INTRODUCTION
Salaries purposefully vary across employees. The justification for giving larger salaries to better performers is to reinforce superior performance and facilitate productivity (Leventhal Reference Leventhal, Berkowitz and Walster1976, 96). Furthermore, poor performers—who receive lower salaries—have an incentive to either improve their performance or leave the system. Competition for salaries that are based on output encourages greater effort (Lazear and Rosen Reference Lazear and Rosen1981). Our question is: does variation in salary indeed reflect measurable differences in output? If yes, which performance indicators are the most strongly linked to larger salaries? If no, what factors other than productivity determine variation in salary?
Data from the National Center for Education Statistics (NCES) for full-time faculty on 9-month contracts for the 2013–2014 academic year reveal that the average salary of a full professor was $109,905; $78,593 for an associate professor; and $66,025 for an assistant professor. Salary data for the political science discipline showed similar patterns ($100,451-full; $81,820-associate; $71,834-assistant). Significant deviation from these means occurs, both at the individual level and among categories of faculty. For example, on average, women of all academic ranks make less than men. Footnote 1 Also, on average, those employed in public institutions make less than those employed in private institutions. Our analyses take into consideration salary differences based on gender, race, and institution-type, in addition to many other control variables, in order to test hypotheses about expected predictors of variation in salaries among political science faculty.
However, this study moves beyond the standard demographic and structural-based explanations for differences in pay. We want to know whether, as some of the literature predicts, higher salaries are consistently associated with greater research output, undermined by heavier teaching loads, and relatively unaffected by service. The reason these determinates of salary are important is that if salary premiums are given exclusively or primarily for publications, then the incentives to faculty are to spend no more than a minimum amount of time on teaching. The price of such academic pay incentive structures is paid for by the students, who may then learn less because their professors are concentrating on their research.
We are also interested in the role that selection into a graduate school program plays in a faculty member’s earning potential. If receiving one’s doctoral degree from a prestigious institution is critically important not only for where one is hired, but for how much one is paid, then productivity within one’s current position becomes secondary to (or is dependent upon) a decision made long before our political scientists became faculty members. It therefore behooves us to advise our undergraduates, quite carefully, on where to do their doctoral studies.
Last, but not least, we are interested in the role that salary negotiations play in salary outcomes. Some research indicates that women negotiate less than men, in part because of the belief that women cannot sincerely argue that they will move if their demands are not met. Booth, Francesconi, and Frank (Reference Booth, Francesconi and Frank2003) develop a “loyal servants” model of outside offers and gender pay differentials, according to which employers can exploit the likelihood that family commitments typically limit female mobility more than male mobility. If indeed differential patterns of negotiation are determining salaries, this may have insidious consequences, with female faculty unlikely to be able to reduce through negotiation the gap between their salaries and those of their male counterparts.
This study is based on the second wave of a panel survey conducted of all recipients of a political science doctoral degree in the United States between 2003 and 2008. Footnote 2 The analyses below are confined to those PhD-recipients who are currently employed as full-time faculty members. In addition to reporting their salaries, respondents reported several characteristics of their graduate school experience. Respondents also provided detailed information about their current employment, including work hours, publication history and teaching responsibilities. Footnote 3 Using data generated from this web survey conducted in 2014 and sponsored by the American Political Science Association, we construct several academic salary models with standard predictors as identified in the literature. We use OLS and WLS regression, plus a selection model to control for factors that place individuals on different trajectories stemming from their graduate school experience. Some noteworthy findings are that negotiation positively affects salary for men, but not for women, and that publications increase salaries among women but not men. For all faculty, teaching more undergraduate courses has a significantly negative effect on salary.
THEORY, LITERATURE, AND PREVIOUS FINDINGS
Following the lead of the literature, our starting analytical framework is that of human capital theory. Human capital is defined as “productive wealth embodied in labor, skills and knowledge” (OECD 2001; Tan Reference Tan2014, 412) and it refers to any stock of knowledge or the innate/acquired characteristics a person has that contribute to his or her economic productivity (Garibaldi Reference Garibaldi2006). The line of causality is as follows: an individual acquires knowledge and skills (human capital) through education and training; the knowledge and skill in turn increase productivity; which in turn brings higher wages (Marginson Reference Marginson1993). Footnote 4
It follows that the reputation of the institution from which the faculty member received her doctoral degree would affect salary. The assumption is that the ranking of the department is a proxy for the quality of training received as a graduate student. Graduation from a highly ranked university may also signal potential employers that the graduate possesses the essential human capital needed for success in future academic pursuits. Or the “value” of the education may simply be higher given the status accorded to the highly ranked degree-granting institution. Research confirms that the ranking of the PhD-granting department is correlated with salary (Ehrenberg, Pieper, and Willis Reference Ehrenberg, Pieper and Willis1998; Formby and Hoover Reference Formby and Hoover2002).
