The speed and efficiency with which language processing takes place have been shown to be supported by linguistic prediction skills. Previous research has demonstrated that language users including (young) children and adults can predict the upcoming linguistic information based on several cues such as phonological information (e.g., DeLong et al., Reference DeLong, Urbach and Kutas2005), prosodic cues (e.g., Brown et al., Reference Brown, Salverda, Dilley and Tanenhaus2011), morphosyntactic information (e.g., Aumeistere et al., Reference Aumeistere, Bultena and Brouwer2022; Kamide, Altmann, et al., Reference Kamide, Altmann and Haywood2003; Kamide, Scheepers, et al., Reference Kamide, Scheepers and Altmann2003), discourse context (e.g., Van Berkum et al., Reference Van Berkum, Brown, Zwitserlood, Kooijman and Hagoort2005), and semantic cues such as thematic restrictions imposed by the verb (e.g., Altmann & Kamide, Reference Altmann and Kamide1999; Brouwer, Özkan, et al., Reference Brouwer, Özkan, Küntay, Blom, Cornips and Schaeffer2017, Reference Brouwer, Özkan and Küntay2019; Trueswell et al.,Reference Trueswell, Tanenhaus and Kello1993). Language users exploit such cues to pre-activate linguistic information on various levels of representation from the phonological (e.g., Allopenna et al., Reference Allopenna, Magnuson and Tanenhaus1998) to the semantic level (e.g., Huettig et al., Reference Huettig, Singh and Mishra2011; see Huettig, Reference Huettig2015 for a review).
Several studies investigated individual variation in linguistic prediction skills and found associations with aspects of language experience/proficiency in adults for language production (Martin et al., Reference Martin, Branzi and Bar2018), receptive vocabulary (Rommers et al., Reference Rommers, Meyer and Huettig2015), literacy (Favier et al., Reference Favier, Meyer and Huettig2021), and nativity (Reuter et al., Reference Reuter, Sullivan and Lew-Williams2021). Similar associations have been found in children between linguistic prediction abilities and input frequency (Garcia et al., Reference Garcia, Rodriguez and Kidd2021), language production (Brouwer, Sprenger, et al., Reference Brouwer, Sprenger and Unsworth2017), receptive vocabulary (Borovsky et al., Reference Borovsky, Elman and Fernald2012), productive vocabulary (Mani & Huettig, Reference Mani and Huettig2012), literacy (Mani & Huettig, Reference Mani and Huettig2014), and language dominance for bilingual children (Theimann et al., Reference Theimann, Kuzmina and Hansen2021). Besides linguistic skills, prior work has also found associations between prediction abilities and cognitive skills such as working memory (WM) capacity in adults (Huettig & Janse, Reference Huettig and Janse2016; Ito et al., Reference Ito, Corley and Pickering2018). A limited number of studies investigated the contributions of a broad set of linguistic and cognitive skills to adults’ linguistic prediction skills (e.g., Hintz et al., Reference Hintz, Meyer and Huettig2017).
The aim of the current paper is to assess whether receptive vocabulary, productive vocabulary, receptive grammar, language production skills, and three components of WM are modulating linguistic prediction in 4- to 8-year-old Turkish-speaking children. This study is novel because it is, to our knowledge, the first time that both verbal and cognitive skills are explored in relation to children’s linguistic prediction. In addition, linguistic prediction is investigated in a relatively less-studied language, that is, Turkish. What makes Turkish unique is that it is characterized by free word order and that it depends on case marking to infer sentence meaning such as “who did what to whom.” Previous research has shown that Turkish-speaking children are able to use case marking in combination with word order to achieve sentence comprehension starting from 2 years old (Göksun et al., Reference Göksun, Küntay and Naigles2008; Slobin & Bever, Reference Slobin and Bever1982). However, when case marking was lacking, 1- to 3-year-old Turkish-speaking children could not use word order cues to comprehend sentence meaning as efficiently as their English-speaking peers did (Candan et al., Reference Candan, Küntay, Yeh, Cheung, Wagner and Naigles2012).
Recently, Özge et al. (Reference Özge, Küntay and Snedeker2019) investigated the use of case marking during online sentence processing in Turkish-speaking 4-year-old children using two eye-tracking experiments. In Experiment 1, children heard sentences with verb-medial (subject–verb–object (SVO) and object–verb–subject (OVS)) word orders, while, in Experiment 2, they heard sentences with verb-final SOV and OSV word orders. The only cue that the children could use to predict the second noun in Experiment 2 sentences was case marking. The results showed that the children use nominative and accusative case markers to predict an upcoming argument both with (Experiment 1) and without (Experiment 2) the help of the verb’s meaning. The current study is a follow-up of Özge et al.’s (Reference Özge, Küntay and Snedeker2019) Experiment 2 but also extends it by (1) looking at children in a broader age range and (2) examining which verbal and cognitive skills of children predict anticipation of the second noun in Turkish verb-final sentences on the basis of case marking on the initial noun.
Contributions of verbal skills to linguistic prediction
Productive and receptive vocabulary skills
Vocabulary size and language processing skills appear to have a bidirectional association. Hurtado and colleagues (Reference Hurtado, Marchman and Fernald2008) found with Spanish-speaking 24-month-old toddlers that productive vocabulary size assessed by parental report and language processing efficiency (recognition of familiar words) measured by the looking-while-listening (LWL) paradigm (Fernald et al., Reference Fernald, Zangl, Portillo, Marchman, Sekerina, Fernandez and Clahsen2008) similarly meditated the effect of maternal speech at 18 months on one another. As such, a large vocabulary size appeared to enhance the processing of familiar words, and the efficiency with which familiar words were processed appeared to facilitate word learning.
A later study found that the effect of child-directed speech at 19 months on productive vocabulary assessed by parental report at 24 months was mediated by language processing efficiency at 19 months, indicating that those children with a high (productive) vocabulary size might be at a processing advantage (Weisleder & Fernald, Reference Weisleder and Fernald2013). This processing advantage is probably maintained across early childhood because early language processing efficiency at 25 months predicts later language outcomes at 8 years (Marchman & Fernald, Reference Marchman and Fernald2008). The language processing efficiency measured with LWL in these studies might reflect an underlying skill, for example, speed of lexical access, speed of access to long-term representations, or speed of orienting visual attention based on verbal input, which is quite likely exploited during linguistic prediction. Indeed, a recent study suggested that the roles of prediction and word recognition skills in language development might overlap by showing that these skills similarly predicted subsequent vocabulary growth within 5–10 months (Gambi et al., Reference Gambi, Jindal, Sharpe, Pickering and Rabagliati2021). Vocabulary skills, on the other hand, might be a proxy for such differences in processing efficiency. Based on these assumptions, a high vocabulary score (therefore a processing advantage) might longitudinally and concurrently predict a high level of linguistic prediction skill. These associations are investigated in the current study.
