other studies, using the same data, have found that support is correlated with left-leaning ideological predispositions (Roosma and van Oorschot, Reference Roosma and van Oorschot2020; Vlandas, Reference Vlandas2019).
To assess respondents’ general redistributive preferences, we include responses to the following question, which is the standard measure used in the literature to measure support for redistribution:
To what extent do you agree with this statement: The government should take measures to reduce differences in income levels.
For the first two questions, participants could respond on a four-point Likert-type scale ranging from “strongly against” and “against” to “in favour” and “strongly in favour” with no middle category. For our analysis we transform these into a binary variable indicating support or no support for a given proposal in order to increase the ease of interpretation of our results. For the question on redistribution, participants could answer on a five-point Likert-type scale, ranging from “agree strongly”, “agree”, “neither agree nor disagree” to “disagree” and “disagree strongly”. In order to allow a comparison to the policy proposals we, again, transformed this question into a dummy variable, differentiating (strongly) agreeing from indifferent or disagreeing individuals. In the robustness section, we show that our findings are robust to the use of categorical variables and other model specifications.
As a fourth dependent variable we use a multidimensional welfare state measure first presented in Roosma et al. (Reference Roosma, Gelissen and van Oorschot2013). This measure is based on the dimensions of welfare state policies (goals, range, degree, efficiency, effectiveness [abuse/underuse] and outcomes [goals, policy, economic and moral]), capturing attitudes towards the welfare state in a broader sense than the previously presented variables. However, since these dimensions were constructed based on the ESS 4 in Roosma et al. (Reference Roosma, Gelissen and van Oorschot2013), there are some minor differences – which we document in greater detail in the appendix (see Table 1 in the Appendix for a comparison of the factors and Table 2 for summary statistics and model coefficients). We include a measure of “welfareism” primarily as a robustness check to our redistribution measure, while also partly mitigating the limitations of that measure, which only captures one particular dimension of welfare state policies.
The main independent variables of interest are two measures of automation risk by Frey and Osborne (Reference Frey and Osborne2017) and Arntz et al. (Reference Arntz, Gregory and Zierahn2016).Footnote 3 We refrain from using a measure of routine task intensity (RTI) which has been used in other papers similar to ours (Thewissen and Rueda, Reference Thewissen and Rueda2019; Dermont and Weisstanner, Reference Dermont and Weisstanner2020), because the available RTI measure uses past data on routinization potential of occupations (ultimately based on a dictionary of US occupations from the 1970s), which, in our view, is less suited to assess the future automation potential of occupations. Above, we already briefly discussed the main differences between the Frey/Osborne and the Arntz et al. measure. To recap briefly, the Frey/Osborne measure taps into automation risk at the level of whole occupations, based on the assessment of experts. The Arntz et al. measure adopts a more fine-grained approach by looking at the automation potential of individual tasks, using data from the OECD’s PIAAC study.Footnote 4 We match the automation risk measures to our survey data via the ISCO-08 occupational codes included in the dataset. Since the Arntz et al. measure is only available for a subset of countries in our sample,Footnote 5 we present two versions of the models using the Frey-Osborne index: one with the full set of countries for which the measure is available, and a second one with a reduced set of countries to match the sample of countries when using the Arntz et al. measure. As these measures are defined based on the respondents’ occupation, we exclude all currently (last seven days) unemployed individuals from the sample. Also, we focus on the working-age population only, excluding retired persons (if not working part-time) and other non-working individuals.
We control for potentially confounding variables and check the robustness of our findings by adding different control variables. Our baseline models include gender, age, education (according to the ISCED scheme), income in country-specific deciles, whether participants or their parents were born in their country of residence, place of living (urban vs. rural) and whether respondents have children living with them. In a next step we add controls for participants’ self-declared political left-right placement. Subsequently, we add risk controls in the form of occupational unemployment (computed from ESS data as the unemployment rate on the ISCO-08 two-digit level) and industrial unemployment (computed from ESS data as the unemployment rate on the level of NACER industries). All non-binary independent variables are standardized before analysis.
