Introduction
Word learning is a continuous and lifelong endeavor, particularly for a second language learner. Native English-speaking adults know anywhere from 27,000 to 52,000 words from approximately 11,000 to 13,000 word families in their native language (Brysbaert et al., Reference Brysbaert, Stevens, Mandera and Keuleers2016). To approach functional proficiency in their targeted language, any second language (L2) learner needs to acquire a similarly large vocabulary. For an English learner, this is estimated to be anywhere from 7,000 up to 9,000 word families (Hirsh & Nation, Reference Hirsh and Nation1992; Nation, Reference Nation2006). Thus, an underlying goal for many language learners is to learn more words better and faster. An increasingly popular way for millions of people to achieve this goal has been through using language learning apps. The data available through language learning apps introduce an exciting opportunity for researchers to test theories of vocabulary acquisition against a plethora of ecologically valid observations obtained in the real-world learning environment.
This study leverages data from the language learning app Lingvist to explore word learning in the wild, that is, outside of highly controlled experiments, using real language stimuli, and with users operating in their natural, self-selected learning habits and timelines. This research is of both academic interest, as it contributes robust and ecologically sound evidence to the current body of word learning literature, as well as applied interest in answering the question “how can we learn languages faster?”
Word learning effects
Some of the factors influencing L2 word learning are very well established. The more exposure a learner has to a word, the better it is learned and the longer it is retained in memory (e.g., Hulme et al., Reference Hulme, Shapiro and Taylor2022). This L2 frequency effect also has an L1 counterpart: Higher-frequency words in the L1 are learned and processed faster in the L2 than low-frequency words (e.g., Carroll, Reference Carroll2013; Crossley et al., Reference Crossley, Subtirelu and Salsbury2013; Elgort et al., Reference Elgort, Brysbaert, Stevens and Van Assche2018). There is a general consensus that the early phases of novel word learning are mainly subserved by the domain-general nonlinguistic capacity for declarative episodic memory, responsible for encoding contextualized representations of personal experiences. As knowledge of the novel word becomes entrenched through practice, word representation, and processing transition to the domain of non-contextualized, more abstract semantic memory (Davis et al., Reference Davis, Di Betta, Macdonald and Gaskell2009; Davis & Gaskell, Reference Davis and Gaskell2009; Gaskell & Ellis, Reference Gaskell and Ellis2009; Hamrick, Reference Hamrick2015; Hamrick et al., Reference Hamrick, Graff and Finch2019; Ullman, Reference Ullman2004, Reference Ullman2020). Dirix and Duyck (Reference Dirix and Duyck2017) also indicate that the age of acquisition in both the L1 and L2 predicts L2 word learning: Words learned earlier are learned better. Furthermore, target language words that look or sound like words in the learner’s L1 (i.e., cognates) are learned faster than word pairs with very different forms in the L1 and L2 (e.g., Brenders et al., Reference Brenders, Van Hell and Dijkstra2011; Comesaña et al., Reference Comesaña, Soares, Sánchez-Casas and Lima2012; de Groot & Keijzer, Reference de Groot and Keijzer2000; Dijkstra et al., Reference Dijkstra, Miwa, Brummelhuis, Sappelli and Baayen2010; Tonzar et al., Reference Tonzar, Lotto and Job2009).
This study targets less commonly studied semantic factors implicated in word learning and recall, such as concreteness and valence. Concreteness distinguishes between words that have tangible or sensory referents (e.g., brick, painting) and more abstract words which do not (e.g., hope, imagination). Children’s productive vocabulary contains more concrete words than abstract words up until the 8th grade, which suggests that concrete words and concepts are acquired earlier and easier than abstract ones (Schwanenflugel, Reference Schwanenflugel1991). Furthermore, a concreteness advantage is reported for adults performing long- and short-term recall tasks (Gee et al., Reference Gee, Nelson and Krawczyk1999; Paivio et al., Reference Paivio, Yuille and Smythe1966; Romani et al., Reference Romani, Mcalpine and Martin2008). Most importantly for the current study, there is also evidence that concrete words in the L2 are learned better than abstract words (de Groot & Keijzer, Reference de Groot and Keijzer2000; Ferré et al., Reference Ferré, Ventura, Comesaña and Fraga2015; Kaushanskaya & Rechtzigel, Reference Kaushanskaya and Rechtzigel2012).
Valence, a measure of how emotionally positive or negative a stimulus is, also affects word learning and recall. A number of studies have shown improved recall for both positively and negatively valenced words (e.g., chocolate and murder, respectively) over neutral words (e.g., status, output) (Adelman & Estes, Reference Adelman and Estes2013; Ferré et al., Reference Ferré, García, Fraga, Sánchez-Casas and Molero2010; Gomes et al., Reference Gomes, Brainerd and Stein2013; Kensinger & Corker, Reference Kensinger and Corkin2003). Similar to concrete words, valenced words are acquired earlier in the lifespan than neutral words (Ponari et al., Reference Ponari, Norbury and Vigliocco2016), and children are shown to learn novel abstract words better when they are valenced compared to neutral abstract words (Ponari et al., Reference Ponari, Norbury and Vigliocco2020). Adult language learners likewise performed better at learning L2 words in backward translation tasks when they were positively or negatively valenced (Ferré et al., Reference Ferré, Ventura, Comesaña and Fraga2015).
One explanation for these concreteness and valence effects is found in theories of embodied cognition, which propose that cognition is grounded in modal simulations, bodily states, and situated actions (e.g., Andrews et al., Reference Andrews, Frank and Vigliocco2014; Barsalou, Reference Barsalou2009; Barsalou et al., Reference Barsalou, Simmons, Barbey and Wilson2003; Decety & Grezes, Reference Decety and Grèzes2006; Moffat et al., Reference Moffat, Siakaluk, Sidhu and Pexman2015; Wilson-Mendenhall et al., Reference Wilson-Mendenhall, Barrett, Simmons and Barsalou2011). Under an embodied account, semantic representations and processing are grounded in sensorimotor and affective information associated with the word meaning and learning context (e.g., Kousta et al., Reference Kousta, Vigliocco, Vinson, Andrews and Del Campo2011; Meteyard et al., Reference Meteyard, Cuadrado, Bahrami and Vigliocco2012; Ponari et al., Reference Ponari, Norbury and Vigliocco2016; Vigliocco et al., Reference Vigliocco, Meteyard, Andrews and Kousta2009, among many others). Learning a word means developing word-to-world mappings; therefore, the richer the semantic representation, the easier it will be to make these mappings (Gleitman et al., Reference Gleitman, Cassidy, Nappa, Papafragou and Trueswell2005; Vigliocco et al, Reference Vigliocco, Meteyard, Andrews and Kousta2009). Hence, concrete words are processed faster than abstract words, because their referents are highly perceptual and thus activate sensorimotor simulations and pathways more readily. In a similar way, valenced words are processed faster than neutral words, because their referents activate emotional states, a type of internal perceptual experience (see Ponari et al., Reference Ponari, Norbury and Vigliocco2016; and Meteyard et al., Reference Meteyard, Cuadrado, Bahrami and Vigliocco2012 and Vigliocco et al., Reference Vigliocco, Meteyard, Andrews and Kousta2009 for review). This study examines the proposed influence of word concreteness and valence in the real-world scenario of a language learning app.
Context effects
One underlying goal of understanding the effects of word learning is to make language learning faster and better. The findings suggest which words are easier and harder to learn. However, in educational practice, a language app or instructor cannot simply avoid teaching difficult-to-learn words. Learners need both abstract and concrete words as well as valenced and neutral words to reach meaningful L2 proficiency. What can be manipulated is the linguistic context in which target words are learned. This reality requires a thorough understanding of the role that linguistic context may play in word learning.
Previous studies have found that the semantics of the linguistic context in which words are learned does indeed impact learning. Snefjella and Kuperman (Reference Snefjella and Kuperman2016) consider the average concreteness, valence, or arousal of lexical contexts in which a word occurs in natural language. These semantic properties of the word’s context are found to explain variance in lexical processing and recognition memory over and above the semantics of the word itself. In their mega-study including over 4800 words, Cortese and Khanna (Reference Cortese and Khanna2022) similarly found that both extreme positive and negative contexts boost memory for words.