Both receptive and productive vocabulary skills were found to be associated with linguistic prediction across early childhood. Mani and Huettig (Reference Mani and Huettig2012) showed that children as young as 2 years could predict the upcoming noun in German sentences based on the semantic restrictions imposed by the verb. This skill was correlated with the children’s productive vocabulary but not with their receptive vocabulary, both assessed by parental report. Mani and colleagues (Reference Mani, Daum and Huettig2016) expanded this finding by showing that parentally reported productive vocabulary skills of 2-year-old German-speaking children were correlated with their prediction skills both when the target word is strongly and weakly associated with the preceding verb (e.g., reading a book vs. a letter). However, productive vocabulary might no longer be predicting linguistic prediction skills of children older than 2 years. For instance, the productive vocabulary skills of 3- to 5-year-old English-speaking children, which were measured by the children’s verbal responses to stimuli pictures, were not associated with their anticipatory looks to the target cued by verb semantics (Gambi et al., Reference Gambi, Pickering and Rabagliati2016).
On the other hand, Borovsky et al. (Reference Borovsky, Elman and Fernald2012) found that English-speaking 3- to 10-year-old children were able to integrate the semantic information from the subject noun and the verb to predict the upcoming object noun, with receptive vocabulary directly assessed as a correlate of their prediction skills. Child-assessed receptive vocabulary was also associated with 3- to 10-year-old English-speaking children’s linguistic predictions based on the integration of inferred talker identity and action (e.g., an implied pirate vs. a princess identity talking about holding a sword vs. a wand; Borovsky & Creel, Reference Borovsky and Creel2014). A recent study, however, showed that 2- to 5-year-old English-speaking children’s receptive vocabulary skills, which were assessed by testing the children, were associated with their revision of erroneous predictions but not with their prediction skills, once age was controlled for (Gambi et al., Reference Gambi, Jindal, Sharpe, Pickering and Rabagliati2021). A subsample of children in this study was found to have predictive associations between their word recognition and prediction skills (Time 1 mean age: 42 months, range: 25–60 months) and their subsequent receptive vocabulary growth within the next 5–10 months (Time 2 mean age: 50 months, range: 34–68 months). This longitudinal association provides support for the link between linguistic processing efficiency and the development of language skills.
Grammar skills
Despite clear evidence for the association of vocabulary skills with linguistic prediction based on lexical (verbal) semantics, data regarding the prediction ability based on structural cues are sparse and not as robust. Although two-and-a-half-year-old English-speaking children were able to use grammatical number on agreeing verbs to anticipate the upcoming targets in interrogative sentences (e.g., where are the good cookies vs. where is the good apple), this prediction skill was not associated with the children’s productive vocabulary skills (Lukyanenko & Fisher, Reference Lukyanenko and Fisher2016).
Another study with Czech-speaking 23-month-old children found that only the group of children with high receptive grammar skills could anticipate the upcoming target based on the grammatical gender markers of the preceding demonstrative pronoun and adjective, while the group of children with high receptive vocabulary skills did not show evidence for linguistic prediction (Smolík & Bláhová, Reference Smolík and Bláhová2019). This study indicated that vocabulary skills alone might be insufficient to propel linguistic prediction based on structural (e.g., morphosyntactic) elements. A high level of grammar skills appears to also be required.
Language production skills
Brouwer, Sprenger, et al. (Reference Brouwer, Sprenger and Unsworth2017) drew a similar conclusion by showing that children needed to systematically produce gender-marked articles in Dutch to be able to use those articles as cues to linguistic prediction. Four- to seven-year-old children in this study were divided into two groups based on their elicited production scores of the Dutch neuter article het. Although the children had high receptive vocabulary skills overall, only those children who showed adult-like performance in elicited productions of the article could predict the upcoming object on the basis of the preceding article. Systematic production of a grammatical structure arguably requires a firm comprehension of it, implying the importance of both language production and grammar skills for linguistic prediction based on structural cues. In the present study, we investigate the roles of grammar and language production skills, as well as receptive and productive vocabulary skills in children’s linguistic prediction.
Contributions of WM components to linguistic prediction
There is also evidence for the role of WM in linguistic prediction. Huettig and Janse (Reference Huettig and Janse2016) found that Dutch-speaking adults’ anticipatory eye movements to the nouns that matched with the preceding gender-marked articles were associated with their WM. In this study, the WM construct was derived by a principal component analysis, using phonological short-term memory (STM), visuospatial STM, and WM capacity measured by backward digit span as underlying skills. The authors suggested WM as a mediating factor for linguistic prediction because of its role in linking short-term linguistic and visual representations, thus “grounding language in space and time.” A recent study by Ito and colleagues (Reference Ito, Corley and Pickering2018) found causal evidence for the involvement of WM during linguistic prediction. In this study, first language (L1) and advanced second language (L2) speakers of English predicted an upcoming noun based on the semantic restrictions imposed by the verb (e.g., higher fixation proportions to the scarf following the verb fold vs. find). The anticipatory eye movements were delayed similarly for L1 and L2 groups when the participants were simultaneously required to remember a five-word list. This latter finding was argued to imply a shared cognitive resource for WM and linguistic prediction.
Collectively, those two studies indicate WM as a source of individual variation for linguistic prediction skills, although the mechanism that propagates this association is unclear. Linguistic prediction arguably requires keeping the incoming linguistic information in mind (i.e., phonological STM), manipulating the cues such as verb semantics and/or structural markers (i.e., central executive), and integrating information from WM contents with long-term memory representations (i.e., episodic buffer).
