Introduction
The mental lexicon contains lexical information that is organized in such a way that permits its effective and automatic function in a millisecond time (Aitchison, Reference Aitchison1994; Libben and Jarema, Reference Libben and Jarema2002), its reconstruction as a result of the learning of the meaning of a new word or the learning of some new information about a known word, and the maintenance of all this information for a lifetime (Libben and Jarema, Reference Libben and Jarema2002). This organization is often described as a network of interconnected words linked by different types of associations, such as semantic, form-based, experience-based, and based on knowledge of the world (Aitchison, Reference Aitchison1994; Gudmundson, Reference Gudmundson, Bardel and Sánchez2020).
Linguistic research provides insights into the monolingual and bilingual mental lexicon using different experimental techniques and tasks, which provide indirect evidence of its organization and its structural characteristics since it is impossible to directly look into the lexicon itself. The indirect data that are provided by these tasks are the lexical production of individuals. The analysis of this lexical production and of the way in which speakers retrieve the representations of the words from their lexicon enable the extraction of information related to the structure of the mental lexicon so that we can examine it even though we do not “see” it (Abbott et al., Reference Abbott, Austerweil and Griffiths2015; Aitchison, Reference Aitchison1994; Ferrer-Xipell, Reference Ferrer-Xipell2019).
The inquiry into the organization and the structural characteristics of the mental lexicon of individuals who possess knowledge of more than one linguistic system, that is, bilinguals and multilinguals, has focused mostly on comparing it to monolingual’s mental lexicon. The current study focuses precisely on the mental lexicon of bilingual learners of a foreign language and explores it by using a semantic fluency task (of the lexical availability task type) so that we can look into the processes of lexical access and lexical selection in L1 and FL through tasks of vocabulary production. In order to represent the results of the semantic fluency task, we use advanced network tools.
Models of lexical access, selection, and production in written lexical fluency tasks
There are different theories regarding lexical processing. Here we focus on those related to the processes of lexical access, selection, and production. Specifically, we present Levelt´s speech production model because it is the most widely accepted and successful proposal for explaining the processes of access and selection (Hernández Muñoz, Reference Hernández Muñoz2006). Additionally, since our study deals with written lexical access, the Independent Network Model of Lexical Access by Caramazza (Reference Caramazza1997) is also used to explain the final stage of the process. Furthermore, the Spreading Activation model (Collins and Loftus, Reference Collins and Loftus1975; Dell, Reference Dell1986) is also accounted for here, since participants are instructed to produce as many words as possible. Finally, the Revised Hierarchical Model proposed by Kroll and Stewart (Reference Kroll and Stewart1994) and other models are presented to understand how this process is taking place in the FL.
Lexical access, selection, and production in L1 in semantic fluency tasks
According to Ferrer-Xipell (Reference Ferrer-Xipell2019), Gullberg (Reference Gullberg and Pavlenko2009), and Hernández Muñoz (Reference Hernández Muñoz2006) among others, one of the most accepted monolingual proposals in the psycholinguistic field that describes the linguistic production is Levelt’s speech production model (Levelt, Reference Levelt1989; Levelt, Roelofs and Meyer, Reference Levelt, Roelofs and Meyer1999). This model is modular as it considers speech production as a process that progresses through several sequential stages (Conceptualization, Formulation, and Articulation).
According to Levelt´s speech production model (Reference Levelt1989), there are various processing components or systems involved in the production of speech. Thus, the Conceptualizer, where various semantic concepts that align with the speaker´s communicative goal are activated, and only the most appropriate concept is finally selected. The conceptual structure/the preverbal message selected is the output of the Conceptualizer and the input for the next processing system, the Formulator.
Accordingly, the Formulator transforms the conceptual structure into a linguistic structure. This conversion includes two stages: grammatical encodingFootnote 1 of the message and phonological encoding.
During grammatical encoding, syntactic information about the activated words is retrieved, that is, information is gathered about their grammatical roles or syntactic functions (Levelt, Reference Levelt1989; Bock and Levelt, Reference Bock, Levelt and Gernsbacher1994, p. 946). Phonological encoding is the final step before the oral or written production of the word. Throughout this final processing step, information about the morphology and the phonology of the lexical item that is to be produced is retrieved from the mental lexicon. A phonetic or articulatory plan is created, i.e., an internal representation of the word (Levelt, Reference Levelt1989, p. 12). This is the output of the Formulator, which becomes the input for the Articulator. The Articulator executes the phonetic plan delivered by the Formulator through the systems that allow the articulation of speech.
However, it is important to mention that during the process of translation of the conceptual structure into lexical node or linguistic structure, lexical selection is required, because according to La Heij (Reference La Heij, Kroll and de Groot2005), Costa (Reference Costa, Bhatia and Ritchie2008), or Tomé Cornejo (Reference Tomé Cornejo2015, p. 30), among others, not every concept coincides with or has an equivalent to one single corresponding lexical item, that is, one concept may have various corresponding lexical items, for example, words that are synonyms, or homonyms, but are used in different contexts. Additionally, these activated words activate at the same time other words that are associated with them (Tomé Cornejo Reference Tomé Cornejo2015, p. 30). We understand that, at this point, the individual has to complete a complex and arduous task—the selection of the word they will finally produce.
The activated words are in competition and the lexical selection is based on the level of activation of the different activated lexical items. That is, the lexical item with the higher level of activation is eventually selected (Levelt, Reference Levelt1989; Caramazza, Reference Caramazza1997; Costa Reference Costa, Bhatia and Ritchie2008, p. 203). If many lexical items present a high level of activation, lexical selection becomes more difficult (Tomé Cornejo Reference Tomé Cornejo2015, p. 30).
Nevertheless, Levelt’s speech production model focuses on oral production, while the written production of words is treated peripherally. Our study involves a written task, so we turn to the Independent Network Model of Lexical Access by Caramazza (Reference Caramazza1997) to explain the final stage of the process: the writing of the lexical item.
According to Caramazza’s model (Reference Caramazza1997), after concept activation, the selection of lexical forms and the syntactic information about them is guided by the production modality, that is, whether the word will be produced in a written or an oral way. Caramazza (Reference Caramazza1997) reached these conclusions primarily based on evidence from language production performance in brain-damaged subjects. Specifically, it has been found that subjects make semantic errors only in speaking and not in writing, and that selective grammatical class deficits can be restricted to either oral or written production.
In experimental tasks where participants are required to provide a single answer to the prompt or stimulus, the process of lexical production finishes after this one word is produced, such as picture-naming tasks. However, this is not the case for some of the semantic fluency tasks, in which participants are instructed to produce as many words as possible in a constrained time frame (Tomé Cornejo, Reference Tomé Cornejo2015, p. 33), i.e., multiple response tasks. According to Gudmundson (Reference Gudmundson, Bardel and Sánchez2020, p. 75), the production of a word activates another unconscious process, the priming. That is, the produced word—the prime word—influences the word that will be generated next. For example, after the production of the word salt, the word pepper might be activated. In this case, salt is the prime word that activates the word pepper. This process could be interpreted as a semantic priming effect due to the semantic connection between these two words. Research has shown that semantic priming between concrete words (e.g. nurse-doctor, bread-butter) can be particularly strong (Jin, Reference Jin1990). Consequently, the basic concept behind this phenomenon of priming is that the activated concept does not activate only one lexical item but a network around the targeted item, where each of these lexical items is a node connected by edges that represent formal, semantic, or conceptual associations. The activation spreads along the paths of this network (Spreading Activation Model) and its strength decreases as it moves further from the initially activated word (Collins and Loftus, Reference Collins and Loftus1975, p. 411; Dell, Reference Dell1986), meaning that the higher the distance between two words, the less related they are.