We analyze our data using Multi-Level-Models (MLM) with random intercepts. While the number of countries in our sample is generally considered to be close to the minimum of the required level-two units for Multi-Level-Modelling (Stegmueller, Reference Stegmueller2011), recent evidence suggests that such an analysis is still feasible and valid (Elff et al., Reference Elff, Heisig, Schaeffer and Shikano2019). To ensure unbiasedness of our results we conduct several robustness checks, as detailed below. For the first three dependent variables, we employ logistic regression, whereas we use simple linear regression for the fourth variable measuring “welfareism”.
Results: Individual-level determinants
Figure 1 shows the results for the variables of interest including different sets of control variables as explained above (see Tables 3-14 in the Appendix for the detailed regression tables). We first use a basic set of controls, then add variables on ideology and occupational risk measures, respectively.
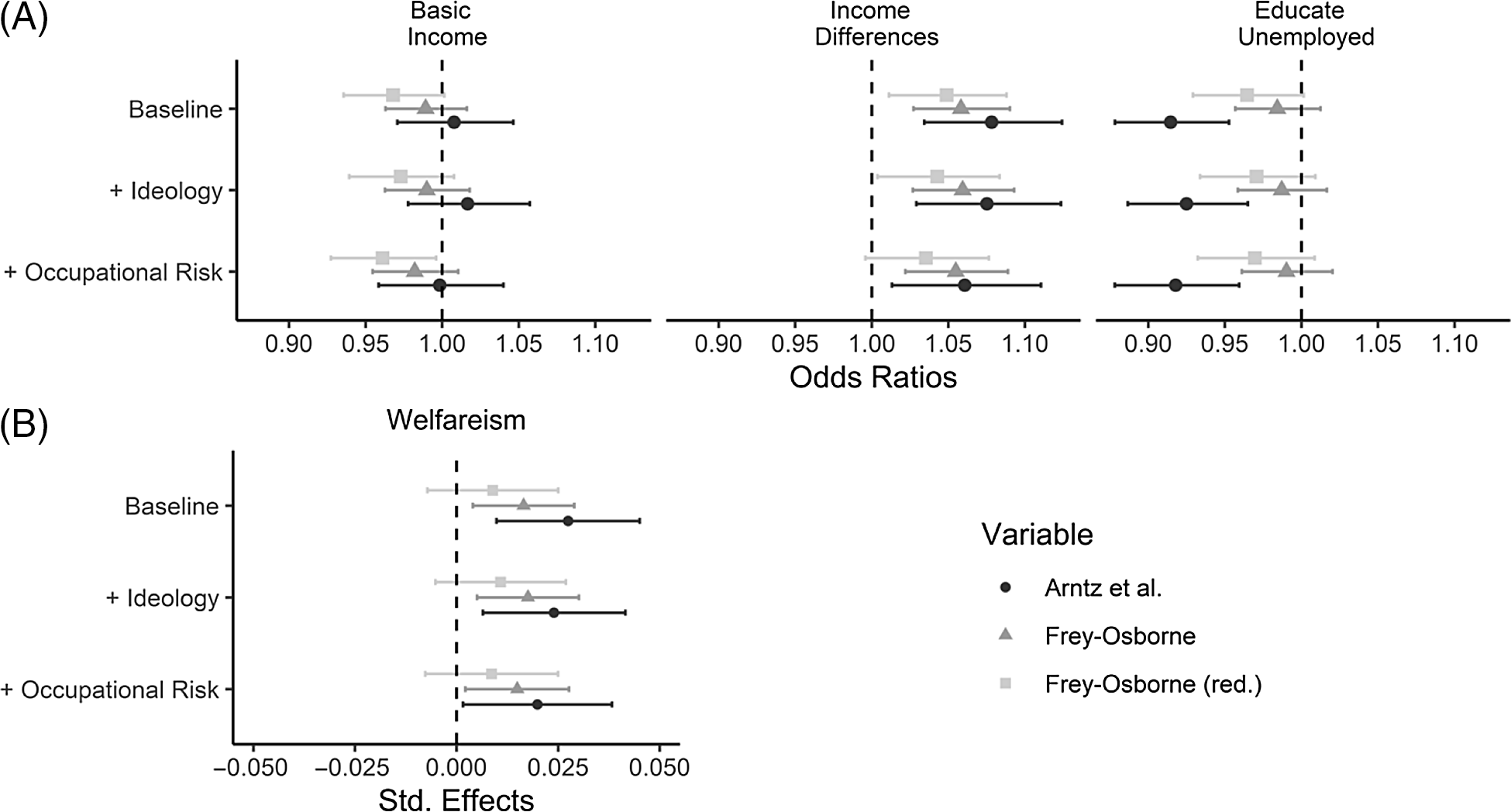
FIGURE 1. Multi Level Models predicting different facets of welfare state support. (A) Logistic regressions predicting support for a basic income scheme, reduction of income differences and social investment in unemployed individuals. (B) Linear regression predicting welfareism. All results display point estimates with 95%-Confidence Intervals.
