Introduction
When people listen to each other, they do not just interpret what they actually hear but also predict what they might hear next (Huettig, Reference Huettig2015; Kuperberg & Jaeger, Reference Kuperberg and Jaeger2016; Pickering & Gambi, Reference Pickering and Gambi2018). Such prediction occurs whether the listener is a native (L1) or non-native (L2) speaker of the language they are hearing (Grüter & Kaan, Reference Grüter and Kaan2021). But what are the characteristics of L2 prediction, and is it more restricted than L1 prediction? As we shall see, there is good evidence that L1 prediction involves (at least) two components. In this paper, we report a visual-world eye-tracking study that investigates whether similar components are involved in L2 prediction.
There is much evidence that L1 speakers use sentence context to predict what a speaker is likely to say. For example, Altmann and Kamide (Reference Altmann and Kamide1999) found that L1 English participants fixated a picture of a cake (rather than other inedible objects) earlier and for longer when they heard the speaker say The boy will eat… compared to when they heard the speaker say The boy will move…. These findings suggest that participants used the semantics of the verb to predict which of the objects was most likely to be mentioned next. In another study, Otten and Van Berkum (Reference Otten and Van Berkum2008; Experiment 1b; Otten & Van Berkum, Reference Otten and Van Berkum2009; Wicha, Moreno, & Kutas, Reference Wicha, Moreno and Kutas2004; but see also Kochari & Flecken, Reference Kochari and Flecken2019) found that participants showed a more positive event-related potential (ERP) when they encountered unexpected words in predictive contexts than in non-predictive contexts. Findings such as these suggest that listeners can predict upcoming meaning.
Most accounts of L1 prediction simply assume it involves a single stage in which comprehenders make their best guess about what the speaker is likely to say next. These predictions might be based on characteristics of a single word, but are more typically based on what is consistent with prior context. In line with this argument, Kamide, Altmann, and Haywood (Reference Kamide, Altmann and Haywood2003; Experiment 2) found that comprehenders fixated a picture of a motorbike when they heard The man will ride the…, but a picture of a carousel when they heard The girl will ride the…, with these fixations starting around verb offset. These findings suggest that prediction depends on the whole context and not merely the verb. But whatever prediction is based on, most theories make no claim that it changes over time (e.g., Kuperberg & Jaeger, Reference Kuperberg and Jaeger2016). A possible exception is Huettig (Reference Huettig2015; see also Kuperberg, Reference Kuperberg2021), who postulated several prediction mechanisms, but did not conclude that they acted at different stages in the comprehension process.
However, there is evidence that L1 prediction involves more than one stage. On the basis of an extensive review, Pickering and Gambi (Reference Pickering and Gambi2018) proposed that prediction involves both automatic and non-automatic processing, where automatic processing is rapid and largely resource-free. Automatic prediction is characterised by rapidly spreading activation between associatively related concepts, which makes those associates easier to process if they are subsequently encountered. Such spreading activation explains classic semantic priming studies (e.g., doctor priming nurse; Meyer & Schvaneveldt, Reference Meyer and Schvaneveldt1971).
But such predictions are highly error-prone, because lexically associated concepts are activated even though they are not likely predictions (e.g., a policeman after Toby arrests…; Kukona, Fang, Aicher, Chen, & Magnuson, Reference Kukona, Fang, Aicher, Chen and Magnuson2011). Thus, comprehenders also predict using non-automatic mechanisms, which are slower, under some degree of control, and cognitively demanding. According to Pickering and Gambi, such predictions make use of the language production system. Comprehenders make these non-automatic predictions using linguistic information, such as their experience producing and comprehending similar utterances, and non-linguistic information, such as characteristics of the speaker.
Somewhat consistent with this proposal, Hintz, Meyer, and Huettig (Reference Hintz, Meyer and Huettig2017) had participants listen to predictable (e.g., The man peels…) and unpredictable (e.g., The man draws…) sentences while viewing images of a target (apple) and three unrelated distractors. In three experiments, they found that looks to the target (apple) were positively correlated with the degree of semantic association between the target and the verb (e.g., peel or draw). In addition, looks to the target were positively correlated with participants’ verbal fluency. The first correlation may reflect prediction due to association, whereas the second correlation may reflect prediction involving production. Production requires access to background knowledge, and so it may be that these two correlations point to two components of prediction.
More recently, Corps, Brooke, and Pickering, (Reference Corps, Brooke and Pickering2022; Experiment 1) provided evidence that prediction involves an automatic stage, based on spreading activation, and a non-automatic stage, based on background knowledge. In particular, L1 English participants listened to male and female speakers produce sentences such as I would like to wear the nice… while viewing four pictures of objects. Two of these objects were semantic associates of the verb (targets; e.g., a tie and a dress), while two were not associates (distractors; e.g., a drill and a hairdryer). One target (the dress) and one distractor (the hairdryer) were stereotypically feminine, while the other target (the tie) and distractor (the drill) were stereotypically masculine (these classifications were based on extensive pre-testing). Participants fixated objects associated with the verb (the tie and the dress) more than objects that were not (the drill and the hairdryer) within 519 ms after verb onset, suggesting they predicted associatively. They also predicted consistently – that is, consistent with their beliefs about what the speaker would actually say. In particular, they fixated associates stereotypically compatible with the speaker's gender (the tie for a male speaker, the dress for a female speaker) more than associates that were not from 641 ms after verb onset. Importantly, these consistent predictions occurred later than associative predictions, suggesting that predicting using perspective is non-automatic and requires cognitive resources. Thus, these findings support a two-stage account of prediction, with an initial associative stage followed by a subsequent (and more resource intensive) consistent stage. The second and third experiments provided further evidence for this account, using sentences in which the word I was replaced with You in Experiment 2 or a name that was stereotypically masculine (James) or feminine (Kate) in Experiment 3.