Furthermore, in semantic fluency tasks, other cognitive mechanisms that come into play are semantic search strategies, clustering, and switching. Clustering refers to the production of sequences of words that are related, and switching refers to the ability to shift to another sequence or subset of words (Troyer, Reference Troyer2000; Troyer, Moscovitch, & Winocur, Reference Troyer, Moscovitch and Winocur1997). The analysis of these strategies has been used as a way to look into the structure of the lexicon (Roberts and Dorze, Reference Roberts and Dorze1997; Rosselli and Ardila, Reference Rosselli and Ardila2002; Borodkin, et al., Reference Borodkin, Kenett, Faust and Mashal2016).
Lexical access, selection and production in L2 in semantic fluency tasks
It seems reasonable to believe that lexical production in a semantic fluency task in an L2, especially if this is a foreign language, is somehow different from task completion in the L1, because of the already existing lexical information of another linguistic system in the mental lexicon. Likewise, we might also argue how vocabulary production in the L1 might differ in the case of monolingual learners and those who possess knowledge of other additional languages, even if they are tested in their dominant L1.
Researchers agree that in the bilingual or multilingual lexicon, there is one single conceptual system (Costa, Miozzo, and Caramazza, Reference Costa, Miozzo and Caramazza1999; De Bot, Reference De Bot1992; Kroll and Stewart, Reference Kroll and Stewart1994). Additionally, research on bilingual speakers has shown that priming effects can be observed between different languages (Chen and Ho, Reference Chen and Ho1986; De Groot and Nas, Reference De Groot and Nas1991; Duyck et al., Reference Duyck, Vanderelst, Desmet and Hartsuiker2008; Green, Reference Green1998; Schoonbaert et al., Reference Schoonbaert, Duyck, Brysbaert and Hatsuiker2009). These findings have been interpreted in favor of a shared conceptual system between the languages. Nevertheless, we have different lexical representations in every language for every concept (Costa, Miozzo, and Caramazza, Reference Costa, Miozzo and Caramazza1999; Kroll and Stewart, Reference Kroll and Stewart1994).
One of the most important models about the connections between the concepts and the lexical representations in the different languages and lexical access in bilinguals is the Revised Hierarchical Model proposed by Kroll and Stewart (Reference Kroll and Stewart1994). According to this model, these connections depend on the linguistic proficiency level in the foreign language displayed by the participants. The lexical representations of the L1 are strongly connected with their corresponding concepts. But in the early stages of FL acquisition, the connections between the word forms of the FL and the conceptual store, which is shared between the L1 and L2, are not so strong, because the individuals access them through the mediation of the L1 (cf. Jiang, Reference Jiang2019). Thus, the word forms of the FL are more strongly connected to their L1 translation than to their corresponding concepts. As proficiency in FL increases, the connection between the concepts and the FL lexical representations gains strength, creating direct links between them and eliminating the need for L1 mediation. Nevertheless, even if we talk about balanced bilinguals, the connections between the concepts and L1 word forms are stronger than the ones between the concepts and the L2 word forms. In any case, we understand that the concepts are somehow connected with the word forms of all the languages the individual speaks (Tomé Cornejo, Reference Tomé Cornejo2015, p. 42).
Even if there has been a debate concerning the nonselective (word forms of every language the individual knows are activated) versus selective (only the word forms of the language that is used in the experiment are activated) lexical access, researchers generally agree that the bilingual lexical access is characterized by non-selectivity (e.g. De Bot, Reference De Bot2004; De Groot et al., Reference De Groot, Delmaar and Lupker2000; Dijkstra and Van Heuven, Reference Dijkstra, Van Heuven, Grainger and Jacobs1998, Reference Dijkstra and Van Heuven2002), even in the case of low-proficient bilinguals (Meuter, Reference Meuter and Pavlenko2009). According to De Bot (Reference De Bot2004, p. 23), words from more than one language compete for activation both in lexical production and lexical perception. That means that in a semantic fluency task, the activated concept activates the word forms of every language the individual knows.
At this stage, the process of lexical selection takes place, which has been described in different ways. For instance, Costa, Miozzo, and Caramazza (Reference Costa, Miozzo and Caramazza1999) support the language-specific selection hypothesis, according to which word forms of the non-selected language are ignored and there is no competition among the word forms of the different languages. Accordingly, the individual has to select not only the corresponding word form but also the correct language (Costa, Reference Costa, Bhatia and Ritchie2008, p. 206). On the contrary, De Bot and other authors (De Bot, Reference De Bot1992; De Bot and Schreuder, Reference De Bot, Schreuder, Schreuder and Weltens1993; Green, Reference Green1998) defend the language nonspecific hypothesis, that is, during the lexical selection, there is competition among the word forms of every language the individual knows.
Additionally, as a result of the presence of several linguistic codes and the subsequent L1-mediation access, L2 lexical access is usually slower than L1 lexical processing. Other reasons might be the degree of use and practice, which is normally greater in the L1 than in L2, and which makes the L2 process less automatized (cf. Plat et al. Reference Plat, Lowie and de Bot2018; Rodriguez Fornells et al., Reference Rodríguez-Fornells, Rotte, Heinze, Nosselt and Munte2002; Wartenburger et al., Reference Wartenburger, Heekeren, Abutalebi, Cappa, Villringer and Perani2003; Abutalebi and Green, Reference Abutalebi and Green2007; Green and Abutalebi, Reference Green and Abutalebi2013).
Lexical selection is based on different factors. The overall level of activation of a language can determine the level of activation of the word forms (De Bot, Reference De Bot2004), but it is not the only determining factor. Word frequency, grammatical class (content or function word), language status (word form of L1 or FL), and language typology may affect the word’s level of activation (De Angelis, Reference De Angelis2005; Gudmundson, Reference Gudmundson, Bardel and Sánchez2020; Poulisse, Reference Poulisse1999). When word forms of the non-selected and of the selected language reach similar levels of activation, we may observe lexical transfer, language switches, or word coinages created by the combination of words of different languages (Jarvis, Reference Jarvis and Pavlenko2009).
Banking on the network metaphor, researchers have recently applied network theory or graph theory techniques and the use of automatic models to the identification of patterns in lexical organization (see e.g. Abbott et al., Reference Abbott, Austerweil and Griffiths2015; Borge-Holthoefer and Arenas, Reference Borge-Holthoefer and Arenas2010; Borodkin et al., Reference Borodkin, Kenett, Faust and Mashal2016; De Deyne et al., Reference De Deyne, Navarro, Perfors and Storms2016; Ferreira and Echeverria, Reference Ferreira and Echeverría2010; Nematzadeh et al. Reference Nematzadeh, Miscevic and Stevenson2016; Wilks and Meara Reference Wilks and Meara2002; see also Collins and Loftus’ Reference Collins and Loftus1975 original proposal, Spreading Activation Theory). The findings of this previous research pointed to an L2 lexicon which, in comparison to the L1 lexicon, is less organized, less connected globally, and therefore harder to navigate. Also, the L2 lexicon displays more unexpected associations than those found in the L1 lexical-semantic network. This results in more loose categories or subcategories, which are more difficult to identify or to group (e.g. Borodkin et al., Reference Borodkin, Kenett, Faust and Mashal2016).
