Introduction
The use of complexity measures as indices of L2 development has a long history in second language research (see, e.g., Larsen-Freeman & Strom, Reference Larsen-Freeman and Strom1977) and together with accuracy and fluency, complexity continues to be considered a key feature in the evaluation of L2 proficiency, that is to say, “a person’s overall competence and ability to perform in L2” (Thomas, Reference Thomas1994, p. 330; Housen et al., Reference Housen, Kuiken and Vedder2012; Skehan, Reference Skehan1998). As discussed by Bulté and Housen (Reference Bulté and Housen2014), the implicit assumption underlying complexity measures is that as a learner becomes more proficient, their linguistic output will incorporate more complex language and structures (e.g., a wider range of vocabulary, more infrequent lexical items, more sophisticated syntactic structures). Although there are a multitude of different ways that linguistic complexity can be operationalized, the most commonly used measures usually focus on solely lexical or syntactic aspects of a text (Bulté & Housen, Reference Bulté, Housen, Housen, Vedder and Kuiken2012).
Paquot (Reference Paquot2019) argues that while such measures are useful in their own right, they fail to fully capture the development of complexity in learners’ interlanguage systems because they do not tap into complexity phenomena at the interface between lexis and grammar. As such, when used on their own, they have only limited validity as indices of L2 proficiency development. In particular, lexical and syntactic complexity measures fail to account for how words naturally combine to form conventional patterns of meaning and use (Sinclair, Reference Sinclair1991).
The study of such word combinations is usually referred to under the umbrella term of phraseology and includes a wide variety of co-occurrence and recurrence phenomena including, for example, collocations, idioms, lexical bundles, colligations, and collostructions (Granger & Paquot, Reference Granger, Paquot, Granger and Meunier2008). These units are phraseological in the sense that they involve “the co-occurrence of a form or a lemma of a lexical item and one or more additional linguistic elements of various kinds which functions as one semantic unit in a clause or sentence and whose frequency of co-occurrence is larger than expected on the basis of chance” (Gries, Reference Gries, Granger and Meunier2008, p. 6). Such units have been shown to be an important component in the development of L2 proficiency. For example, various studies have found that compared to beginners, advanced learners use phraseological units with a higher pointwise mutual informationFootnote 1 (PMI) (e.g., Granger & Bestgen, Reference Granger and Bestgen2014; Kim et al., Reference Kim, Crossley and Kyle2018; Kyle & Eguchi, Reference Kyle, Eguchi and Granger2021; Zhang & Li, Reference Zhang and Li2021) and that the PMI of phraseological units used by learners increases over time (e.g., Bestgen & Granger, Reference Bestgen, Granger, Hoffmann, Sand, Arndt-Lappe and Dillmann2018; Edmonds & Gudmestad, Reference Edmonds and Gudmestad2021; Siyanova-Chanturia, Reference Siyanova-Chanturia2015).
While the authors of these studies did not use the term complexity, Paquot (Reference Paquot2019) argues that in measuring PMI, they were in fact tapping into the complexity of learners’ phraseological systems. Paquot therefore attempted to build a bridge between L2 phraseology research and L2 complexity research by proposing the construct of phraseological complexity, which she defined as the diversity and sophistication of phraseological units (following Ortega, Reference Ortega2003). Phraseological diversity represents a learner’s breadth of phraseological knowledge. In the same way that lexical diversity measures such as the type-token ratio index the number of different lexical units (words, lemmas, lexemes) that the learner uses, and are therefore indicative of the size of the learner’s vocabulary, a learner production that displays a large number of different phraseological units is assumed to be indicative of a learner with a larger phraseological repertoire. Phraseological sophistication, on the other hand, represents the learner’s knowledge of “sophisticated” phraseological units, those units that may be more specific and appropriate to a particular topic, register, or style. Thus, a learner production with greater phraseological sophistication would be indicative of a learner who is able to draw on a larger phraseological repertoire to select more specific or informative expressions.
Empirical evidence suggests that phraseological complexity develops with L2 proficiency. For example, using a corpus of linguistics term papers written by L2 learners of English, Paquot (Reference Paquot2019) measured the complexity of three types of phraseological units that have been found to be difficult for L2 learners, namely, adjectival modifiers (e.g., black + hair), adverbial modifiers (e.g., eat + slowly), and direct objects (e.g., win + lottery). Each text in the corpus was manually assessed by a minimum of two professional raters who assigned it a global proficiency score ranging from B2 to C2 on the Common European Framework of Reference (CEFR) scale. When comparing across proficiency levels, Paquot found no significant differences in lexical or syntactic complexity but did find that phraseological sophistication, operationalized as the mean PMI of the units, increased significantly across proficiency levels. However, phraseological diversity, operationalized as the root type-token ratio (RTTR) of the units, was not significantly different across proficiency levels. Similar results were reported by Vandeweerd, Housen, and Paquot (Reference Paquot2021) who conducted a partial replication study using L2 French argumentative essays (B2–C2). While the diversity of adjectival modifiers did increase significantly from C1 to C2, this measure was not found to be an important predictor of the scores given to a text by professional raters, when controlling for other aspects of complexity (i.e., lexical, syntactic, morphological).
These studies suggest that the construct of phraseological complexity (in particular sophistication)Footnote 2 can be a useful index of L2 proficiency and development at the upper levels of proficiency but they are limited by the fact that the focus has been exclusively on the written mode. To make generalizable claims about the construct validity of phraseological complexity, it is important to compare phraseological complexity measures across various tasks and performances (Purpura et al., Reference Purpura, Brown and Schoonen2015). Moreover, each of the previously mentioned studies has used a pseudo-longitudinal design, comparing phraseological complexity across learners at different proficiency levels. As Paquot and Granger (Reference Paquot and Granger2012) point out, longitudinal studies are needed to identify patterns of phraseological development and allow the inference of more powerful cause and effect claims about L2 developmental processes (Ortega & Iberri-Shea, Reference Ortega and Iberri-Shea2005). While there are a handful of studies that have compared the longitudinal development of phraseology (Bestgen & Granger, Reference Bestgen, Granger, Hoffmann, Sand, Arndt-Lappe and Dillmann2018; Edmonds & Gudmestad, Reference Edmonds and Gudmestad2021; Kim et al., Reference Kim, Crossley and Kyle2018; Paquot et al., Reference Paquot, Naets, Gries, Le Bruyn and Paquot2021; Qi & Ding, Reference Qi and Ding2011), no study has yet directly compared the development of phraseology across oral and written L2 productions or compared the dimensions of diversity and sophistication across modes. This is important because not only the quantity but also the type of phraseological units may differ between modes (Biber et al., Reference Biber, Conrad and Cortes2004) and studies have shown that the patterns of phraseological complexity observed in L2 writing do not necessarily carry over to L2 speech. For example, Paquot et al. (Reference Paquot, Gablasova, Brezina, Naets, Lénko-Szymańska and Götz2022) measured the diversity (RTTR) and sophistication (PMI) of direct objects in a corpus of oral exams and found that, in contrast to the results for writing, there was a significant increase in diversity with proficiency (B2–C1) and a significant decrease in sophistication with proficiency (B1–B2), which they attributed to an increase in creativity on the part of the learners.
