Not only is the prevalence of obesity ever increasing on a global scale, but so is the number of individuals living with dementia and cognitive impairment: the number of people living with obesity has nearly tripled since 1975 to over 650 million worldwide(1). For dementia, the Global Burden of Disease Dementia Forecasting Collaboration estimated an increase from 57 million to 153 million cases from 2019 to 2050(Reference Nichols, Steinmetz and Vollset2). This not only poses a threat to individual health, but also displays an ever increasing rise in health care costs for society, both for obesity and dementia the annual costs of obesity-related illness in the UK, for example, will likely rise from £6⋅1 in 2017 to £9⋅7 billion in 2050(Reference Holmes3), and those of dementia will likely double from £26 to £55 billion until 2040 in the UK(Reference Prince, Guerchet and Mccrone4).
These numbers underline the importance to understand the drivers of obesity and of accelerated brain ageing, and to develop effective prevention strategies. Notably, both conditions may benefit from interventions that ultimately target both metabolic and neuronal function: overnutrition and weight gain have been linked with abnormal functionality of homoeostasis brain networks and subtle changes in cognitive functions(Reference Garcia-Garcia, Michaud and Jurado5,Reference Thaler and Schwartz6) . In parallel, obesity in midlife increases the risk to develop dementia(Reference Garcia-Garcia, Michaud and Jurado5,Reference Lee, Woodward and Batty7–Reference Hassing, Dahl and Thorvaldsson9) , probably through metabolic, inflammatory and vascular routes (e.g.Reference Garcia-Garcia, Michaud and Jurado5,Reference Lee, Woodward and Batty7,Reference Tabassum, Misrani and Yang10,Reference Gupta, Osadchiy and Mayer11) ) (Fig. 1, left).
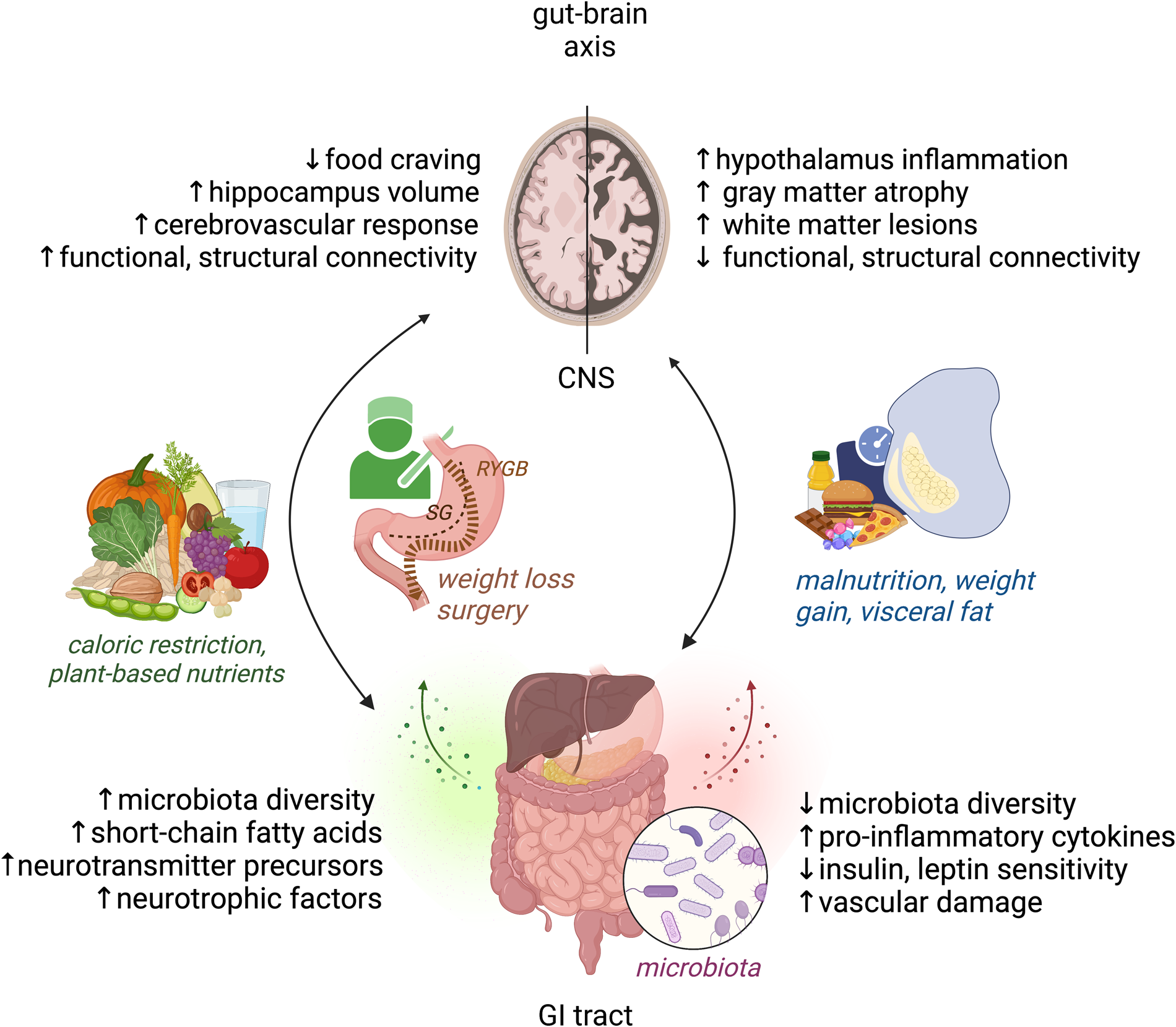