The current study
This study investigates the contributions of verbal skills (i.e., earlier measure of productive vocabulary and concurrent measures of receptive grammar, receptive and productive vocabulary, and language production) and three components of Baddeley’s (Reference Baddeley2007) WM model (i.e., phonological STM, central executive, and episodic buffer; following Boyle et al., Reference Boyle, Lindell and Kidd2013) to Turkish-speaking 4- to 8-year-old children’s linguistic prediction on the basis of case marking. We aim to explore whether verbal skills and WM components are associated with the prediction skills of the children by using a visual world eye-tracking paradigm (VWP; Cooper, Reference Cooper1974; Tanenhaus et al., Reference Tanenhaus, Spivey-Knowlton, Eberhard and Sedivy1995). As in Experiment 2 of Özge et al. (Reference Özge, Küntay and Snedeker2019), the current experiment involved verb-final sentences that followed SOV and OSV orders (e.g., Hızlı tavşan nominative birazdan şuradaki havucu accusative yiyecek “The fast rabbit will soon eat the carrot over there” and Hızlı tavşanı accusative birazdan şuradaki tilki nominative yiyecek “The fox over there will soon eat the fast rabbit”), which were accompanied by three-object visual scenes representing the referent of the first noun (e.g., rabbit), a plausible agent that could perform an action on the referent of the first noun (e.g., the fox eats the rabbit), and a plausible patient that could undergo an action performed by the referent of the first noun (e.g., the rabbit eats the carrot).
We expected to find a positive association between the children’s early productive vocabulary scores and their prediction skills. We also conjectured that receptive and productive vocabulary and receptive grammar skills might contribute to the prediction skills of the children because (1) strong vocabulary skills were associated with strong lexical representations that were thought to be easily retrieved from the long-term memory (Mani & Huettig, Reference Mani and Huettig2014) and easily processed (Fernald et al., Reference Fernald, Perfors and Marchman2006) and (2) the morphosyntactic prediction task administered in the current study required grammatical competence, unlike other studies that assessed prediction based on semantic cues (Borovsky et al., Reference Borovsky, Elman and Fernald2012). In addition, we expected to find a positive association between children’s language production and prediction skills (Brouwer, Sprenger, et al., Reference Brouwer, Sprenger and Unsworth2017; Martin et al., Reference Martin, Branzi and Bar2018). Finally, we anticipated that the components of WM would be differently associated with the prediction skills of children. We expected that the episodic buffer might particularly be related to linguistic prediction skills measured in the current study because of its linking function between the WM components and long-term representations (Baddeley, Reference Baddeley2012). Phonological STM and the central executive components might also be involved in linguistic prediction because of their storage and control functions, respectively. Nevertheless, such functions might be more important for processing complex or ambiguous sentences compared to the simple transitive structures used in the current study (Kidd, Reference Kidd2013).
Method
Data, the analysis script, model outputs, and research materials are available at https://osf.io/qtcf3/.Footnote 1
Participants
The sample of the current study was selected among those children whose parents reported on a vocabulary checklist, Turkish Communicative Development Inventory (TCDI; Aksu-Koç et al., Reference Aksu-Koç, Acarlar, Küntay, Maviş, Sofu, Topbaş, Turan and Aktürk-Arı2019), which was the Turkish adaptation of the MacArthur-Bates Communicative Development Inventory (Fenson et al., Reference Fenson, Marchman, Thal, Dale, Reznick and Bates2007), when the children were between 8 and 36 months old (M = 23.3, SD = 9.4).Footnote 2 Other criteria for the children’s inclusion in the sample were using Turkish as their first language, residing in Istanbul, Turkey, not having any known developmental problems, and not having any hearing or vision problems.
We recruited 78 children. However, two children could not complete the eye-tracking task due to technical problems (N = 1) and the child’s reluctance to participate (N = 1). The final sample consisted of 76 children (47.4 % female) with an age range of 49–102 months (M = 72.3, SD = 16.0). We focused on this age range to (1) cover a similar range with previous studies (e.g., Borovsky et al., Reference Borovsky, Elman and Fernald2012) and (2) recruit as many participants as possible from the original TCDI sample. There were missing data in all child assessments in this sample due to experimenter error (N = 11), the child’s reluctance to participate (N = 5), or the family’s unavailability for a home visit (N = 12). The sample size for each assessment is indicated in the Measures section.
Auditory and visual material
The VWP eye-tracking experiment used in the current study was the same as Experiment 2 in Özge et al. (Reference Özge, Küntay and Snedeker2019). The auditory stimuli involved experimental, practice, and filler items. The experimental items were verb-final transitive Turkish sentences with agent (action doer) and patient (action undergoer) realized as overt arguments. They differed by the case marker on the head of the first noun phrase (NP1) of the sentence. In the nominative condition, NP1 was marked with the nominative case, which identified it as the agent of the action. In the accusative condition, NP1 was marked with the accusative case, which identified it as the patient of the action.Footnote 3 Thus, the sentences in the nominative condition followed the subject–object–verb (SOV) order, while the sentences in the accusative condition followed the object–subject–verb (OSV) order, as represented in (1) and (2), respectively. The practice and filler items were intransitive verb-final sentences with complex NP subjects, such as (3).



The experiment began with three practice items and involved 10 experimental sentences (five in the nominative and five in the accusative condition), with 10 filler items interspersed to mask the purpose of the experiment. The experimental sentence pairs, such as in (1) and (2), were sorted into two counterbalanced lists, in which the same practice and filler items were used. Two experimental items from the same condition were always intervened by two filler items, with an experimental item from the other condition in between (e.g., nominative item – filler item – accusative item – filler item – nominative item).
The sentences of the experiment were recorded by a female native speaker of Turkish with a natural prosody. The sound files were manipulated by inserting a 200-ms silence at the beginning and a 1500-ms silence at the end of each sentence. For the experimental items, a 300-ms pause was added before the adverbial birazdan “soon” and a 200-ms pause was added before the modifier şuradaki “there” to adjust the prosodic breaks.
Each sentence was accompanied by a visual scene with three referents (Figure 1), which were depicted as colored line drawings at a resolution of 640 × 480 pixels. For the experimental items, one of the pictures represented the first noun (i.e., the topic rabbit). The other two pictures represented a plausible agent (e.g., fox), which could potentially perform an action on the topic, and a plausible patient (e.g., carrot), which could potentially undergo an action performed by the topic. For the practice and filler items, two of the pictures represented the two nouns in the subject NP (e.g., woman and egg), while the third picture represented an irrelevant distractor (e.g., gardener).

Figure 1. A Sample Visual Display for the Experimental Sentences Exemplified in (1) and (2).
Individual difference assessments
The individual difference assessments that were used in the current study consisted of a set of measures that assessed verbal skills and a set of measures that assessed performance in WM components. All measures were based on child assessments except for TCDI, which was assessed by maternal report. Raw scores were used for all measures except for TCDI, for which we used the percentile scores because the children’s early productive vocabularies were assessed by using age-appropriate forms (i.e., TCDI-I form for children between 8 and 16 months, TCDI-II form for children between 16 and 36 months at the time of assessment).