In comparison to writing, speech is usually more interactive, it tends to require more online processing and there is less control over output (Ravid & Tolchinsky, Reference Ravid and Tolchinsky2002). Importantly, because speech happens in real time, the limits of online processing constrain the amount of information that can be conveyed orally. This is especially evident in the case of L2 production due to the developing and less firmly entrenched state of the interlanguage system (De Bot, Reference De Bot1992). Under pressured conditions, writing is hypothesized to allow learners to devote more resources to conceptualizing, formulating, and monitoring linguistic output than is possible with speech because there is more opportunity for online (i.e., within task) planning (Skehan, Reference Skehan and Skehan2014). Along these lines, several studies have found higher levels of lexical diversity and/or sophistication in writing as compared to speaking in L2 production (e.g., Ellis & Yuan, Reference Ellis, Yuan and Ellis2005; Granfeldt, Reference Granfeldt, van Daele, Housen, Kuiken, Pierrard and Vedder2007; Kormos, Reference Kormos, Byrnes and Manchón2014; Kuiken & Vedder, Reference Kuiken and Vedder2011). In a similar way, writing may also promote higher levels of phraseological complexity. Biber and Gray (Reference Biber and Gray2013) compared oral and written responses to L2 English proficiency exams by counting the number of collocations for five highly frequent verbs (get, give, have, make, and take). The results showed that spoken responses had a higher quantity of frequent collocatesFootnote 3 for these verbs than written responses, suggesting that in the pressured situation of online speech production, learners were more likely to use highly frequent collocations but in the written task, learners were more likely to use less frequent and, hence, more sophisticated collocations. These results seem to be in line with psycholinguistic research showing that L2 learners process highly frequent collocations more quickly than infrequent collocations (Ellis et al., Reference Ellis, Simpson-Vlach and Maynard2008). In other words, to the extent that writing allows for more online planning, it may promote the use of more sophisticated word combinations.
However, characterizing modality as a binary distinction between writing and speaking is somewhat reductive because modality often intersects with register. Studies of register variation in a number of languages have found that certain types of speech and writing have similar linguistic features because they serve a similar communicative purpose (Biber, Reference Biber2019). For example, oral and written texts with a more “personal or involved” focus such as face-to-face conversations and personal letters tend to be more clausal in nature and are both characterized by the use of certain verb classes and similar types of verbal modification (Biber, Reference Biber2014). Texts that are more informational in purpose, however, tend to be more nominal in nature and are characterized by the use of noun phrases, attributive adjectives, and more lexical diversity. The communicative function of a text is also relevant at the level of phraseology. In a study comparing spoken and written academic corpora, Biber, Conrad, and Cortes (Reference Biber, Conrad and Cortes2004) found that although oral corpora contained a higher quantity of lexical bundles overall compared to written corpora, conversation and classroom teaching differed with respect to the type of lexical bundles they contained. Compared to conversation, classroom teaching was associated with a higher proportion of noun phrase based “referential bundles,” which the authors attributed to the informational purpose of those texts. These results suggest that both the diversity as well as sophistication of phraseological units used by a learner may be mediated by the register of a given task. For example, a learner may use a less diverse set of phraseological units because they are not required for the communicative purpose of a given task or they may use more sophisticated phraseological units because they are specifically elicited by the register characteristics of that task.
Regarding French, which is the target language of the learners in our study, no one has yet compared the development of phraseology across modes, but phraseological complexity has been shown to be associated with proficiency separately in each modality (Forsberg, Reference Forsberg2010; Vandeweerd et al., Reference Vandeweerd, Housen and Paquot2021)Footnote 4 and has been shown to increase over time during a study abroad (Edmonds & Gudmestad, Reference Edmonds and Gudmestad2021). While these studies suggest that phraseology develops with proficiency in French, no study has yet traced the development of phraseological complexity in terms of diversity and sophistication over time, nor compared the longitudinal development across oral and written tasks. The current study aims to address these gaps by comparing phraseological complexity development in oral and written tasks completed by both learners of French as well as L1 users of French, thus also improving on the state of the art by following Housen and Kuiken’s (Reference Housen and Kuiken2009) suggestion to include native benchmark data in studies investigating L2 complexity, accuracy, and fluency. Including L1 data in this way can help “explore which influences derive from tasks alone, rather than native speakerness” (Skehan & Foster, Reference Skehan, Foster, Van Daele, Housen, Kuiken, Pierrard and Vedder2008, p. 204). However, it should be noted that the inclusion of native data is not meant to serve as a goal toward which the learners should strive (cf. recent criticisms of native-speakerism in L2 teaching and research by, among others, Holliday, Reference Holliday2006 and Ortega, Reference Ortega2019) but rather as a benchmark group whose cumulative experience as users of the target language means that their linguistic system is likely “richer, more accessible and better organized” (Skehan & Foster, Reference Skehan, Foster, Van Daele, Housen, Kuiken, Pierrard and Vedder2008, p. 207) as compared to the learner group. In other words, L1 data can provide context for observed patterns of learner development (e.g., if the learners exhibit similar levels of phraseological complexity as the L1 group at the beginning of the study period, how much scope for further development can really be expected?). This question is especially important given that the construct of phraseological complexity is still new and so it remains an open question how much phraseological complexity is required to complete a task successfully and to date, no study of phraseological complexity has included a native benchmark group.
Our study sought to address these issues by investigating the complexity of two phraseological units (adjectival modifiers and direct objects) in a longitudinal corpus of oral and written production by learners of French and L1 users of French. Our research questions are the following:
RQ1. To what extent are there differences in phraseological complexity between oral and written tasks completed by the L1 group and the learner group?
RQ2. To what extent does the development of phraseological complexity differ between oral and written tasks completed by learners of French?
Methodology
Data
The data in this study come from the LANGSNAP corpus.Footnote 5 This corpus contains data from learners of French and Spanish in their second year of studies at a large university in the United Kingdom. The research team documented their language development over a 21-month period during which the students participated in a 9-month sojourn abroad. Data were also collected from a comparable group of L1 speakers (n = 10) who were Erasmus exchange students at the UK university. In the current study, we focus on the 29 learners of French as an additional language and the 10 native French speakers. The majority of the learners are L1 English speakers (n = 27) but the group also includes one L1 Spanish speaker and one L1 Finnish speaker. The mean age of the learner group is 21 (range 20–24) and includes 26 females and three males.Footnote 6 Prior to participation in the project, the learners had on average 11 years (range 9–15) of previous studies in French in an institutional setting. In addition to French, some of the participants were also studying other European languages (e.g., German, Spanish, Portuguese). For this reason, we refer to them as “learners of French” throughout. The background of the participants as well as their individual trajectories over the course of their stay abroad has been extensively reported elsewhere (see, e.g., Mitchell et al., Reference Mitchell, Tracy-Ventura and McManus2017) so we will not go into further detail here.