A later study, Snefjella et al. (Reference Snefjella, Lana and Kuperman2020), exposed English L1 adults to novel words with concrete meanings in either positive, negative, or neutral contexts. Learners showed higher accuracy on orthographic and semantic vocabulary post-tests for novel words presented in positive contexts, lower accuracy for those in neutral contexts, and the lowest accuracy for those in negative ones. Lana and Kuperman (Reference Lana and Kuperman2023) replicated this finding for novel words with concrete and abstract meanings. Frances et al. (Reference Frances, De Bruin and Duñabeitia2020) similarly found positive contexts to boost learning more than neutral ones, although their study did not include words learned in negative contexts. Contrary to Snefjella et al. (Reference Snefjella, Lana and Kuperman2020), Driver (Reference Driver2022) reported that both positively and negatively valenced words and contexts harmed rather than facilitated L2 vocabulary learning.
These conflicting findings call into question whether context effects on semantics are found in real-world learning scenarios and, if they are, what shape those effects take. Regarding context valence, existing theories of affective processing lead to differing predictions. The embodied cognition account supports U-shaped effects because it argues that both positive and negative valence enrich semantic representations. The U-shaped effect can also be explained from a memory and attention perspective. Several studies have shown that emotionally arousing stimuli are more attention-grabbing and remembered more accurately than non-arousing neutral stimuli (e.g., D’Argembeau & Van der Linden, Reference D’Argembeau and Van der Linden2004; Doerksen & Shimamura, Reference Doerksen and Shimamura2001; Kensinger & Corkin, Reference Kensinger and Corkin2003; Vuilleumier, Reference Vuilleumier2005). Thus, we would predict that both positively and negatively valenced words will be recalled better than more neutral words. Similarly, words presented in valenced-context sentences are also expected to be learned better than those presented in neutral contexts.
Alternatively, better learning for novel words in positive rather than neutral and negative contexts, as reported in Snefjella et al. (Reference Snefjella, Lana and Kuperman2020) and Lana and Kuperman (Reference Lana and Kuperman2023), is predicted by another attention mechanism. The automatic vigilance theory posits a threat-driven attentional mechanism where more threatening (negative) stimuli take longer to process and slowed response times (e.g., Algom et al., Reference Algom, Chajut and Lev2004; Erdelyi, Reference Erdelyi1974; Estes & Adelman, Reference Estes and Adelman2008). This focus on negative stimuli comes at the expense of peripheral details compared to positive stimuli (e.g., Christianson & Loftus, Reference Christianson and Loftus1990; Derryberry & Reed, Reference Derryberry and Reed1998; Rowe et al., Reference Rowe, Hirsh and Anderson2007). Therefore, in word learning, we could expect positive words to be learned better because they allow for a broader attentional focus, allowing the learner to better utilize peripheral semantic information about the word itself and its surrounding context.
There is little research into the effect of context concreteness on word learning, and existing experimental work does not find strong support for this effect in lab-based studies (Lana & Kuperman, Reference Lana and Kuperman2023). Below, we outline how the present study examines the effects of valence and concreteness both for words learned in the L2 and their linguistic contexts.
The present study
While the existing literature shows strong support for the roles of word concreteness and valence, and some support for context concreteness and valence in word learning, prior work also has its limitations. Many of these studies were highly controlled experiments using carefully selected stimuli. For instance, Ponari et al. (Reference Ponari, Norbury and Vigliocco2020) selected words from a pool of 5–7 letter long words that had available subtitle frequency data and normative ratings for age of acquisition, valence, and concreteness. Missing values for such ratings are not necessarily randomly distributed across the lexicon (Snefjella & Blank, Reference Snefjella and Blank2020). In the case of Snefjella et al. (Reference Snefjella, Lana and Kuperman2020) and Frances et al. (Reference Frances, De Bruin and Duñabeitia2020), participants learned pseudowords: This task reduces the ecological validity and generalizability of findings compared to natural language stimuli. Furthermore, participants in these studies are typically exposed to relatively small sets of novel target words; as few as 9 words in the case of Snefjella et al. (Reference Snefjella, Lana and Kuperman2020). Finally, either the novel words to be learned, their contexts, or both are selected to represent highly divergent values of critical predictors, e.g., valence or concreteness, rather than the range of values naturally occurring in a language.
The necessarily artificial nature of stimuli and tasks in laboratory experimentation and corpus research begs the question of whether the relatively small effects of semantic variables like valence and concreteness survive in noisier learning conditions and larger datasets. While controlled experiments are unquestionably useful tools for controlling noise and isolating variables of interest, one of their limitations is the generalizability of their findings to the real-world demands of say learning a second language where learners must acquire thousands of words with a wide range of lexicosemantic properties. This paper seeks to complement such experimental work and respond to these limitations by exploring word learning effects in the wild—outside of highly controlled experiments, namely, in a language learning app called Lingvist.
Why a language learning app?
Such apps provide access to valuable data. First, language learning apps accumulate performance from thousands to millions of users learning thousands of words in their target language. This massive amount of data provides a significant and highly desirable improvement to the statistical power of second language learning studies (Brysbaert, Reference Brysbaert2021). Second, language apps expose learners to greater lexical, syntactic, and contextual diversity than they may see in a controlled experiment where the stimuli are carefully selected. Third, app users tend to have multiple exposures to target words or contexts, allowing us to study how semantic effects on learning change in size over the course of the learning experience. Another benefit of language apps is that they are highly accessible and appeal to a variety of learners; therefore, their data represent users coming from a range of ages, occupations, and educational backgrounds. Finally, language apps differ from controlled experiments with regard to learner motivation and commitment. Participants in experiments are compensated for their participation and typically engage with the stimuli in a single hour-long session or shorter. In contrast, users of language apps often pay a subscription fee to learn and they engage with the app at their own discretion over the course of months or years. Therefore, language apps can provide unique and highly powered insights into the factors affecting word learning, particularly in a second language.
The present study uses learner data from the Lingvist language learning app to explore the effects of semantic properties on L2 word learning. In particular, we focus on how target word valence and concreteness as well as context valence and concreteness impact learning accuracy. The first multifaceted goal of this study is to determine (i) whether the valence of the word or the context influences word leaning and what shape these valence effects take, (ii) whether and how word valence and context valence interact, and (iii) how valence effects unfold across multiple encounters with a word. As a second goal, the study asks the same questions with regard to concreteness. While the word concreteness advantage has been widely demonstrated in word learning, evidence for the potential effect of context concreteness is sparce (see the Introduction). An additional third goal of this study is to extend the current body of literature demonstrating how Big Data from increasingly popular language learning apps can shed light on questions of long-standing interest for psycholinguists (Hopman et al., Reference Hopman, Thompson, Austerweil and Lupyan2018; Skalicky et al., Reference Skalicky, Crossley and Berger2019).
Methods
The study used secondary data that were originally collected by Lingvist Technologies, a language-learning app. The data were shared under a collaboration agreement, and ethics clearance #5923 was obtained from the research ethics board of the authors’ university in May 2022. The study was not preregistered. The data and code of this paper, including the Supplementary Materials, are available on the project’s OSF page: https://osf.io/78jsu/.
Lingvist app and data
The Lingvist app is a digital language learning tool focused on building the learner’s vocabulary. It currently supports 47 pairings of target languages (the language to be learned) and source languages (the language known to the user). The source language is not necessarily the learner’s first language (L1); however, learners are encouraged to select the available language in which they are most proficient.
The translation task
In the most common task of the app, learners are presented with a fill-in-the-blank sentence in the target language and a matched complete translation of the sentence in the source language. For example, if the target language is Spanish and the source language is English, as it was in this study, the learner would see trials like (1). Note that the prompt also includes grammatical cues such as gender and number indicators for nouns or tense and number indicators for verbs.