Verbal skills assessments
Early productive vocabulary
Early productive vocabulary was assessed by TCDI (Aksu-Koç et al., Reference Aksu-Koç, Acarlar, Küntay, Maviş, Sofu, Topbaş, Turan and Aktürk-Arı2019). TCDI had two forms for different age ranges (TCDI-I for 8–16 months, TCDI-II for 16–36 months). It included maternally reported items for the children’s early receptive (only TCDI-I) and productive vocabularies (both TCDI-I and TCDI-II), comprehension and production of gestures (only TCDI-I), and grammatical development (only TCDI-II; e.g., complexity of sentence structures produced). The current study involved children who either participated in TCDI-I or TCDI-II. Because the two forms had separate age-appropriate items for assessing the children’s productive vocabulary, we used the early productive vocabulary percentile scores based on age and gender norms, collapsed across two forms (henceforth collectively referred to as TCDI). This measure had an observed range between 5 and 100 (N = 72, M = 64.8, SD = 27.4).
Receptive grammar
Receptive grammar was assessed by a Turkish translation of the Test for Reception of Grammar (TROG-2; Bishop, Reference Bishop2003). The test involved 20 blocks of four items, each block measuring the comprehension of a specific type of grammatical structure (e.g., passive sentences). The children listened to the prerecorded sentences as they looked at four pictures on the computer screen, and they picked the picture that depicted the sentence that they heard. The stimuli were presented using E-Prime 2.0 software (Psychology Software Tools, Pittsburgh, PA). The experimenter recorded the children’s responses by pressing a number key that corresponded to the picture pointed by the child. E-Prime program automatically calculated the accuracy of the responses. The test was terminated if the child failed on five consecutive blocks. The receptive grammar score was calculated as the total number of blocks successfully passed, where the child was required to respond correctly to all four items to pass a block. This test had an observed range between 0 and 15 (N = 75, M = 6.9, SD = 4.1).
Receptive and productive vocabulary
The receptive and productive subscales of the Turkish Productive and Receptive Language Test (TIFALDI; Berument & Güven, Reference Berument and Guven2013) were used to assess the receptive and productive vocabulary scores of the children. TIFALDI is an adaptive test, which is applicable to children between 2 and 12 years old. The children started the test with age-appropriate items. Based on their performance, they were tested on simpler items until a basal score was set by eight consecutive correct items. The receptive test included 104 items and was terminated if the child failed in eight items within a set of 10 questions. The productive test included 80 items and was terminated if the child failed in six items within a set of eight questions. In the receptive test, the children were asked to pick the picture that correctly represented the word that they heard out of a four-picture representation. In the productive test, the children were asked to name the picture that they were shown. The experimenter recorded the responses of the children. Raw scores were used for both tests. The receptive test had an observed range between 30 and 103 (N = 62, M = 84.0, SD = 15.4). The productive test had an observed range between 31 and 78 (N = 63, M = 60.7, SD = 11.8).
Language production
The language production task required the children to produce continuations for sentence fragments based on accompanying three-object visual scenes. The same female speaker in the eye-tracking experiment recorded the sentence fragments, which were derived by using the same experimental and filler items in this experiment. The sentence fragments involved the adverbial and the first noun (e.g., birazdan tavşan… “soon the rabbit…”). The children were expected to provide a grammatical continuation for those fragments by relating them to the visual scenes that were simultaneously presented. In order to circumvent an unlikely recollection of the exact items from the lab session, the children were provided with the alternative list of items (i.e., if a child received List 1 in the eye-tracking experiment, this child received List 2 in the language production assessment). The stimuli were presented using E-Prime 2.0 software (Psychology Software Tools, Pittsburgh, PA). The experimenter recorded the children’s responses, which were later coded with respect to their grammaticality and appropriateness to the visual scene. The correct answers received a score of 1, and the incorrect answers received a score of 0. There were 10 experimental items. This assessment had an observed range between 2 and 10 (N = 64, M = 7.6, SD = 2.2).
WM assessments
Phonological short-term memory
Phonological STM was assessed by a forward digit span test, which required the children to listen to a series of digits and report them back in the given order. The test included seven blocks of digit sets that progressively increased in length. The first block involved three digits and the last block involved nine digits. There were three items within each block. The experimenter read aloud the digits for the children by inserting an approximately 1 s break between each digit and recorded the children’s responses for each item. The test began with a two-digit practice trial, which was repeated for a maximum of three times if the child failed to give the correct response. Feedback and encouragement were provided during the practice trial only. The test was terminated if the child failed in all three items within a given block. The child’s score was the digit length of the block preceding the termination of the test (e.g., if the test was terminated at the block with five-digit items, the child’s score became four). This test had an observed range between 3 and 7 (N = 73, M = 4.2, SD = 1.1).
Central executive
Central executive skills of the children were measured by a backward digit span test, which required listening to a series of digits and reporting them back in the reverse order. The backward digit span test is suggested as an appropriate measure of central executive skills because of its requirement for storage and processing (Boyle et al., Reference Boyle, Lindell and Kidd2013). The test included eight blocks of digit sets that progressively increased in length. The first blocks involved two digits and the last block involved nine digits. There were three items within each block. The implementation and scoring of this test were the same as the forward digit span. Before the practice trial began, the experimenter modeled the backward reporting requirement of the test with another experimenter to facilitate the children’s comprehension of the task. This test had an observed range between 0 and 6 (N = 70, M = 2.5, SD = 1.5).
Episodic buffer
A sentence repetition task was administered to measure episodic buffer (Aksu-Koç et al., Reference Aksu-Koç, Erguvanlı-Taylan and Bekman2002). Alloway et al. (Reference Alloway, Gathercole, Willis and Adams2004) showed with confirmatory factor analysis that this task required a separate skill than the skills that short-term storage and central executive tasks called for and concluded that it tapped the episodic buffer component in Baddeley’s (Reference Baddeley2000) WM model. The sentence repetition task used in the current study included 20 items that progressively became more complex in length and grammatical structure. The experimenter read aloud the sentences one by one to the children, who were required to provide verbatim repetitions instantly. We adopted the coding scheme of Boyle et al. (Reference Boyle, Lindell and Kidd2013) to evaluate the children’s responses. The first four items were simple sentences with a length of two to four words. For these items, verbatim repetitions received two points, repetitions with one error received one point, and repetitions with more errors received zero points. The remaining items were complex sentences with a length of four to six words, except for one item, which was a five-word simple sentence. For all these items, verbatim repetitions received three points, repetitions with one error received two points, repetitions with two and three errors received one point, and repetitions with more errors received zero points. The child’s score was the sum of the scores that were obtained from all items (maximum points that a child could get were 56). This assessment had an observed range between 28 and 56 (N = 72, M = 52.7, SD = 5.2).