Are similar stages involved in predictions made by L2 speakers? Kaan (Reference Kaan2014) claimed that the mechanisms of predictive processing do not differ between L1 and L2 speakers. If so, L2 prediction would involve multiple stages, much like L1 prediction. But the second, non-automatic, stage may be delayed for L2 speakers compared to L1 speakers because L2 comprehension is more cognitively demanding than L1 comprehension (e.g., Segalowitz & Hulstijn, Reference Segalowitz, Hulstijn, Kroll and De Groot2009; see also Ito & Pickering, Reference Ito, Pickering, Kaan and Grüter2021, who apply a prediction-by-production model to L2 speakers and regard automaticity as graded rather than dichotomous). As a result, it may take them longer to activate the representations necessary for prediction.
In fact, there is some evidence that L2 speakers predict more slowly or predict less information than L1 speakers, perhaps because L2 speakers are less proficient than L1 speakers (e.g., Chambers & Cooke, Reference Chambers and Cooke2009; Peters, Grüter, & Borovsky, Reference Peters, Grüter and Borovsky2015). For example, Ito, Pickering, and Corley (Reference Ito, Pickering and Corley2018) found that both L1 and L2 speakers predictively fixated targets (e.g., a picture of a cloud) when listening to sentences (e.g., The tourists expected rain when the sun went behind the cloud). But only L1 speakers predictively fixated competitors that were phonologically related to the target (a clown) – L2 speakers fixated the phonological competitor only after the target was named. These findings suggest that L2 participants did not predict the form of the target word. In another study, Hopp (Reference Hopp2015) found that both L1 and L2 German speakers (with L1 English) predictively fixated a target object (e.g., a deer) after hearing a verb (e.g., kill) in subject-verb-object sentences such as The wolf will soon kill the deer (Der-Nom Wolf tötet- V gleich den-Acc Hirsch). Thus, they used verb semantics to predict an upcoming word. But L2 speakers, unlike L1 speakers, also fixated this same object when they heard object-verb-subject sentences, such as The hunter will soon kill the wolf (Den-Acc Wolf tötet-V gleich den-Nom Jäger). These findings suggest that L1 speakers used the case marker to predict that the upcoming referent would be an agent of the verb, but L2 speakers did not.
In sum, there is evidence that L1 prediction involves two stages: a rapid, resource-free associative stage, followed by a subsequent resource-intensive consistent stage that draws on world knowledge and is consistent with beliefs about what the speaker is likely to say. There is also evidence that L2 speakers have difficulty with some predictions. But we do not know (1) whether L2 prediction involves multiple stages, and (2) whether difficulty occurs because the consistent stage is delayed for L2 speakers relative to L1 speakers. To address these questions, we used a procedure identical to Corps et al. (Reference Corps, Brooke and Pickering2022), but we recruited male and female L2 English speakers who listened to male and female speakers produce sentences about stereotypically masculine and feminine objects displayed on-screen.
Our manipulation is thus based on gender stereotyping. Note that our discussion of gender refers to (cisgender) males and females and does not consider other gender identities (e.g., Hyde, Bigler, Joel, Tate, & van Anders, Reference Hyde, Bigler, Joel, Tate and van Anders2019), primarily because all our participants identified as either male or female and reported that their gender matched their birth gender. We also assume that our participants have gender-binary stereotypes. For example, a participant might regard a dress as stereotypically feminine; they could also regard it as stereotypically masculine or gender-neutral, but they could not regard it as stereotypically of another gender.
We expected to replicate previous research showing associative semantic predictions in L1 speakers (Corps et al., Reference Corps, Brooke and Pickering2022). We also expected participants to predict consistently, fixating the target stereotypically compatible with the speaker's gender more than stereotypically incompatible target. To determine whether L2 prediction involves two stages, we compared the time-course of associative and consistent prediction. Importantly, if consistent prediction is non-automatic and requires cognitive resources, then we expect it to be delayed in L2 speakers relative to L1 speakers. To test this hypothesis, we compared the time-course of the consistent effect in this experiment (with L2 speakers) to the time-course of the consistent effect in Experiment 1 from Corps et al. (Reference Corps, Brooke and Pickering2022).
Method
Participants
Thirty-two (Mage = 25.33, 16 males, 16 females) L2 English speakers studying at the University of Edinburgh participated in exchange for £10. Participants had no known speaking, reading, or hearing impairments, and were aged between 18 and 35. All participants indicated their gender and whether they identified as the gender they were assigned at birth. These questions were open-ended (i.e., gender was not assumed to be binary), and so participants could answer in any way they wished. Importantly, all participants reported being male or female and identified as the gender they were assigned at birth.
Participants also filled in a language background questionnaire and completed a Transparent Language English proficiency test (assessing grammar, vocabulary, and comprehension; https://www.transparent.com/learn-english/proficiency-test.html) at the end of the experiment. Participants’ native languages were Chinese (15), Italian (5), Spanish (4), German (2), Dutch (2), Japanese, Russian, Greek, and Malay. On average, participants were first exposed to English when they were eight years old (SD = 2.62) and were exposed to English for an average of 17.50 years (SD = 3.49). Participants had an average English proficiency of 92% on the Transparent proficiency test, indicating that they were advanced learners of English. Participants also provided their official test scores. Twenty-five of them provided an International English Language Testing System (IELTS) score (M = 7.46; SD = 0.70), six provided a Test of English as a Foreign Language (TOEFL) score (M = 105, SD = 10.11), and one provided a Certificate of Proficiency in English (CPE) score (B). All of our participants were thus highly proficient in English. We selected this sample to ensure they could recognise the words and objects used in our experiment. All participants were included in the main analysis, regardless of their ratings in the gender stereotypy post-test.
Our sample size was based on previous studies using the visual-world paradigm with a similar design (in particular, Corps et al., Reference Corps, Brooke and Pickering2022; see also Altmann & Kamide, Reference Altmann and Kamide1999). Our experiment involved more items than previous experiments (e.g., 28 critical sentences vs. 16 in Altmann & Kamide, Reference Altmann and Kamide1999) and we had at least a similar number of critical trials to previous studies (e.g., Kukona et al., Reference Kukona, Fang, Aicher, Chen and Magnuson2011 had 640 trials; Altmann & Kamide, Reference Altmann and Kamide1999 had 384; we had 672). Thus, we likely had sufficient power to detect an effect.