We start our discussion of the findings with the association between individual automation risk and support for redistribution and a more generous welfare state (Hypothesis 1). As expected, we find a positive association between automation risk and support for redistribution, independent of which measure of automation risk is used, confirming the previous results from Thewissen and Rueda (Reference Thewissen and Rueda2019). We also find a positive association between automation risk and support for the welfare state broadly defined; although in this case, the association is statistically significant only when using the more fine-grained measure provided by Arntz et al. The overall positive association between automation risk and support for redistribution also holds when including a measure of occupational risk, which takes into account other sources of labor market risk besides technological change.
To provide a sense of the magnitude of these effects, we calculate the difference between two expected values (EVs) for the Arntz et al. automation measure, based on simulations from our models with full controls (King et al., Reference King, Tomz and Wittenberg2000). In the case of support for redistribution, an increase in automation risk by one standard deviation is associated with an increase in support by 2.37 percentage points, which is significantly smaller than changes in predicted probability associated with a similar change in income (minus 9.8 percentage points) or ideology (minus 17.48 percentage points) – two variables that are widely known to have a strong effect on welfare state attitudes. For welfareism, the results are similar. In his case, the difference in EVs amounts to an increase of 0.04 in the dependent variable, which is considerably smaller than −0.26 for income and -0.45 for ideology.
Next, we probe the association between automation risk and support for social investment policies (Hypothesis 2). Here, against expectations, we find a negative association between automation risk and support for social investment policies in the domain of unemployment insurance when using the Arntz et al. measure. When using the Frey-Osborne measure, the association turns non-significant. As stipulated above, we rather expected a positive, albeit weaker association between automation risk and support for social investment. Furthermore, the magnitude of the effect is rather large: performing a similar simulation to the one above, a change of one standard deviation in automation risk is associated in a reduction of 4 percentage points in support for social investment, which is comparable in magnitude to the effect of income (6.05%) and ideology (5.65%). Generally, right-leaning and economically affluent individuals are significantly more likely to support social investment policies. Conversely, being female, old age, having children and having a migrant background is negatively associated with support for social investment.
Finally, we find, as expected, a weak association between support for basic income and automation risk, in line with Hypothesis 3. When using the Frey-Osborne measure, the models indicate a negative association between support for basic income and automation risk, which even turns statistically significant in the model specification that includes controls for occupational unemployment risk. When using the more fine-grained Arntz et al. measure, the relationship becomes a non-association, however. Thus, even though the UBI is often discussed in public debates about digitalization and automation as a potential solution to the negative side effects of technological change, it seems that the supporting coalition for the UBI is made up of left-leaning, low-income, urban and poorer individuals (Roosma and van Oorschot, Reference Roosma and van Oorschot2020; Vlandas, Reference Vlandas2019) rather than those directly affected by technological change in the labor market.
Taken together, our findings have important implications. Even though pundit and academic experts regularly recommend further investments in lifelong learning, vocational training, human capital and skills in order to cope with the challenges of the digital transformation of the world of work (Colin and Palier, Reference Colin and Palier2015; McAfee and Brynjolfsson, Reference McAfee and Brynjolfsson2016), our analysis shows that those most likely to be directly affected by automation are less supportive of these kinds of social investment policies. At best, they are indifferent, at worst they actually seem to be against shifting the priorities of unemployment policies from passive compensation to active training measures. Instead, those affected by automation are more supportive of governmental redistribution. This certainly represents a challenge for policymakers seeking to build coalitions in support for transforming welfare states to meet the challenge of digitalization. A straightforward and plausible explanation for this finding is that workers simply prefer direct and short-term forms of compensation over long-term oriented policies, even though the latter could eventually be more effective in reducing future automation risk.