It has been already proven that lexical access, selection, and production in L1 and FL in a semantic fluency task show some differences, even for advanced L2 speakers, at least concerning the number of words retrieved and the types of associations established (see e.g. Lemhöfer et al., Reference Lemhöfer, Dijkstra, Schriefers, Baayen, Grainger and Zwitserlood2008, p. 27). Therefore, in order to examine these differences that are related to the organization and the structure of the lexicon, and to get a better understanding of how lexical organization and access happen in the L1 and FL, a semantic fluency task is used. Two groups of participants were tested, first, native speakers of Greek who are learning Spanish as FL, who were tested in Greek and Spanish, and native Spanish speakers, tested in Spanish, who were asked to retrieve as many different lexical items as they could in the category of Fruits and Vegetables.
Experimental tasks for analyzing lexical production
There is a variety of experimental tasks used for analyzing the lexical production in psychological research. The tasks relevant to the present study include the semantic fluency task and the word association task. Verbal fluency tasks come in two types: semantic verbal fluency and phonological verbal fluency (Hernández Muñoz, Reference Hernández Muñoz2006; Fumagalli et al., Reference Fumagalli, Soriano, Shalóm, Barreyoro and Martínez-Cuitiño2017)Footnote 2. According to Borodkin et al. (Reference Borodkin, Kenett, Faust and Mashal2016), in the semantic fluency task, individuals are instructed to generate as many words as possible related to a given prompt or stimulus word belonging to a specific semantic category (for instance, animals and fruits). Performance can be oral or written (see e.g. Soriano et al., Reference Soriano, Fumagalli, Shalóm, Carden, Borovinsky, Manes and Martínez-Cuitiño2015) and it is time-constrained, typically lasting 1 or 2 minutes. Based on this definition and in line with other researchers (Hernández Muñoz, Reference Hernández Muñoz2006), the task used here is considered a semantic fluency task. This task allows the analysis of responses in terms of clustering and switching (Goñi et al. Reference Goñi, Arrondo, Sepulcre, Martincorena, Vélez de Mendizábal, Corominas-Murtra, Bejarano, Ardanza-Trevijano, Peraita, Wall and Villoslada2010; Voorspoels et al, Reference Voorspoels, Storms, Longenecker, Verheyen, Weinberger and Elvevåg2014). Such analysis sheds light on the process flow of activating, selecting, and producing a word.
The word association task is used in both L1 and FL lexical studies (Zareva, Reference Zareva2007) and has been employed to demonstrate the associative organization of lexical connections in the mental lexicon (Wright, Reference Wright2001; Peppard, Reference Peppard2007; Zareva, Reference Zareva2007; Séguin, Reference Séguin2015). The production can be discrete or continuous, and it may involve recalling a single term or a list of terms associated with each other (Hernández Muñoz, Reference Hernández Muñoz2006; Borodkin et al., Reference Borodkin, Kenett, Faust and Mashal2016). In this type of task, the responses are not only thematic, and they do not necessarily belong to the same semantic field as the stimulus, as observed in the semantic fluency task. Responses may also be classified as syntagmatic (take – bath), or phonological (pumpkin – napkin). Therefore, the task used in this study also exhibits characteristics of a word association task.
The present study intends to compare the lexical production in FL (Spanish) and in L1 (Greek) of the same individuals responding to the semantic fluency task in Greek L1 and Spanish FL. More responses are expected in L1 because of the stronger connections between concepts and L1 words, as well as because of the larger lexical repertoire in the L1. Additionally, we want to compare the lexical production in Spanish FL and in Spanish L1 of different individuals. For that reason, we asked a group of native speakers of Spanish to complete the same fluency task in Spanish. Here, again more responses are expected in Spanish L1 because of the stronger connections between concepts and L1 word forms and the larger vocabulary size of the former. Finally, comparison of L1 (Spanish) and L1 (Greek) of different individuals is also carried out to compare lexical access, connections among lexical items, and lexical retrieval in the two languages.
Thus, the main objective of the present empirical study reads as follows:
Examine the lexicon organization of native speakers of Greek who are learners of Spanish as an FL and compare this organization to that of i) native speakers of Spanish and ii) organization of the participants’ Greek L1 lexicon. Lexicon structure and organization are to be operationalized in terms of words produced, lexical availability, lexical profiles, lexical access, and relations among words.
Methodology
Participants
The sample included 105 participants, who were undergraduate and graduate students at Universidad de la Rioja in Spain and at the National and Kapodistrian University of Athens in Greece, aged between 20 and 45. Fifty-two of the participants were native speakers of Spanish living and studying in Spain who were asked to complete a semantic fluency task in Spanish responding to the category fruits and vegetables. Additionally, 53 Greek L1 participants living and studying in Greece answered the same semantic fluency task both in Greek L1 and in Spanish FL.
Accordingly, there are three main data sets that were used for analyses and 3 main contrasts were established and examined. Contrast #1 explored similarities and differences between Spanish L1 and Spanish FL data (at the three proficiency levels); Contrast #2 examined Spanish L1 and Greek L1 data; and finally, Contrast #3 dealt with differences between Greek L1 and Spanish FL data. Results report on these contrasts and are organized accordingly.
Instrument: semantic fluency task
Traditionally, as explained above, semantic fluency tasks have been used to access the mental lexicon and explore lexical and semantic organization (cf. Goñi et al., Reference Goñi, Arrondo, Sepulcre, Martincorena, Vélez de Mendizábal, Corominas-Murtra, Bejarano, Ardanza-Trevijano, Peraita, Wall and Villoslada2010; Reverberi et al., Reference Reverberi, Cherubini, Baldinelli and Luzzi2014). In semantic fluency tasks, also called category fluency or free listing, participants have to produce as many related or associated words as possible in a limited time as a response to a semantic category, typically animals, food and drink, or professions. The semantic category serves as a stimulus or prompts to activate the retrieval of words as they come to the participant’s mind. Here, participants had two minutes to complete a category-generation task of the semantic fluency type, where they had to respond to the prompt fruits and vegetables (cf. Borodkin et al., Reference Borodkin, Kenett, Faust and Mashal2016; Tomé Cornejo Reference Tomé Cornejo2015), i.e. participants had to generate as many different words as came to their mind during the two-minute slot. Native speakers of Spanish performed the task in Spanish only, whereas native speakers of Greek had to respond in Spanish FL first, and some days later, they repeated the task answering in Greek L1.
This type of fluency task has frequently been used to tap into lexical retrieval processes and thus inform about how words are stored in the mental lexicon.
Procedures and analyses
The semantic fluency task was a pen-and-paper task that was completed by participants on a voluntary basis after having been informed of the task and given their consent. Responses were typed into computer-readable files. The data were carefully edited, adopting the following criteria:
1. No repetitions per informant were allowed,
2. Spelling errors were corrected,
3. Multiple-word responses were hyphenated in order for them to be counted as a single word (e.g., fresh air).
Once the editing process was complete, semantic fluency data were processed and analyzed using semantic network graph theory (via dispogen and dispografo (Echeverría et al. Reference Echeverría, Vargas, Urzua and Ferreira2008) and Gephi (www.gephi.org) (Cherven Reference Cherven2015) and corpus linguistics applications and data-based analyses (via Antconc, Anthony Reference Anthony2022). These tools allow for automatic calculation of several measures such as total tokens, types, mean tokes, cohesion index of the response sample, bigrams (pairs of words that are associated most frequently), and availability index (see below). Gephi constructs graphs from association data that allow for calculation of different key statistical measures, such as, for instance, average degree, clustering coefficient, and diameter, just to name a few of the ones used here. These measures help us better picture and understand how the semantic network is built, accessed, and navigated. Descriptive metrics, as well as inferential data calculated via SPSS 26.0, wherever possible, are presented together with graph metrics.