The learner then types in the missing target word (the correct answer being mayoría) and is provided with immediate feedback. When the learner is incorrect, the correct response appears in red and an audio recording of the target word plays. If the learner’s response was partially correct, the correct letters appear in green and the incorrect letters appear in red. When the learner enters a synonym for the target word, no audio is played, and the response box appears in orange. A visual prompt also appears indicating that the learner has found a synonym, but that the app is trying to teach them a different word, and then, the first letter of the desired target word is displayed. Responding with a synonym to the target word does not count as an error. When the learner types the correct response, an audio recording of the complete sentence is played, and the target word appears in green. Once the correct response has been submitted, the next trial appears.
The app employs spaced repetition to boost learning. As such, learners encounter target words multiple times. The number of times and how soon the learner encounters the same target word depends on their previous performance. Words that the user consistently gives correct responses are repeated less and the repetitions are spaced farther apart.
Participants
We analyzed learning data from 4,665 Lingvist users who selected Spanish as their target language and English as their source language (i.e., the language of the full translated sentence) from April 2020 to April 2022. The app collects relatively little meta-data on the demographic or linguistic background of the users. The learners’ age, level of education, proficiency in the source and target language, and what other languages they know or are learning are unknown. Learners pay a subscription fee to use the app and learning is self-paced in the sense that learners decide how many trials they complete in a session and how often they interact with the app. All learners were active users at the time the data were collected, meaning they completed at least one trial in the 3 months prior to data extraction.
Variables
The dependent variable of this study was response accuracy which is a measure of how orthographically close the user’s answer is to the target response. Response accuracy is scored from 0 to 1 based on string-edit distance from the correct answer, where a score of 1 indicates a perfect match. Close synonyms are also accepted as correct responses, receiving a score of 1.
In line with the research questions outlined in the Introduction, the following independent variables were considered critical for this study: the valence and concreteness of the target word, and the valence and concreteness of the context. As a control, we also considered word arousal and context arousal. These variables were estimated for the English (source language) translations of the target words, as well as for the contexts. We opted for source language (English) measures over those in the target language (Spanish), because this is the language in which the learners are most proficient and have developed semantic representations for the words, while their semantic representations for the target language words are under development. Valence and arousal ratings for the target English words (e.g., Most in Example 1) were obtained from Warriner et al. (Reference Warriner, Kuperman and Brysbaert2013), which report average ratings per word for valence on the scale from 1 (sad) to 9 (happy) and for arousal on the scale from 1 (calm) to 9 (arousing). Concreteness ratings were obtained from Brysbaert et al. (Reference Brysbaert, Warriner and Kuperman2014), on the scale from 1 (abstract) to 5 (concrete). In cases where there were multiple translations for the target word, we took the mean valence, arousal, and concreteness value from all translations of the word found in the dataset.
To estimate the respective values for the context sentences (e.g., Most people walk to work in that town.), stop-words (i.e., function words and modal verbs, including to, in, that), and target words (i.e., most) were first removed. Then, the concreteness, valence, and arousal ratings were matched to the remaining words (people, walk, work, town). Concreteness, arousal, and valence of the context were defined as the mean of the available respective ratings across all words in the context. As with the target words, when there were multiple translations, the mean value from all given translations of context was used.
A number of control variables were additionally accounted for. Log frequency of the target word (e.g., most) in the source language (English) was obtained from the 51-million-token corpus SUBTLEX-US of subtitles for US media and films (Brysbaert & New, Reference Brysbaert and New2009). The Levenshtein distance between the target word and source language translation (defined as the number of orthographic insertions, deletions, and replacements needed to transform one-word form into another) was calculated for every Spanish target word and English translation. The Levenshtein distance is a measure of cross-linguistic orthographic similarity and is especially low when the word pairs in the two languages are cognates or borrowings (competición vs. competition produced the Levenshtein distance of 1). In cases where there were multiple translations, we used the Levenshtein distance between the target word and the orthographically closest translation (i.e., the minimum Levenshtein distance). Additional control variables were the number of errors in the previous encounters with the target word and success on the immediately preceding trial. We also included the log-transformed number of trials that the user had completed in the app at the time of response as a measure of the user’s experience with the app. Finally, we looked at the log-transformed “age of acquisition” in the app, i.e., the ordinal number of the trial in which the learner encountered the given target word for the first time while using the app.
Data cleaning and trimming
The raw data contained information from 6,513 unique users learning 13,419 target words over 11.7 million trials. The following data preprocessing and trimming steps were applied. First, as our interest is in how context impacts learning, trials where the target word appeared in isolation (i.e., not embedded in a context) were removed (n = 200,583). Second, any trials presenting a target word for which concreteness, valence, or arousal ratings were not available were removed (n = 3,763,950). Contexts containing less than 4 words with non-missing values for concreteness, valence, and arousal were also removed (n = 5,516,946). Additionally, 21 rows were removed which contained invalid values for minimum Levenshtein distance. We further limited our investigation to the first five encounters with the target words. There were far fewer users encountering fewer target words 6 or more times, leading to sample sizes that were an order of magnitude smaller than those for the first five encounters. Therefore, we removed observations for the target words’ sixth encounter and beyond (n = 995,498). Finally, our investigation centers on effects on the learning process during the use of the app rather than the user’s prior knowledge. The first encounter the user had with a word was recorded before the correct L2 target was revealed to the user. We reasoned that response accuracy to that first encounter reflects that user’s prior word knowledge rather than learning within the app. Therefore, we excluded observations from users’ first encounters with the target word (n = 426,186). After all trimming procedures described above, the cleaned dataset contained 832,565 observations from 4,665 users responding to 4,319 unique target words. The final sample size for encounter two was N = 258,580, for encounter three N = 211,089, for encounter four N = 194,415, and for encounter five N = 168,361 (see Table 1 and Supplementary Materials for additional details).
Table 1. Predicted accuracy and effect size of word valence across encounters with context valence held constant
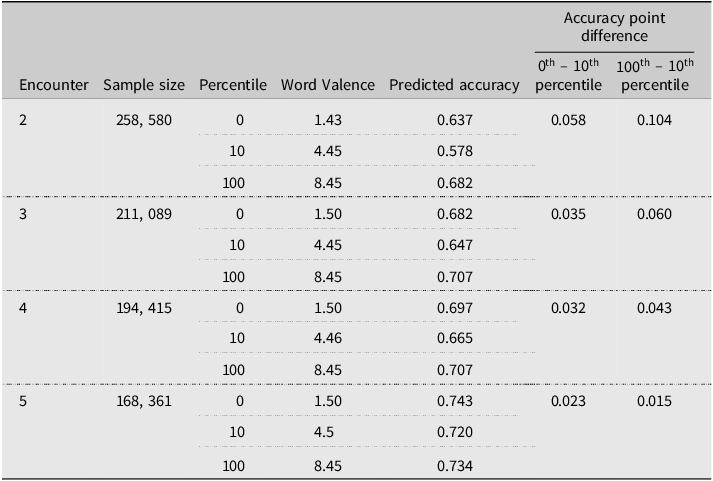
Statistical considerations
We used generalized additive mixed models (GAMMs) to model the effects of valence and concreteness of the target word and context on word learning. GAMMs extend standard linear modeling by combining a series of non-linear smoothing functions calculated for each predictor in the model (Gareth et al., Reference Gareth, Daniela, Trevor and Robert2013; Wood, Reference Wood2017). This mathematical framework makes modeling non-linear functional relationships between individual predictors and the dependent variable possible using splines, as well as modeling nonplanar surfaces to represent interactions between predictors using tensor products. These features are particularly useful for research goal (i), which is concerned with the shape of the valence effect. Furthermore, GAMMs allow for multivariate analysis and random effects structures, thus accounting for multiple predictors and within-participant and within-item variability.
The model included response accuracy (a continuous variable with a 0-to-1 range) at a specific encounter with the target word as the dependent variable. In line with our research goal (i), the main predictors of interest were the valence of the target word and valence of the context, as well as concreteness of the target word and the context. Prior research suggested that semantic properties of words and contexts are separable and can show a multiplicative effect on word learning or processing (see the Introduction). For this reason, and in line with goal (ii), tensor product smooths were used to model the potentially nonplanar interactions between the valence of the target word and context, and between the concreteness of the target word and context, respectively.