Fig. 1. Modifiable factors of the bi-directional gut–brain axis cross-talk, relevant to obesity and brain health. Right: Malnutrition, weight gain and visceral fat accumulation are linked with detrimental structural and functional brain changes as well as disbalance in microbiota, inflammation, metabolic and vascular factors. Left: Weight loss and plant-based dietary factors link to improved structural and functional brain changes as well as beneficial microbiota and metabolic mediators of brain health. CNS, central nervous system, GI, gastrointestinal, RYGB, gastric bypass, SG, sleeve gastrectomy. Figure created with Biorender.com.
This narrative review evaluates the link between obesity and brain structure and function based on population neuroimaging, explores the potential of diet, weight loss and the gut–brain axis to modify cognition and brain health (Fig. 1, right), and scrutinises new open science ways to improve reliability of nutrition neuroimaging studies.
Obesity and brain structure and function
In the past few decades, midlife obesity has repeatedly been shown to increase the risk for dementia and cognitive decline later in life(Reference Garcia-Garcia, Michaud and Jurado5,Reference Lee, Woodward and Batty7) , suggesting causal routes or common aetiologies between obesity and dementia. Due to the assumed very slowly progressing disease in the pre-symptomatic and early phase of dementia, enciphering subtle changes in neuroimaging markers of brain health in population-based studies attracts increasing research interest. Such ‘big data’ is becoming increasingly available in large-scale collaborative efforts, for example the UK Biobank(Reference Lee, Woodward and Batty7,Reference Singh-Manoux, Dugravot and Shipley12) , the German National Cohort(Reference Bycroft, Freeman and Petkova13) or the US OASIS-3 study(Reference Schipf, Schöne and Schmidt14). However, the neurobiological underpinnings of how obesity contributes to accelerated brain ageing are only beginning to be understood.