Results: Cross-level interactions
In the next step, we probe whether the individual level-associations between automation risk and welfare state attitudes identified in the previous section are mediated by macro-level contexts, i.e. the institutional set-up of the welfare state. We first focus on the overall size of the welfare state. We approximate welfare state generosity with the share of social spending relative to the gross domestic product for the year 2016 (OECD, 2020). Even though social spending is only a proxy for welfare state generosity, it is in this particular case preferable to alternatives measures of welfare state generosity such as the Comparative Welfare Entitlements Dataset (CWED) because it is more widely available and not only focused on the generosity of transfers as generosity data is. In a second step, we look at cross-level interaction effects between automation risk and spending on passive and active labor market policies, respectively, also taken from the OECD’s Social Expenditure Database. As an additional control for country-level contexts, we include the Gini coefficient of income inequality after taxes and transfers (as this is the type of inequality that is immediately experienced by individuals) from 2016 (Solt, Reference Solt2019) in our analyses since this is expected to be associated with demand for redistribution (Finseraas, Reference Finseraas2009).Footnote 6 Furthermore, we include the full set of individual controls as previously presented. All country-level variables are standardized before analysis.
Our findings largely confirm our theoretical expectations. Figure 2 is a graphical representation of the cross-level interaction between automation risk and welfare state generosity. In the top row, we use the Frey-Osborne measure for automation risk, whereas the lower row uses the Arntz et al. measure. We analyze cross-level interactions for attitudes towards basic income (left column), redistribution preferences (center column) and social investment labor market policies (right column). The detailed regression models are available in Table 15 in the Appendix.
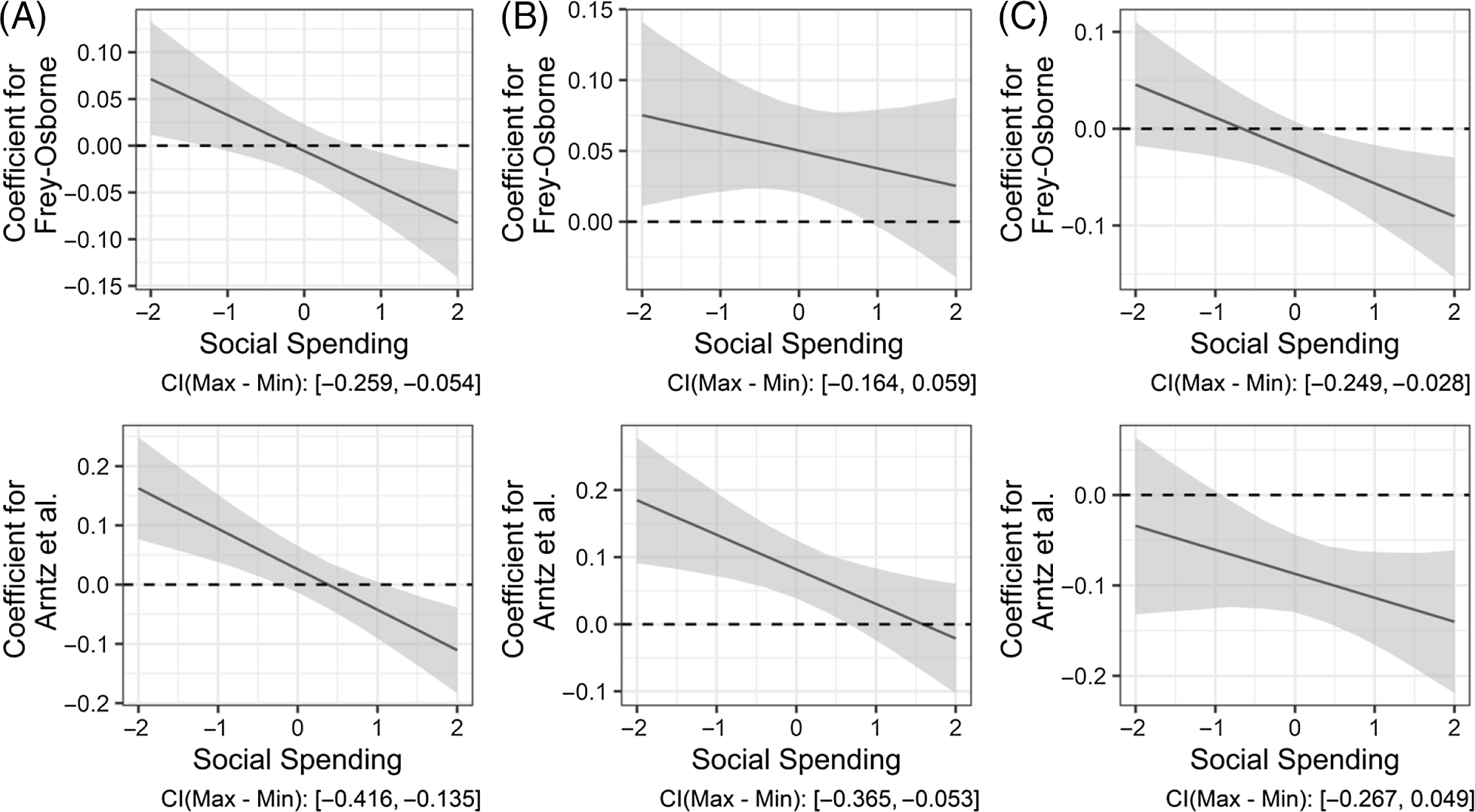
FIGURE 2. Multi Level Models predicting support for (A) basic income, (B) reducing differences in income and (C) education for the unemployed. All models include the cross-level interaction of automation risk and social spending. Grey areas indicate 95% Confidence Intervals. Confidence Intervals of the moderation are indicated at the bottom.
Across all specifications, we find a clear negative cross-level interaction effect between automation risk and support for different types of social policy. The fact that the regression lines (as well as the confidence intervals) cross the zero mark indicates that, at low levels of social spending, the interaction effect between automation risk and support for a particular social policy is positive (and often in a statistically significant manner), but turns negative for higher levels of spending. Broadly speaking, in residual welfare states, the association between automation risk and support for social policy is more positive compared to more generous welfare states. Thus, a more generous welfare state indeed plays a buffering role for the negative side effects of technological change: if the welfare state is more (less) developed, demand for a further expansion of social policy is decreased (increased).