Responses were analyzed using the lexical availability index put forward by Echeverría and colleagues (Echeverría et al. Reference Echeverría, Urzúa and Figueroa2005) and calculated automatically by the Dispogen, which takes into account not only frequency of appearance but also position of appearance. The exact formula it uses to calculate the availability of a specific word is the following (see Callealta Barroso and Gallego Reference Callealta Barroso and Gallego Gallego2016):

where
n = max position reached by the word in the sample.
i = position of the word at the specific test explored.
j = target word index.
e = Euler’s number (Napier constant) (2,718281828459045…)
fji = absolute frequency of word j in position i.
I1 = number of informants in the sample.
D(Pj) = target word’s j availability.
Results
In order to fulfill our objective, we submitted our data to several analyses. First, we wanted to know the most frequent responses produced and the overlap between the lists of responses produced, i.e. what were the coincidences in the responses among the group of responses with shared and non-shared word responses (convergence/ divergence). Table 1 presents the data for descriptive measures of lexical production.
Table 1. Lexical availability metrics (dispogen)
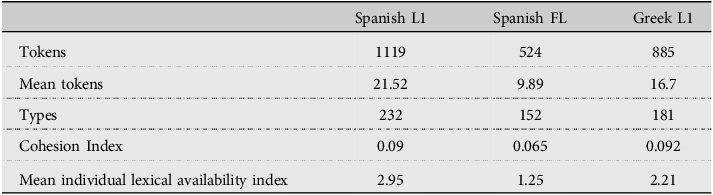
From the information in the table, it can be observed that Spanish L1 participants produce more tokens and types than Spanish FL learners (Greek participants responding in Spanish FL), and also than Greek L1 participants (responding in Greek). The cohesion index is quite similar between native responses and it is higher than in learners’ responses, which points to learners’ semantic fields being less homogeneous and compact. In other words, native respondents show higher degrees of coincidence in their responses when considered within group. This means that Spanish L1 and Greek L1 participants have similar conceptualizations and ideas of what the category fruits and vegetables entails. Greek learners of Spanish foreign language (SFL), on their part, show more variability and a more unstable, less compact picture of the semantic field. The measure of lexical cohesion corresponds to fields labeled as “disperse” or little compact, i.e. where there is room for differences in its conceptualization (cf. García Casero, Reference García Casero2013; Gomez Molina and Gomez Devis, Reference Gómez Molina and Gómez Devís2004).
Finally, the individual index of lexical availability reflects the overlap of each individual response against the total responses. Accordingly, the higher the individual lexical availability index, the better placed is an individual to engage in successful communication with the rest of the members of their group. Here, it can be observed that Spanish L1 participants display a higher individual lexical availability index, which probably points to a more efficient communication among members of this group than for the other two groups of participants (Greek L1 and Greek SFL).
For the fruits and vegetables category, the contrast #1 comparison of Spanish L1 Spanish FL output, i.e. total number of responses, was significant (between-participants), Mann WhitneyFootnote 3 = 257.5, p >.05, with more words by native speakers of Spanish (M= 21.52, SD = 6.9) than Greek learners of Spanish. The contrast # 2 comparison (between-participants) of Spanish L1 and Greek L1 output was also significant (Mann Whitney = 806.5, p >.05), with more words produced by Spanish L1 participants than by Greek L1 participants (M= 16.7, SD = 6.61). The contrast #3 comparison (within-participants) of Greek participants responding in Spanish FL and in Greek L1 was also significant, Mann Whitney = 590, p >.05.
Network parameters were calculated for each of the three cohorts (see Table 2). Metrics from a random graphFootnote 4 were also calculated and included in the comparison (see also Table 2).
Table 2. Graph metrics (Gephi)
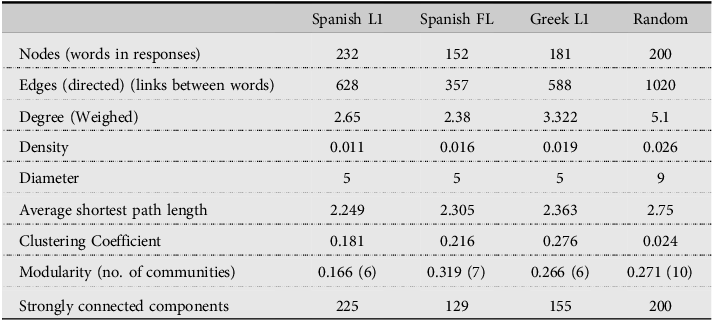
From the information presented in Table 2, the observation stands that Greek participants responding in their L1 display a highly tight network, with high degree, density, and high clustering coefficient. Native speakers of Spanish produce more responses but lower degree, i.e., fewer connections and lower values for density and clustering coefficient, i.e., nodes or words are less connected. However, because the size of the graphs (that is the total number of nodes [words]) was different, a decision was made to prune the data so that every node with only one connection, for instance, end-of-chain singleton nodes, was eliminated. Accordingly, the resulting graphs are more similar in size, and therefore easier to compare. Additionally, a random graph was also calculated.
From the observation of the pruned graph metrics (see Table 3), it stands out that the experimental graphs show very connected lexicons, more than in the random graph, with a high mean degree (number of connections among the nodes). Again graph density (the ratio of the number of connections with respect to the maximum possible connections or edges) shows very high-density values as compared with the randomly generated graph, which again indicates high connectivity. This is especially so in the Greek learners’ data probably pointing to fewer but repeated responses to the categories. Graph density is also related to total number of nodes (fewer nodes-higher density), so the fact that the learners’ graph is smaller might help explain this result. It does not explain, however, how experimental graphs with L1 data show much larger densities than the same-size random graph. Clustering coefficient values (the degree to which the nodes tend to establish connections among themselves) are larger than the random and average path length shorter. Average path length refers to the average distance between two nodes in the shortest path considering all pairs of nodes. This indicates that our experimental graphs do not display small-world-ness property as some natural language graphs do (cf. Borodkin et al., Reference Borodkin, Kenett, Faust and Mashal2016). As Table 3 indicates, clustering coefficient was larger and modularity (number of highly connected components in the network) smaller for Spanish FL than for native data. This is true across the two contrasts, in other words, regardless of whether the L1 was Spanish or Greek. The L2 network is more densely connected locally and less modular than the L1 network (cf. Borodkin et al., Reference Borodkin, Kenett, Faust and Mashal2016 for similar results). This means that the L2 network is less spread and less compartmentalized than the L1 networks (see Figures 1–3) and has denser connections within the modules, but loosely connected modules. This numerical parameter ties in with qualitative data for communities or clusters (see Table 4). Additionally, modularity parameters for the two native groups show negative values, which indicate less edges between nodes than would be expected by chance and point to unexpected links or associations between nodes.