Additional controls included log word frequency, arousal, and Levenshtein distance between the target word and source language translation as a measure of L1–L2 “cognateness.” Furthermore, we included the log number of trials completed, the ordinal number of the first encounter with the word, the number of errors in previous encounters with the word, and success on the previous trial as additional controls. Visual inspection of the effects of Levenshtein distance, word frequency in the source language, the current number of trials completed, and the ordinal trial number of the word’s first appearance on accuracy suggested that they were nonlinear. Therefore, splines were used to model these effects. Target word arousal, context arousal, previous errors on the target word, and success on the previous trial were modeled as parametric (linear) coefficients. Finally, the model included random intercepts for users and target words and random slopes for valence and concreteness of the target word by user.
We used the mgcv package in R (Wood, Reference Wood2011) and the following formula to fit the models:
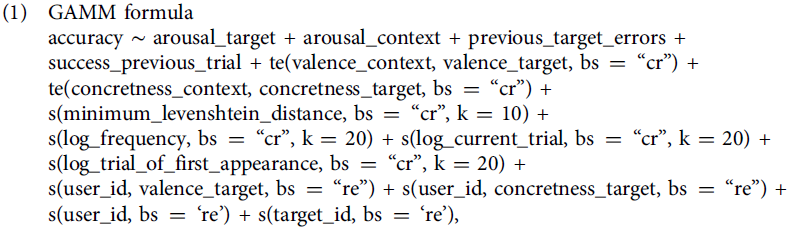
where te() stands for the tensor product and s() for a smooth spline-based function.
To explore goal (iii)—how do valence effects unfold over subsequent encounters with the target word—we fit a separate model with the structure described above to responses at encounters two to five with the target word. The findings are presented in the Results section.
Results
To investigate the semantic effects of the target word and context on L2 word learning, we analyzed user responses from the second to fifth encounters with the target word. We begin with addressing research goals (i)–(iii) with regard to word and context valence and then, in turn, with regard to concreteness.
Valence effects
The word valence x context valence interaction term was significant (p < 0.001) in all four models (encounters 2–5 with the target word), see Supplementary Materials for full details. Figure 1 shows the interactive effect of word valence and context valence on response accuracy for users’ second encounter with the target word (N = 258,580), which reflects the initial stage of word learning in the app. The plot demonstrates a clear U-shaped effect of word valence on learning, where learners are more accurate when responding to low-valence (negative) words and high-valence (positive) words compared to neutral words.
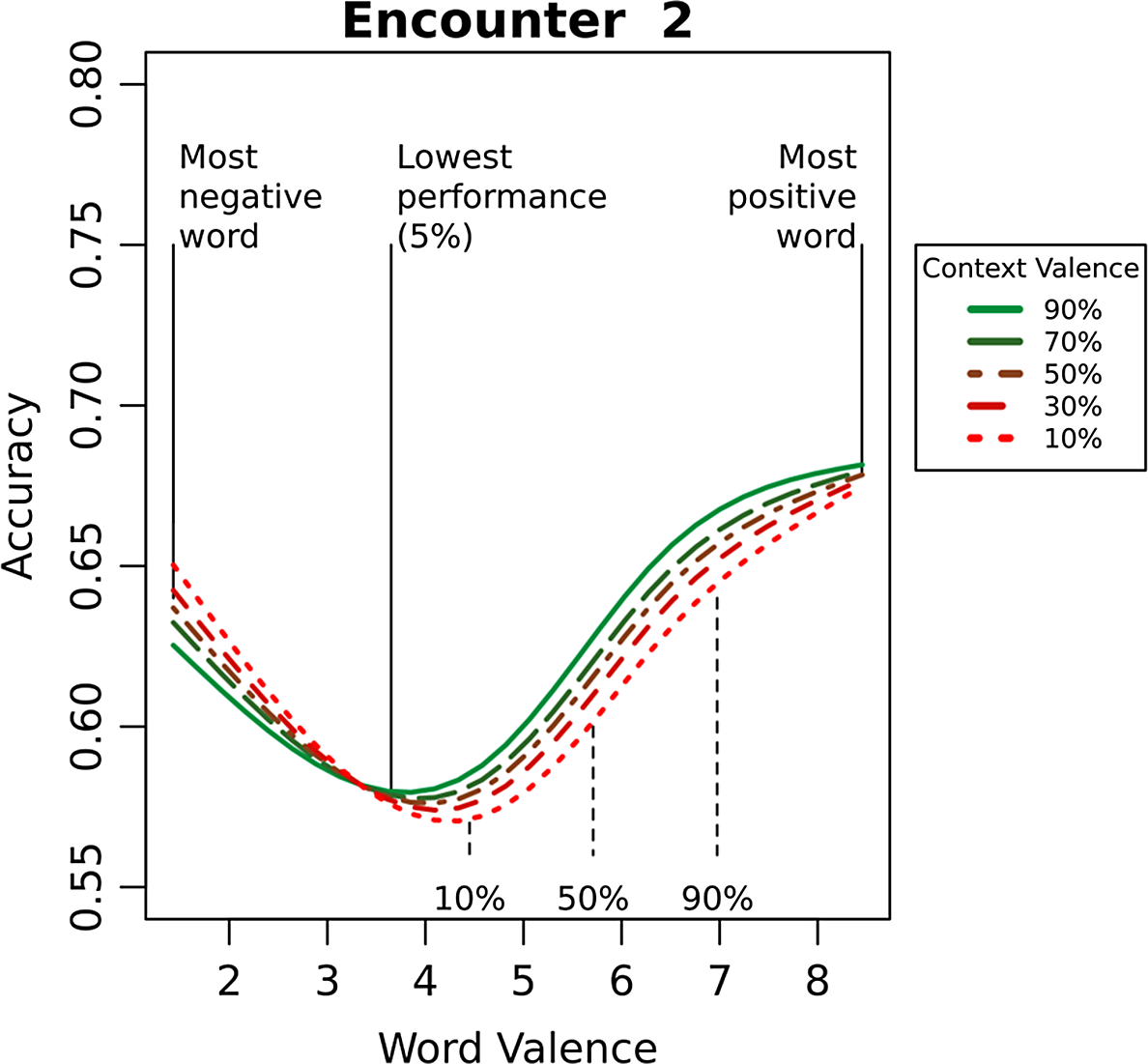
Figure 1. Accuracy on second encounter with target word by word and context valence.
Note: Solid vertical lines mark the most negative word (0th percentile of word valence), point of lowest performance (5th percentile of word valence), and most positive word (100th percentile of word valence). Dashed vertical lines indicate the 10th, 50th, and 90th percentiles of word valences.
To quantify the size of the main effect of word valence in the second encounter with the target word, we report the predicted accuracy values for every 5 percentile points in the word valence distribution while holding all other continuous predictors constant at their median values and categorical predictors at their reference levels, see Table 2. The lowest predicted accuracy was observed among target words at the 5th and 10th percentiles of the word valence distribution (valence = 3.65 and 4.45, predicted accuracy = .578 and .579). Given that the effective valence scale ranged from 1.43 to 8.45 with a median of 5.71 points, such values of valence qualify the words as slightly negative (see Table 2). Compared to this minimum of accuracy, users experience a 5.8% boost in predicted accuracy for the most negative words (valence = 1.43, accuracy = 0.637) and a 10.0% boost for the most positive words (valence = 8.45, accuracy = 0.679). Although the negativity boost is observed only in the bottom 10% of the word valence distribution (between the minimum observed valence and the 10th percentile), it should be noted that 10% out of the total 258,580 observations used to model encounter 2 is still over 25,000 observations, i.e., a sample size that affords superior statistical power.