Procedure
Informed consents were received from the parents of the children. The data were collected through direct assessments of the children in the Language and Communication Development Lab at Koç University and during home visits, respectively. The assessment procedure lasted 1.5–2 hr in total, half of which was conducted in one of the respective settings. The eye-tracking experiment, the WM assessments, and the receptive grammar test were administered during the lab sessions. The receptive and productive vocabulary tests and the language production assessments were administered during home visits, in the given order. The lab and home visit sessions occurred within 2 weeks of each other. The TCDI data were collected through in-person interviews with the mothers or through a checklist completed by the mothers, depending on their educational level (M = 12.5 years of completed education, SD = 4.9).
We used a Tobii T20 eye tracker at 60 Hz sampling rate to record the children’s eye movements. The stimuli were presented using E-Prime 2.0 software (Psychology Software Tools, Pittsburgh, PA). The children were seated in front of the eye tracker screen with an approximately 60-cm distance. We used a 9-point calibration procedure to map the eye orientation before the experimental procedure began. The children were asked to listen to the sentences as they naturally viewed the accompanying pictures, during which their eye movements were recorded. At the end of each item presentation, the children watched a short animation and were asked to respond if this correctly depicted the event that they had heard. The experimenter recorded the children’s responses. The purpose of the animations was to keep the children engaged in the task. Therefore, these responses were not included in the analyses. Following the animation slide, the experiment proceeded automatically to the next item once the children successfully fixated the inter-stimulus fixation circle.
Data analysis
We used generalized linear mixed-effects models with a logit link function, using glmer function in lme4 software package (Bates et al., Reference Bates, Mächler, Bolker and Walker2015) in R version 4.0.4 (R Core Team, 2021). The aims of the analyses were to investigate (1) the size of the prediction effect; (2) the verbal (early productive vocabulary, receptive grammar, receptive and productive vocabularies, and language production), and (3) WM (phonological STM, central executive, and episodic buffer) predictors of the prediction effect. Our binary outcome variable was agent fixations. We computed this variable by coding the fixations on the referent of the potential agent (e.g., fox) as 1 and the fixations on the referents of the potential patient (e.g., carrot) and the topic noun (e.g., rabbit) as 0. In the nominative condition, an increase in potential patient fixations is expected, whereas in the accusative condition, an increase in potential agent fixations is expected. The fixed effects in our models were Time (50-ms bins), Condition (nominative vs. accusative), Age (in months) as a covariate, and the individual differences measures. We centered and scaled the continuous predictors Time and Age for model convergence (Baguley, Reference Baguley2012). For the categorical predictor Condition, the nominative condition was set to reference level.
The prediction effect was conceptualized as a significant increase in agent fixations over Time as a function of Condition (i.e., a significant Time-by-Condition interaction). A significantly higher increase in agent fixations in the accusative compared to the nominative condition over time would mean that the participants showed the prediction effect. The contribution of each individual difference variable to the prediction effect was estimated in separate models because of convergence problems. The individual difference variables were added to the prediction effect as interaction terms (i.e., a three-way interaction between Time, Condition, and the individual difference variable). Age was controlled for in all models as a main effect due to (1) its significant correlations with all concurrent child assessments (r ranging between .37 and .67, p < .01) and (2) its significant effect on agent fixations (see the Results section), resulting in a model structure with a three-way interaction between Time, Condition, and the individual difference variable and the covariate Age added as a main effect.
The random effects structure of the models included by-participant and by-item random intercepts and random slopes for Condition, as well as correlations between random intercepts and random slopes (Barr et al., Reference Barr, Levy, Scheepers and Tily2013). We simplified the random effect structure when a model did not converge first by suppressing the correlation parameters. If this strategy did not work, we removed the random slopes for Condition from the model.
We preprocessed the data prior to the analyses by removing blinks and gaze recordings between stimuli presentations. The blinks involved those instances where the participants were not fixating any of the three referents on the eye-tracker screen during stimuli presentation (i.e., true blinks and off-screen fixations; M = 15.68%).
The data onset was time-locked to NP1 offset, where the case marker cue became perceptually available, and the data were aggregated into 50-ms bins to account for the non-independence of individual gaze samples (Barr, Reference Barr2008). The data to be analyzed were restricted to the prediction window that encompassed the time between NP1 offset till NP2 onset. We offset this window by 200 ms to account for saccade latency (Salverda et al., Reference Salverda, Kleinschmidt and Tanenhaus2014). In order to detect outlier trials, we investigated the number of observations for each subject and trial in this time window. We removed those trials for which a given subject had 3 SDs below or above the mean number of observations (M = 91.03, SD = 18.16). This computation resulted in the removal of 17 trials in total (0.51% data loss). Some participants had already zero number of observations for some trials (seven in total). We did not remove any of the participants from the analyses because our algorithm for outlier removal did not result in the removal of 50% or more trials for any of the participants.
Results
Data, the analysis script, and the outputs of all models presented in the Results section are provided through https://osf.io/qtcf3/.
Table 1 shows the age-controlled partial correlations of child assessments. Figure 2 shows the agent fixations in the accusative and the nominative conditions for the entire presentation, starting from the 200-ms silence prior to the adjective hızlı “fast” that modifies NP1 until verb offset.
Table 1. Age-controlled partial correlations of child assessments

Note. *p < .05. **p < .01. ***p < .001.

Figure 2. Agent Fixations in the Accusative (Black Curve) and the Nominative Condition (Grey Curve) During Stimuli Presentation.
The model with the fixed effects of Time, Condition, and their interaction revealed a significant interaction between Time and Condition (estimate = 0.073, SE = 0.036, z = 2.016, p = .044). This model indicated that the increase in agent fixations was significantly larger in the accusative than in the nominative condition. When added to this model as a fixed effect, Age was found to be significantly and positively associated with agent fixations (estimate = 0.080, SE = 0.035, z = 2.300, p = .022), indicating that the older children had a higher proportion of agent looks than the younger children. Hence, it was added to the subsequent models as a covariate.