Materials
We used the same stimuli as Corps et al. (2022; a full list of stimuli can be found in the Appendix). Specifically, participants heard 56 sentences, each paired with a display of four coloured objects. These sentences contained predictive verbs (e.g., wear in the sentence I would like to wear the nice…), so that two of the four depicted objects were plausible targets of the verb (e.g., tie and dress), while the other two were distractors (e.g., drill and hairdryer).
Twenty-eight of these sentences were gendered, so that two of the four objects were stereotypically feminine (e.g., one feminine target: dress; one feminine distractor: hairdryer), while the other two were stereotypically masculine (e.g., one masculine target: tie; one masculine distractor: drill). Note that object names were matched for their syllable length (t(54) = 0.88, p = .38; see Table 1).
Table 1. The means (and standard deviations) of agreement on the name of the object, job, or activity depicted in the image, the syllable length of the object, and the difference between the average stereotypy rating and the maximum or minimum of the rating scale for targets in the gendered and gender-neutral sentences. Ratings are reported collapsed across all participants, and separately for male and female participants.

a Difference between average stereotypy ratings and the maximum or minimum of the scale. For one group of participants, 1 indicated that the depicted object, activity, or job was masculine, while 100 indicate it was feminine. If these participants rated a feminine object, then distance was calculated as the object's average stereotypy rating (across all participants) subtracted from 100 (the corresponding maximum of the scale). If these participants rated a masculine object, however, distance was calculated as the object's average stereotypy rating minus 1 (the corresponding minimum of the scale). For the other group of participants, the rating scale was reversed (1 = feminine, 100 = masculine).
We assessed the stereotypy of these objects using a pen-and-paper post-test, administered on the same set of participants after they had completed the eye-tracking experiment. We administered the stereotypy test after the main experiment, rather than before, to avoid making participants aware of the stereotypy manipulation. Note that this detail differed from Corps et al. (Reference Corps, Brooke and Pickering2022), in which the participants for the stereotypy test did not take part in any of the eye-tracking experiments. In Corps et al., we reasoned that we could safely sample participants from the same population (L1 English undergraduates aged 18-25 at the University of Edinburgh), as they were likely to be relatively homogenous in their stereotypy judgments for our items. But any sample of L2 participants is likely to be more diverse in this respect (e.g., whether they regarded a particular article of clothing as strongly stereotypical), and so we judged it safer for the same participants to take part in the eye-tracking experiment and the post-test.
Participants were randomly assigned to one of two lists of stimuli (16 participants per list), each containing the 112 colour clipart images used in the eye-tracking experiment and their respective names. For each image, participants rated the masculinity or femininity of the object, activity, or job depicted in the image on a scale of 1-100. For half the male and half the female participants, 1 indicated that the object, activity, or job was strongly masculine, and 100 indicated that it was strongly feminine. This rating scale was reversed for the other half of participants.
On average, masculine objects were considered masculine (an average rating of 21.67 when 1 = masculine, and 78.85 when 100 = masculine) and feminine objects were considered feminine (75.97 when 100 = feminine, 20.98 when 1 = feminine). We collapsed the two rating scales, so that we could determine whether ratings were affected by object and participant gender. In particular, we calculated the difference between the maximum or minimum of the rating scale and the average stereotypy rating. The difference between the maximum or minimum of the rating scale and the average stereotypy rating did not differ for the masculine and feminine objects (t(54) = .41, p = .68; see Table 1), suggesting that masculine objects were considered just as masculine as feminine objects were considered feminine. The difference between the maximum or minimum of the rating scale and the average stereotypy rating was also similar for the male and the female participants (F(1, 220) = 1.66, p = .20), suggesting that ratings were unaffected by participant gender. Finally, there was no interaction between target and participant gender (F(1, 220) = .51, p = .47), suggesting that male and female participants did not rate masculine and feminine objects differently. These ratings were similar to the ratings made by L1 participants in Corps et al. (Reference Corps, Brooke and Pickering2022). In that study, the difference between the maximum or minimum of the rating scale and the average stereotypy rating was 18.19 for the masculine objects, and 16.74 for the feminine objects.
The sentences also included 28 gender-neutral filler sentences, which were designed to make our gender manipulation less obvious and to further test the time-course of associative prediction. These gender-neutral sentences also contained predictive verbs (e.g., I would like to eat the nice…), but the four accompanying objects were rated as gender neutral in the post-test (an average stereotypy rating of 47.44 when 1 = masculine, and 50.16 when 100 = masculine; see Table 1). Two of the four objects were potential targets of the verb (e.g., apple and banana), while the other two were distractors (e.g., water and milk). Even though the targets were rated as gender neutral, females rated targets as nearer to gender neutral than males (F(1, 220) = 6.25, p = .01). This gender difference does not pose a problem for our analysis of the gender-neutral sentences because they were not designed to test gender effects. Again, these ratings were similar to those observed by Corps et al. (Reference Corps, Brooke and Pickering2022) – the difference between the maximum or minimum of the rating scale and the average stereotypy rating was 48.34 for gender-neutral object one, and 48.61 for gender-neutral object two.
Sentences were recorded by one native British English male speaker and one native British English female speaker, who produced the sentences at a natural, slow rate. We determined speech rate by dividing the duration of the sentence by the number of syllables. On average, sentences were produced at a rate of 266 ms for both the gendered (SD = 37 ms) and neutral (SD = 34 ms) sentences. The male and female speakers did not differ in their speech rate when producing either the gendered (t(54) = 0.28, p = .78) or neutral (t(54) = 0.88, p = .38) sentences.
For the gendered sentences, speakers referred to the target that was stereotypical for their gender (i.e., the male speaker said tie and the female speaker said dress), so that any predictions participants made using the speaker's gender were always accurate. For the gender-neutral sentences, the speaker referred to one of the two targets, and this target was consistent across the two speakers. Sentences were between 2221 and 4472 ms. Sentences produced by the two speakers were matched for their duration, the onset and offset of the critical verb, and the onset of the target (all ps > .21 in t-tests; see Table 2). These recordings were also used in Experiment 1 of Corps et al. (Reference Corps, Brooke and Pickering2022).