Table 2. Predicted accuracy by word valence on second encounter with target word
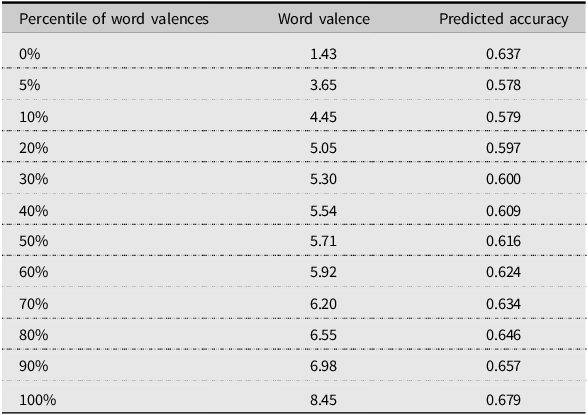
We now describe and quantify the effect of context valence in interaction with word valence. Users show higher accuracy when positive words are presented in more positive contexts, or when negative words are presented in more negative contexts, compared to the mixed cases. That is, congruency between the target word valence and context valence boosts initial learning (in encounter 2). Figure 1 plots the effects of word valence estimated at the 10th, 30th, 50th, 70th, and 90th percentiles of the context valence distribution. Table 3 reports the predicted accuracies by word and context valence for encounter 2. Users are 7.7% more accurate at learning positive words in the most positive contexts (0.684) than they are at learning negative words in the most positive contexts (0.607). Similarly, learners are 7.1% more accurate at learning negative words in the most negative contexts (0.685) compared to negative words in the most positive contexts (0.614). Although the range of context valences is all on the positive side of the valence scale, we see a clear trend that the more negative the context is, the more accurately users perform on negative words and vice versa for the positive words.
Table 3. Predicted accuracy on the second encounter with the target word by word and context valence
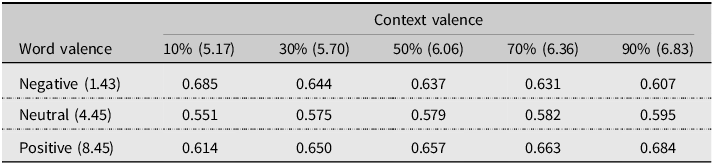
Finally, to investigate research goal (iii), how valence effects unfold over subsequent exposures to the target word, we consider the GAMM model fits across the second to fifth encounters with the target word (see Methods for justification). Figure 2 plots the word valence x context valence interaction as a predictor of accuracy across encounters. As expected, users’ overall accuracy increases across subsequent encounters with the target word. Moreover, the characteristic U-shape of the word valence effect remains the same throughout the word learning. However, most importantly for this study, the plot shows that valence effects are strongest for the second encounter with the target word. That is, the greatest accuracy boost for extremely valenced words over neutral words occurs on encounter 2 and flattens out over subsequent encounters. Additionally, the congruent interaction between context valence and target word valence is only seen in encounter 2 and disappears in encounters 3, 4, and 5. In summary, valence effects, whether carried by the word to be learned or its context, gradually diminish as the word’s meaning becomes entrenched in the learner’s mental lexicon.
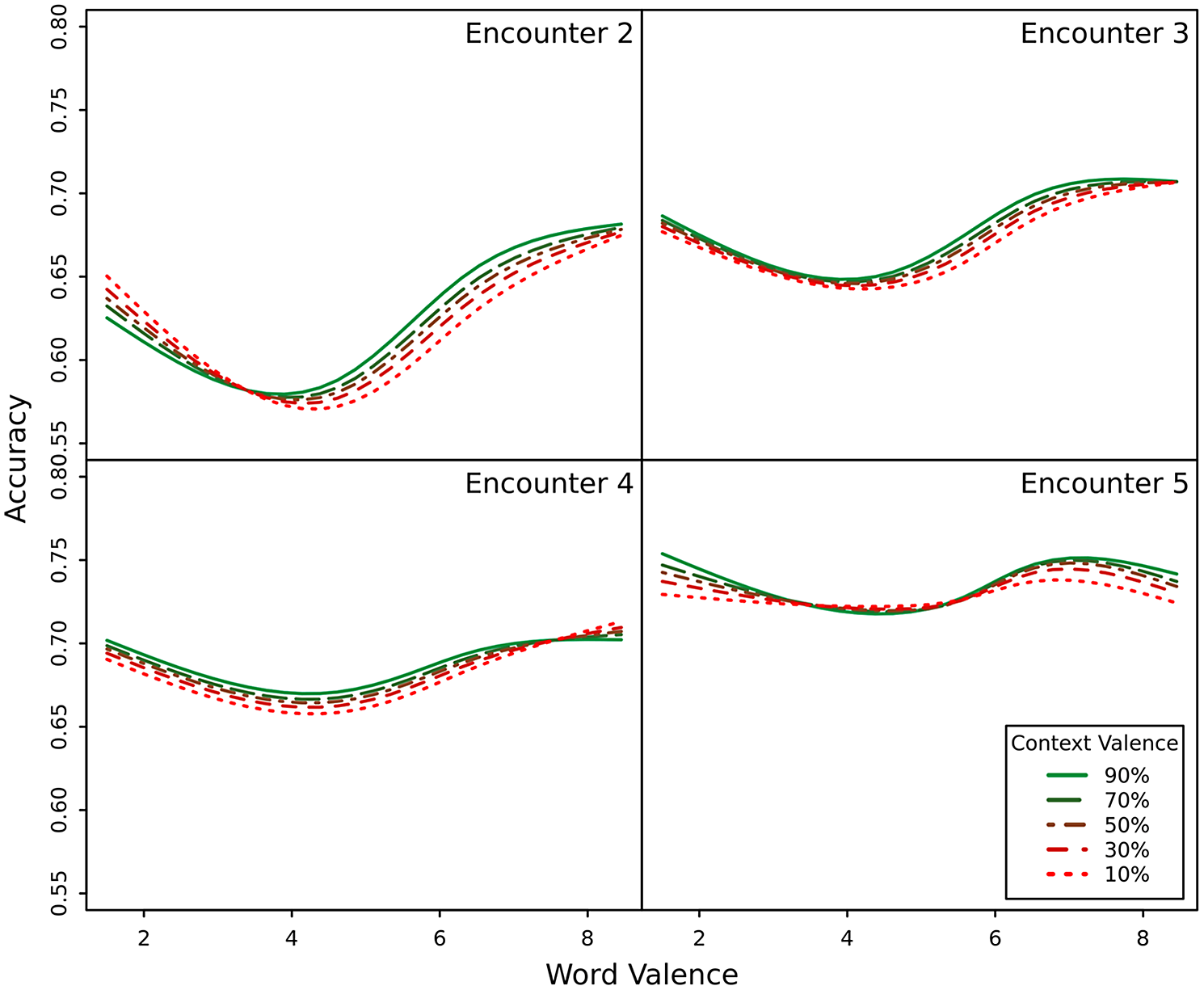
Figure 2. Accuracy by word and context valence for encounters 2 through 5 with the target word.
Table 1 reports predicted accuracy and word valence effect sizes (in percent difference) across encounters while holding all other predictors constant.
Concreteness effects
As our second goal, we considered the interaction between word and context concreteness across encounters. Like with valence, the word concreteness x context concreteness interaction term was significant (p < 0.001) in each model, see Supplementary Materials for full details. Figure 3 plots the concreteness interaction as a predictor of accuracy across encounters. Word concreteness effectively showed a flat effect on accuracy with minor fluctuations at the extreme ends of the word concreteness scale. While statistically significant, the interaction produced effects that were practically unimportant and possibly due to the edge effects that GAMM may demonstrate (Webster et al., Reference Webster, Vieira, Weinberg and Aschengrau2006).