Data were collected from the learners on six occasions before, during, and following their stay abroad in France (see Table 1). At each collection point, they completed three production tasks. As a measure of general proficiency, the participants also completed an Elicited Imitation Test (EIT) at three time points (for details see Tracy-Ventura et al., Reference Tracy-Ventura, McManus, Norris, Ortega, Leclercq, Edmonds and Hilton2014). The three production tasks included a written argumentative essay, a semi-guided oral interview and a picture-based oral narrative. The argumentative essay task was set up to run offline on stand-alone computers. Participants were provided with one of three essay prompts in the following list, each of which was repeated once during the study:
-
• Penses-tu que les couples homosexuels ont le droit de se marier et d’adopter des enfants ? ‘Do you think that homosexual couples have the right to get married and adopt children?’
-
• Pensez-vous que la marijuana devrait être légalisée ? ‘Do you think that marijuana should be legalized?’
-
• Pensez-vous que, de manière à inciter les gens à manger sainement, on devrait taxer les boissons sucrées et les aliments gras ? ‘Do you think that in order to encourage people to eat in a healthy manner, sugary beverages and fatty foods should be taxed?’
Table 1. Data collection schedule (adapted from Mitchell, Tracy-Ventura, and McManus, Reference Mitchell, Tracy-Ventura and McManus2017, p. 56)
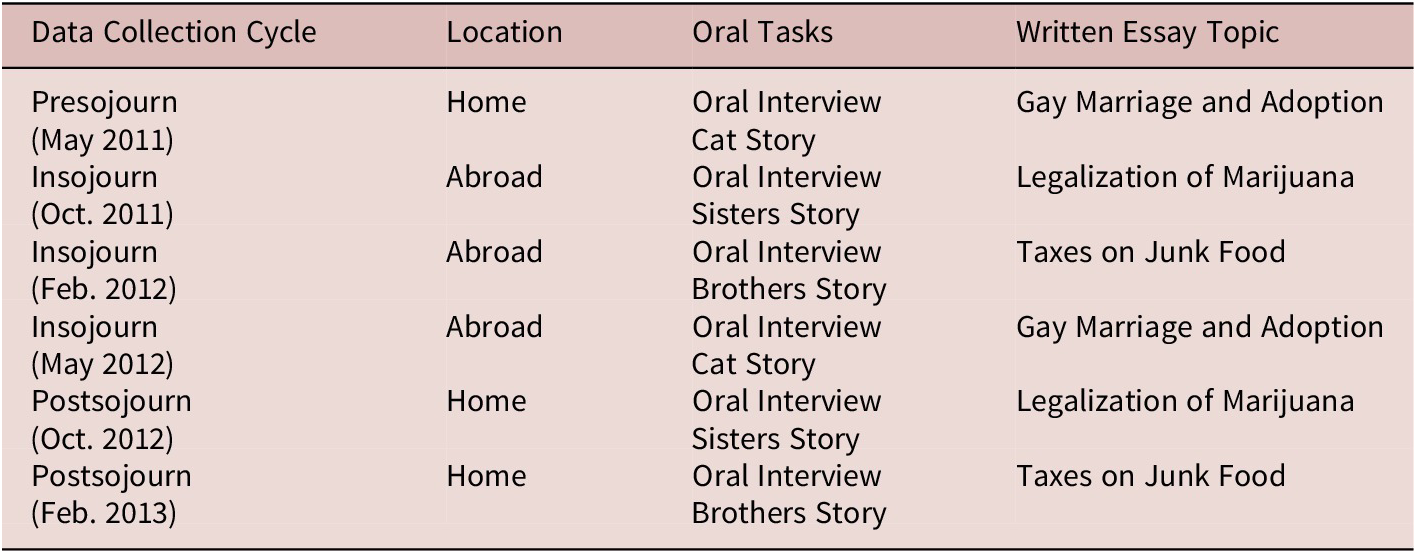
Participants were allowed three minutes for planning and note taking before being automatically taken to the writing page where they had 15 minutes to write approximately 200 words. After the time had elapsed, the program exited automatically and the participants could no longer edit the text. The oral narrative task involved an oral description of one of three picture-based narratives. Participants were given a short amount of planning time, during which they could ask clarification questions. Each of the stories were set in the past and contained a similar number of color images. They were also designed to elicit perfective and habitual events and contained two characters. The oral interview was a semi-structured interview administered by one of the researchers. Each interview followed a list of preestablished questions about the participants’ anticipations and experiences during their sojourn abroad. Questions were designed to elicit discussion of present, future, past, and hypothetical events.
To facilitate the extraction of phraseological units, the original CHAT transcripts of these tasks were converted to plain text files using in house R scripts (R Core Team, 2021). The text files were then lemmatized and part of speech tagged using TreeTagger (Schmid, Reference Schmid1994). Table 2 shows the median text lengths for each of the task types following preprocessing.
Table 2. Median text lengths across tasks at each collection point (IQR in brackets)
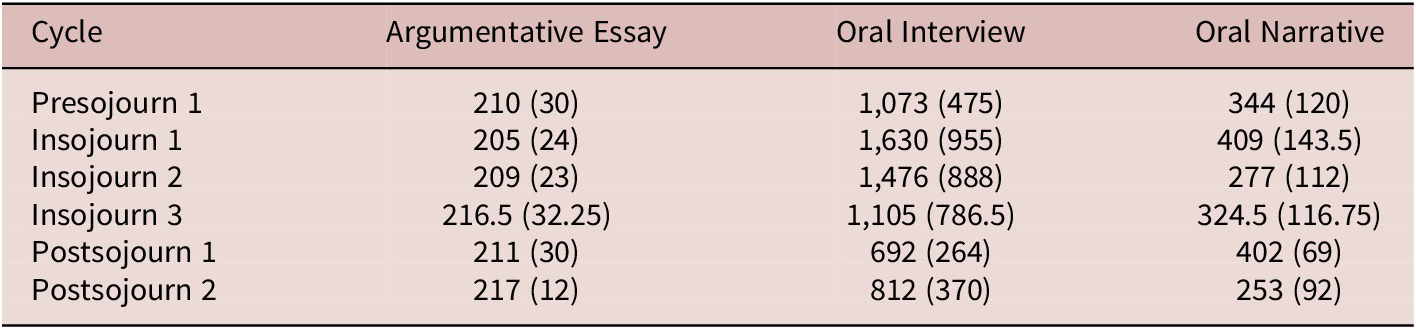
Phraseological Complexity Measures
We follow Gries’s (Reference Gries, Granger and Meunier2008) definition of phraseological units as the co-occurrence of two linguistic units at a frequency higher than expected on the basis of chance. Because phraseological complexity research is still relatively new, only a handful of possible phraseological units have thus far been examined. Two units, namely adjectival modifier (adjective + noun) and direct object (verb + noun) relations have received particular attention because previous research has shown that L2 productions tend to exhibit less variety in these units and that learners tend to produce units with lower collocational strength as compared to native speakers (Altenberg & Granger, Reference Altenberg and Granger2001; Durrant & Schmitt, Reference Durrant and Schmitt2009; Laufer & Waldman, Reference Laufer and Waldman2011; Nesselhauf, Reference Nesselhauf2005). We have decided to focus on these two units for the purpose of this study as well, given that the complexity of these units has been found to increase with proficiency levels in previous studies of phraseological complexity in L2 English, L2 Dutch, and L2 French (Paquot, Reference Paquot2019; Rubin et al., Reference Rubin, Housen, Paquot and Granger2021; Vandeweerd et al., Reference Vandeweerd, Housen and Paquot2021). The inclusion of one noun phrase-based unit and one verb phrase-based unit also taps into the potential register differences that may be induced by the different task types (as shown by Biber et al., Reference Biber, Conrad and Cortes2004). Specifically, in line with the literature on register variation, we expect the more personal or involved focus of the narrative and interview tasks will promote the complexity of direct objects whereas the informational focus of the essay will instead promote the complexity of in adjectival modifiers.