Brain age prediction
In a series of studies, we assayed high-resolution brain MRI techniques to examine modifiable predictors of changes in brain health markers using data from LIFE-Adult, a deeply phenotyped population-based cohort(Reference Loeffler, Engel and Ahnert15) with MRI data of about 2600 randomly selected adults (47 % female, 18–80 years of age, BMI 18–47 kg/m2), representative of the German middle- to older-aged population. Using novel deep learning ‘artificial intelligence’ methods, we observe that overweight and obesity, measured using BMI and waist-to-hip ratio, relates to a higher ‘brain age’(Reference Hofmann, Beyer and Lapuschkin16). Brain age has been conceptualised as the biological age of the individual's brain derived from structural MRI data in contrast to the true individual's age [for review, see(Reference Franke and Gaser17)]. For example, it has been found using data from the Alzheimer's disease neuroimaging initiative(Reference Franke and Gaser18) and LIFE(Reference Liem, Varoquaux and Kynast19) that a higher estimate of biological age, relative to the true chronological age, predicts cognitive decline in pathological and normal ageing. In addition, higher brain age related to cardiovascular risk factors(Reference Franke, Gaser and Manor20,Reference de Lange, Anatürk and Suri21) and estimates changed even in correlation with pregnancy, childbirth(Reference Luders, Gingnell and Poromaa22) and oestradiol levels during menstrual cycle in women(Reference Franke, Hagemann and Schleussner23). Notably, the obesity-associated higher gap in brain age further links to cognitive disadvantages in the LIFE cohort(Reference Hofmann, Beyer and Lapuschkin16), proposing brain age based on raw MRI a valuable tool for future precision medicine. In our openly available code(Reference Hofmann, Beyer and Lapuschkin16), we further provide three-dimensional image reconstructions showing each individual's brain regions that convey relevant information for their brain age prediction, such as lesioned white matter or enlarged ventricles in older participants. The new window into deep learning techniques thus not only offers the possibility to predict trajectories of cognitive ageing, but also helps to explain the underlying mechanisms of obesity-associated brain ageing with data-driven unsupervised methodology.
Changes in grey and white matter
Another approach is to assay neuroimaging correlates of subcortical and cortical grey and white matter inflammation or atrophy more directly using extensive hypothesis-driven MRI analyses pipelines. With this, a growing number of studies investigated the link to overweight and obesity across the adult lifespan, reporting mostly detrimental links between grey and white matter structure with measures of obesity (for a recent review, see(Reference Garcia-Garcia, Michaud and Jurado5)). Consistently, diminished volume or cortical thickness related to obesity or higher BMI in the prefrontal/orbitofrontal cortex and temporal areas [e.g. see(Reference Beyer, García‐García and Heinrich24); and meta-analysis in n 5882 individuals(Reference Garcia-Garcia, Michaud and Dadar25)]. Notably, ENIGMA (https://enigma.ini.usc.edu/) data proposed a mediating role of lower prefrontal cortex volume (and suggested changes in cognitive function) in mediating genetic risk for developing obesity(Reference Opel, Redlich and Kaehler26). Own work using diffusion-weighted MRI markers of hypothalamic inflammation indicated that less intact microstructure specifically in the hypothalamus correlated with a higher BMI(Reference Thomas, Beyer and Lewe27). This result is in line with previous work suggesting high-fat diet and weight gain to induce hypothalamus dysfunction(Reference Thaler, Yi and Schur28). In a large UK Biobank sample (n > 12 000), obesity measures were related to lower subcortical volumes(Reference Dekkers, Jansen and Lamb29), and in a sample of over 600 cognitively healthy adults aged 60 years and older, a higher BMI and more so visceral fat accumulation, measured using waist-to-hip ratio, correlated with subtle grey matter atrophy in widespread cortical, subcortical and cerebellar areas of the brain, even after adjustment for confounding effects of age, sex/gender, education and obesity-related co-morbidities(Reference Beyer, Kharabian Masouleh and Kratzsch30). Further work suggested that obesity also impacts the functional and structural coupling between brain areas of the default mode, the salience and reward network in young and old adulthood(Reference Beyer, García‐García and Heinrich24,Reference Dekkers, Jansen and Lamb29,Reference Beyer, Zhang and Scholz31–Reference Park, Park and Morys33) . In most studies, differences in neuroimaging correlates of overweight and obesity further explained subtle inter-individual differences in memory and executive function performance, measured using wordlist learning, trail making tests and verbal fluency tests.