Verbal measures as predictors of children’s prediction skills
Table 2 shows the contribution of each verbal measure to the children’s prediction skills (i.e., the size of the prediction effect). We report only the three-way interactions between Time, Condition, and each verbal measure because the lower order effects were not informative for individual differences (i.e., it was the three-way interactions that informed us about the contribution of individual measures to the prediction effect, which itself was conceptualized as Time-by-Condition interaction).
Table 2. Statistics for the three-way interactions from separate models that estimated the contribution of five different verbal skills to the prediction skill

Note. The models presented here had the following structure: glmer(DV ∼ Condition * Time * IndividualDifferenceMeasure + Age + (Condition|Participants) + (Condition|Items), family = binomial(link-logit). The random effect structure was simplified if needed for model convergence. The covariate Age was a significant predictor only in the models with receptive and productive vocabulary. TCDI represents early productive vocabulary.
Among the verbal predictors, only early productive vocabulary and language production skills were found to significantly contribute to the prediction effect. Figure 3 shows the increase in agent fixations in both conditions for the first and the third quartiles of the centered and scaled early productive vocabulary (TCDI) scores (Gambi et al., Reference Gambi, Jindal, Sharpe, Pickering and Rabagliati2021). The children in the third quartile exhibited a larger difference in agent fixations between the two conditions and they started showing the prediction effect earlier, compared to the children in the first quartile. Nevertheless, the children in the first quartile had a higher and steeper increase in agent fixations for both conditions, compared to the children in the third quartile.
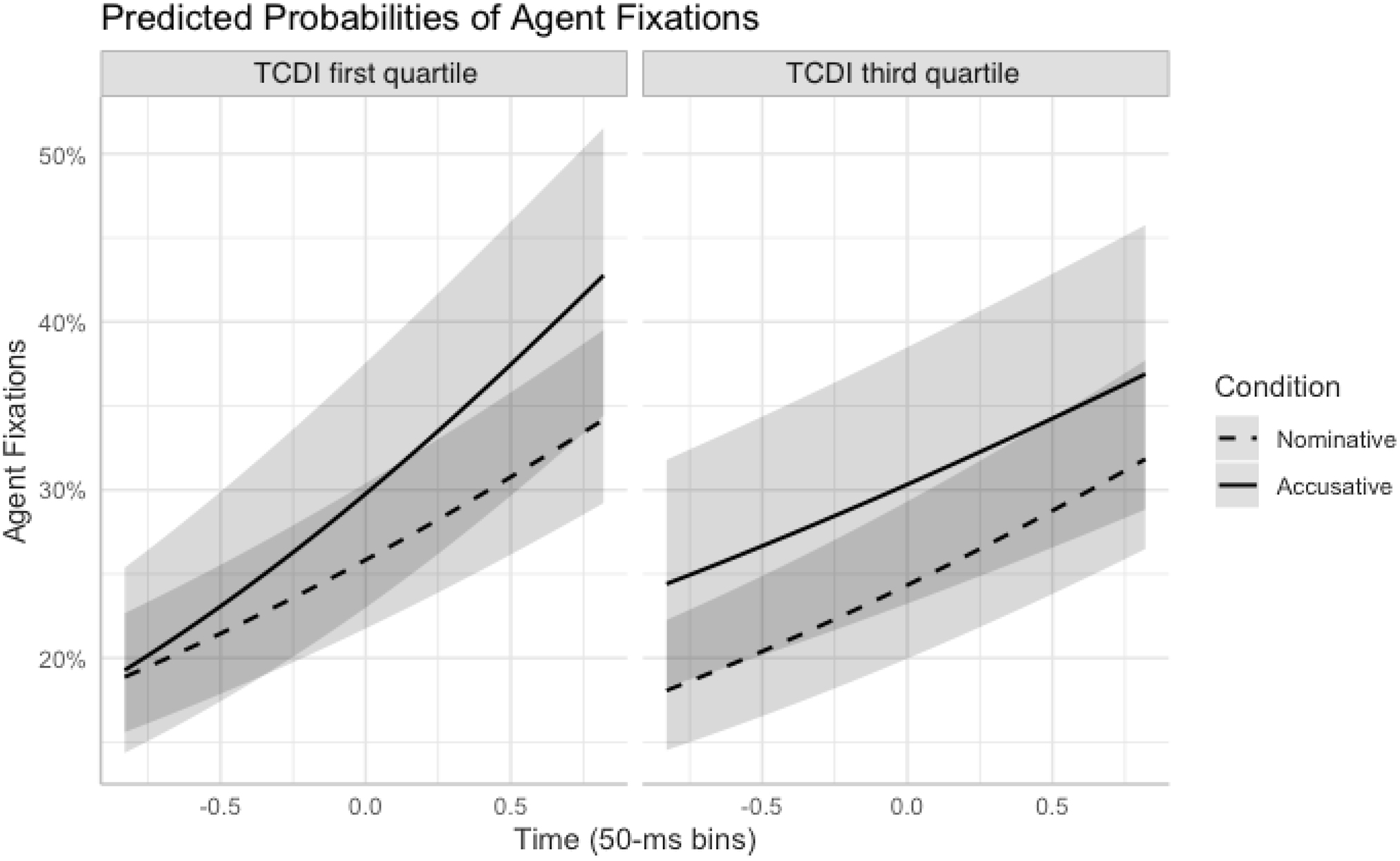
Figure 3. Increase in Agent Fixations in the Accusative and the Nominative Conditions for the Children in the First and the Third Quartiles of the Earlier Productive Vocabulary (TCDI) Scores as Outputted by the Model.
Figure 4 shows the increase in agent fixations in both conditions for the first and the third quartiles of the centered and scaled language production scores. The children in the third quartile exhibited a larger difference in agent fixations between the two conditions compared to the children in the first quartile, indicating a larger prediction effect. They also showed a steeper increase in agent fixations in the accusative condition compared to the nominative condition and compared to the children in the first quartile. As such, the prediction effect increased over time for the children in the third quartile but not for the children in the first quartile. The children in the first quartile did not display much difference in agent fixations between the two conditions.

Figure 4. Increase in Agent Fixations in the Accusative and the Nominative Conditions for the Children in the First and the Third Quartiles of the Language Production Scores as Outputted by the Model.