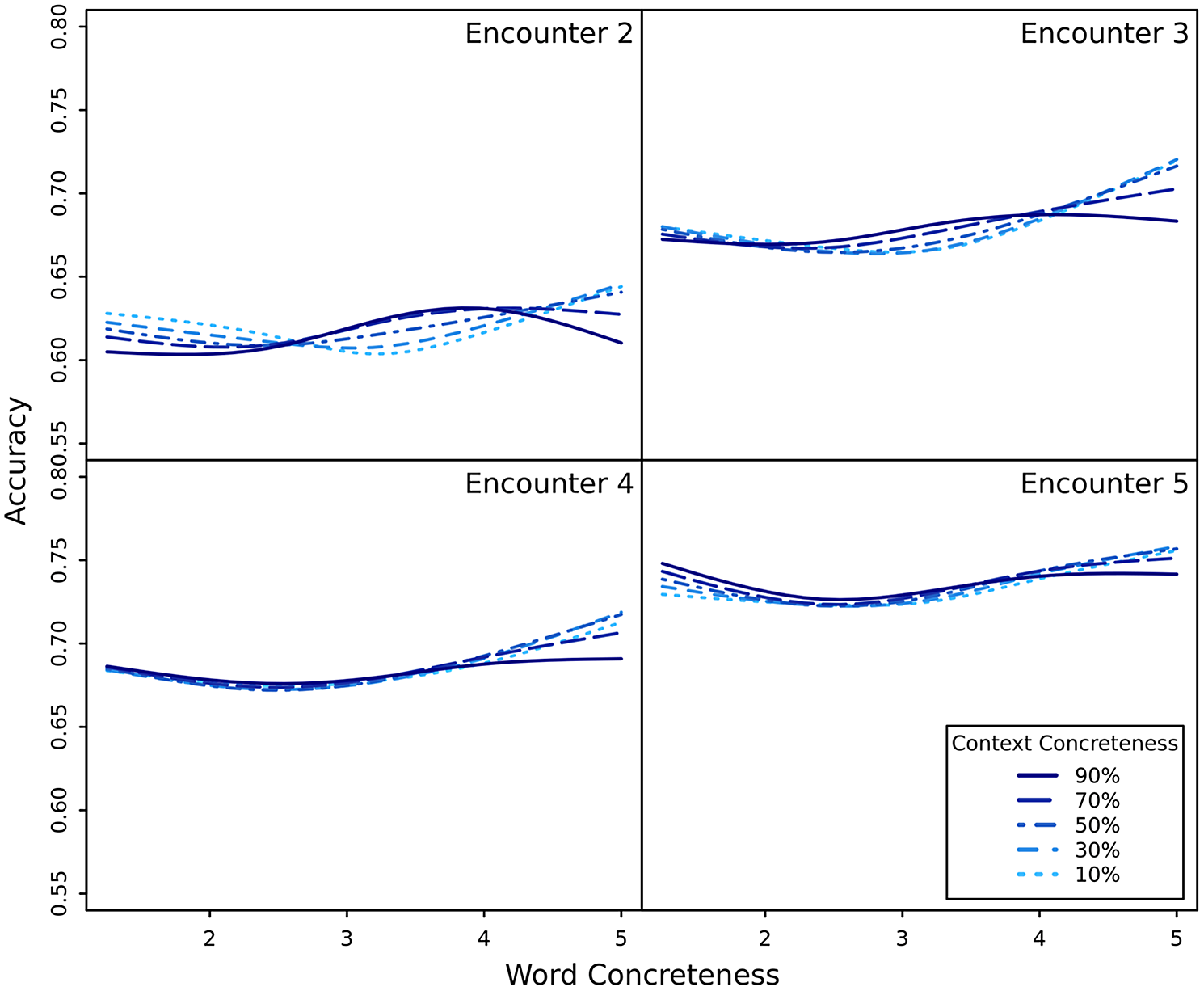
Figure 3. Accuracy by word and context concreteness for encounters 2 through 5 with the target word.
Other effects
As described in the Methods section, a number of control variables were also included in the GAMMs. In each of the second through fifth encounters, Levenshtein distance, log number of trials completed, log ordinal number of first encounter with the target word, errors in previous encounters with the word, and success on the user’s previous trial were all significant predictors of accuracy (all p < 0.01, see Supplementary Materials). As Levenshtein distance between the target word and source language translation increased, accuracy decreased across all four encounters (Figure S1 in Supplementary Materials): This confirms the well-described processing and learning advantage for cognate words. With regard to the log number of trials completed, in encounter 2, the earlier the user encountered the word in their history of app use, the better their accuracy. Meanwhile, in encounters 3, 4, and 5, the effect of the number of trials completed is inverse-U shaped where accuracy improves the more trials the user completes, up to a point, and then begins to decrease (Figure S2 in Supplemental Materials).
We also found that words that are first encountered later in the course of using the app are learned better (Figure S3 in Supplementary materials). This likely indicates the effect of increasing proficiency of the app user. A further finding was that users tend to be less accurate on words they have previously answered incorrectly, and in general, accuracy decreases the more incorrect responses that the user gave (Figure S4 in Supplementary Materials). Finally, success on the previous trial led to higher accuracy on the current trial for encounters 2, 3, and 5 with the target word, while the reverse was true for encounter 4 (Figure S5 in Supplementary Materials).
Notably, neither arousal of the context nor log word frequency were significant predictors of learning in any of the encounters (all p > 0.25). Arousal of the target word approached significance for encounter 2 (p = 0.084) and encounter 3 (p = 0.070), and reached significance in encounters 4 and 5 (both p < 0.01). As arousal rating of the target word increased, accuracy also improved (Figure S6 in Supplemental Materials).
In summary, the results show that valence indeed has an effect on word learning in a second language. Specifically, the valence of the target word has a U-shaped effect on learning where learners are more successful at recalling positive and negative words than neutral words. Furthermore, the valence of the context in which the target word appears also impacts learning. The valence of the context interacts congruently with target word valence, such that positive words presented in positive contexts and negative words in negative contexts resulted in higher accuracy than mismatched cases. Both of the valence effects described above are strongest on the second encounter with the target word and diminish over subsequent encounters. Finally, while the results indicated that the concreteness interactions were statistically significant across encounters, the effects of either word concreteness or context concreteness are practically unimportant and do not translate into a noticeable change in the learning quality. These findings are discussed in detail below.
Discussion
One of the nagging questions in applied linguistics is how to make language learning faster and more efficient. Acquiring vocabulary is an essential part of becoming proficient in a second language, and while many word learning effects are well established by controlled experiments and corpus studies (see Introduction), few have been tested against the Big Data of real-world learning scenarios. This study used a large-scale dataset from the language learning app Lingvist to gain insights into how valence and concreteness impact word learning success. We focus on two sources of semantic information known to have separable effects on word processing (Snefjella & Kuperman, Reference Snefjella and Blank2020): the word as the target of the learning and the linguistic context in which the word occurs. Specifically, we investigated (i) whether the valence and concreteness of target words and their contexts influence word leaning and the shape that these effects take; (ii) how valence and concreteness of target words and linguistic contexts interact; and (iii) how valence and concreteness effects unfold across subsequent encounters with a target word.
Valence
In line with prior research, both positively and negatively valenced words were recalled more accurately than neutral words (Kensinger & Corker, Reference Kensinger and Corkin2003; Ferré et al., Reference Ferré, García, Fraga, Sánchez-Casas and Molero2010; Adelman & Estes, Reference Adelman and Estes2013; Gomes et al., Reference Gomes, Brainerd and Stein2013; Ferré et al., Reference Ferré, Ventura, Comesaña and Fraga2015; Ponari et al., Reference Ponari, Norbury and Vigliocco2020). The effect size was largest on the second encounter, where valenced words experienced a 5.8% and 10.0% boost for the most negative and most positive words, respectively. This finding confirms that word valence effects are robust and survive even in the noisy data of real-world learning.
Concerning the shape of valence effects in L2 word learning, we found that word valence had a U-shaped effect, as opposed to the general positivity advantage reported in Snefjella et al. (Reference Snefjella, Lana and Kuperman2020) and Lana and Kuperman (Reference Lana and Kuperman2023). Furthermore, we found the advantage in the opposite direction of Driver (Reference Driver2022), with the advantage for valenced words over neutral ones. These effects cannot be explained by automatic vigilance, since negative words also facilitated learning. These results are more in line with previous studies which report enhanced attention and memory for emotional stimuli (e.g., D’Argembeau & Van der Linden, Reference D’Argembeau and Van der Linden2004; Doerksen & Shimamura, Reference Doerksen and Shimamura2001; Kensinger & Corkin, Reference Kensinger and Corkin2003; Vuilleumier, Reference Vuilleumier2005). Emotional words and emotional contexts are more attention-grabbing, thus facilitating learning and recall over neutral words and contexts. The U-shaped effect of valence can also be understood through the lens of embodied cognition which predicts that more semantic information, including valence information, supports word learning (e.g., Vigliocco et al., Reference Vigliocco, Meteyard, Andrews and Kousta2009). We argue that the affective information associated with valenced words enriches their semantic representation to a greater degree than neutral words, thus facilitating the mapping of form and meaning, leading to superior lexical quality (Perfetti, Reference Perfetti2007) and learning quality.