Graduation from a highly ranked university may also signal potential employers that the graduate possesses the essential human capital needed for success in future academic pursuits.
“Years of work experience” is a basic variable in human capital-based salary models, as work experience is understood as a proxy for on-the-job training (Melguizo and Strober Reference Melguizo and Strober2007; Perna Reference Perna2001). Related to years of experience is faculty rank: assistant, associate, or full professor. Koch and Chizmar (Reference Koch and Chizmar1973) report that rank and number of years the professor has possessed the PhD degree are among the most important determinants of the absolute salary structure. Academic salary models frequently use faculty rank as an explanatory variable (Moore Reference Moore1993).
Labor mobility is also a core component of human capital theory. According to De Riemer, Quarles, and Temple (Reference De Riemer, Quarles and Temple1982, 141), “Mobile faculty, by tradition mostly males, are presumably paid more because of salary increases associated with relocation” (see also Astin and Bayer Reference Astin and Bayer1972, 117). Soliciting employment offers from another university is associated with higher salaries (Kasten Reference Kasten1984), and women can be disadvantaged if they are less geographically mobile than men, or if they are less comfortable in seeking such outside offers (Blackaby, Booth, and Frank Reference Blackaby, Booth and Frank2005). In addition, women may be penalized for bargaining because such tactics violate supervisors’ expectations about appropriate female behavior (Dreher, Dougherty and Whitely Reference Dreher, Dougherty and Whitely1989). Gerhart and Rynes (Reference Gerhart and Rynes1991, 259–260) report that “bargaining led to higher initial salaries for both men and women” but payoffs were larger for negotiating men than negotiating women. Footnote 5
Human capital theory primarily explains wage variations using the factors outlined above (Becker Reference Becker1964). Criticisms of this somewhat restricted approach led to supplementing human capital with structural theory. Structural models expand the list of predictor variables with the attributes of the colleges and universities within which faculty work (Pfeffer and Langton Reference Pfeffer and Langton1988, 603; Youn Reference Youn, Breneman and Youn1988). Melguizo and Strober (Reference Melguizo and Strober2007) note that in the competitive bidding process for highly sought-after faculty, PhD-granting departments have an advantage over departments that offer only bachelor degrees. Differential customs or norms concerning the extent to which unequal rewards are desirable may also be long embedded in specific institutions. Private institutions may have the endowments that allow them to pay more than public institutions. Unsurprisingly, the type of academic institution where the faculty member is employed is a key determinant of faculty salaries (Barbezat and Hughes Reference Barbezat and Hughes2005, 627).
Structural models posit that salary differences between men and women occur in part because women are segregated into certain types of institutions (Smart Reference Smart1991). Toutkoushian and Conley (Reference Toutkoushian and Conley2005, 20) find that gender-based pay differentials were more prominent in certain kinds of academic institutions compared with others. Yet, Astin and Bayer (Reference Astin and Bayer1972, 141) report, “even women employed by large and prestigious institutions made less money than men at the same institutions.”
Having reviewed human capital and structural theories, we turn to faculty productivity. We consider research, teaching, and service as indicators of productivity. Studies show that research productivity is a primary factor in determining salary (Fairweather Reference Fairweather1993; Webster Reference Webster1995). In looking at publications, Barbezat and Hughes (Reference Barbezat and Hughes2005) find that both refereed journal articles and chapters/reviews achieve statistical significance, but that texts/monographs do not. Funded research grants significantly improve salary independently of the positive effect associated with publications (Melguizo and Strober Reference Melguizo and Strober2007; Perna Reference Perna2001).