WM components as predictors of children’s prediction skills
Table 3 shows the contribution of separate WM components to the prediction skill. We reported again only the three-way interactions between Time, Condition, and each WM component because the lower order effects were not informative for individual differences.
Table 3. Statistics for the three-way interactions from separate models that estimated the contribution of three different WM components to the prediction skill

Note. The models presented here had the following structure: glmer(DV ∼ Condition * Time * IndividualDifferenceMeasure + Age + (Condition|Participants) + (Condition|Items), family = binomial(link-logit). The random effect structure was simplified if needed for model convergence. The covariate Age was a significant predictor only in the model with episodic buffer.
Among the WM components, only the episodic buffer measure was found to significantly contribute to the prediction effect. Figure 5 shows the increase in agent fixations in both the nominative and accusative conditions for the first and the third quartiles of the centered and scaled episodic buffer scores. The children in the third quartile exhibited a larger difference in agent fixations between two conditions compared to the children in the first quartile, indicating a larger prediction effect. They also showed a steeper increase in agent fixations in the accusative condition compared to the nominative condition and compared to the children in the first quartile. Moreover, the prediction effect increased over time for the children in the third quartile but not for the children in the first quartile. The children in the first quartile did not display much difference in agent fixations between the two conditions.

Figure 5. Increase in Agent Fixations in the Accusative and the Nominative Conditions for the Children in the First and the Third Quartiles of the Episodic Buffer Scores as Outputted by the Model.
Discussion
In this study, the contribution of several verbal skills and WM components to the prediction skills of 4- to 8-year-old Turkish-speaking children was investigated. The results demonstrated four main findings. First of all, our results revealed a significant overall morphosyntactic prediction effect. This finding replicated previous work with 4- to 5-year-old Turkish children (e.g., Özge et al., Reference Özge, Küntay and Snedeker2019).
Secondly, among the verbal predictors, the early productive vocabulary skills were found to significantly contribute to the children’s prediction skills. This finding was in line with our expectations that the children who had larger productive vocabularies when they were between 8 and 36 months would show a stronger and earlier prediction effect than children who had smaller productive vocabularies. Extending Marchman and Fernald’s (Reference Marchman and Fernald2008) finding that early processing efficiency predicted later language outcomes, the current study suggests that those children whose early productive vocabulary skills were relatively high probably maintained a processing advantage, which provided them with better and faster prediction skills than the children whose early productive vocabulary skills were relatively low. Thus, this finding confirmed that early language skills might play a role in the development of the prediction skills. Early high vocabulary skills probably mean a processing advantage, either because having a large vocabulary would facilitate processing or because a large vocabulary is already a proxy for better processing skills, and better processing skills may directly contribute to the development of the prediction skills (see Huettig & Janse, Reference Huettig and Janse2016 for evidence that processing speed contributes to prediction skills). The mechanisms through which early language skills might be associated with later prediction skills merit further investigation.
Thirdly, the language production skills, another verbal ability, were also a significant predictor of linguistic prediction. As expected, the children with low language production skills exhibited a smaller prediction effect than the children with high language production skills. Earlier studies also found an association between language production and prediction skills. For instance, Brouwer, Sprenger, et al. (Reference Brouwer, Sprenger and Unsworth2017) proposed that it was not until children could systematically produce a structure (e.g., gender-marked articles) that they could utilize it as a cue for linguistic prediction. Our finding lent support to this proposal and to the theories that put emphasis on the relation between language production and prediction (Pickering & Gambi, Reference Pickering and Gambi2018). One important aspect of the language production assessment used in the current study was that it focused on the utilization of the nominative and the accusative cases for producing the target utterances. As such, the current finding indicated that those children who could correctly use the case markers in their productions were adept at using them as cues for predicting the upcoming referents in the sentence.
Finally, among the WM components, the episodic buffer was, as expected, a significant predictor of linguistic prediction. The episodic buffer is proposed to be a buffer store, where binding of information from different streams takes place (Baddeley & Hitch, Reference Baddeley and Hitch2019), specifically linking WM components with one another, WM contents with perception, and WM contents with long-term representations (Baddeley, Reference Baddeley2012). Among these associations, the sentence repetition task assesses the binding of short-term auditory information with long-term representations (Alloway et al., Reference Alloway, Gathercole, Willis and Adams2004). Nevertheless, the relation between the children’s episodic buffer and prediction skills in the current study also lent support to Huettig and Janse’s (Reference Huettig and Janse2016) argument that linguistic prediction required linking of short-term linguistic and visual representations by WM.
Our final main finding, therefore, suggested that the children with high episodic buffer scores could efficiently integrate what they had heard with their long-term linguistic and semantic representations. Such efficient integration probably facilitated the interpretation of the case on the first noun, the assignment of a correct role to it, and finally the prediction of the upcoming argument. Thus, in line with Boyle et al. (Reference Boyle, Lindell and Kidd2013), which also found in a picture-pointing task with 4- to 6-year-old English-speaking children that only the episodic buffer performance was significantly associated with the children’s offline comprehension of the non-canonical sentences (i.e., passives and object relative clauses), the current finding supported an experience-dependent explanation of the association of language comprehension with WM. This approach argues that the association of WM measures and language comprehension is due to the shared variance in long-term linguistic knowledge, while language comprehension is probably further constrained by domain-general learning mechanisms such as statistical learning (Kidd et al., Reference Kidd, Donnelly and Christiansen2018; MacDonald & Christiansen, Reference MacDonald and Christiansen2002).
Collectively, our findings provide support for the multiple-mechanisms account of linguistic prediction, which suggests that more than one factor might drive linguistic prediction (Huettig, Reference Huettig2015). Huettig suggests that linguistic prediction minimally requires four mechanisms, including production-based, association-based, combinatorial-based, and simulation-based prediction mechanism. Production-based mechanism drives linguistic prediction by providing production representations. Association-based mechanism is involved in the pre-activation of the upcoming linguistic material through priming information at various levels (e.g., phonological and semantic). Combinatorial-based mechanism helps developing higher order meaning by combining sequential linguistic information. Finally, event simulation-based mechanism uses heuristics to pre-activate linguistic (and non-linguistic) representations. Importantly, these mechanisms may work in an interactive manner.
Among these mechanisms, our findings highlight the importance of the production-based prediction mechanism. It appears that the children’s ability to systematically use case markers in their utterances enables them to predict the upcoming sentential content based on these markers, probably through providing the comprehension system with well-specified production representations to pre-activate linguistic input. This assumption is based on the premise that language comprehension and production systems are interlaced, which underlies the capacity of linguistic prediction (Pickering & Garrod, Reference Pickering and Garrod2013).