Table 3. Graph metrics with graph pruned to nodes with 2 or more and a shape-similar random graph (Gephi)
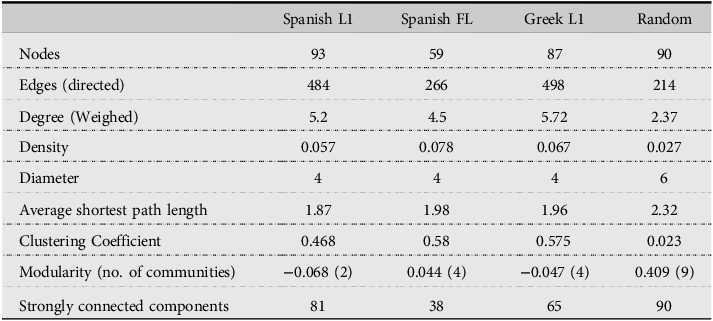
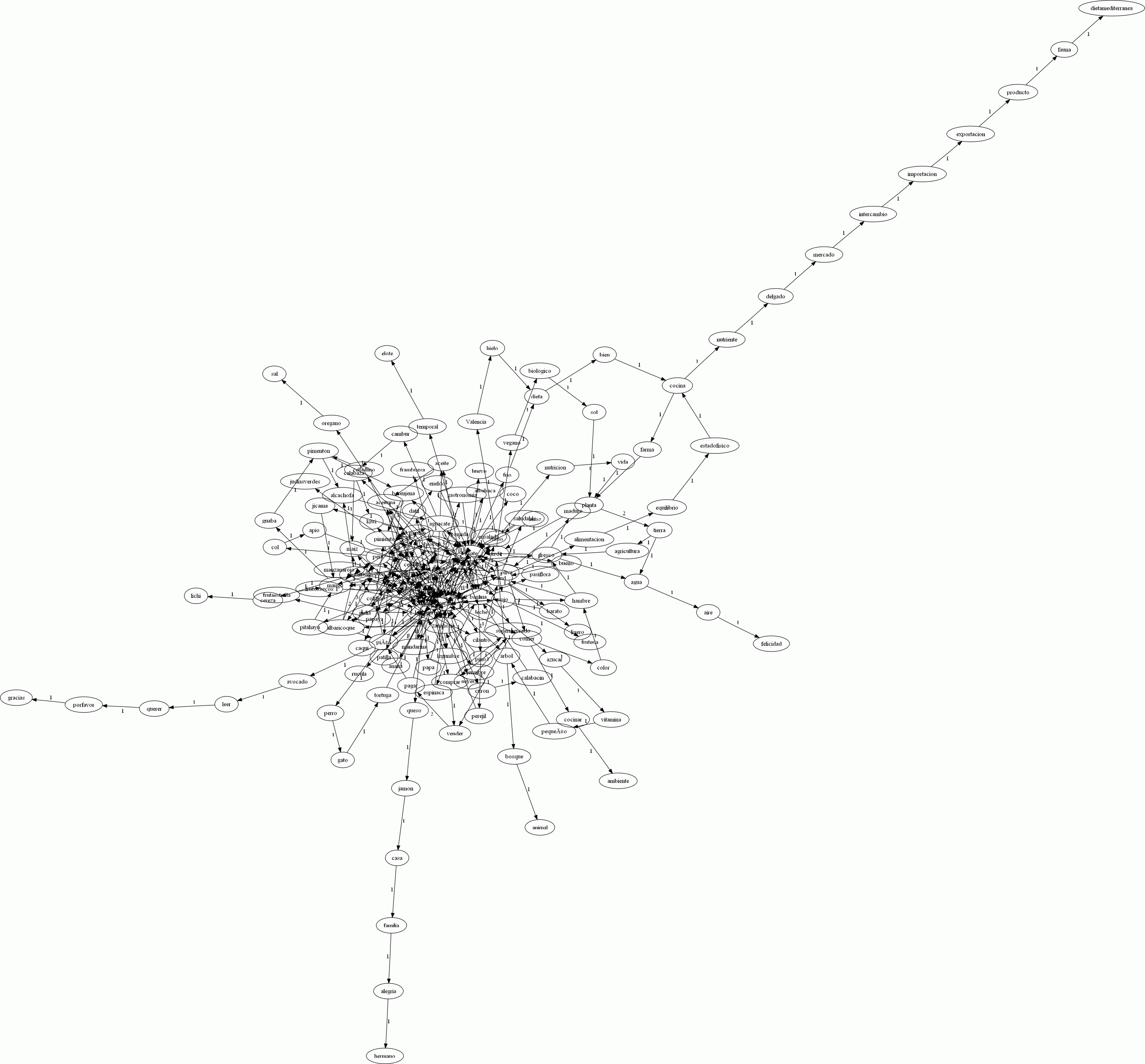
Figure 1. Greek responses in Spanish foreign language.
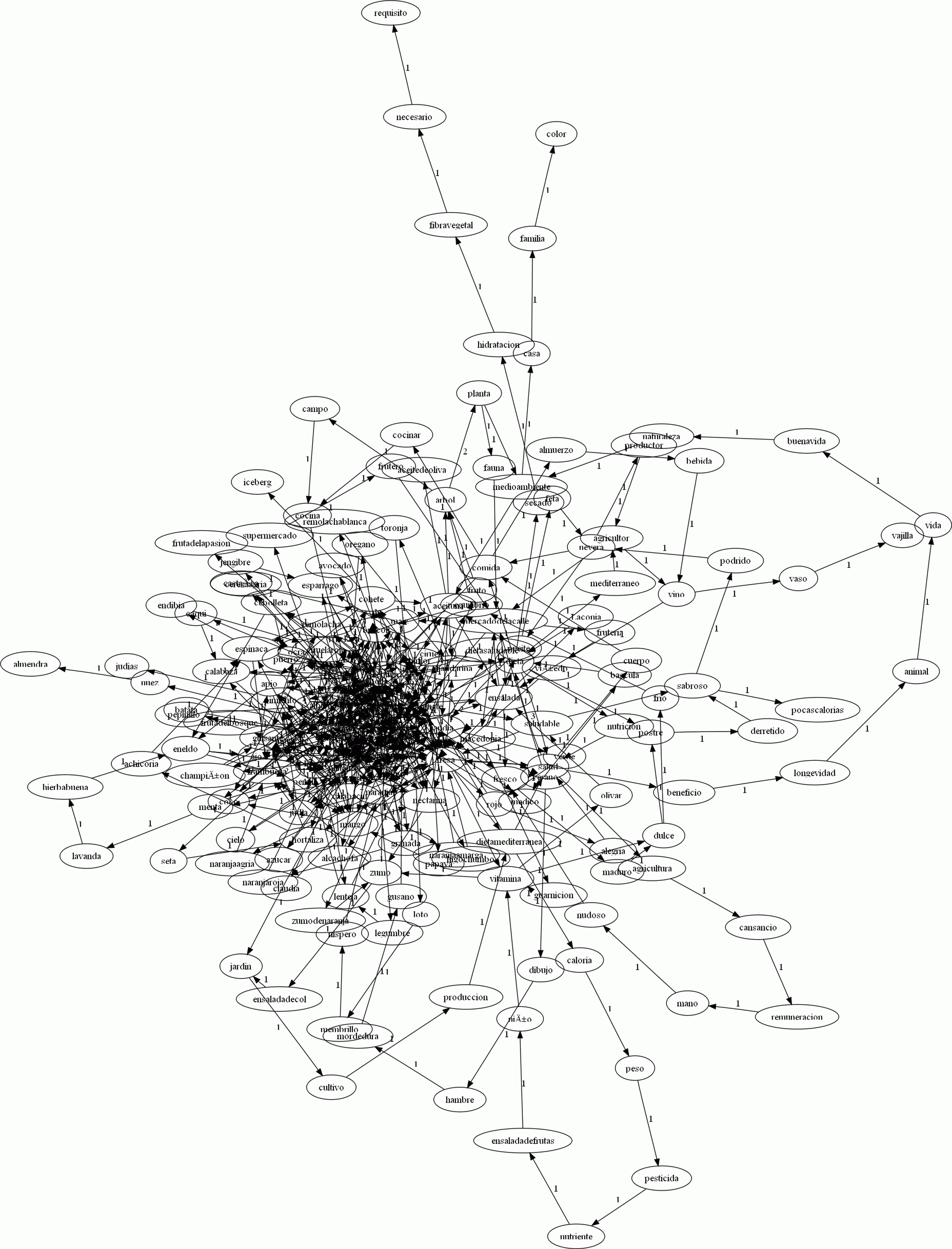
Figure 2. Greek responses in L1.