Inflammation and white matter lesions
Together, these findings support the hypothesis that brain and cognitive differences may pre-dispose individuals to high-caloric food craving and excessive weight gain(Reference Volkow, Wang and Baler34). In parallel, overweight and obesity might promote or trigger brain damage, possibly through neuronal and vascular mechanisms(Reference Hakim35–Reference Olsthoorn, Vreeken and Kiliaan37). Particularly considering visceral fat, several large-scale studies could support preclinical evidence suggesting a pathomechanistic link between visceral fat, chronic low-grade systemic inflammation and accumulation of white matter brain lesions, presumably of vascular origin(Reference Lampe, Zhang and Beyer38,Reference Morys, Dadar and Dagher39) . For example, in 1825 adults (aged: 20–82 years; BMI: 18⋅4–55⋅4 kg/m2), a whole-brain analysis of fluid attenuated inversion recovery inversion-MRI detected that a higher waist-to-hip ratio, indicative of visceral obesity, predicted more frequent white matter hyperintensities in the deep white matter (Fig. 2)(Reference Lampe, Zhang and Beyer38). Statistical mediation analyses proposed that higher visceral fat led to white matter lesions through higher levels of IL-6 in blood(Reference Lampe, Zhang and Beyer38), potentially indicative of a higher release of proinflammatory cytokines from visceral fat. The Saguenay Youth Study in 872 adolescents (12–18 years, 48 % males) indicated similar mediations of visceral fat-related systemic inflammation on coarse measures of white matter structure, suggesting detrimental obesity-related effects on brain health already early in life(Reference Syme, Pelletier and Shin40). However, due to the cross-sectional nature, these datasets could not differentiate between causation and causality in this regard. Further analyses of e.g. prospective UK Biobank data(Reference Morys, Dadar and Dagher39) or of the newly acquired 6 years follow-up assessment in the LIFE-study (n about 1000) will help to confirm or refine these findings.

Fig. 2. Relation between regional white matter hyperintensities and higher waist-to-hip ratio (linear, red), and higher age (exponential, yellow; TFCE, P < 0⋅05, FWE-corrected). n 1825. FWE, family-wise error; TFCE, threshold-free cluster enhancement. Figure taken from(Reference Lampe, Zhang and Beyer38).
Relation to gender and gender hormones
Both obesity and dementia prevalence show remarkable differences between gender, yet whether this stems from shared or even additive mechanistic pathways remains largely understudied. In a sample of 974 cognitively healthy adults, we examined the effects of gender and sex hormones on visceral fat accumulation in the LIFE-Adult cohort. Indeed, women accumulated visceral fat slightly later across adulthood, and with a less steep decline compared to men(Reference Zsido, Heinrich and Slavich41). Moreover, circulating oestradiol statistically moderated the negative effect of visceral fat on grey matter structural networks independent of age, i.e. that in women with higher oestradiol levels, the link between visceral fat and lower grey matter volume was less pronounced(Reference Zsido, Heinrich and Slavich41). A smaller study in twenty-three adult male twin pairs lends further support that circulating sex hormones, in this case testosterone, relate to both visceral fat and brain structure, i.e. MRI-derived brain measures of hypothalamic gliosis(Reference Berkseth, Rubinow and Melhorn42). While again longitudinal studies need to confirm these findings, they underline the need to conduct sex/gender-stratified analyses in population neuroimaging, and to better account for the gender-gap in biomedical research (see e.g.(Reference Sugimoto, Ahn and Smith43)).
Modifiable lifestyle factors may be protective and improve brain health
The earlier described findings convey the following considerations: if lifestyle-related factors, in particular weight status and visceral fat accumulation, are relevant for brain health, modification of those should be a possible route to counteract some of the detrimental effects on the brain. In addition, obesity-preventing factors triggering a mild stress response such as aerobic exercise or energetic restriction may exert unique beneficial effects on brain health through additional routes, such as longevity-gene expression and release of neurotrophic factors(Reference Mattson, Maudsley and Martin44). Indeed, work on intervention studies may give some insights into mechanistic evidence, how brain structure and function may be preserved or protected from age-associated disease by modifiable lifestyle factors. Tools for modifying weight status are obesity surgery in individuals with severe obesity and changing dietary patterns or supplementing dietary compounds.