Table 2. The means (and standard deviations) of sentence duration (ms), critical verb onset and offset, and target onset for the sentences produced by male and female speakers.

Design
The design was also the same as Corps et al.'s (2022) Experiment 1. We manipulated speaker gender within items and participants. There were two versions of each item: one produced by a male speaker, and one produced by a female speaker. Participants were assigned to one of two stimulus lists so that they heard only one version of each item, but heard: (1) 28 gendered sentences and 28 gender-neutral sentences, and (2) 14 sentences produced by a male speaker and 14 sentences produced by a female speaker for each sentence type. In all lists, each object was shown on-screen twice: once as a target and once as a distractor.
For the gendered sentences, each visual layout consisted of an agent-compatible target, which was a potential target and matched the speaker's gender (e.g., a tie when a male speaker said I would like to wear the nice…), an agent-incompatible target, which was a potential target of the opposite gender (e.g., a dress), an agent-compatible distractor, which matched the speaker's gender but was not a target of the verb (e.g., a drill), and an agent-incompatible distractor, which matched the opposite gender and was not a target (e.g., a hairdryer). For the gender-neutral trials, participants were shown two targets and two distractors which were gender neutral. Twenty layout combinations (e.g., agent-compatible target top left, agent-incompatible target top right, agent-compatible distractor bottom left, agent-incompatible distractor bottom right) were used once, and four randomly selected layouts were used twice.
Procedure
Before beginning the eye-tracking experiment, participants were familiarised with the names of the images. During familiarisation, participants were given ten minutes to study the 112 coloured images and their corresponding object names. Participants were then presented with the images (without their names) and were instructed to name the object, activity, or job depicted in the image. On average, participants correctly named 89% of the images, suggesting names were readily identifiable.
Participants were then seated in front of a 1024 x 768 pixel monitor and were instructed to listen to the sentences and look at the accompanying pictures. Their eye movements were recorded using an Eyelink 1000 Tower mounted eye-tracker sampling at 1000 Hz from the right eye. After reading the instructions, participants placed their head on the chin rest and the eye-tracker was calibrated using a nine-point calibration grid.
Each speaker then introduced themselves once (with order counterbalanced across participants). Participants first saw a fixation dot, which was followed by a blank screen displayed for 1000 ms. The speaker's picture was then displayed in the center of the screen (at a size of 300 x 300 pixels), and they introduced themselves 1000 ms later by saying: “Hi, I am Sarah/Andrew and you are going to hear me describe some objects. Please listen carefully and look at the objects on-screen”.
After the speakers introduced themselves, participants matched each speaker's voice to their picture. Participants first saw a fixation dot, followed by a blank screen displayed for 1000 ms. Both speakers’ pictures were displayed on the center of the screen (one on the left and one on the right, counterbalanced across participants), and each speaker said “Which one am I?” (with order counterbalanced). Participants then pressed a button on the button-box (left button for the speaker displayed on the left; right button for the speaker displayed on the right) to indicate which picture corresponded to the heard speaker.
Participants then began the main experiment. Each trial started with a drift correct, followed by a 300 x 300 pixel picture of the speaker displayed in the centre of the screen for 1000 ms. A blank screen was then displayed for 500 ms and four pictures were presented in each of the four corners of the screen. Sentence playback began 1000 ms later, and the pictures remained on-screen for 750 ms after sentence end. Participants then answered a comprehension question, which asked if the speaker referred to a particular object displayed on-screen (e.g., Did the speaker say hairdryer?). Half of the time, the comprehension question mentioned an object the speaker had referred to; the other half of the time, the question referred to one of the other three unmentioned objects. Participants pressed the left button on the response box to answer yes, and right to answer no. The next trial then began immediately. No feedback was given during the experiment. Participants completed four practice trials and were given the opportunity to take a break after 28 experimental trials. After the experiment was complete, participants were handed the post-test (unlike Corps et al., Reference Corps, Brooke and Pickering2022).
Data analysis
We analysed the eye-tracking data in RStudio (version 1.2.5042) using the same procedure as Corps et al. (Reference Corps, Brooke and Pickering2022). Fixations to the four pictures were coded binomially (fixated = 1; not fixated = 0) for each 50 ms bin from 1000 ms before to 1500 ms after verb onset. Fixations were directed towards a particular object if they fell in the 300 x 300 pixel area around the picture. Blinks and fixations outside the interest areas were coded as 0 (i.e., no fixation on any of the objects) and were included in the data. Our analysis focuses on the gendered trials, and tests: (1) whether participants predicted associatively, fixating semantic associates of the verb (e.g., looking at wearable objects after hearing the verb wear); (2) whether they predicted consistently (or egocentrically), fixating the target stereotypically compatible with the speaker's gender over the target stereotypically compatible with their own gender (or vice versa); and (3) whether associative prediction occurred before consistent prediction (in accord with a two-stage account). We also compared looks to the two targets to looks to the two distractors on the gender-neutral trials to further test for associative predictions.
We analysed our data using a bootstrapping analysis, which deals with the non-independence of fixations in our data. We chose bootstrapping over the typical binning analyses because: (1) binning involves fitting as many models as there are timepoints, which increases the chance of Type 1 error (Hochberg & Tamhane, Reference Hochberg and Tamhane1987), and (2) fixations in adjacent bins are often highly correlated. Bootstrapping identifies the time point at which looks to one object diverge from looks to another (Stone, Lago, & Schad, Reference Stone, Lago and Schad2020). The analysis involves three steps. First, we apply a one-sample t-test to fixation proportions at each timepoint, aggregating over items. Average fixation proportions are compared to .50, with a significant p value indicating that the object attracted more than half of the fixations (out of the two objects we are comparing fixations to). A divergence point is then identified by determining the first significant timepoint in a run of at least ten consecutive significant time points (i.e., 500 ms). New datasets are then generated 2000 times, using a non-parametric bootstrap, which resamples data from the original data set using the categories participant, timepoint, and image type. A new divergence point is estimated after each resample, and the mean is calculated. Confidence intervals (CIs) indicate variability around the average divergence point.