We wrote an R script to search the lemmatized versions of the texts and extract adjectival modifiers and direct objects using the part of speech tags generated by TreeTagger. The decision to use the lemmatized version of texts is due to the highly inflected nature of French (see Treffers-Daller, Reference Treffers-Daller, Jarvis and Daller2013). This is particularly important when comparing speech and writing given that many verbal inflections are only marked orthographically (and not phonologically) in French (see Blanche-Benveniste & Adam, Reference Blanche-Benveniste and Adam1999). To evaluate the reliability of the extraction method, a subset of 100 sentences from the written essays and 100 utterancesFootnote
7 from the oral tasks was annotated by two researchers for the presence of adjectival modifier and direct object relations following the definitions in Appendix 1. The annotators reached a high level of agreement for the units in both written (
$ {\kappa}_{amod} $
= 0.92,
$ {\kappa}_{dobj} $
= 0.84) and oral data (
$ {\kappa}_{amod} $
= 0.94,
$ {\kappa}_{dobj} $
= 0.95). All kappa values are above the minimal thresholds for reliability in SLA (0.83) according to Plonsky and Derrick (Reference Plonsky and Derrick2016). In total, there were 39 cases of disagreement between the two annotators. Each of these cases were discussed and the annotation guidelines were refined. Following these discussions, one researcher annotated a further 400 oral and 400 written sentences. The combined sets of 500 written and oral sentences were then used as baseline to evaluate the reliability of the automatic method. As shown in Table 3, F-scores, which represent the balance between precision (not identifying incorrect units) and recall (not failing to identify correct units) were found to be acceptable for both types of units. Table 4 shows the median number of adjectival modifiers and direct objects tokens per 100 words across the three task types.
Table 3. Comparison of manual and automatic annotation
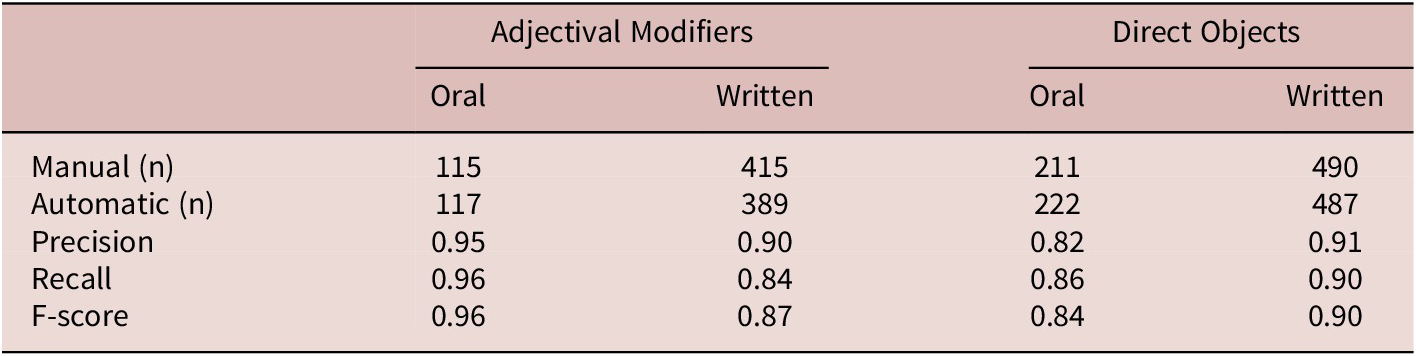
Table 4. Median number of tokens per 100 words (IQR)

Following Paquot (Reference Paquot2019), we measured phraseological complexity in terms of diversity and sophistication. Because of the large differences between the tasks regarding text length (see Table 2), measures were not calculated based on the entire text but rather as the average over 100-wordFootnote 8 moving windows, moving the window forward by an increment of 10 words after each sample. This is similar to a commonly used method used to control for text-length differences when calculating lexical diversity (MATTR; Covington & McFall, Reference Covington and McFall2010). A moving window-based approach was necessary because transformations of type-token ratios such as Guiraud’s (Reference Guiraud1954) root type-token ratio (as used by Paquot, Reference Paquot2019 and Vandeweerd et al., Reference Vandeweerd, Housen and Paquot2021) were found to be correlated with text length. Other more sophisticated diversity measures such as MTLD (McCarthy & Jarvis, Reference McCarthy and Jarvis2010) also could not be used because they require a minimum number of units (at least 100 as suggested by Koizumi, Reference Koizumi2012) and some texts in the corpus had few to no phraseological units of a given type.Footnote 9 To maintain comparability between the diversity and sophistication measures, we used this method for both types of measures. Phraseological diversity was operationalized as the average number of unique adjectival modifier and direct object types (i.e., unique units) in 100-word windows. Phraseological sophistication was operationalized as the mean PMIFootnote 10 score of adjectival modifiers and direct objects in 100-word windows.Footnote 11 As in Paquot (Reference Paquot2021), PMI was calculated on the basis of a large web-scraped reference corpus. The reference corpus used in this study was the 10-billion-word FRCOW16 corpus (Schäfer, Reference Schäfer, Bański, Biber, Breiteneder, Kupietz, Lüngen and Witt2015; Schäfer & Bildhauer, Reference Schäfer, Bildhauer, Calzolari, Choukri, Declerck, Doğan, Maegaard, Mariani, Moreno, Odijk and Piperidis2012). The FRCOW16 corpus is provided with dependency annotation generated by Malt Parser (Nivre et al., Reference Nivre, Hall, Nilsson, Calzolari, Choukri, Gangemi, Maegaard, Mariani, Odijk and Tapias2006) trained on the French Tree Bank (Abeillé & Barrier, Reference Abeillé, Barrier, Lino, Xavier, Ferreira, Costa and Silva2004). Adjectival modifiers and direct objects were extracted from the corpus on the basis of these dependency annotations using R scripts. We did not directly test the accuracy of this extraction method for the reference corpus given that it has previously been shown to have a relatively high level of accuracy (87% labeled attachment; Candito et al., Reference Candito, Nivre, Denis, Anguiano, Huang and Jurafsky2010). When it comes to adjectival modifiers and direct objects, we also reported high levels of reliability (F-scores of 0.81 and 0.82, respectively) for these units in a previous study (Vandeweerd et al., Reference Vandeweerd, Housen and Paquot2021). In addition, the FRCOW16 corpus contains POS tags generated by TreeTagger, the same POS tagger used to process the learner corpus. Following the method described by Paquot (Reference Paquot2019), a PMI value was calculated on the basis of the FRCOW16 corpus for the lemmatized version of each of the phraseological units extracted from the learner corpus. Units that contained a lemma unknown to TreeTagger (e.g., proper nouns, calques from English) were excluded as were units that occurred fewer than five times in the reference corpus and units that occurred in the writing prompts or were provided in the picture stories. The final list of extracted units was also checked and obvious examples of erroneous POS tags were also removed (e.g., hier, “yesterday” tagged as a verb). The mean PMI for adjectival modifiers and direct objects within each 100-word moving window was calculated for every text.