Although research productivity is regularly linked to higher salaries, the effects of teaching and service on salary are not as well-determined. Blackaby, Booth, and Frank (Reference Blackaby, Booth and Frank2005, F90) find that “professorial pay depends on productivity (grants, publications and teaching).” Yet, McLaughlin, Smart, and Montgomery (Reference McLaughlin, Smart and Montgomery1978, 81) determine that “instructional activities apparently have minimal impact on salary.” Similarly, Melguizo and Strober (Reference Melguizo and Strober2007) assert that time spent on teaching has no effect on salary. Perna (Reference Perna2001) reports that more time spent on research rather than teaching correlates with higher salaries. She also evaluates committee work, which is associated with lower salaries. Koch and Chizmar (Reference Koch and Chizmar1973) find that evaluation of service by peers affects absolute salary. Mamiseishvili and Rosser (Reference Mamiseishvili and Rosser2010, 92) point out that “service work… is an important aspect of faculty socialization within the academy and is viewed as a commitment to the institution and the profession.”
We design statistical models that use human capital, structural, and productivity measures to predict salary. Footnote 6 These encompass the full set of factors that “should” affect salary, and when we consider these as controls, we are able to assess whether male and female faculty with comparable qualifications receive comparable pay. Thus, our models allow us to assess the persistent finding of an unexplained (by appropriate controls) wage gap between male and female faculty (Perna Reference Perna2001, 295; Toutkoushian Reference Toutkoushian1998; Toutkoushian and Conley Reference Toutkoushian and Conley2005, for a review). Differences have been quantified: decades ago, being a female costs a faculty member an average of 69 dollars per month” (Koch and Chizmar Reference Koch and Chizmar1973, 32). Footnote 7 Our analyses also allow us to investigate interactions between various predictors of salary and gender: Lewis, Wanner, and Gregorio (Reference Lewis, Wanner and Gregorio1979), for example, argue that publication records are important for the salary of females, but not for males. In contrast, Ward (Reference Ward2001) finds a premium being paid to male academics for publications, with an insignificant coefficient associated with publications of female academics.
We design statistical models that use human capital, structural, and productivity measures to predict salary. These encompass the full set of factors that “should” affect salary, and when we consider these as controls, we are able to assess whether male and female faculty with comparable qualifications receive comparable pay.
HYPOTHESES AND VARIABLES
Dependent Variable: Salary
The dependent variable used in the analyses is based on self-reported salaries by each respondent at the time the survey was administered. Footnote 8 Our measure of annual salary for full-time faculty ranges from $20,000 to $243,000. Footnote 9 For analytic convenience, we divide this raw annual salary by $1,000. In appendix A, we compare average salary figures based on the self-reports in our APSA survey data with average salary data from National Center for Education Statistics (NCES). The APSA survey was restricted to those with political science doctorate degrees, whereas NCES data is for faculty from all academic fields. Nonetheless, the comparison confirms the validity of the self-reported salaries from the APSA web survey. Footnote 10
The independent variables used in the models are grouped into the following categories: demographics, human capital, structural characteristics, individual behavior, and productivity.
Demographics
Our primary hypothesis, with regard to demographics, is that female faculty members are, on average, paid less than male faculty members. Thus, we include in our models Female, which is a dichotomous self-reported measure of gender. Footnote 11 From appendix C, we see that our sample (composed primarily of assistant and associate professors, given our focus on the 2003–2008 PhD-recipient cohort) is 39% female. Again, these statistics confirm that our sample represents well the full population of political science faculty, among whom females comprise 39% of assistant professors and 30% of associate professors. Footnote 12
We expected that those with children might be paid less than those with no children (primarily due to lower labor mobility), so we include the variable Children, a dichotomous measure indicating whether the respondent or the respondent’s spouse/partner has any children. Footnote 13 We also include the variable Caucasian, which takes a value of 1 if the respondent reports this as his or her race or ethnic origin, and a value of 0 (zero) for all other responses. Footnote 14
Human Capital
Given the emphasis human capital theory places on the importance of investments in education, we include a measure based on the National Research Council (NRC) rank of the respondent’s PhD-granting graduate program. The variable NRC Top 20 is a dichotomous measure of whether the respondent received their PhD degree from a university ranked in the top-20, according to the NRC in 2014. Our hypothesis is that investment in education (i.e., a higher ranked department where the respondent received his or her PhD) has a greater effect on salary for women than men. In addition, graduate programs located in different parts of the country may be subject to regional effects in labor markets, so we introduced controls for the geographic location of the PhD-granting institution. The models reported here include a control for Northeast/West, which takes a value of 1 if a respondent’s PhD program was located in the Northeast or West region of the United States, and 0 otherwise. Footnote 15
In a Heckman selection model reported below, we incorporate an additional human capital variable associated with the graduate school experience: employment as a research assistant during graduate school (Graduate Research Assistant). A reasonable human capital measure of on-the-job training is whether the individual held an assistantship during graduate school (Perna Reference Perna2001). Relatedly, we also include years of experience in this human capital category. Salary is expected to grow over the years with continued investment in (on-the-job) training. Footnote 16 Similar to Melguizo and Strober (Reference Melguizo and Strober2007), we use number of years since receiving the PhD as a measure of work experience. Years Since Degree is only used in models without academic rank, as this variable is correlated with academic rank.