In spite of the broad tendency identified above, we find interesting differences across the three policy types. In the case of support for basic income, the association between automation risk and support is positive and significant for low levels of social spending and turns negative (and significant) for high levels of social spending. In the case of redistribution preferences, we also find a significant and positive association between automation risk and support for redistribution in residual welfare states, but the association does not turn negative for high levels of social spending, but merely insignificant. Finally, in the case of social investment labor market policies, the negative association between automation risk and support for these policies identified above turns even more negative (and is statistically significant) for individuals residing in generous welfare states. This partly puzzling finding reaffirms our previous finding from above that those that are most likely to be affected by technological change are still less likely to support activation measures in labor market policy, presumably preferring direct means of compensation (although not necessarily basic income schemes as our other findings show).
In a second step, we zoom in on institutional differences between welfare states, focusing on the distinction between passive and active labor market spending. For reasons of space, we only include models using the Frey-Osborne measure in Figure 3 (see Table 16 in the appendix for detailed results); the findings using the Arntz et al. measure are similar, but usually have larger confidence intervals because of a loss of observations due to the more limited country coverage of this measure. Somewhat different from what we expected above, the cross-level associations between being at high risk of automation and different levels of spending on active and passive labor market policies are very similar to each other as well as to the interaction with overall levels of spending. Again, being at high risk of automation is associated with higher (lower) support for a universal basic income at low (high) levels of spending. Similarly, we find a negative effect of automation risk on support for active labor market spending if either PLMP or ALMP spending is high already. An important difference between Figures 2 and 3 is that, when looking at the interaction with PLMP and ALMP spending, there is no statistically significant interaction effect with support for redistribution. Taken together, these findings indicate that the overall size of the welfare state apparently matters more than relative spending levels across its different dimensions. Further research is clearly necessary here, e.g. providing a more fine-grained operationalization of institutional differences between welfare states.