Analysis
We built mixed-effects regression models using the nlme package (Pinheiro et al., Reference Pinheiro, Bates, DebRoy and Sarkar2020) in R to predict each of the four phraseological complexity variables as outlined in the preceding text: (a) adjectival modifier types, (b) adjectival modifier PMI, (c) direct object types, and (d) direct object PMI. In each model, the phraseological complexity variable was the dependent variable. The main independent variables in the model were time (in months), task type, and the interaction between time and task type. Separate models were run for the L1 and learner data. For the learner models, given that previous study abroad research has shown a positive effect of initial proficiency on development while abroad (DeKeyser, Reference DeKeyser and DeKeyser2007), we additionally included an interaction effect between EIT.PRE (initial proficiency) and time in months to control for the moderating effect of initial proficiency. To control for topic differences (both between prompts and between tasks), we calculated the cosine similarity between each text and the first argumentative essay written by the same learner. This measure represents the similarity of vocabulary between the two texts and ranges from 0 (indicating no overlap in vocabulary) to 1 (Wang & Dong, Reference Wang and Dong2020). Including cosine similarity in the model allows us to account for the variation in phraseological complexity that is due to differences in vocabulary between different prompts and task types. Because this measure is quite right-skewed (most texts are dissimilar from each other), we applied a log transformation. Prior to modeling, the variables EIT.PRE (presojourn EIT scores) and COSINE.log (log of cosine similarity) were converted to z-scores. The random effects structure included random intercepts and by-participant random slopes for months. Planned orthogonal contrasts were set to compare written versus oral tasks as a whole and narrative versus interviews. The L1 models included only one fixed effect (task type) and included random intercepts for participants given that each participant provided seven different productions (for each task type and each topic). Initial models revealed problems with homoscedasticity that were resolved by weighting variance according to task type. For maximum comparability, we included all predictors in each model instead of using a model selection approach. This allows us to make clearer comparisons between models regarding our principle research questions.
The supplementary materials for this article are provided on an OSF repository.Footnote 12 These include the scripts used to preprocess the texts and extract phraseological units from the two corpora, further details about the process used to verify the reliability of the extraction method and calculate cosine reliability as well as the full model fitting procedure.
Results
The Learner Models
As shown in Table 5, significant predictors of adjectival modifier diversity (number of types) in the learner data included task type (both written vs. oral and narrative vs. interview), the interaction between task type (narrative vs. interview) and months (though this is right on the threshold for significance at an alpha level of 5%), and cosine similarity. In general, the written argumentative essays were found to have about 0.87 more adjectival modifier types per 100 words than both of the oral tasks and the interview task was also found to have 0.35 more adjectival modifier types than the narrative task. In terms of developmental trends, there was a significant effect of time in months, but this was not the same across all task types given that there was a significant interaction effect of task type and time in months. As shown in in Table 6, development was only significant in the case of the interviews, which decreased by 0.01 per month (the 95% confidence intervals for the slope (b) of the essay and the narrative overlap with 0). No other significant effects of time were found for the other task types and neither initial proficiency nor the interaction of initial proficiency and time in months was significant, indicating that initial proficiency did not have a direct effect on the number of adjectival modifier types used nor on the development of adjectival modifier types over the study period. There was, however, a significant effect of topic (COSINE.log) such that a one standard deviation increase in vocabulary similarity to the gay marriage and adoption essay was associated with the use of 0.07 more adjectival modifier types. In total, 65.47% of the variance in the number of adjectival modifier types was explained by this model.
Table 5. Adjectival modifier diversity (learner data)

Marginal R2: 0.631
Conditional R2: 0.655
Table 6. Linear trends for adjectival modifier types (learner data)

As shown in Table 7, the only significant predictor of adjectival modifier sophistication (PMI) was task type (written vs. oral). The average PMI of adjectival modifiers in written argumentative essays was higher by 0.38 as compared to the oral tasks. Neither the main effect of time in months nor the interaction of time in months with task type or initial proficiency was significant. The effect of topic was likewise nonsignificant. The predictors in this model explained 7.97% of the variance in adjectival modifier PMI.