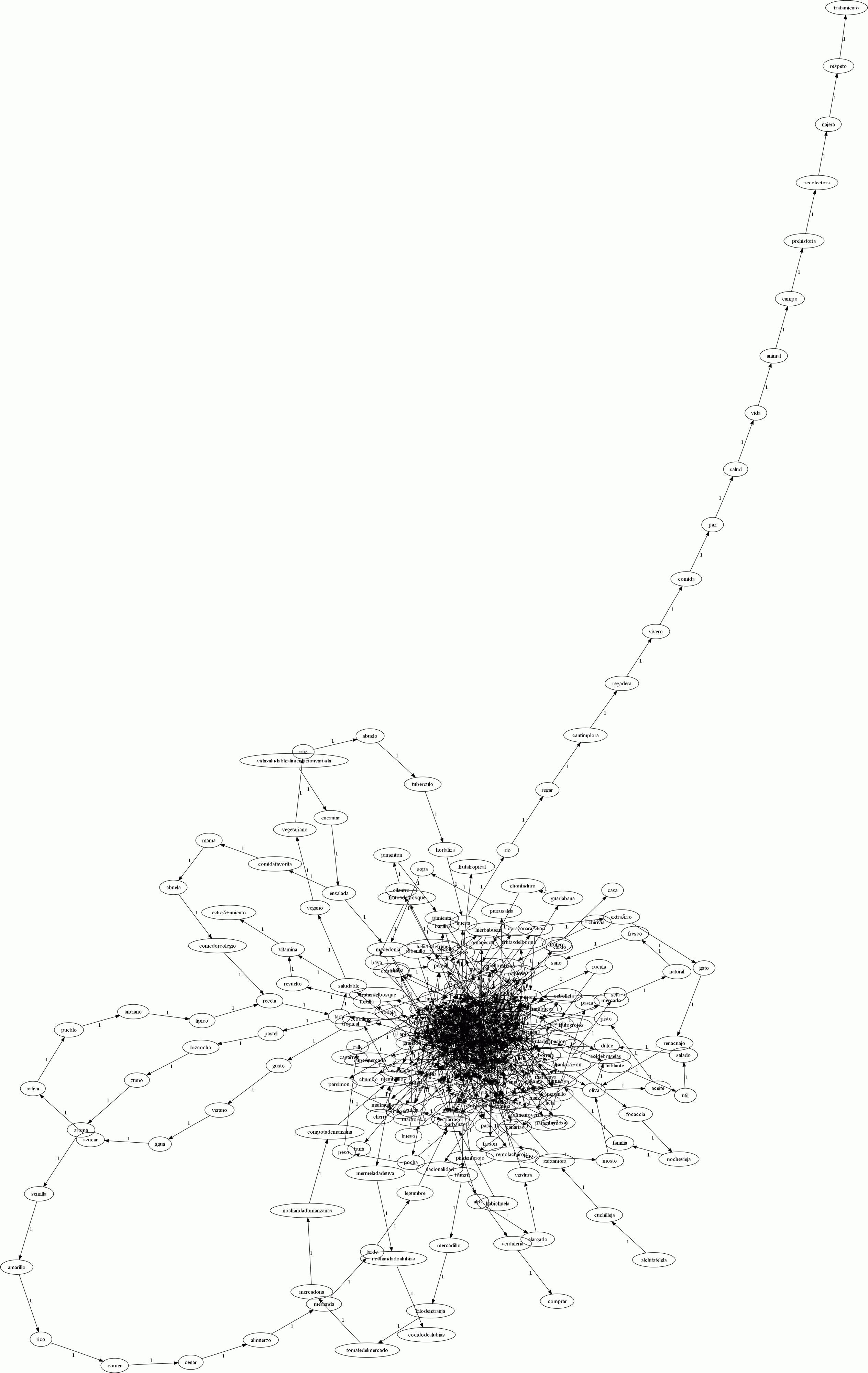
Figure 3. Spanish responses in L1.
Table 4. Communities (*anchor word: highest degree [most accessible])
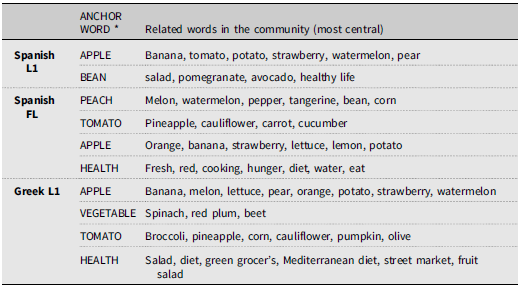
Nevertheless, Table 4 also shows that Greek data show very similar results disregarding whether participants respond in their L1 or in SFL.
Furthermore, communitiesFootnote 5 were also found and are presented in Table 4.
From the data of the anchorFootnote 6 words, we can observe that Greek learners display similar categorization structures and keywords in both their L1 and L2 and these are slightly different from native speakers of Spanish. This result leads us to believe that FL lexicon organization is influenced by, and might even calque, L1 lexicon organization. This translates to FL learners resorting to the same word-word associations in the FL as they do in the L1 and organizing their mental lexicon around the same anchor words, which, in turn, differ from Spanish L1 organization.
On a more qualitative level, the lists of responses produced were compared to look for shared lexical items and overlaps in the responses. Accordingly, for Contrast #1 (Contrast Spanish L1-Spanish FL), 72 shared words were identified, which accounts for 31 % overlap for L1 and 47.4 % overlap for SFL. This means that almost half of the words produced by SFL learners are also produced as responses by native speakers. This might point to a partial overlap of the conceptualization of the category fruits and vegetables. For Contrast #2 (Contrast Spanish L1-Greek L1), around 101 shared words were identified, which means 56% overlap between the responses in the participants’ L1. Finally, for contrast #3 (Contrast Spanish FL-Greek L1), an overlap of 60% with 90 shared words was identified. These results point to categorization in the native language being slightly different from categorization in the FL, and this is irrespective of the specific languages at stake. The overlap is bigger in learners’ responses in L1 and Spanish FL (same learners responding in the different languages) than between responses in Spanish L1 and Spanish FL (different learners responding in the same language).
This result concurs with the assumptions of the Revised Hierarchical Model (Kroll and Stewart, Reference Kroll and Stewart1994), which suggests that word forms in the foreign language are more connected to their translations in the native language than to their corresponding concepts. Thus, while performing in FL, participants do not activate concepts, they rather translate lexical forms from their L1. This is why the overlap is greater in learners’ responses in the native language and Spanish as a foreign language than responses between native Spanish and Spanish as a foreign language.