To determine whether participants predicted associatively, we compared fixation proportions for the agent-compatible target to fixations for the agent-compatible distractor. Note that we could have also tested whether participants predicted associatively by comparing fixations to the two targets to the two distractors. But this analysis would be based on twice the amount of data as a consistent or egocentric analysis, which compares one target to another target. Thus, for comparability, our associative analysis also compares one target to one distractor.
To determine whether participants predicted consistently or egocentrically, we compared fixation proportions for the agent-compatible target to fixations for the agent-incompatible target. In Corps et al. (Reference Corps, Brooke and Pickering2022), we ran the divergence point bootstrapping analysis from verb onset (0 ms) to 1500 ms after verb onset. Figure 2, however, suggests that participants may have preferred agent-compatible targets, agent-compatible distractors, and agent-incompatible targets more than agent-incompatible distractors even before verb onset. For this reason, we ran the bootstrapping analysis from 1000 ms before to 1500 ms after verb onset.

Figure 1. Schematic representation of the eye-tracking procedure.
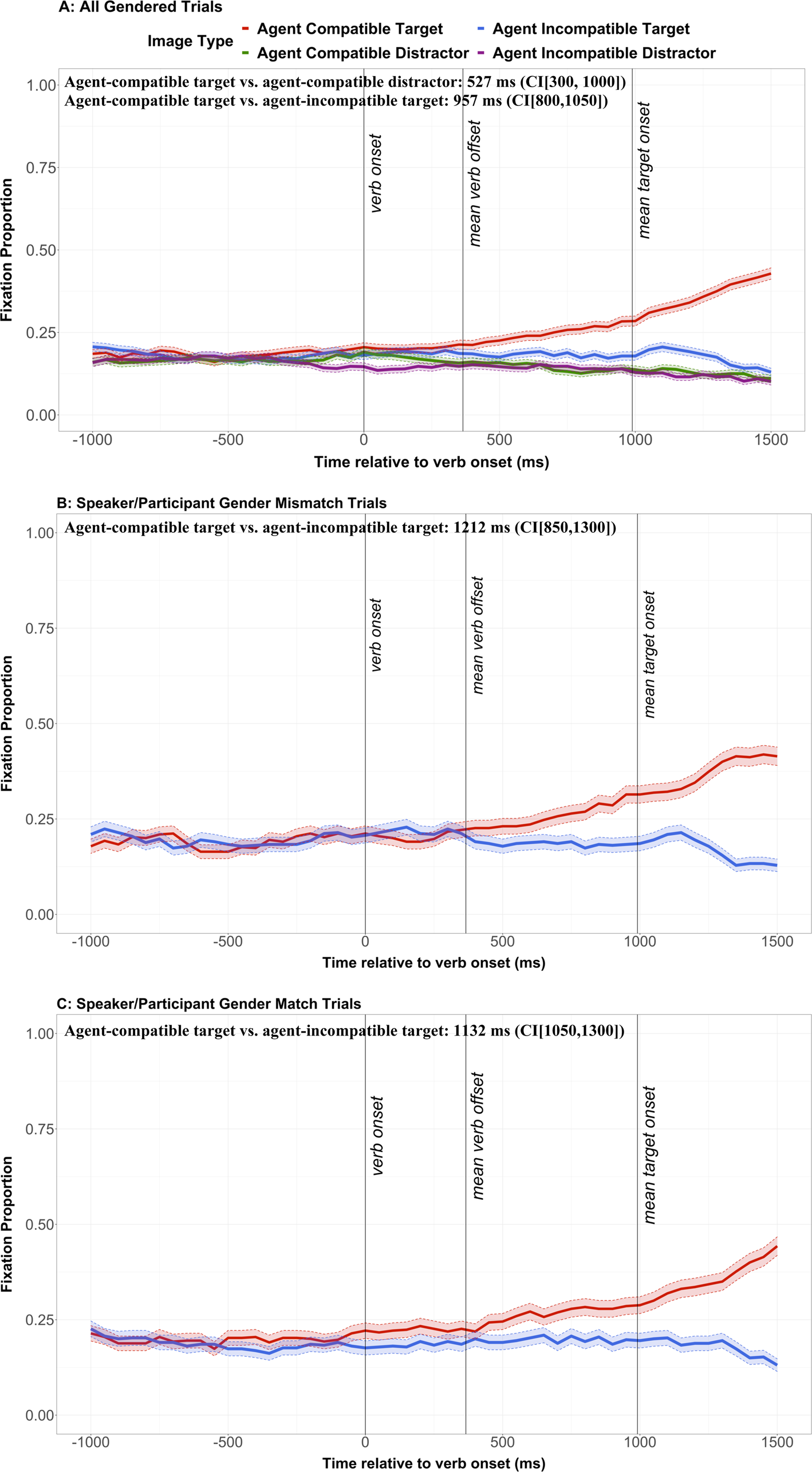
Figure 2. Eye-tracking results for the gendered trials. Panel A shows the mean fixation proportions on the four pictures for all gendered trials. Panels B and C show the mean fixation proportions on agent-compatible and agent-incompatible targets for the gender-mismatch trials (speaker and participant have different gender; panel B) and the gender-match trials (speaker and participant have same gender; panel C). Transparent thick lines are error bars representing standard errors. The text provides divergence points and confidence intervals (CIs).