To account for variations in salary due to promotions and current academic position, dummy variables, Assistant, Associate, and Full Professor, are created to indicate the reported academic rank for each respondent. The excluded category (when all three of these are included in an equation) is composed of visiting professors, instructors, lecturers, postdocs, fellows, and adjuncts.
Structural Characteristics
Two variables are included to account for the respondent’s current institution of employment. First, we control for being employed in a private institution (rather than public) (as do Formby and Hoover Reference Formby and Hoover2002; Melguizo and Strober Reference Melguizo and Strober2007; and Perna Reference Perna2001). The variable Private Institution takes a value of 1 if the respondent’s current employment is at a private college or university, and 0 otherwise. We also include a dummy variable PhD Granting, which takes a value of 1 if the respondent’s current employing department grants a doctoral degree, and 0 otherwise. Both of these variables are meant to provide measures of institutional resources or serve as indicators of different norm structures.
Behavior
Our behavior category allows us to account for work commitment and negotiation efforts. Work Hours measures the self-reported number of hours an individual works per week. Salary Negotiation takes a value of 1 if the respondent reported negotiating their salary when they were hired, and 0 otherwise. We hypothesize that negotiation leads to higher salaries for both men and women, but more significantly among men.
Productivity
Research productivity measures include the following: Journal Articles—the number of peer-reviewed journal articles published, Books—the number of books published, Books Edited—the number of edited books published, Book Chapters—the number of book chapters published, and External Grants—the number of external grants. All of these measures are for a respondent’s entire career. Footnote 17 Among these different measures of research productivity, we expect that articles produced are more likely to contribute to higher salaries than are books. We unfortunately do not have measures of quality, only quantity, but we can surmise that articles are likely to be subject to the most rigorous referee process. Among all the research productivity measures, articles have the greatest likelihood of contributing to the reputation of the faculty member and the institution, and thusly, we expect, will be most strongly related to salary.
Teaching and service are captured with Undergraduate Courses and Total Service Index. Undergraduate Courses is a basic count of the number of undergraduate courses taught by the respondent over the past year. Service Index is an additive measure of the amount of service the respondent committed to their university and to the profession over the past year. It counts the frequency of committee membership and chairing for the respondent’s department, another department, the university, or a professional organization, with chairing a committee being weighted twice as much as being a member of a committee. With regard to overall productivity, we hypothesize that better publication records are associated with better salaries (and that the relationship is stronger for females than males), that heavier teaching loads are associated with lower salaries, and that service is unrelated to salary.
Empirical Results
As described above, the dependent variable in the following analyses is the respondent’s self-reported salary. The first four columns of table 1 report the results of testing models using OLS regression. Model 1 represents our baseline model, which includes demographics, human capital, and structural variables, plus negotiation, teaching, and service. The baseline model highlights the important role that these factors have in determining faculty salary. The negative coefficient on females reveals that, given the other variables in the baseline model, women are paid significantly less than men (by about $4,000). Model 1 also reveals that Caucasians are paid more on average than those who self-identify in one of the other racial/ethnic categories. Having a PhD granted by a top-ranked political science program is significantly related to higher salary, as is attending a graduate school in the Northeast or the West. Characteristics of the department/institution where one is currently employed are also important: PhD- granting departments and private institutions are associated with higher salaries. Negotiation improves salary, while the number of undergraduate courses taught has a large, negative effect on salary. More service is associated with higher salaries. Footnote 18
Table 1 OLS & WLS Salary Regressions
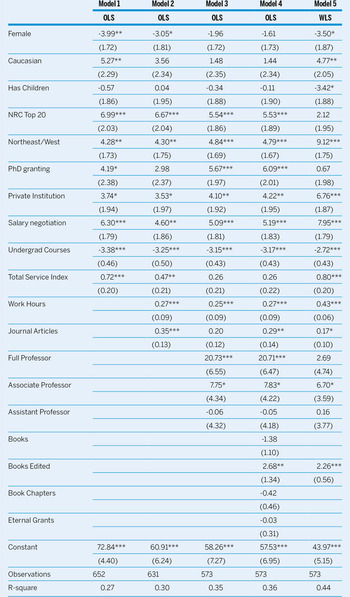
Unstandardized estimated coefficients are presented with standard errors in parentheses. *p < 0.10, **p < 0.05, ***p < 0.01.