Table 7. Adjectival modifier sophistication (learner data)
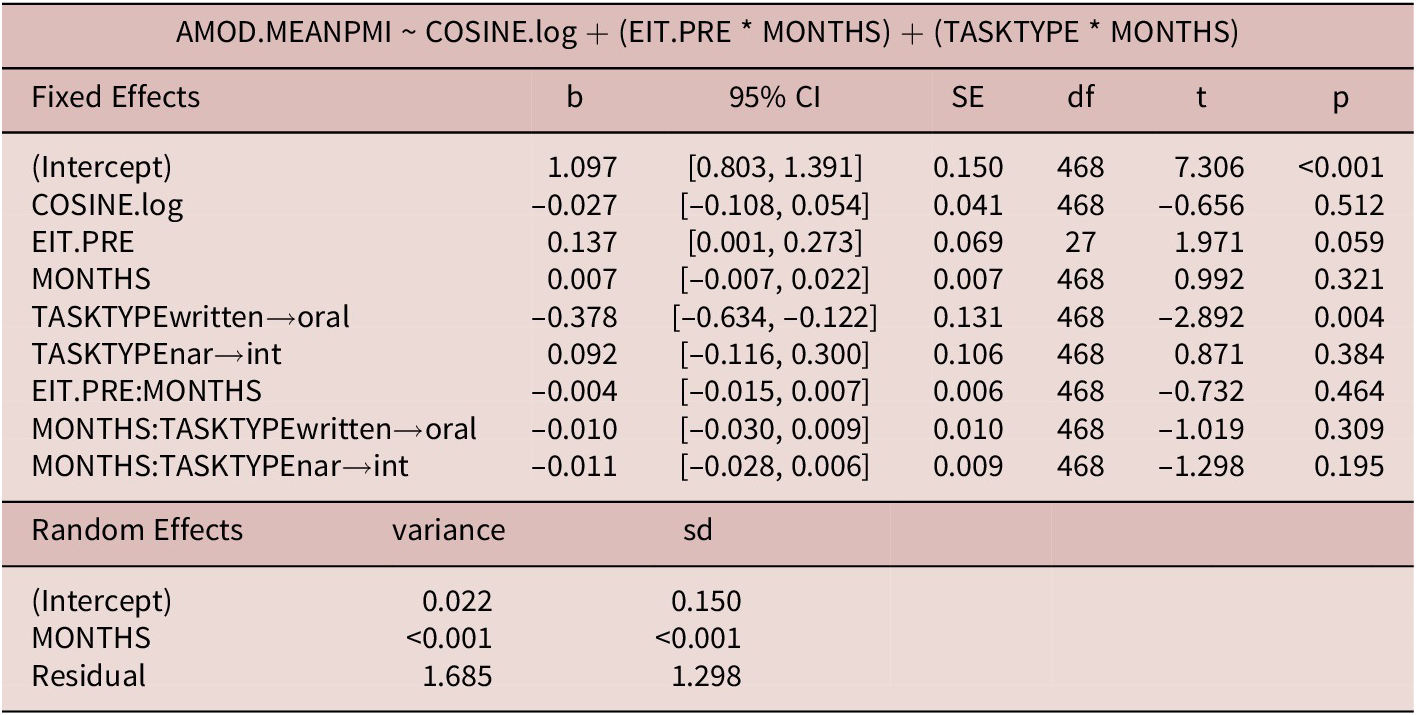
Marginal R2: 0.067
Conditional R2: 0.08
As shown in Table 8, the only significant predictor of direct object diversity (number of types) was task type (both written vs. oral and narrative vs. interview). In general, the written argumentative essays were found to have about 0.38 more direct object types per 100 words than both of the oral tasks and the narrative task was found to have 0.24 more direct object types than the interview task. Neither the main effect of time in months nor the interaction of time in months with task type or initial proficiency was significant. The effect of topic was also found to be nonsignificant. This model explained 26.16% of the variance in direct object types.
Table 8. Direct object diversity (learner data)
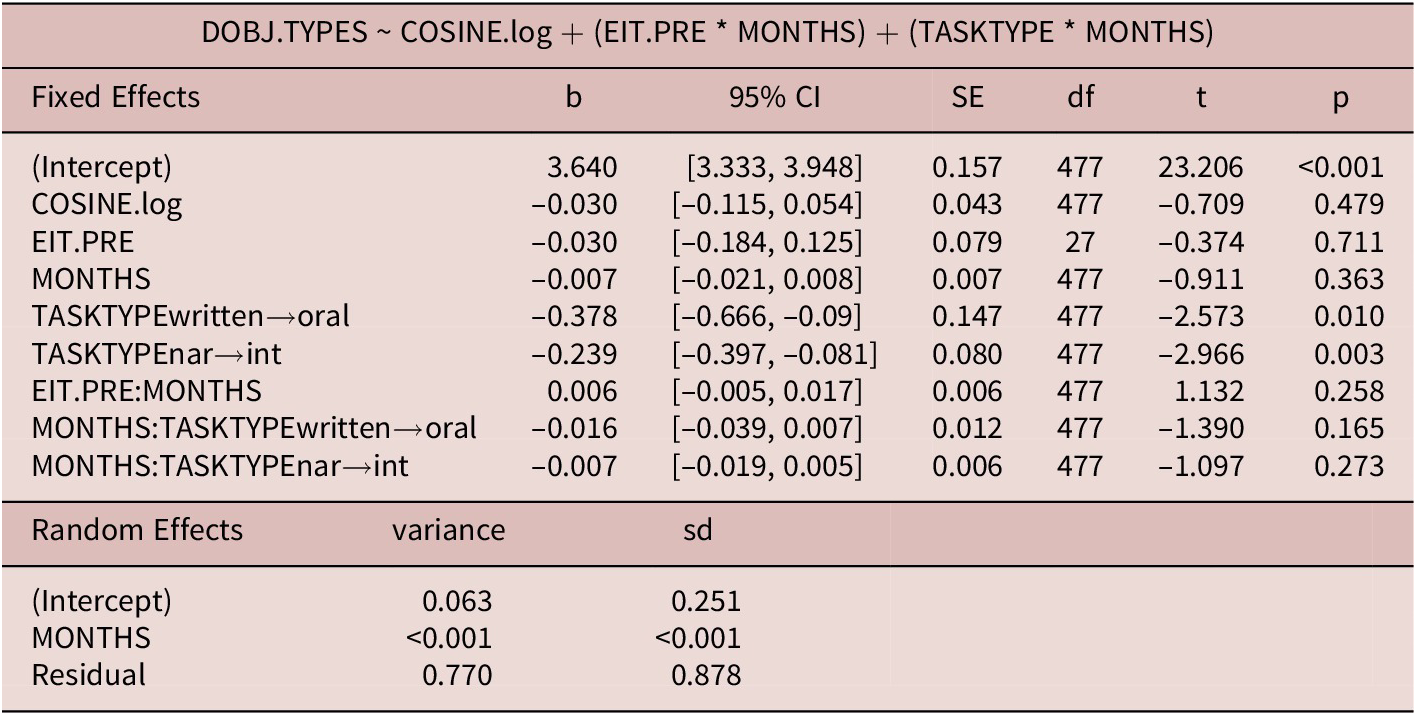
Marginal R2: 0.201
Conditional R2: 0.262
As shown in Table 9, significant predictors of direct object sophistication (PMI) included task type (narrative vs. interview), time in months, and cosine similarity. The average PMI of direct objects in narratives was found to be higher by 0.12 as compared to interviews. In terms of developmental trends, the sophistication of direct objects increased by 0.01 per month. This developmental rate did not differ significantly across tasks (the interactions between task type and months were n.s.). Neither initial proficiency nor the interaction of initial proficiency and time in months was significant. There was, however, a significant effect of topic such that a one standard deviation increase in vocabulary similarity to the gay marriage and adoption essay was associated with decrease in direct object PMI of 0.06. This model explained 14.4% of the variance in direct object PMI.