These analyses were based on all the gendered trials – that is, the ones in which the participant and speaker had the same gender (the gender-match trials) and the ones in which they had different genders (the gender-mismatch trials). It is clear from Figure 2 that there was no point at which participants predicted egocentrically, fixating the agent-incompatible target more than the agent-compatible target. However, it is possible that participants were initially egocentric in their predictions, but this egocentricity was drowned out by the larger consistency effect. We tested this possibility in a third analysis, in which we compared looks to the agent-compatible target to looks to the agent-incompatible target for the mismatch trials only. Running a comparable analysis on the match trials is not necessary for testing our predictions since these trials do not isolate egocentric and consistent effects. But for the sake of completeness, we conducted an identical analysis for the gender-match trials. Note that for these analyses, a divergence point was identified by defining the first significant timepoint in a run of at least five consecutive significant timepoints (i.e., 250 ms), rather than ten (i.e., 500 ms). When we ran these models with a criterion of ten timepoints, the bootstrap returned NAs, indicating that there was not a run of ten timepoints where looks to the agent-compatible target significantly differed from looks to the agent-incompatible target. These NAs make sense, given that Figures 2B and 2C indicate that looks to the two objects do not begin to diverge until around 600 ms after verb onset, and the time window for analysis runs to only 1500 ms after verb onset. Thus, we used a lower criterion which did not return NAs, and which reported a run of five significant timepoints.
To determine whether associative prediction occurred before consistent prediction, we bootstrapped the difference between their divergence points. In particular, we subtracted the onset of the associative effect from the onset of the consistent effect, following the same procedure as Stone et al. (Reference Stone, Lago and Schad2020). In the gender-mismatch analysis, we found no evidence that participants ever predicted egocentrically. As a result, we calculated a difference for all the gendered trials, regardless of whether the participant and the speaker had matching or mismatching genders.
Finally, we compared the time-course of consistent prediction in L2 and L1 speakers. In particular, we bootstrapped the difference between the divergence points of the consistent effect (agent-compatible target vs. agent-incompatible target) in this experiment and the consistent effect reported in Experiment 1 of Corps et al. (Reference Corps, Brooke and Pickering2022). To do so, we subtracted the onset of the consistent effect in L2 speakers from the onset of the consistent effect in L1 speakers. We did not expect there to be any difference in the time-course of associative prediction for the two groups, since associative prediction is automatic and based on spreading activation (e.g., Pickering & Gambi, Reference Pickering and Gambi2018). But to confirm this claim, we also compared the time-course of the associative prediction in L2 and L1 speakers by subtracting the onset of the associative effect (agent-compatible target vs. agent-compatible distractor) in L2 speakers from the onset of the associative effect in L1 speakers. Raw data and scripts for all analyses are available on Open Science Framework at https://osf.io/a6y24.
Results
Comprehension question accuracy
The mean accuracy for the comprehension questions in all trials was 99%.
Eye-tracking results
Figure 2 shows the mean fixation proportions to the four pictures for the gendered sentences (panel A), which is then divided into the mean fixation proportions on agent-compatible and agent-incompatible targets for the gender-mismatch (panel B) and gender-match (panel C) trials. Time was synchronised to verb onset, and the plot shows the time window from 1000 ms before to 1500 ms after verb onset (as in Corps et al., Reference Corps, Brooke and Pickering2022).
Participants fixated the agent-compatible target more than the agent-compatible distractor from 527 ms (CI[300, 1000]) after verb onset. The CI does not contain zero, and so supports a significant difference in looks to the two objects at 527 ms after verb onset. We observed a similar pattern for the gender-neutral trials: participants fixated the two targets more than the two distractors from 562 ms (CI[500, 700]; Figure 3). Thus, participants predicted associatively.

Figure 3. Eye-tracking results for the gender-neutral trials. Transparent thick lines are error bars representing standard errors. The text provides divergence points and confidence intervals (CIs).
Participants also fixated the agent-compatible target, which the speaker actually referred to, more than the agent-incompatible target, which they did not, from 957 ms after verb onset (CI[800, 1050]). Thus, participants predicted consistently, from the speaker's perspective, rather than egocentrically, from their own perspective. Our separate analysis of the gender-mismatch trials confirmed there was no point at which participants predicted egocentrically: they fixated the agent-compatible target more than the agent-incompatible target from 1212 ms (CI[850, 1300]). We found similar results in the gender-match trials: participants fixated the agent-compatible target more than the agent-incompatible target from 1132 ms (CI[1050, 1300]).
After demonstrating that L2 speakers predicted associatively and consistently, we tested the difference in the time-course of these two types of prediction by subtracting the onset of the associative effect from the onset of the consistent effect. This analysis showed that the consistent effect occurred 362 ms after the associative effect (CI[45, 750]). Thus, we found evidence for two stages of prediction.
We were also interested in whether the consistent stage of prediction is non-automatic and requires cognitive resources. In Corps et al. (2022; Experiment 1), we found that L1 participants predicted associatively 519 ms (CI[500, 650]) after verb onset and consistently 641 ms (CI[600, 950]) after verb onset. It appears that consistent predictions occurred numerically later for L2 speakers (957 ms after verb onset) than for L1 speakers. But to statistically test this difference, we subtracted the onset of the consistent effect for the L1 speakers in Corps et al. from the onset of the consistent effect for the L2 speakers in this experiment. This analysis showed that the consistent predictions were made 251 ms later in the L2 speakers than in the L1 speakers. The confidence intervals (CI[150, 450]) did not contain zero, thus showing that the difference was statistically significant. Figure 4A shows the time-course of consistent prediction for L1 and L2 speakers.