Moreover, we found that context valence interacts congruently with target word valence. That is, positive words are learned best in positive contexts, and negative words were learned better in more negative contexts, receiving a 7.7% and 7.1% boost, respectively, over their mixed conditions. For the applied goal of learning languages faster, this 7% learning boost from congruent contexts translates to learning 107 vs. 100 words in the same amount of time. This novel finding complements Snefjella et al.’s (Reference Snefjella, Lana and Kuperman2020) findings on the facilitatory role of context valence. It also expands on Frances et al. (Reference Frances, De Bruin and Duñabeitia2020) by showing that more negative contexts can also aid memory and learning, as long as they are supporting negative target words. Given that target words absorb affective quality from their linguistic context (Snefjella et al., Reference Snefjella, Lana and Kuperman2020), we propose that words appearing in congruent contexts develop more deeply entrenched affective representations than words in mixed conditions. Thus, following the argument above, richer semantic representations improve lexical quality which in turn supports learning (Perfetti, Reference Perfetti2007).
Concreteness
In contrast to the valence findings, the effects of concreteness in app-based word learning were less clear-cut. According to prior studies (see the Introduction), users are expected to have higher accuracy for more concrete words than more abstract ones. However, we found that concreteness had essentially no effect on word learning. This is surprising given the role concreteness plays in lexical processing and memory (de Groot & Keijzer, Reference de Groot and Keijzer2000; Ferré et al., Reference Ferré, Ventura, Comesaña and Fraga2015; Gee et al., Reference Gee, Nelson and Krawczyk1999; Kaushanskaya & Rechtzigel, Reference Kaushanskaya and Rechtzigel2012; Paivio et al., Reference Paivio, Yuille and Smythe1966; Romani et al., Reference Romani, Mcalpine and Martin2008). Hopman et al. (Reference Hopman, Thompson, Austerweil and Lupyan2018) used data from Duolingo, another language app, to investigate select predictors of words learning. They similarly reported unreliable concreteness effects on accuracy where some user groups experienced a slight boost for more concrete words and other groups experienced a slight disadvantage. Therefore, there is growing evidence that concreteness may not be as important a predictor of learning success, at least in app-based learning, as it is for lexical processing.
One explanation for the lack of concreteness effects and simultaneous presence of valence effects in language app data may be the difference between L1 and L2 lexical acquisition. Children and adults learning novel words in their L1 are simultaneously forming mental representations for both the form and the meaning of the word. The faster the representation of the meaning can be developed, the faster the word can be learned; hence, words for more readily imageable concrete concepts are acquired faster than less imageable abstract concepts. In contrast, L2 learners already have well-developed mental representations for both concrete and abstract concepts in their L1 and are simply learning to map additional labels or forms to the existing concepts. Concreteness measures tap into the imageability and tangibility of the concept itself, and thus may play a minor role in the tasks where concept learning is not involved. Conversely, valence taps into the concept’s attention-grabbing and releasing properties (Algom et al., Reference Algom, Chajut and Lev2004; Fox et al., Reference Fox, Russo, Bowles and Dutton2001; Kousta et al., Reference Kousta, Vinson and Vigliocco2009; Lang et al., Reference Lang, Bradley and Cuthbert1990; Rowe et al., Reference Rowe, Hirsh and Anderson2007) and is likely influential in the tasks where form-meaning mappings need to emerge. The Lingvist translation task primarily tests users’ recall of the target word. Therefore, attention rather than concept-forming mechanisms are expected to have greater impact on performance. Notably, two of the three studies in the Introduction that report L2 concreteness effects, de Groot and Keijzer (Reference de Groot and Keijzer2000) and Kaushanskaya and Rechtzigel (Reference Kaushanskaya and Rechtzigel2012), do not control for word valence. The third study, Ferré et al. (Reference Ferré, Ventura, Comesaña and Fraga2015), does control for valence; however, the concreteness advantage significantly declined by the second session. Moreover, all three studies tested less than 50 participants on small sets (n = 12–60) of target words. Thus, it is possible that the concreteness effects seen in these small populations and tightly controlled stimuli sets are not strong enough to survive in larger noisier data from language apps. More research is needed to tease out concreteness effect differences in L1 and L2 word learning as well as differences between learning modalities. We leave this to future research.
As far as we know, the interaction between word and context concreteness in word learning has not been previously studied. While the models indicate a statistically significant interaction across encounters, it appears that the interaction may be occurring primarily in the extreme ends of word concreteness. Given that GAMMs are known to have edge effects as artifacts of their fitting algorithms, different modeling choices may clarify the picture for future investigations.
Effects across encounters
Finally, we present the novel insight into how semantic effects unfold over subsequent encounters with the target word. In general, users’ accuracy improves with additional exposures to the word as expected (Hulme et al., Reference Hulme, Shapiro and Taylor2022). Interestingly, the U-shaped effect of word valence is strongest on the second encounter with the target word and gradually flattens out by the fifth encounter. The gradual attenuation of semantic effects on word learning is in line with the theoretical premises of the lexical quality hypothesis (Perfetti, Reference Perfetti2017; Perfetti & Hart, Reference Perfetti and Hart2002). Formal and semantic properties of the word and the context in which it appears play the most prominent role during the initial mapping of the (orthographic and phonological) form to lexical meaning. The more the form and the meaning become entrenched in the mental lexicon and the more “crisp” and automatic the associations between those representations become, the less the readers need to rely on specific properties to activate the word’s form and meaning. Evidence in support of this theoretical account comes from a robust observation that more proficient readers or readers that have a better knowledge of a specific word show attenuation of even the strongest predictors of lexical processing, such as word length and word frequency (e.g., Diependaele et al., Reference Diependaele, Lemhöfer and Brysbaert2013; Kuperman & Van Dyke, Reference Kuperman and Van Dyke2011; Perfetti & Hart, Reference Perfetti and Hart2002; Slattery & Yates, Reference Slattery and Yates2018; Taylor & Perfetti, Reference Taylor and Perfetti2016). We argue that word and context valence effects follow the same trajectory. As suggested by the embodied cognition accounts, they facilitate the early stages of L2 word learning, i.e., the mapping of form and meaning. As the mapping becomes more entrenched, these effects diminish in their importance.
Another, not mutually exclusive, explanation for the diminishing effect of semantic variables over the multiple encounters with the word comes from the literature on the role of declarative memory in word learning (Baddeley, Reference Baddeley2001). Most researchers agree that early phases of word learning engage episodic memory, which encodes highly detailed and contextualized human experiences, arguably including the affective and sensorimotor facets of the word’s meaning and the word’s linguistic context. Further practice with the word (or consolidation of episodic memory traces during sleep) leads to increasing abstraction in the word representation and its transition to the domain of non-contextualized factual knowledge, subserved by semantic memory (Davis et al., Reference Davis, Di Betta, Macdonald and Gaskell2009; Davis & Gaskell, Reference Davis and Gaskell2009; Gaskell & Ellis, Reference Gaskell and Ellis2009; Hamrick, Reference Hamrick2015; Hamrick et al., Reference Hamrick, Graff and Finch2019; Ullman, Reference Ullman2004, Reference Ullman2020). As a toy example, early encounters with the new word “COVID-19” might be associated in the learner with feelings of fear conveyed through the linguistic and extra-linguistic context in which the word occurs (e.g., Luo et al., Reference Luo, Ghanei Gheshlagh, Dalvand, Saedmoucheshi and Li2021). As the word is entrenched through practice or consolidation, its semantic representation (i.e., COVID-19 is an infectious disease caused by a virus) may become more abstract and detached from the emotional and sensorimotor experiences of specific contexts. The time-course of engagement that subsystems of declarative memory show during novel word learning may explain the gradually attenuating effects of word and context valence and concreteness.