Model 2 in table 1 adds the information on the number of hours worked per week and the number of journal publications. The estimated coefficients reveal that more journal publications and extra work hours are associated with higher salaries. Model 3 appends academic rank dummy variables to model 2. These rank variables indicate that full and associate professors are paid more than those in our reference category. What is noteworthy from our perspective is which of the other variables in model 3 hold their significance when controls for academic rank are included in the equation. All demographics, the service index, and number of journal publications lose their significant effects on salary when academic rank is included, but the human capital, structural, negotiation and teaching measures all remain significant. Model 4 expands on the previous model by adding other measures of productivity including number of books published, number of books edited, number of book chapters published, and the number of external grants applied for over the respondent’s entire career. This model is reported to show that the types of research productivity most often associated with higher salaries are journal articles and edited books. Footnote 19 Note that the addition of different measures of productivity provides only a very small increase in the amount of variance in salary explained (R2 increases from .35 to .36 from model 3 to model 4). Thus, in subsequent models, we carry forward just two key measures of research productivity—journal articles and edited books.
We were concerned about the possibility of heteroscedasticity in these models, whereby the variance of the errors is not constant across ranges of the independent variables. This could occur, for example, if faculty having relatively few publications experience a greater variance in their salaries than the most published faculty in the sample. We first tested the residuals in the OLS models to see if the assumption of constant variance for the errors holds. The results of Breusch-Pagan tests confirm that we violate the assumption of homoscedastic errors for each of the OLS models in table 1. Footnote 20 In the presence of this problem, OLS coefficient estimates are still unbiased, but the standard errors are biased, rendering hypothesis testing more dubious. After confirming the presence of heteroscedasticity, we checked the residual plots against each explanatory variable in the baseline model. Three variables in particular stood out as culprit variables causing non-constant error variance: work hours, the number of journal publications, and the number of edited books. For the edited books and journal publications variables, we see larger variance in salaries at lower levels of publications; for the Work Hours variable, we see a larger variance in salaries in the middle range of the variable. We deal with this issue by relying on the method of weighted least squares (WLS). We present only one example of this WLS procedure (others are available from the authors). Column 5 of table 1 presents a similar specification as examined in model 3 of table 1, using the weighted least squares method to account for heteroskedasticity in the data. Footnote 21 Model 5 in table 1 weights the variable Work Hours. Once we control for heteroscedasticity, we see that the negative salary gap for females ($3,500) and the positive salary gap for Caucasians ($4,770) are confirmed. What emerges clearly in this model that was not apparent in other models is the negative effect having children has on salary ($3,420).
Using the single equation form, as presented in table 1, requires the assumption that all predictor variables have an independent impact on salary. Given the previous literature, we have reason to believe that this assumption is not met for men and women. Table 2 presents split-sample models by self-reported gender in order to discern differing effects of important variables in predicting salary for male and female faculty members. In the first model in table 2 (model 1), we control for academic rank. In the second model (2), we control for the number of years since receipt of the doctoral degree. A number of interesting results emerge from this analysis. Looking first at model 1, and with regard to demographics, we see a $4,870 positive salary boost for Caucasians, but this effect is significant only among males. Secondly, males who negotiated their salary reported higher salaries ($5,880 in model 1) than male respondents who did not negotiate. Among females, no such benefit from negotiation exists, which is consistent with literature suggesting that women do not get the same positive outcomes from negotiations as men (Babcock and Laschever Reference Babcock and Laschever2003). In addition, increasing the number of work hours among female academics improves salary (around $420 per hour in model 1), but no such relationship is found among males. Also noteworthy is that research productivity, as measured by journal articles, is unrelated to salary among male faculty (given other controls in the model). Among women, increasing the number of journal articles is related to higher salaries ($580 higher salary per article in model 1). From model 2 in table 2, we see the effect of “years since degree”: given all the other variables in the equations, more work experience significantly boosts the salary of female faculty, but not male faculty. For men only, number of books edited emerges as a significant predictor of salary in model 2 of table 2.