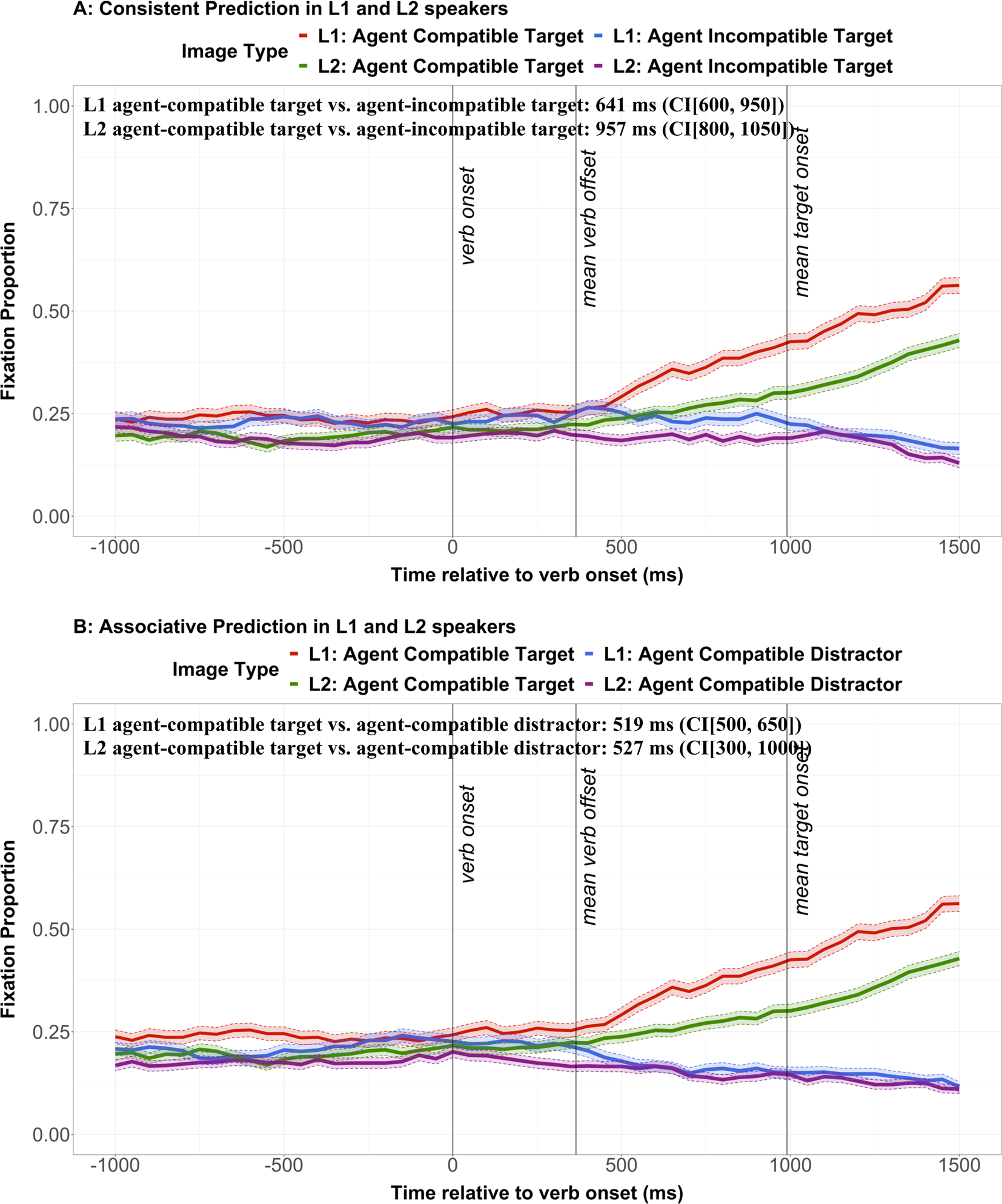
Figure 4. Comparison of the time-course of consistent prediction (panel A) and associative prediction (panel B) in L1 participants (from Corps et al., 2022) and L2 participants (this experiment). Transparent thick lines are error bars representing standard errors. The text provides divergence points and confidence intervals (CIs).
We did not expect the time-course of associative prediction to differ greatly between L1 and L2 speakers, because associative predictions are based on automatic mechanisms. Note, however, that there may be a small difference because non-predictive lexical processing might take longer for L2 than L1 speakers. In fact, the time-course of associative prediction was almost identical for the two groups of participants, with associative predictions occurring 8 ms later for the L1 speakers than for the L2 speakers. But to confirm this hypothesis, we subtracted the onset of the associative effect for the L1 speakers from the onset of the effect for L2 speakers. This analysis showed that associative predictions were made 33 ms later in the L1 speakers than in the L2 speakers (note that this number differs from the 8 ms numerical difference because it is based on an average divergence point calculated across 2000 resamples). The confidence intervals (CI[-300, 450]) contained zero, showing that the difference was not statistically significant. Figure 4A shows the time-course of associative prediction for L1 and L2 speakers.
Figure 4 suggests that L1 speakers fixated the agent-compatible target more than L2 speakers. We calculated the sum of the fixation proportions to the four objects in each time bin (from 1000 ms before to 1500 ms after verb onset) for the experimental trials. We found that L2 speakers fixated the pictures on average 75% of the time, while the L1 speakers fixated them 91% of the time. Thus, L1 speakers fixated all four pictures more than the L2 speakers, suggesting that L2 speakers spent more time looking elsewhere, either at the white space between the pictures or away from the screen entirely.
Discussion
In this experiment, we used a visual-world eye-tracking paradigm to investigate whether L2 prediction involves two different stages. We found that participants predicted associatively, rapidly fixating objects semantically associated with critical verbs (e.g., hearing wear and fixating wearable objects). They also predicted consistently, from the speaker's perspective – they heard a female speaker say wear and predicted that she would refer to a stereotypically feminine wearable object (e.g., a dress) rather than a stereotypically masculine wearable object (e.g., a tie). These consistent predictions were made later than associative predictions, and a comparison with L1 speakers from Corps et al. (2022; Experiment 1) showed that L2 speakers were much slower at making these consistent predictions.
Our findings are compatible with two-stage accounts of prediction, which claim that prediction involves both automatic and non-automatic processing (e.g., Huettig, Reference Huettig2015; Pickering & Gambi, Reference Pickering and Gambi2018). For example, Pickering and Gambi proposed that automatic prediction is characterised by rapidly spreading activation between associatively related concepts, while non-automatic prediction draws on the comprehender's previous experience of producing and comprehending similar utterances or their knowledge of the speaker's perspective. Studies providing evidence for these accounts have mainly been conducted in L1 speakers. However, our results provide evidence that L2 prediction also involves two stages, and so is similar to L1 prediction. This finding is consistent with Kaan's (Reference Kaan2014) claim that the mechanisms of L1 and L2 prediction do not fundamentally differ.