Obesity surgery
Surgical therapy for weight loss has been discussed to convey more benefits to the patient than solely on the metabolic level, including neuroplasticity(Reference Makaronidis and Batterham45,Reference Hankir, Al-Bas and Rullmann46) . A considerable number of pre- to-post-surgery MRI analyses in relatively small sample sizes indeed indicated a certain recovery of structural and functional brain measures as well as cognitive performance after surgery [reviewed in(Reference Nota, Vreeken and Wiesmann47)], with the common limitation of lacking a longitudinal obese control group (see also Section ‘Challenges of MRI in obesity and intervention studies’): for an in-depth review on bariatric surgery and weight loss mechanisms, see(Reference Albaugh, He and Münzberg48). When compared to waiting list patients, a recent study found that reduction of stomach size (i.e. sleeve gastrectomy or gastric bypass) and related energetic restriction and weight loss did not change cognitive abilities significantly in a group of forty obese patients compared to twenty-nine controls, yet that post-surgery brain volumes in regions related to reward processing were reduced in size, while hippocampus volumes increased(Reference Prehn, Profitlich and Rangus49). Further, at a functional brain level, we found no strong intervention effects on the reward or default mode network, yet network connectivity was related to BMI change after surgery and when accounting for head motion during scanning(Reference Heinrichs, Beyer and Medawar50). Note also that bariatric surgery can lead to adverse outcomes such as regain in weight and depressive symptomatology in some individuals. Future controlled studies incorporating larger sample sizes now need to address the underlying mechanisms.
Diet and brain health
Diet is more and more regarded a potent tool to preserve and ameliorate brain structure and function in obesity and ageing(Reference Jensen, Leoni and Klein-Flügge51,Reference Scarmeas, Anastasiou and Yannakoulia52) , despite critical discussions on the limitations of nutritional research in epidemiology(Reference Giovannucci53,Reference Ioannidis54) . Besides energetic restriction as a promising approach to not only lose weight but to also benefit cognition and brain structure(Reference Jensen, Leoni and Klein-Flügge51,Reference Prehn, Jumpertz von Schwartzenberg and Mai55,Reference Witte, Fobker and Gellner56) , dietary modifications that do not explicitly involve fasting may be easier to adopt for most individuals(Reference Donnelly, Shaw and Pegington57). In a cross-sectional epidemiological analysis in >8000 adults, we found that less animal-based dietary intake (resembling vegetarian and vegan diets and those similar to Mediterranean diet) was linked to better weight status and to certain differences in personality traits, i.e. to lower extraversion, measured with the Big Five personality traits(Reference Medawar, Enzenbach and Roehr58). Brain health in relation to diet in this sample is currently under investigation.
Here, not only presumably lower energetic density in plant-based food but also specific nutrients and synergistic nutrient patterns may impact bottom-up gut–brain signalling and thereby potentially modifying brain and behaviour(Reference Gupta, Osadchiy and Mayer11,Reference Rogers, Keating and Young59) . For example, the gut microbiome has been discussed to influence eating behaviour via a combination of immune, endocrine, neural and humoral routes involving, among other, SCFA metabolism(Reference Dalile, Van Oudenhove and Vervliet60). Besides dietary intake of small amounts of SCFA through e.g. cheese and milk, the vast majority of SCFA stems from fermentation of dietary fibre in whole grain, legumes, vegetables and fruit by SCFA-producing gut microbiota. In a systematic review including twenty-seven studies we discussed the link between those plant-based dietary patterns and gut–brain communication and its potential role in mediating some of the beneficial effects of healthy diets on the metabolism and on behaviour and brain health(Reference Medawar, Huhn and Villringer61).
One possible mechanistic route could be fibre-derived SCFA, i.e. acetate, butyrate and propionate, that regulate hunger and energy homoeostasis(Reference Byrne, Chambers and Morrison62). In a translational study, propionate could stimulate gut peptide secretion in human colonic cells in vitro, and further induced weight loss via gut peptides in sixty overweight human subjects in a randomised cross-over controlled trial over 24 weeks(Reference Chambers, Viardot and Psichas63). Inflammation-related mechanisms have been suggested to mediate positive effects of pre- and probiotic treatments on the ageing brain, including implications for vascular dementia and stroke(Reference Medawar, Enzenbach and Roehr58,Reference Lee, Mohd Ismail and Wei64) , as well as Alzheimer's disease(Reference Pluta, Ułamek-Kozioł and Januszewski65).