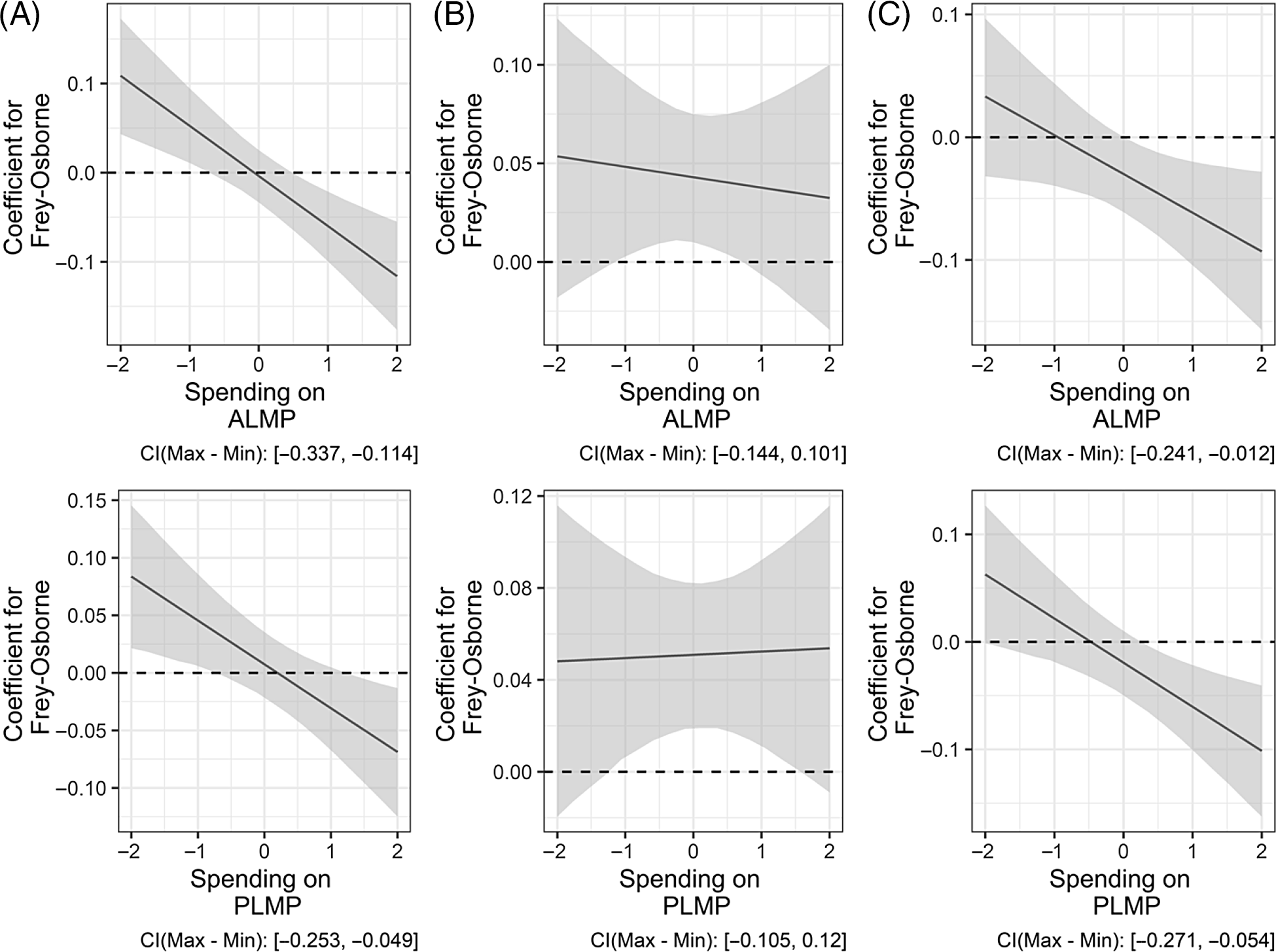
FIGURE 3. Multi Level Models predicting support for (A) basic income, (B) reducing differences in income and (C) education for the unemployed. All models include the cross-level interaction of automation risk and spending of ALMP (upper row) and PLMP (lower row). Grey areas indicate 95% Confidence Intervals. Confidence Intervals of the moderation are indicated at the bottom.