Table 2 OLS Salary Regressions Split by Gender
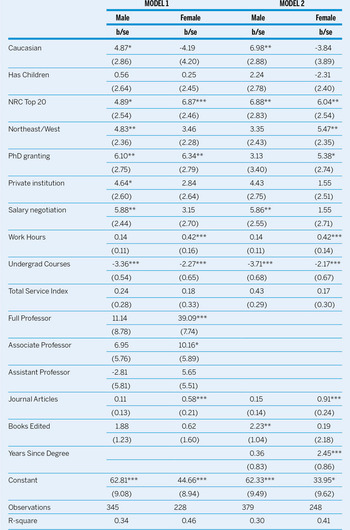
Unstandardized estimated coefficients are presented with standard errors in parentheses. *p < 0.10, **p < 0.05, ***p < 0.01.
We also estimate models separately for different academic ranks (table 3). Perna (Reference Perna2001) cautions that salary determination processes vary across different rank/experience cohorts. Footnote 22 Thus, the relative effects of human capital, productivity, and structural characteristics on faculty salaries may differ across different levels of academic rank and experience. Several authors have argued that the inclusion of academic rank in salary models tends to mask latent gender (and possibly race) differences in the promotion and tenure process (Boudreau et al. Reference Boudreau, Sullivan, Balzer, Ryan, Yonker, Thorsteinson and Hutchinson1997). Given that both salary and faculty rank models include similar explanatory variables, it should be expected that rank would be correlated with the error term when it is included in salary models (Strathman Reference Strathman2000). This will potentially result in biased parameter estimates. Rank should thus be treated as an endogenous variable in salary models (Ramsay Reference Ramsay, Pezzullo and Brittingham1979).
Table 3 OLS Salary Regression Split by Academic Rank
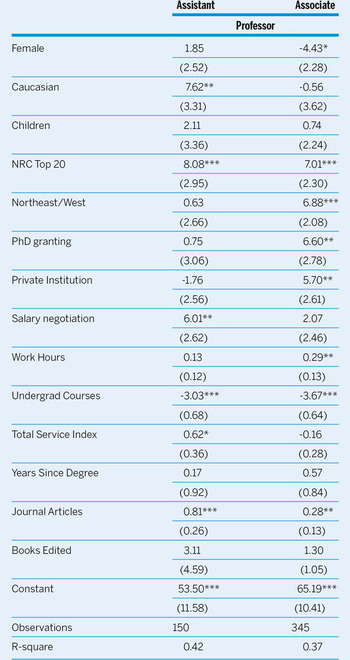
Unstandardized estimated coefficients are presented with standard errors in parentheses. *p < 0.10, **p < 0.05, ***p < 0.01.
Given that we are examining a cohort that received their doctoral degrees between 2003 and 2008, we have few full professors in the sample that was surveyed in 2014. Therefore, table 3 presents split sample models by rank for assistant and associate professors, but not for full professors. These models highlight important ways that demographics predict salary. At the assistant professor level, no statistically significant wage gap between males and females exists. There is, however, among assistant professors, a large salary advantage for those reporting to be Caucasian ($7,620 higher salary). At the associate professor level, the effects of these variables change. The gender wage gap for females is negative and significant ($4,430), but we no longer see a significant effect of being Caucasian. Structural variables are important for determining the salary of associate professors, but not assistant professors. Behaviorally, among assistant professors, negotiating one’s salary at the time of being hired into one’s job significantly boosts salary, while among associate professors, salary is boosted by working more hours. Noteworthy is that more service is associated with higher salaries among assistant professors, but not associate professors. For both assistant and associate professors, the greater the teaching load, the lower the salary, while a greater number of journal publications increases salary.
Noteworthy is that more service is associated with higher salaries among assistant professors, but not associate professors.
The final model that we report is a Heckman selection model. Having a salary variable as our dependent variable, our analysis directly faces the problem of sample selection (Heckman Reference Heckman1979). Since individuals’ choice to participate in the labor market is not random, selection bias is expected in a given sample with individuals’ reported income. We address this issue by relying on a two-stage Heckman probit model, in which the sample selection process is directly estimated. In the first stage, we use publications while in graduate school as the selection criterion and employ the following predictors: graduate school satisfaction, a dummy variable for having children, and a dummy variable for having had a graduate school research assistantship. In the second stage, given the observation is selected on this process, our analysis estimates the effects of the variables included in the baseline model on individuals’ salary. The results are presented in table 4.