However, human neuroimaging evidence of mechanistic links to eating behaviour or brain ageing is still anecdotal. In a recent cross-sectional analysis we observed that eating behaviour, measured with the Three Factor Eating Questionnaire was indeed linked to certain microbiotal genera such as Parabacteroides (phylum Bacteroidetes) and Roseburia (phylum Firmicutes) in a sample of twenty-seven young to middle-aged overweight adults. Further, we found in a second sample of twenty-three middle to old age obese and overweight patients about 2 years post-bariatric surgery, compared to seventeen BMI-matched controls, that those microbiotal genera were also related to treatment success after surgery(Reference Medawar, Haange and Rolle-Kampczyk66). Resting-state functional connectivity analyses of 287 obese and non-obese adults indicated that obesity links to a higher Prevotella to Bacteroides (P/B) ratio, which in turn correlated with higher centrality of the nucleus accumbens, a brain region involved in reward evaluation(Reference Dong, Guan and Mayer67).
Supplementary polyphenols
Single compounds of plant-based diets, such as plant polyphenols, have been studied more extensively on improving bodily functions and cognitive performance when given as supplements (these studies have been reviewed elsewhere e.g. in(Reference de Vries, Medawar and Korosi68,Reference Huhn, Kharabian Masouleh and Stumvoll69) ). In a small randomised clinical trial (RCT) of forty-eight middle and older, overweight, cognitively healthy adults, we found for example that supplementary resveratrol (200 mg/d in combination with quercetin) over 6 months improved retention memory in a verbal learning task, compared to placebo(Reference Witte, Kerti and Margulies70). In parallel, resveratrol significantly lowered glycated HbA1c in blood, a long-term marker of glucose control, and the reduction in HbA1c correlated with intervention-induced increases in functional coupling between the hippocampus and medial prefrontal areas, measured using resting-state functional MRI, indicating a mechanistic role of improved glucose metabolism (Fig. 3)(Reference Witte, Kerti and Margulies70). A follow-up RCT using the same dietary supplements, however, showed diverging results in a group of lean and overweight adults: here, we found no significant effect on cognition or on volumetric markers of the hippocampus(Reference Huhn, Beyer and Zhang71). Others could show a significant effect of resveratrol on cerebrovascular response in postmenopausal women, but not on cognition(Reference Evans, Howe and Wong72,Reference Thaung Zaw, Howe and Wong73) . Across a recent systematic review of sixty-six RCTs including a meta-analysis on a subset of forty-eight studies, a similar inconclusive picture emerged considering effects of resveratrol and other polyphenols on memory performance: while on average, polyphenols exerted some beneficial effects on healthy and cognitively impaired mid- to late life, results were heterogeneous and a positivity bias in reporting could not be excluded(Reference Medawar, Huhn and Villringer61,Reference de Vries, Medawar and Korosi68,Reference Huhn, Kharabian Masouleh and Stumvoll69,Reference Huhn, Beyer and Zhang71) .

Fig. 3. Effects of supplementary resveratrol on memory retention performance, glycated HbA1c in blood and functional connectivity (FC) of the hippocampus after 6 months, compared to placebo, in forty-eight overweight older participants. Figure adapted from(Reference Witte, Kerti and Margulies70). SE, standard error; Prec = Precuneus; mPFC = medial prefrontal cortex.
In sum, plant-based dietary patterns and single nutrients as modifiers of brain health attract increasing research interest, but drawing definite conclusions based on the available data remains difficult. As outlined earlier, some studies indicate beneficial effects of weight loss and healthy diets on behaviour, cognition and markers of brain ageing, while others do not support these claims. What drives the unequivocal study results? On the one hand, both obesity and brain ageing are likely multi-factorial conditions that evolve over long-term time periods, rendering small effect sizes difficult to detect. Moreover, a careful evaluation of the presented study outcomes suggests that confounding effects related to common challenges in designing and monitoring dietary RCTs, and those inherent to neuroimaging acquisition, analysis and inference testing may help to explain some of the divergent findings. Consequently, implementing measures to foster quality and methodological rigour could move the field forward when studying diet and obesity in relation to brain and behaviour.