Robustness Checks
Since the number of countries (level-2 units) in our analysis is generally considered low for unbiased results from MLMs, we replicate our results using a recently proposed methodology by Elff et al. (Reference Elff, Heisig, Schaeffer and Shikano2019). In their study, the authors find that unbiased estimation is possible even with as few as five level-two clusters if Restricted Maximum Likelihood (REML) estimation is used and the appropriate degrees of freedom for the coefficient t-tests are applied (see Tables 17-20 in the Appendix). Using this method, we can fully replicate our previous findings. To further strengthen confidence in our results, we estimate country fixed-effects models with country-clustered standard errors for all our dependent variables in their original coding (see Tables 21-24 in the Appendix). Again, the results closely mirror the previous findings with only minor exceptions. In a further extension, we include dummy variables for different industrial sectors (Figure 1 in the Appendix), which does not affect the main findings. Finally, since we recoded ordinal responses into a binary measure of support for the first three dependent variables used above, we run a series of ordinal MLMs for redistribution preferences, support for social investment and basic income, respectively (see Tables 25-27 in the Appendix). We also run a series of linear probability models (Figure 2 in the Appendix). All previous results are corroborated when using these models.
Conclusions
This paper has studied the association between automation risk and individual-level support for different types of social policy. Complementing the few existing studies on the association between automation risk and welfare state attitudes, this paper provides a new perspective by looking at the three dimensions of redistributive policies, social investment and UBI jointly. It is also the first to explore the cross-level interaction between automation risk at the micro level and the macro level of welfare state institutions and policies. Our analysis confirms that automation risk is positively related to support for redistribution, but not statistically associated with support for basic income and negatively with support for social investment policies, at least in the domain of labor market policy. The latter findings needs to be put in perspective, because – as explained above – the particular wording of this question in the survey might lead to an underestimation of the support for social investment policies. We also found evidence that the welfare state context matters with individuals in generous welfare state being less supportive of further expansions of social policy due to automation compared to individuals in residual welfare states. Generally speaking, however, the association between automation risk and welfare state attitudes is somewhat less substantial and less robust compared to other factors such as income or ideology, which are widely known to have a strong effect on attitudes. This could be because individuals may not be aware (yet) of the extent of automation risk. Further research is clearly needed here.
Our research has important policy implications. Increasing investments in human capital is a commonly heard policy recommendation in response to technological change (Colin and Palier, Reference Colin and Palier2015; McAfee and Brynjolfsson, Reference McAfee and Brynjolfsson2016). Yet, our analysis suggests that those that are most affected by technological change are not actually in favor of such a policy approach, but would rather support more redistributive measures. On the one hand, this is understandable since individuals confronted with unemployment risk might simply opt for short-term compensation rather than long-term investment. On the other hand, the findings are worrying since they demonstrate that the political debate about the transformation of welfare states towards the social investment model might face political resistance.
Admittedly, our study has a number of limitations. For instance, the questions available in the ESS are either quite broad and vague (e.g. the measure of redistributive preferences) or worded in particular ways that delimit their broad applicability (e.g. the question on active labor market policy). Furthermore, the ESS is a cross-sectional survey that does not allow to trace the impact of technological change on how attitudes towards the welfare state change over time, as would be possible with panel data. Given the limitations of the available data, we could only focus on three possible policy responses. Although these cover important dimensions of welfare state policy-making, other policy reactions to technological change are imaginable. Also, the particular wording of the questions in the ESS creates the difficulty of comparing preferences in constrained (trade-off) scenarios with preferences in unconstrained settings, which should ideally be avoided. Furthermore, this paper just started the exploration of the role of cross-national differences in the institutional set-up of the welfare state in affecting the micro-level dynamics of attitude formation. More research is clearly needed here, e.g. using more fine-grained measures of institutional differences between welfare state models. Finally, our measures of automation risk are focused on the occupational rather than the individual level, thus not entirely ruling out that they capture inherent and idiosyncratic characteristics of occupations rather than individual automation risk. Against this background, this paper should be regarded as a mere first step in the broader research agenda on the study of the implications of technological change for the welfare state.
Competing interests
The authors declare none.
Supplementary Material
To view supplementary material for this article, please visit https://doi.org/10.1017/S0047279421000519