Table 9. Direct object sophistication (learner data)
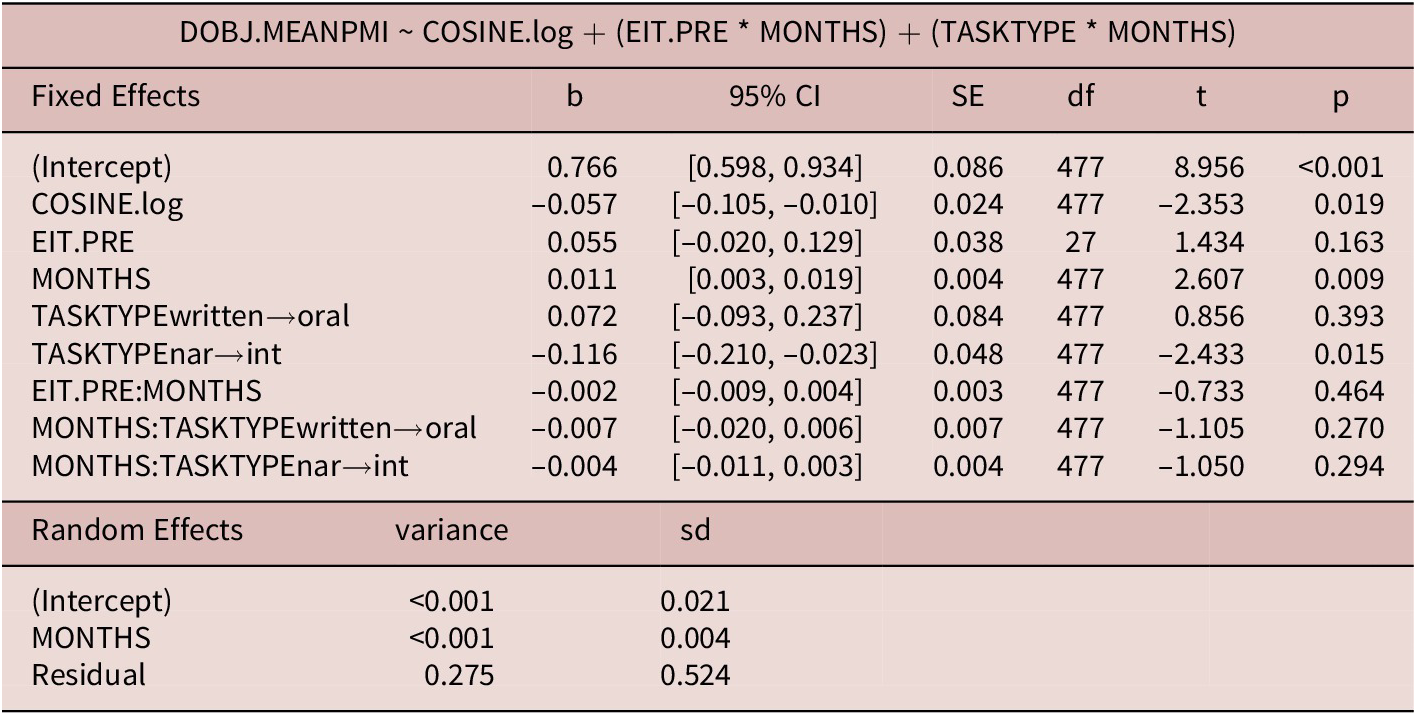
Marginal R2: 0.136
Conditional R2: 0.144
The L1 Models
We have limited the discussion here of the L1 models to the estimated marginal means and pairwise comparisons for each variable according to task type but the full model output and diagnostics are available in the supplementary materials. As shown in Table 10, adjectival modifier diversity (number of types) was significantly higher in the argumentative essays (Mean = 3.47, CI = 2.89, 4.04) as compared to the narratives (Mean = 1.12, CI = 0.78, 1.46) and the interviews (Mean = 1.44, CI = 1.16, 1.73) and adjectival modifier diversity between the two oral tasks was not significantly different. A different pattern was found for the three other phraseological complexity measures. The sophistication of adjectival modifiers (PMI) was significantly higher in both the essays (Mean = 2.15, CI = 1.80, 2.50) and the narratives (Mean = 1.93, CI = 1.54, 2.33) than in the interviews (Mean = 1.20, CI = 0.83, 1.57) and the difference between the essays and the narratives was not found to be significant. Similarly, direct object diversity (number of types) was found to be significantly higher in both the essays (Mean = 4.21, CI = 3.53, 4.89) and the narratives (Mean = 3.86, CI = 3.44, 4.29) than in the interviews (Mean = 2.98, CI = 2.76, 3.20), and the difference between the essays and the narratives was not found to be significant. Lastly, direct object sophistication (PMI) was significantly higher in the narratives (Mean = 1.55, CI = 1.39, 1.72) as compared to the interviews (Mean = 1.09, CI = 0.83, 1.35). The mean PMI of direct objects was also higher in the essays (Mean = 1.54, CI = 1.19, 1.90) as compared to the interviews but this difference was just beyond the threshold for significance at an alpha level of 5%. Again, the difference between the essays and the narratives was not significant. To summarize, written argumentative essays were found to contain a higher number of adjectival modifier types as compared to the oral tasks. However, for the three other measures, namely the number of direct object types as well as the mean PMI of both adjectival modifier and direct object relations, higher values were found in the written essay and oral narrative as compared to the oral interview task.
Table 10. Task differences for L1 Group (n = 10)

Discussion
RQ1. To what extent are there differences in phraseological complexity between oral and written tasks completed by the L1 group and the learner group?
In general, the written learner productions exhibited higher levels of phraseological complexity as compared to the oral productions. Specifically, the argumentative essays were found to use more adjectival modifier and direct object types and used adjectival modifiers with a higher PMI as compared to oral tasks. In the case of the L1 data, the only measure that was found to distinguish between the oral and written tasks was the number of adjectival modifier types. The fact that both L1 and learner written tasks had more adjectival modifier types as compared to oral tasks is likely related to the communicative function of the task. As shown in Example 1, the argumentative essays tended to be composed of long noun phrases, containing one or more adjectival modifiers. This is characteristic of texts with an “informational” communicative purpose (Biber, Reference Biber2014). In contrast, both oral tasks are more verbal in nature. This is unsurprising given that both the oral narrative and the oral interview require participants to relate a sequence of events: either events in a story in the case of the narrative task (Example 2) or events occurring in their own lives in the case of the interviews (Example 3). As a result, the most important information in the oral tasks is conveyed through the use of verb phrases rather than complex noun phrases. This pattern is also in line with the register-variation literature showing that narrative functions such as these are associated with a more clausal style (Biber, Reference Biber2014).