Ways to improve evidence on the influence of lifestyle factors on brain health and the importance of open science
Monitoring, study duration and standardisation
In a previous RCT testing the effects of dietary polyphenol intake compared to placebo(Reference Huhn, Beyer and Zhang71), we observed concurrent changes in other lifestyle factors over the course of the intervention: both intervention and control groups showed significant increases in body weight and reported less physical activity and higher energetic intake at follow-up, which might seem insightful given that baseline appointments were in spring, and 6-months follow-up in winter about Christmas time in Germany. In addition, taking daily supplements such as pills might be perceived a healthy habit, leading to less stringent health behaviours in daily routine such as unhealthy dieting, thereby potentially masking or ‘overriding’ the effects of the supplemented nutrient, similar to what has been described as barriers to implement lifestyle changes (see e.g.(Reference Stonerock and Blumenthal74)). Therefore, estimated small effects of single-lifestyle factors should be tested in carefully designed RCTs, accounting for seasonal or competing lifestyle changes with e.g. a longer intervention period and through a tight monitoring of lifestyle habits and compliance to the study instructions.
In free-living situations, these could include for example offered prepared meals delivered at e.g. the work place or home, educative counselling, regular phone calls of study staff, dietary and physical activity protocols, supplement counting, app-based engagement with the study or other means to improve participant's study engagement. However, quantifying e.g. dietary intake in real-world scenarios remains challenging(Reference Konig, Van Emmenis and Nurmi75), and novel tools to analyse nutrient intake based on food frequency questionnaires or dietary protocols considering polyphenols and dietary fibre(Reference Medawar, Witte and Thieleking76,77) .
In addition, implementing sensitive behavioural and cognitive tests can contribute to improving signal-to-noise ratio. Here, adopting standard operating procedures and using carefully validated and updated test versions and stimuli to use in test–retest study settings is a necessary pre-requisite for reliable data acquisition. These could help to reduce unknown biases stemming from different interviewers and test versions or handling of sample material. For openly available examples of computer-assisted tests and stimuli used in our group, see e.g. the mnemonic similarity task(Reference Stark, Kirwan and Stark78), Food-pics database(Reference Blechert, Lender and Polk79), art.pics database(Reference Thieleking, Medawar and Disch80) and a German version of a food frequency questionnaire including nutrient scoring(Reference Thieleking, Schneidewind and Kanyamibwa81).
Challenges of MRI in obesity and dietary intervention studies
Implementing MRI in nutrition and obesity studies is a potent tool to detect early signs of brain ageing and offers the possibility to explore neurobiological mechanisms of high-energetic food craving in the living human brain. These exciting possibilities, however, rely on complex physics, sophisticated reconstruction of the head's magnetic resonance signals into interpretable intensity values and subsequent multivariate statistics, and all these aspects come along with potential sources of variability between study outcomes(Reference Botvinik-Nezer, Holzmeister and Camerer82).
Starting with data acquisition, we observed that a common image artefact resulting from head micromovements in the scanner during MRI measurement is systematically more severe in participants with a higher BMI, and that profound weight loss (up to 5⋅5 kg/m2) after bariatric surgery reduces within-scanner head motion(Reference Beyer, Prehn and Wüsten83). Indeed, removal of motion-related physiological artefacts from breathing in MRI data can be effectively removed(Reference Power, Plitt and Gotts84). As head motion affects the estimates of all kinds of neuroimaging outcomes, the collinearity between head motion and BMI confounds all BMI-related statistical inference in a given dataset. Therefore, head motion should be rigorously controlled for in neuroimaging of participants that vary in BMI, at best already during data acquisition, for example with simple tapes on the participant's head that effectively reduce head movements(Reference Heinrichs, Beyer and Medawar50,Reference Krause, Benjamins and Eck85) .
In particular for longitudinal or multi-centre studies, reliability and comparability of magnetic resonance-based brain measures are also essential. For the MRI scanner used in the LIFE-Adult study, we assessed the potential impact of a scanner upgrade on data comparability in a within-subject design. Across two modalities, structural and diffusion-weighted brain imaging, we found significant differences between scanner versions, both in concrete outcome data (e.g. regional grey matter volume), and in the magnitude of effects of interest, such as ageing effects(Reference Medawar, Thieleking and Manuilova86,Reference Thieleking, Zhang and Paerisch87) . This highlights the need to invest in a posteriori MRI harmonisation tools in MRI analysis, and at best to plan wisely before changing MRI hard- and software in-between longitudinal assessments.