Our observation of the null effect of word frequency in the source language (English) may appear surprising given the pervasive role of frequency effects in word learning and processing (see review in Ellis, Reference Ellis2002). Yet, in a situation of learning a completely unknown foreign language, word frequency in the source language is irrelevant: The learner will lack the knowledge of all L2 words, regardless of how frequent their equivalents are in L1. The relevant frequency is L2 (target) word frequency, which in our study is controlled through the number of encounters with the word within the app. While it is possible that learners have (partial) knowledge of some L2 words prior to joining the app, and these would be the words that are more frequent in their L1 and L2, the data suggest that this prior knowledge was not sufficient for the L1 frequency effect to emerge in our statistical models.
Limitations and future directions
One limitation of the study was the amount of data loss during the trimming procedures. The vast majority of the data (∼79%) was removed due to missing values for semantic ratings.
For our goal of exploring the semantic effects of target words and linguistic contexts on word learning, it was necessary to have complete cases of target word arousal, concreteness, and valence measures, as well as ratings for a sufficient number of words in the context sentences. However, removing the data introduced bias, as normative ratings are not necessarily randomly distributed across the lexicon (Snefjella & Blank, Reference Snefjella and Blank2020). Future studies working with large amounts of natural language should consider employing statistical methods to interpolate normative ratings such as multiple imputation (see study 2 in Snefjella & Blank, Reference Snefjella and Blank2020) to reduce the amount of missingness in the data. This being said, the current study presents a significant improvement to the statistical power of previous word-learning studies (see Brysbaert, Reference Brysbaert2021). For instance, most experiments reviewed in the Introduction presented fewer than 50 target words to a few dozen to a few hundred participants. By comparison, our trimmed data contained close to 1 million observations from over 4,500 users responding to over 4,000 unique target words.
Another limitation, particularly for research goal (ii)—how target word and context valence interact—was the small range of context valence ratings found in the dataset. Context valence was defined as the average valence of non-target and nonstop words in the linguistic context. As a result, context valence in encounter 2, for instance, ranged from 5.17 (10th percentile) to 6.83 (90th percentile), suggesting that all contexts were slightly positive. By the current operationalization of context valence, we cannot draw definitive conclusions about truly negative contexts (valence ratings below 4.5). However, we can conclude that increased context positivity boosts learning for positive words, and increased negativity boosts learning for negative words.
Using average valence poses at least two problems. First, averaging valence values of constituents disguises the polarity of the context. For example, a context containing the words vacation (positive valence rating of 8.53) and murder (negative valence rating of 1.48) would have a mean valence rating of around 5.01 or slightly positive valence. A more sensitive measure of polarity could be to calculate the proportion of positive or negative words in the context. Alternatively, a measure of the degree of valence, that is, the absolute difference of the word or context valence rating from the midpoint of the valence rating scale such as in Cortese and Khanna (Reference Cortese and Khanna2022) could be used to explore how the extent of valence rather than the directionality of valence impacts learning.
The second problem with using mean context valence is that it does not account for how the words in a sentence relate to one another. For example, the overall sentiment of the sentence The child eating chocolate was hit by a bus is negative, yet the average valence of child, eat, chocolate, hit, and bus is 6.2 (moderately positive). Thus, the measure of mean context valence demonstrates a clear positivity bias. Future investigations should consider alternative measures such as context polarity or using sentiment analysis approaches that take relationships between words in a sentence into account.
Finally, while language apps provide powerful insights into more real-world language learning, they are also limited by the nature of their tasks. To start, learning is largely decontextualized as it lacks the rich social and environmental information of immersed language use or even classroom use. In a recent study, Liu et al. (Reference Liu, Jeong, Cui, Dewaele, Okamoto, Suzuki and Sugiura2024) reported a link between the intensity of foreign language learners’ social interactions and their neural representations of positive and negative words, highlighting the potential role of social interactions in processing L2 words. Furthermore, the app data in this study only captures learning through a written fill-in-the-blank translation task and thus provides a limited window into how words are learned. Future research is needed to explore other word learning tasks such as picture naming and sentence completion as well as aural/oral tasks to build a fuller picture of the factors affecting L2 word learning.
In this study, we present a first step toward complementing and validating valence and concreteness effects on word learning reported in previous experimental work (see Introduction) using Big Data from a language app. A similar approach can be applied to investigating any number of lexical properties such as socialness ratings (Diveica et al., Reference Diveica, Pexman and Binney2023), translation ambiguity (Bracken et al., Reference Bracken, Degani, Eddington and Tokowicz2017), part of speech, as well as semantic and phonological neighborhood size to name a few. The widespread popularity of language learning apps also provides opportunities for comparing word learning across platforms. Duolingo, for example, boasts its commitment to sharing data with the research community and frequently posts publicly available datasets for research and competitions (https://research.duolingo.com/).
Conclusion
Our results show that valence but not concreteness effects on word learning survive in the real-world setting of app-based learning. We report that the valence of the target word has a U-shaped effect on accuracy, where valence facilitates learning regardless of polarity. Context valence also matters, and learning is facilitated when target words are presented in congruent contexts (i.e., positive words in more positive contexts and negative words in more negative contexts). Finally, we present the novel finding that valence matters most for recall accuracy on the user’s second encounter with the target word and diminishes with subsequent encounters.
These findings have both theoretical and applied significance. On the theoretical front, finding valence effects in the wild supports embodied cognition accounts of word learning, and the attenuating semantic effects across encounters support the lexical quality hypothesis. On the applied front, word learning is an essential part of developing second language proficiency, and many learners have the underlying goal to learn faster and more efficiently. Our findings demonstrate some ways in which achieving this goal can be made easier, e.g., by making the linguistic context affectively similar to the word to be learned.
Supplementary material
To view supplementary material for this article, please visit https://doi.org/10.1017/S0142716424000304
Replication package
Replication materials and analysis for this article can be found at https://osf.io/78jsu/.
Data availability statement
Replication materials and analysis for this article can be found at https://osf.io/78jsu/. The authors are secondary users of the data for the word frequency, valence, and concreteness ratings. Frequency data were obtained from the SUBTLEX-US corpus and can be found at https://osf.io/djpqz/. Data for the concreteness ratings can be found at https://static-content.springer.com/esm/art%3A10.3758%2Fs13428-013-0403-5/MediaObjects/13428_2013_403_MOESM1_ESM.xlsx. Data for the valence ratings can be found at https://static-content.springer.com/esm/art%3A10.3758%2Fs13428-012-0314-x/MediaObjects/13428_2012_314_MOESM1_ESM.zip. The main app usage and learning data used in this study was originally collected by Lingvist Technologies and shared under a collaboration agreement.
This is proprietary data which is owned by Lingvist Technologies. Per agreement with Lingvist Technologies, the data must be treated as confidential and cannot be shared or made public. Researchers may inquire about a similar collaboration agreement by contacting Lingvist Technologies directly at media@lingvist.io.
Acknowledgments
Excerpts from this paper his paper and a published one-page abstract were presented at the International Conference on the Mental Lexicon in 2022. We thank our partners at Lingvist for providing the learner data used in this study and the reviewers for their insightful comments and feedback.
Funding statement
This work was partially supported by the Social Sciences and Humanities Research Council of Canada Partnered Research Training Grant, 895-2016-1008, (Libben, PI) and Insight Grant 435-2021-0657 (Kuperman, PI). The first author was additionally supported by the Social Sciences and Humanities Research Council of Canada – Canada Graduate Scholarships Masters (2021-2022) and Ontario Graduate Scholarships Program (2022-2023). The second author was additionally supported by the Canada Research Chair (Tier 2; Kuperman, PI), and the CFI Leaders Opportunity Fund (Kuperman, PI). We additionally acknowledge our partners at Lingvist Technologies for providing the language learning data analysed in this study.
Competing interests
The first author is a paid intern at Lingvist Technologies, the company that provided the data for this research.
Ethical standards
Ethics clearance #5923 was obtained from the McMaster Research Ethics Board in May 2022.