To delve deeper into this comparison, keywordsFootnote 7 were identified among the words that appeared unusually frequently in the lists for each contrast. Thus, we could identify green and health as keywords for the Spanish FL corpus, and diet as the keyword for the Greek L1 corpus. This means that these words are especially frequent in the said corpora with reference to the Spanish native data, which is used as the reference corpus.
Turning to identification of the most accessible or prototypical words used, the 10 most available or prototypical words were identified using a formula that combines frequency and position of appearance (see Table 5).
Table 5. Ten most available words
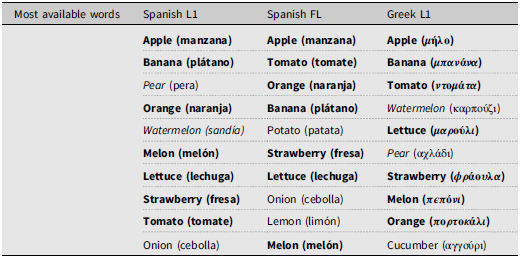
A total of 7 prototypical words (70% coincidence) are shared by all three groups indicating high levels of prototypicality for this field, i.e., the majority of the most accessible lexical items are shared among the groups. These words are apple, banana, orange, melon, lettuce, strawberry, and tomato. Native speakers of Spanish and Greek SFL learners also include onion reaching 80% overlap. Additionally, native speakers of Spanish and Greek also include among the most prototypical words: pear and watermelon arriving at a 90% overlap in their lists of most prototypical words.
Finally, Table 6 depicts the most frequent (non-weighed) bigrams trying to represent the most recurrent associations. Three of these bigrams stand out since they are shared by Spanish and Greek L1 participants: melon-watermelon, apple-pear, and tomato-lettuce. If the first one bases its association on similarity regarding the type of fruit they allude to (not formal, since the words are melón-sandía in Spanish and πεπόνι-καρπούζι in Greek) and experiential co-occurrence, the second one is mainly based on linguistic collocation in SpanishFootnote 8 and on experiential co-occurrence in Greek, and the third bigram alludes to two vegetables that appear together in experience in both languages/cultures. This clearly indicates that Spanish and Greek extralinguistic experiences and cultural experiences are similar to some extent, at least as food is considered. This is no surprise since both are Mediterranean countries with similar natural produce and dietary products/diets where fruits and vegetables play an important role.
Table 6. Most frequent bigrams
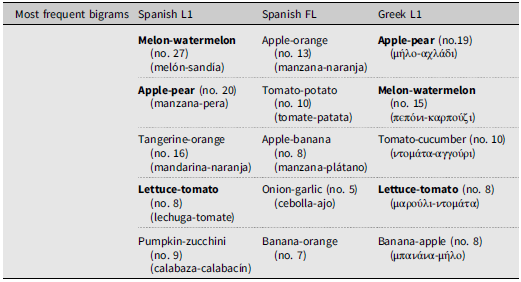
A specific mention is needed for the bigram apple-banana, which shows two fruits associated in experiential co-occurrence in Greek (μήλο-μπανάνα) and thus tend to be associated in the data, even when Greek participants write in SFL.
Discussion
Using network theory tools, we were able to demonstrate that the L2 lexical network was generally more densely connected at the local level, i.e. very highly connected central elements and sparsely connected outer nodes. In general, these results suggest that the L2 network is less well-organized than the L1 equivalents in both Contrasts (Spanish FL vs. Spanish L1 and vs. Greek L1) and that it is harder to navigate, i.e. to access target lexical items. In other words, L2 networks display some very connected central parts, but very loosely connected outer islands. Native networks, however, show higher organization with more connections among words and more repeated links, allowing for quicker transitions between lexical items. These networks are more complex and display a greater range of associations. We agree here with Durrant et al. (Reference Durrant, Siyanova-Chanturia, Kremmel and Sonbul2022, p. 95) that while L1 speakers are typically rather homogeneous in their characteristics, L2 learners are usually less so.
When comparing the native versus the L2 networks, two main observations stand out. First, learners produced fewer responses to the semantic fluency task in L2. This result is not surprising, since they have a smaller vocabulary size (see Meara, Reference Meara2009; Wong et al. Reference Wong, Goghari, Sanford, Lim, Clark, Metzak, Rossell, Menon and Woodward2020). For instance, Shao et al. (Reference Shao, Janse, Visser and Meyer2014) found that vocabulary size, a general measure of lexical knowledge, was positively correlated with performance on category-generation tasks. Previous research shows findings in this line, with L2 learners displaying overall lower verbal productivity compared to L1 participants when retrieving emotional and non-emotional vocabulary (Lam and Marquardt, Reference Lam and Marquardt2022).
Additionally, it appears that these processes are somewhat more intricate when learners are performing in the L2. According to the language-specific selection hypothesis (Costa, Miozzo, and Caramazza, Reference Costa, Miozzo and Caramazza1999), when word forms of the non-selected language are disregarded, individuals must not only select the corresponding word form for the concept but also choose the correct language (Costa, Reference Costa, Bhatia and Ritchie2008, p. 206). In this sense, lexical selection involves competition among the word forms of every language the individual knows (De Bot, Reference De Bot1992; De Bot and Schreuder, Reference De Bot, Schreuder, Schreuder and Weltens1993; Green, Reference Green1998). Consequently, it can be assumed that more time is required for lexical retrieval when several languages are at stake, ultimately resulting in the production of fewer words. Indeed, various studies have demonstrated that the process in L2 is slower and less automatized (Rodriguez Fornells et al., Reference Rodríguez-Fornells, Rotte, Heinze, Nosselt and Munte2002; Wartenburger et al., Reference Wartenburger, Heekeren, Abutalebi, Cappa, Villringer and Perani2003; Abutalebi and Green, Reference Abutalebi and Green2007; Green and Abutalebi, Reference Green and Abutalebi2013; Plat et al., Reference Plat, Lowie and de Bot2018). Our results concur with this.
Second, and more qualitatively, there is a big overlap in the prototypical words participants produce, being this overlap is larger within L1 responses with L2 learners showing more heterogeneity in their most prototypical responses (e.g. Lin et al, Reference Lin, Schwanenflugel and Wisenbaker1990; Nuñez Romero, Reference Núñez Romero and Villatoro2008; Schwanenflugel and Rey, Reference Schwanenflugel and Rey1986). Additionally, and in this line, three out of the 5 most frequent bigrams coincide in the L1 data, whereas Greek learners only produce one coincidental bigram when responding in L1 and L2. These results demonstrate that there are some structural differences between the L1 and L2 networks, mainly as regards their size and the strength of the connectivity.
Nevertheless, despite the differences mentioned before, results also suggest that the L1 and L2 lexical networks of participants also share some characteristics. For instance, there is a big overlap in the response types produced by participants responding in L1 and L2, an overlap bigger than when respondents use Spanish (L1 vs FL). This result might suggest an L2 categorization of fruits and vegetables, which is led and determined by L1 standards, associative behaviors, and scenarios. It also indicates that this categorization differs slightly in Greek compared to Spanish, regardless of whether Greek is considered L1 or L2. These results tie in with the belief that not only thematic associations and semantic similarity but, mainly, real-world experience lie on the basis of semantic knowledge and categorization, i.e. lexicon organization (cf. e.g. Shivabasappa et al., Reference Shivabasappa, Peña and Bedore2017). Furthermore, this finding together with the previous one regarding the high coincidence of prototypes in the three data subsets concurs with Malt’s et al. (Reference Malt, Sloman and Gennari2003) idea, they refer to it as same prototypes, varying boundaries, that speakers of all languages build their categories around very coincidental or universal prototypes, while borderline concepts further from the prototypes are more diverse and more highly influenced by linguistic and cultural factors, i.e. categories might share a conceptual core but differ in less associated elements.