Huettig (Reference Huettig2015) further proposes mediating factors of linguistic prediction, including WM and processing skills. The current findings provide direct support for the role of WM in linguistic prediction and expand Huettig and colleagues’ original conceptualization of WM as connecting visual and linguistic information with time and with one another (e.g., Huettig & Janse, Reference Huettig and Janse2016) by adding the function of connecting short-term and long-term representations. Our finding that early productive vocabulary skills predict linguistic prediction also lends indirect support for the role of processing skills in linguistic prediction if the assumption that early vocabulary skills represent underlying processing capacity is correct.
Our analyses disconfirmed the predictions that receptive grammar and receptive and productive vocabulary skills would be associated with the prediction skills. These null results were surprising given the significant associations found by previous studies (e.g., Borovsky & Creel, Reference Borovsky and Creel2014; Mani & Huettig, Reference Mani and Huettig2012). Nevertheless, some recent studies revealed that variation in morphosyntactic prediction skills was not related to vocabulary size of the children (e.g., Lukyanenko & Fisher, Reference Lukyanenko and Fisher2016; Smolík & Bláhová, Reference Smolík and Bláhová2019). As such, our null results might be explained by a consideration of the requirements of the type of prediction measured in the current study. In the current prediction task, the participants needed to detect the case of the first noun, assign a (thematic) role to it accordingly (subject/agent or object/patient), and predict the upcoming sentential content, all the while reconciling their concurrent and long-term linguistic representations, as well as the auditory and visual information that they received. Those operations might be supported by lexical and grammatical skills, but such skills were probably ineffective in explaining variation in prediction based on morphosyntactic cues.
Furthermore, it is hard to directly compare the current study to previous studies that investigated individual differences in linguistic prediction because these studies have used samples with different age ranges, different sample sizes, different languages, and different statistical analyses. The previous individual difference studies have also not all shown the same individual differences effects. For example, Mani and Huettig (Reference Mani and Huettig2012) did not find a relation between comprehension vocabulary and prediction skills of 2-year-old children. Similarly, Borovsky et al. (Reference Borovsky, Elman and Fernald2012) only showed an association with comprehension vocabulary in a sample of 3- to 10-year-old children but not with age (see Aumeistere et al., Reference Aumeistere, Bultena and Brouwer2022 for a similar result) or a measure of spoken language. Therefore, it is possible that the effects are not as robust and clear-cut as we think, and there might be more than one factor responsible for well-developed prediction skills (see Huettig, Reference Huettig2015 for a similar discussion).
We also did not find significant associations of the phonological STM and the central executive with the children’s prediction skills, unlike several previous studies that indicated an association between (verbal) WM and children’s language comprehension (see Kidd, Reference Kidd2013 for a review). One plausible explanation for the lack of those associations could be that the current study assessed online comprehension of simple structures, while the previous studies largely investigated the offline and online comprehension of complex structures such as relative clauses and passives (e.g., Montgomery et al., Reference Montgomery, Magimairaj and O’Malley2008). The phonological loop and the central executive might be particularly involved in online comprehension of complex structures because of their specific requirements for sequential storage and attentional control.
The current study had two limitations. First, our episodic buffer assessment did not involve a task that captured the binding of information in the auditory and visual modalities. Although we exploited an eye-tracking paradigm that depended on such binding, we could not mount an argument for its role in linguistic prediction. On the other hand, the sentence repetition task was criticized to be another measure of children’s language skills (Klem et. al., Reference Klem, Melby-Lervåg, Hagtvet, Lyster, Gustafsson and Hulme2015). It was beyond the scope of the current paper and the aim of our analyses to test this claim. Nevertheless, our analyses revealed that the skill assessed by the sentence repetition task predicted linguistic prediction, which itself was not associated with other linguistic skills such as grammar and vocabulary.
Second, the current study did not assess other skills that could potentially be related to the prediction skills, such as inhibition and/or cognitive control (Nozari et al., Reference Nozari, Trueswell and Thompson-Schill2016; Zirnstein et al., Reference Zirnstein, van Hell and Kroll2018). For instance, a recent study showed the role of cognitive control in both young and older adults’ flexibility of prediction skills based on the communicative context (Dave et al., Reference Dave, Brothers, Hoversten, Traxler and Swaab2021). The effects of these variables on the children’s prediction skills could be interesting for future research.
An important implication of the current study was that a production-based mechanism seemed to contribute to the type of linguistic prediction investigated here, providing support for one of the mechanisms in the multiple-mechanisms account of prediction (Huettig, Reference Huettig2015). Our findings also implied that processing skills might (longitudinally) mediate linguistic prediction. These implications need to be substantiated by future research. Another future direction might be looking further into longitudinal associations with linguistic prediction skills, perhaps by taking different languages and different cues to prediction into account in one study. In addition, future studies using a vocabulary checklist might consider supplementing this measure with parent confidence ratings on vocabulary items to reduce noise in the data.
In conclusion, this study investigated the role of verbal and WM skills in Turkish-speaking children’s linguistic prediction skills based on case marking. Our results indicated the importance of early productive vocabulary, language production, and episodic buffer for children’s linguistic prediction. To our knowledge, this was the first time that a broad set of verbal and WM skills was investigated in relation to children’s linguistic prediction. Overall, the current study supported the multiple-mechanisms account of linguistic prediction (Huettig, Reference Huettig2015) by showing that the production-based mechanism was likely involved when it comes to linguistic prediction based on case marking. The current study further strengthened the role of WM as a mediating factor of linguistic prediction, while pointing at (early) processing skills as another mediator.
Acknowledgments
This research received generous support from the members of Language and Communication Development Lab and Language and Cognition Lab at Koç University. We thank Buse Naz Yılmaz, Cansu Uçansu, Ege Ekin Özer, Gülgün Uzunoğlu, Melike Coşkun, Minel Güler, Sena Arman, Sera Zeynep Melik, Zeynep Adıgüzel, Zeynep Ayyıldız, and Zeynep Kızıldağ for their assistance in recruitment, data collection, and coding.
Replication Package
Replication data and materials for this article can be found at https://osf.io/qtcf3/.
Conflict of interests
Author S. Brouwer served as associate editor for Applied Psycholinguistics during the submission of this manuscript but played no role in the editorial process for this manuscript.