But we also found that L2 prediction differed from L1 prediction. In particular, consistent predictions occurred later in L2 than in L1 participants. In accord with Pickering and Gambi (Reference Pickering and Gambi2018), one would expect consistent predictions to be non-automatic because they are based on world knowledge and involve cognitively demanding adjustments for differences in the perspective of the speaker and the listener. Hitherto, the only evidence for this claim was that this consistent stage was delayed relative to an associative stage (Corps et al., Reference Corps, Brooke and Pickering2022). Our comparison between Corps et al.'s Experiment 1 and the current experiment showed that consistent predictions are delayed in L2 speakers relative to L1 speakers. This finding implies that such predictions draw on resources that are more limited in L2 than L1 speakers, and thus that they are non-automatic.
But why were L2 speakers slower to make these consistent predictions than L1 speakers? And what resources are limited in L2 speakers compared to L1 speakers? One possibility is that L2 comprehension is more cognitively demanding than L1 comprehension (e.g., Segalowitz & Hulstijn, Reference Segalowitz, Hulstijn, Kroll and De Groot2009), perhaps because L2 speakers are less proficient than L1 speakers (e.g., Peters et al., Reference Peters, Grüter and Borovsky2015) and so they do not have the necessary information to quickly make self-other adjustments. Alternatively, L2 speakers may need to allocate more cognitive resources to inhibiting incorrect predictions (e.g., Cunnings, Reference Cunnings2016). As a result, L2 speakers may have less information or fewer cognitive resources available to make the self-other adjustments necessary to predict from the speaker's perspective. All of our participants were highly proficient in English, however, and so further research is needed to investigate this issue.
Another possibility is that the consistent effect emerged later in L2 speakers because it takes them longer than L1 speakers to access gender-stereotyped information at the verb. This explanation would still fit with a two-stage account of prediction, but the second stage would be limited to information about gender stereotypy. However, this explanation may be inconsistent with the evidence that gender stereotyping occurs rapidly (e.g., Banaji & Hardin, Reference Banaji and Hardin1996; Reynolds, Garnham, & Oakhill, Reference Reynolds, Garnham and Oakhill2006). Finally, it is also possible that L2 speakers were slower than L1 speakers when making consistent predictions because they had less knowledge of gender stereotypes, or they did not strongly believe these stereotypes. As a result, they may not have had a strong preference for objects stereotypically consistent with the speaker's gender. But participants rated the items as strongly stereotypically masculine or feminine (much like the L1 speakers), which rules out this explanation.
Importantly, we found no evidence that associative predictions differed in their time-course in L1 and L2 speakers. This finding suggests that this associative stage is automatic, and so is not affected by differences in the availability of cognitive resources in L1 and L2 speakers. In contrast, we argue that the consistent stage is non-automatic, and so is affected by differences in the availability of cognitive resources in L1 and L2 speakers.
But could this consistent effect also be driven by semantic associations? For example, the participant may hear a male speaker say wear and predict he will say tie by associating the verb, the speaker's voice, and a wearable object (a three-way association), rather than by adjusting for differences in perspective. But this explanation seems unlikely, since it would lead to a different pattern of results from the one that we observed. In particular, we would expect participants to fixate agent-compatible pictures more than agent-incompatible pictures shortly after sentence onset, because they could already use the speaker's voice to predict the speaker will refer to stereotypically masculine associates. We would then expect participants to fixate the agent-compatible target more than the agent-compatible distractor once they heard the verb. But we did not observe this pattern of results, and so there is no evidence that participants were using the speaker's gender to predict associatively.
Our results thus suggest that the stages involved in L2 prediction are identical to those involved in L1 prediction, but there is a specific delay in the second stage. Apart from having implications for L2 processing, our findings are also consistent with modular (or encapsulated) accounts of language comprehension (Fodor, Reference Fodor1983). Previously, the major focus was on how comprehenders initially select among analyses of syntactically ambiguous sentences. One-stage (or interactive) accounts assume that people immediately draw on all potentially relevant information (e.g., MacDonald, Pearlmutter, & Seidenberg, Reference MacDonald, Pearlmutter and Seidenberg1994), whereas two-stage (or modular) accounts assume that initial decisions are based on some sources of information (e.g., some aspects of syntax) but not others (e.g., real-world knowledge; e.g., Frazier, Reference Frazier and Coltheart1987). Our findings relate to an additional issue, and suggest that the process of making prediction about language is modular.
It is worth noting that the L2 speakers fixated the pictures less than the L1 speakers. This difference may have occurred because L2 comprehension is more cognitively demanding than L1 comprehension (e.g., Segalowitz & Hulstijn, Reference Segalowitz, Hulstijn, Kroll and De Groot2009). As a result, L2 speakers have a higher cognitive load than L1 speakers, which may have interfered with visual processing, including identifying the objects displayed on-screen or moving their eyes to the target object. Nevertheless, our results show that L2 speakers do fixate the objects, and they use the linguistic and non-linguistic context to predict what a speaker is likely to say.
In sum, we used the visual-world paradigm to demonstrate that non-native prediction involves two stages. We found that participants predicted associatively, rapidly fixating objects semantically associated with critical verbs (e.g., wear). We also found that participants predicted consistently, from the speaker's perspective. In particular, they homed in on associates stereotypically compatible with the speaker's gender. These consistent predictions were made later than associative predictions, suggesting that L2 prediction involves two components. When comparing L2 predictions with predictions made by participants with L1 English, we found that consistent predictions were delayed for L2 participants. We conclude that prediction involves two stages, with the second stage delayed in L2 speakers relative to L1 speakers.
Acknowledgements
Ruth Corps was supported by a Leverhulme Research Project Grant [RPG-2018-259] awarded to Martin Pickering. We thank Kate Stone for useful advice on data analysis.
Competing interests
The authors declare none.
Data Availability
The data that support the findings of this study (and the analysis script) are openly available on Open Science Framework at https://osf.io/a6y24/
Appendices
Appendix A: Gendered and gender-neutral sentence fragments and target picture names used in Experiment 1. Predictable verbs are highlighted in bold.
Table A1. Gendered sentences used in Experiment 1. The speaker always referred to the target stereotypically compatible with their gender.

Table A2. Gender-neutral sentences used in Experiment 1. The speaker referred to one of the two targets, but this target was the same for a male and female speaker.