Our findings provide evidence of the priming process. Thus, the word that is produced first (the prime word) influences and serves as a prime for the generation of the next (Gudmundson, Reference Gudmundson, Bardel and Sánchez2020, p. 75). This is why, in our data, certain bigrams have been consistently produced and repeated. This observation indicates that the first member of the bigram has triggered the production of the second member. Furthermore, the recurrence of the same bigrams (e.g., melon-watermelon, apple-pear) across different participants aligns with findings from other studies (e.g., Jin, Reference Jin1990), which suggest that semantic priming between specific words can be particularly robust.
In addition to this, the presence of communities provides evidence of the implementation of semantic search strategies, that is, clustering and switching. Communities have been analyzed (see also Roberts and Dorze, Reference Roberts and Dorze1997; Rosselli and Ardila, Reference Rosselli and Ardila2002; Borodkin, et al., Reference Borodkin, Kenett, Faust and Mashal2016) to identify the use of these strategies.
Our findings support the idea that the structural properties of the lexico-semantic network are also influenced by the specific linguistic and cultural background of the respondents (cf. Borodkin et al., Reference Borodkin, Kenett, Faust and Mashal2016; Wong et al., Reference Wong, Goghari, Sanford, Lim, Clark, Metzak, Rossell, Menon and Woodward2020). Thus, our data could identify the different communities attached to a central anchor word. Accordingly, in Spanish L1, two anchor words were identified: APPLE and BEAN. For APPLE, categorially similar neighboring words were identified, whereas for BEAN, a much more diverse neighborhood comes into stage. Greek learners in L1 and L2 conditions organize their lexicon for fruit and vegetables around four anchor words, which are very similar. Here, the Greek L1 clusters show very high connectivity and strong semantic links among the words in each community (see health, for instance, in Table 4). Greek participants responding in Spanish L2 show less strong connections and more separation among communities, but the similarities with the Greek L1 data are striking, especially if we bear in mind that learners are responding in Spanish, where more loose connections are found. This finding concurs with previous research, which concluded that participants with different native languages and cultures answered differently in a semantic fluency task of the type used here (Aitchison, Reference Aitchison, Arnaud and Béjoint1992; Carcedo González, Reference Carcedo González2000; Núñez Romero, Reference Núñez Romero and Villatoro2008). In a similar fashion, Pavlenko (Reference Pavlenko and Pavlenko2009) claimed that when L2 learning begins after early childhood, as is the case of our participants, the L1 lexical-semantic network is borrowed to represent the links between the L2 words (see Borodkin et al. Reference Borodkin, Kenett, Faust and Mashal2016) and as experience with the L2 progresses the web accommodates L2 linguistic and cultural specificities.
Finally, if we assume the optimal foraging pattern of lexico-semantic search to be true (Abbott et al., Reference Abbott, Austerweil and Griffiths2015; Hills et al., Reference Hills, Jones and Todd2012; Nematzadeh et al., Reference Nematzadeh, Miscevic and Stevenson2016), where learners exploit a semantic patch until the rate of finding a new word is less than the average rate of retrieval, when they then turn to explore a new semantic patch or cluster, then the observation strikes that Greek and Spanish offer different semantic organizations and that Greek SFL learners follow their native organization and search strategies even when producing in their FL (see Table 4). Accordingly, one can also assume that when learning a new language, one also needs to learn the structure of the lexicon in that language, i.e. how words are semantically clustered in the network (cf. Nematzadeh et al. Reference Nematzadeh, Miscevic and Stevenson2016) in order to achieve efficient navigating and access. This seems to be a crucial knowledge in lexical production in the foreign language.
Our findings support and extend previous research using the semantic fluency task and confirm that words are retrieved according to concepts, as suggested in Levelt’s model. Accordingly, participants detect associations between related concepts in their effort to fit the retrieved words in the specific semantic field or group, which supports the idea that several lexical items might be activated per each concept (Kroll and Stewart, Reference Kroll and Stewart1994). Most of those links refer to the L1 lexical and conceptual system, which is most accessible, and from which learners borrow to complete the task in the L2. In this sense, our results concur with Matusevich et al. (Reference Matusevych, Dehaghi and Stevenson2018) who found that learners produce different associations in their FL than native respondents. They brandish three main mechanisms responsible for those differences. First, learners most possibly rely on native associations via translations, as we could attest in our data. Second, they can resort to collocational patterns, a mechanism that is not apparent in this specific semantic field, and finally, they might rely on formal similarity between words. In Matusevich et al. (Reference Matusevych, Dehaghi and Stevenson2018), translation proves to be the main mechanism used by learners when completing a semantic fluency task in the FL. Our present results concur with this. Jiang (Reference Jiang2019) argues in the same line and also found that L1 responses are heavily culture-loaded and are also more numerous and their range is larger than that of the L2. Our results, again, reflect these findings.
Conclusion
The present study aimed at comparing the structure and lexical organization of L1 and L2 mental lexicons. For this purpose, we analyzed both in a quantitative and a qualitative way the responses produced by L1 Spanish participants and by Greek participants responding both in their mother tongue and in SFL during a semantic fluency task. To summarize, results point to a retrieval mechanism based on L1-mediated access for SFL learners and slightly different structures of the mental lexicon. Even in very advanced learners, lexical organization and word retrieval in the FL resemble their own L1 organization.
We believe that the use of Graph-based models or semantic networks is a very useful tool in the research on such complicated processes like lexical access, selection, and production in L1 and L2 and in studies focused on the structure of the lexical network and semantic memory in L1 and L2. This approach can complete a quantitative analysis of the data, which is normally made in these types of studies by offering some kind of visual representation of the internal structure of the semantic memory.
This paper offers a novel approach to describing the mental lexicon and categorization in the native and foreign languages by means of using complex network tools to analyze the data (banking on the network metaphor for lexicon representation) and constructing graphs from long edges using bigrams or pairs of words. The exploration of FL lexicon organization itself is not a widely explored issue either. However, it is of crucial importance to understand how learners organize the words of the new language in their minds. We also want to understand what learners do when they learn a new word: where it fits into that network, which words it establishes associations with, and how strong those links are. Do they follow the same criteria when incorporating new words into the FL lexicon as they do in their L1? The methodology used in the paper, not alien to the field, but not frequently used either, has helped to identify some interesting lexical organization behaviors in FL learners by constructing graphs using bigrams coming from long response chains in an aggregated fashion and without discarding any responses, even infrequent ones. These tools and novel approaches allow us to conclude that there seems to be a lexical item retrieval mechanism based on L1-mediated access. Likewise, we could identify slightly different structures of the mental lexicon in L1 and in FL learners, with the structure of the native language of the participants enforcing itself even when participants respond in the FL. This probably points to a FL lexicon organized according to the laws of the participants’ native lexicon. These findings represent a novel contribution to the field.
This work follows the line of research that tries to utilize lexical networks, semantic fluency, and category-generation tasks in order to compare the structural characteristics of the L1 and L2 mental lexicons. The results of this study are not exhaustive and further research needs to be conducted. Future works could use semantic categories with different characteristics, and learners of FL of different ages and of more distant cultures.
Ackowledgments
This study was partially funded by research project PID2022-137337NB-C21, funded by MICIU/AEI/10.13039/501100011033/ and ERDF/UE.