Table 4 Heckman Selection Model for Salary
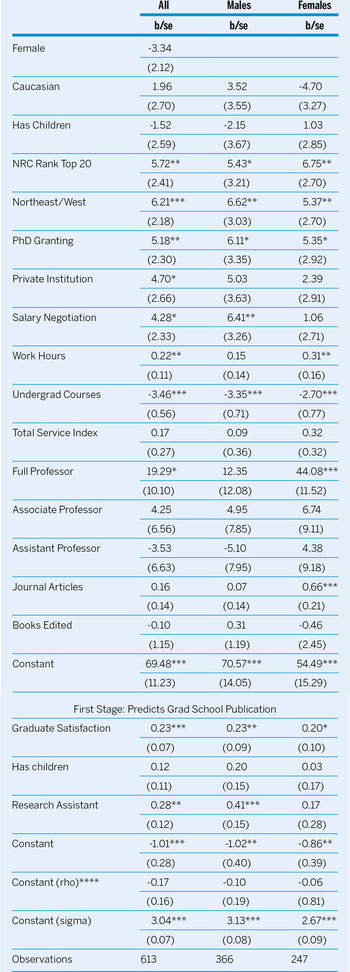
* p < 0.10, **p < 0.05, ***p < 0.01.
**** When Rho is negative, this indicates that any unobserved variable that makes selection into the sample more likely also decreases salary.
The important finding associated with the Heckman model in table 4, is the continuing importance of journal publications for higher female salaries, as well as their statistical irrelevance to the salaries of males. The estimation of the Heckman model also confirms that negotiation matters for the salaries of men, but not women. These findings resemble the differential effects for men and women of journal publications and negotiation on salary found in table 2. Race, however, plays no significant role once we select based on whether the respondent had a publication in graduate school.
DISCUSSION
In specifying models for the analysis, we carefully addressed four types of methodological issues. First, when concerns exist about multicollinearity among variables, such as the systematic relationship between the number of years since graduation and faculty rank, we do not put them into the same model. Second, given that we expect gender and rank to affect other predictors of salary, we split the sample (disaggregate) by gender and academic rank, and then estimate our salary model separately among these subsets. Third, we account for heteroscedasticity by estimating a WLS model, and fourth, we account for selection bias with a Heckman model.
Indeed, a nuanced interpretation of the data requires this varied estimation approach. Let us first consider the negative salary gap for female faculty. The negative coefficient for female is significant in the OLS models 1 and 2, of table 1, but the significance disappears in the OLS models 3 and 4 when academic rank is added to the equation. The weighted least squares result in table 1 (model 5), shows the negative salary gap between male and female faculty. When we look at table 3, which splits the sample between assistant and associate professors, the negative salary gap for females is significant for associate professors, but not for assistant professors. This finding can be further understood by looking at the average reported salaries for female and male assistant professors ($73,994 and $69,982, respectively) and female and male associate professors ($79,274 and $83,330, respectively). Footnote 23 The implication is that women start off with somewhat higher salaries at the assistant professor level (although the difference is not significant given other controls in the model (see model 1 of table 3)), but that female academics are paid significantly less on average as they become associate professors (this negative salary gap among associate professors is statistically significant (model 2 of table 3)).
Women fall behind men in terms of faculty salaries when they progress through the academic ranks. Differences between men and women in negotiation frequency, timing, style, and administrator response are all likely responsible for the larger salary gaps at the associate professor and full professor levels. Noteworthy in this regard is our finding reported above of the different effect of negotiation on salary for men as compared with women. In general, we conclude that negotiation improves salary. As reported above, however, this finding, does not hold when we look at female faculty only (tables 2 and 4). Thus, the general finding of the importance of salary negotiation is driven by the negotiation success of men, not women.
If men complain more frequently than women about their raises and get more outside offers, this will create increasing gaps over time. Our own personal observations reveal that men who go on the market are more likely to receive offers than women on the market. This also means that men are in a better position to get counteroffers. We also know from our previously published work that women publish less frequently than men and take on more service, teaching, and advising (Hesli and Lee Reference Hesli and Lee2011; Mitchell and Hesli Reference Mitchell and Hesli2013), so these likely have accumulating effects toward larger salary gaps among male and female faculty at higher ranks.