Opportunities of open science
In the past decade, important attempts have been made to enhance transparency and harmonise assessments, analyses and reporting of nutrition neuroimaging(Reference Smeets, Dagher and Hare88). Since recently, the open science movement has developed further tools that are capable to decisively improve the scientific evidence of our research: researchers are encouraged to pre-register, to adequately power studies, to publish null or negative results, to make code and data openly available and other open science practices improving transparency and reliability of research evidence(Reference Allen and Mehler89,Reference LeBel, Campbell and Loving90) . In neuroimaging in particular, recent efforts have been made to harmonise data structure(Reference Gorgolewski, Auer and Calhoun91) and brain measures of interest(Reference Pomponio, Erus and Habes92) across different studies to not only be able to pool data but also to compare results more directly. In nutrition-related neuroimaging, a set of good practices have been developed, including methodological and reporting-related practices(Reference Smeets, Dagher and Hare88).
Overall, the field has to cope with flaws or shortcomings in study design, such as cross-sectional and observational data leading to limitations in drawing causal conclusions. Instead, RCTs, as the gold standard, also applying double blinding, mid- to long-term interventions in a cross-over within-subject design should be pursued as an overall high-quality standard. As these are cost-intensive studies and not affordable for all researchers though, data sharing and meta-analysis need to be facilitated to enable diversity in analysis and interpretation of the available common data.
Pre-registering study analysis plans and hypotheses as well as publishing registered reports can help to move the field forward from publication bias of overly positive research results. False positives due to limited statistical power in studies with small samples can be improved by data pooling and multi-centre studies, as well as meta-analyses synthesising single-study results. Still, even with small-sized studies publications benefit from transparent reporting, and distinguishing confirmative from explorative analyses(Reference Dirnagl93). Further, global publication bias also includes that studies most often focus on Western educated industrialised rich democratic populations, which introduces skewed evidence and knowledge of selected societal strata(Reference Laird94,Reference LeWinn, Sheridan and Keyes95) . The research and publication process should be opened to more diverse, inclusive settings, which could be achieved by adopting more open science practices, in particular open data and open code.
Conclusion
We here summarised epidemiological MRI studies and interventional trials of the past decade indicating that overweight and obesity relate to changes in brain structure and function, and that targeting the mechanistic pathways of obesity-related brain damage through obesity surgery and dietary strategies might improve and preserve brain and cognitive health. Cautious interpretation of cross-sectional data analysis and related meta-analyses of polyphenol RCTs, though, urges the need to pursue more longitudinal MRI analyses and to implement methodological improvements including sensitive measures and pre-registration in dietary RCTs at a larger scale. Some attempts have been made in our group to start implementing this agenda: recently, we have finalised acquisition of a 6 years follow-up assessments of the LIFE-Adult study and assessed markers of brain health in the same individuals over longer time periods. In an ongoing analysis using linear-mixed models on within-person MRI change data, we now ask for example whether the effect of plant-based dietary adherence leads to less steep decline in estimated brain age scores, hippocampus atrophy and white matter lesions (osf.io/2nxfh). In parallel, fully pre-registered in(Reference Medawar, Witte and Beyer96), we ask using functional MRI whether a 2-week high-fibre intervention compared to placebo improves food-decision making through changes in gut–brain communication in overweight adults in a double-blind within-subject cross-over design. The expected data will help to clarify the role of the gut microbiota in gut–brain crosstalk and if plant-based dietary nutrients causally modify brain functions. Lastly, sustainability is not only a momentum to scientific methods such as open science, but research on obesity and dietary interventions may also help to acknowledge relevant impacts on society. For example, global shifts in obesity-related malnutrition (comprising cheap, often processed animal-based foods) towards a dietary consumer behaviour to adapt more plant-based diets is regarded a potent lever to reach societal public health and climate goals likewise, especially in Western countries(Reference Swinburn, Kraak and Allender97). Overall, future studies are warranted to further explore modifiers of healthy plant-based dietary choices, to potentially improve weight status and brain and cognitive health.
Acknowledgements
We thank all participants who took part in our studies. We thank Silke Friedrich for help with formatting.
Financial Support
This work was supported by grants from the German Research Foundation no. WI 3342/3-1 and 209933838 CRC1052-03 A1 (A. V. W.).
Conflict of Interest
None.
Authorship
The authors had sole responsibility for all aspects of preparation of the present paper.