If we take into consideration the selection bias behind entry into a faculty position associated with publications in graduate school, the female salary gap is insignificant, again indicating that the negative forces associated with being a woman are more likely to manifest themselves around the promotion process to associate professor, rather than at the initial stage of being hired as an assistant professor. Although we cannot support this with data, we speculate that reporting requirements and information sharing about salaries paid to newly employed assistant professors push toward gender equality in salaries, at this stage. In contrast, salary data is less readily available and not as transparent at the stages of tenure and promotion to associate professor. Thus, the more insidious forces that contribute to the gender gap in faculty salaries can more easily filter into the decision-making processes at these later stages. We advise individual institutions of higher education to respond with corrective measures, should analyses of their own salary data reveal significant gender gaps left unexplained with appropriate controls.
With regard to race, the significant positive effect on salary of being caucasian is apparent in models 1 and 5, of table 1, among male professors in table 2, and among assistant professors in table 3. Many accept that higher salaries should be used to reward superior performance. If, however, gender or racial pay gaps persist, even when models include controls for human capital and output, discrimination may be occurring. As with gender gaps, administrators should be on guard against the manifestation of race-based salary gaps.
The effects of the human capital variables were as expected. The positive effect on salary associated with graduation from a highly ranked PhD program is quite consistent across the different estimation procedures. This reveals the lasting importance of one’s graduate school experience, and the importance (for salary) of attending one of the more highly ranked programs. Problematic is the systematic negative effect on salary of a high undergraduate teaching load. It is clear that large undergraduate teaching loads undermine salary. Thus, our salary reward structures may need reconsideration if we value teaching.
The effect of the service index on faculty salary, however, is more dependent upon other predictors in the model. In general, we can say that more service is related to higher salaries (see WLS result in table 1), but the effect of service on salary disappears when we split the sample and look at men and women separately. Here, the implication is the different service levels are correlated with gender (Mitchell and Hesli Reference Mitchell and Hesli2013). Noteworthy as well is the finding from table 3 that more service is associated with higher salaries among assistant professors. Oft, assistant professors are advised to avoid service, but our results reveal that more service boosts the salary of an assistant professor.
Structural variables had differential effects on associate, in contrast with assistant professors. When the structural predictors do have significant effects, these are in the expected directions. Interesting is that being employed at a private institution or within a PhD-granting department has its effective boost on salary when or after one is promoted to associate professor, rather than at the entry, assistant-level rank (table 3).
With regard to research productivity, we focused our analysis on the two best performing indicators: the number of journal publications and the number of edited books. Publishing more journal articles appears, in general, to have a positive effect on salary, however, we find that more journal publications boost only the salary of females, not males (tables 2 and 4). Male faculty may find this result – that their salaries are not responsive to their research productivity – disconcerting, but we think the differential effect of journal publications on salary for men, versus women, is even more troubling for female faculty. Other results using APSA survey data show that women publish significantly fewer articles than men (Hesli and Lee Reference Hesli and Lee2011). The sample we employ herein confirms this pattern as well, with the mean publication values being higher for male faculty than female faculty on most productivity dimensions. Footnote 24 Thus, while women can improve their salary through publishing, other structural factors work against their success on the publication front, which may help to explain why we observe a larger gender salary gap at the associate professor rank.
In summation, it appears that research productivity is an important criterion when determining the salary of female academics, but not males. (Note that the salaries of males are determined by human capital and structural forces, and by negotiation.) If research productivity is more critical for the salaries of females as compared to males, then the institution must ensure that female faculty have adequate access to the opportunities that promote research productivity (Perna Reference Perna2001, 305). Such factors include leave opportunities, lower teaching loads, support for securing external funds, reduced advising and service responsibilities, and access to graduate research assistance. We also reiterate the point that we made above: that more transparency in the salary assignment process could help prevent subtle biases that contribute to the negative salary gap for female faculty. Salaries should be widely publicized and salary differentials should be explained and justified to all faculty members, thus leading to more accountability and possible corrections in the salary assignment process. Future research should delve more deeply into institutional policies and procedures that might help to alleviate the gender gap in salaries, documented herein.
SUPPLEMENTARY MATERIAL
To view supplementary material for this article, please visit https://doi.org/10.1017/S104909651600233X