-
(1) bien que c’est possible qu’une telle composition familiale puisse constituer une entrave pour l’enfant dans son développement il faut absolument note que ceci n’est pas forcément une conséquence du type de famille en soi même c’est à dire de l’orientation sexuelle des parents. ‘although it’s possible that such a family composition can constitute an obstacle for the child in his development, it is necessary [to] note that this is not necessarily a consequence of the type of family itself, that is to say, the sexual orientation of the parents’ (G126d).
-
(2) et pendant ce temps là Jacques pensait à toutes les choses qu’ils faisaient ensemble. ils riraient ensembles. ils lisaient des histoires. et ils jouaient dans les arbres. ‘and during that time, Jacques thought of all of the things they did together. they laughed together. they read stories. and they played in the trees.’ (B100c)
-
(3) oui j’ai envoyé ma candidature à l’université. et j’ai choisi des cours que je veux faire. et. je vais continuer avec l’allemand la civilisation allemande. et j’espère aussi faire un cours qui s’agit de la langue alsacien. ‘yes, I sent in my application to the university. and I chose courses that I want to do. and. I am going to continue with German, German civilization. and I also hope to take a course about the Alsacian language.’ (0118a).
In addition to the more varied use of adjectival modifier types, written learner productions also used adjectival modifiers with a higher PMI as compared to the oral productions. The most frequent adjectival modifiers in the written data include units such as couple_NOM hétérosexuel_ADJ (‘heterosexual couple’; PMI = 6.57, n = 23) and nourriture_NOM sain_ADJ (“healthy food”; PMI = 4.49, n = 17), which are directly related to the essay topics. That may also explain why we found a slight topic effect for adjectival modifiers. Texts that contained vocabulary that was more similar to that of the gay marriage and adoption essay were found to contain slightly more adjectival modifier types (0.07) for every one standard deviation increase in COSINE.log. In other words, it appears that the argumentative essays promoted adjectival modifiers in general and certain topics required the use of specialized vocabulary, which promoted the use of a more diverse set of adjectival modifiers related to the topic. In the oral data, however, the most frequent adjectival modifiers tended to be more general and have a lower PMI: temps_NOM même_ADJ (‘same time’; PMI = 3.12, n = 68), chose_NOM autre_ADJ (‘other thing’; PMI = 2.31, n = 57). This contrasts somewhat with the native data, which showed that the mean PMI of adjectival modifiers was relatively similar between the essay and the narrative and that the major difference was between both of those tasks and the interview. If access to appropriate, topic-specific adjectival modifier relations is somewhat more proceduralized for the native speakers, it may explain why the extra planning afforded by the written mode did not have a significant impact on the use of high PMI units by the native speakers but did have an impact for the learners in that it allowed learners to devote more attentional resources to selecting adjectival modifier relations (Ellis et al., Reference Ellis, Skehan, Li, Shintani, Lambert, Ellis, Skehan, Li, Shintani and Lambert2019; Skehan, Reference Skehan1998). To that extent, these results are in line with previous research showing higher levels of both lexical diversity and sophistication (use of infrequent lexical items) in written tasks as compared to oral tasks in L2 French (Bulté & Housen, Reference Bulté and Housen2009; Granfeldt, Reference Granfeldt, van Daele, Housen, Kuiken, Pierrard and Vedder2007).
The reduced opportunity for online planning afforded by the oral tasks may also explain why those tasks exhibited less diversity of direct objects as compared to the written task. The pressure of online production seems to have favored the use and repetition of more frequent, “safe” direct objects containing high frequency verbs such as faire (‘make/do’) and avoir (‘have’). In one interview for example, the direct object faire_VER devoir_NOM (‘do homework’) is repeated five times throughout. The phenomenon of learners sticking to a restricted set of highly frequent collocations, so-called lexico-grammatical teddy bears, has been previously attested in L2 written (Altenberg & Granger, Reference Altenberg and Granger2001; Nesselhauf, Reference Nesselhauf2005) and oral (Paquot et al., Reference Paquot, Gablasova, Brezina, Naets, Lénko-Szymańska and Götz2022) production. Because these highly frequent collocations often have a low PMI, this also has an effect on sophistication. While all three tasks exhibit direct objects with a negative PMI, indicating a lack of association (e.g., changer_VER problème_NOM; ‘change problem’; PMI = –1.31), the large proportion of the direct objects with a low PMI (1–5) in the oral tasks seems to have counterbalanced the effect of the direct objects with negative PMI in those tasks. In other words, direct object sophistication is not significantly different between the oral and written tasks not because the direct objects used in the oral tasks are particularly sophisticated but, rather, because in pressured oral production, learners used (and repeated) a smaller set of safer, low PMI direct objects. Of course, recycling vocabulary can be a very useful communicative strategy, which may allow learners to increase fluency (see Dabrowska, Reference Dabrowska2014), but such an increase in fluency often comes at the expense of complexity (Skehan, Reference Skehan2009b), which is what the measures presented here aim to capture.
Putting everything together, the results show that phraseological complexity did indeed differ between oral and written tasks. In the case of the learners, this seems to be a result of both the functional characteristics of the task (e.g., the use of specific adjectival modifiers in the argumentative essays) as well as the opportunities for online planning afforded by the written modality. For the L1 participants, whose production is likely more proceduralized than the learners, phraseological complexity seems to be determined more so by the functional requirements of a task than modality per se.
RQ2. To what extent does the development of phraseological complexity differ between oral and written tasks completed by learners of French?
The raw phraseological complexity measures for all texts are plotted in Figure 1. The red lines in each graph show the estimated linear trends over time (with 95% confidence intervals) as predicted by the model and the blue dashed line shows the estimated marginal mean for the L1 group (95% confidence intervals indicated by gray shading). As shown by the figure, the two dimensions of phraseological complexity, namely diversity and sophistication showed different patterns of development. For diversity, the only significant change was observed in the number of adjectival modifier types in the oral interviews, which decreased slightly over the study period. The fact that no significant increase was observed for phraseological diversity contrasts somewhat with the results of Qi and Ding (Reference Qi and Ding2011), the only longitudinal study to our knowledge that has measured the development of phraseological diversity, finding a significant increase over time in the diversity of manually identified “formulaic sequences” in oral monologues. However, that study was carried out over a longer period (four years) and involved a different type of phraseological unit so it is possible that the diversity of adjectival modifiers and direct objects simply develops too slowly to have been observed over the course of the 21-month period of the LANGSNAP project. That being said, given that the predicted phraseological diversity of the learners is generally within the same range as the L1 group, it may be that the learners had already mastered a level of complexity that was sufficient for these tasks. This may be why cross-sectional studies have also failed to show significant differences with respect to phraseological diversity between B2 and C2 level learners (Paquot, Reference Paquot2019) and between highly advanced learners and native speakers (Forsberg, Reference Forsberg2010). In other words, this suggests that there is an upper limit on how phraseologically diverse a task needs to be, after which point an increase in proficiency is no longer associated with an increase in phraseological diversity.
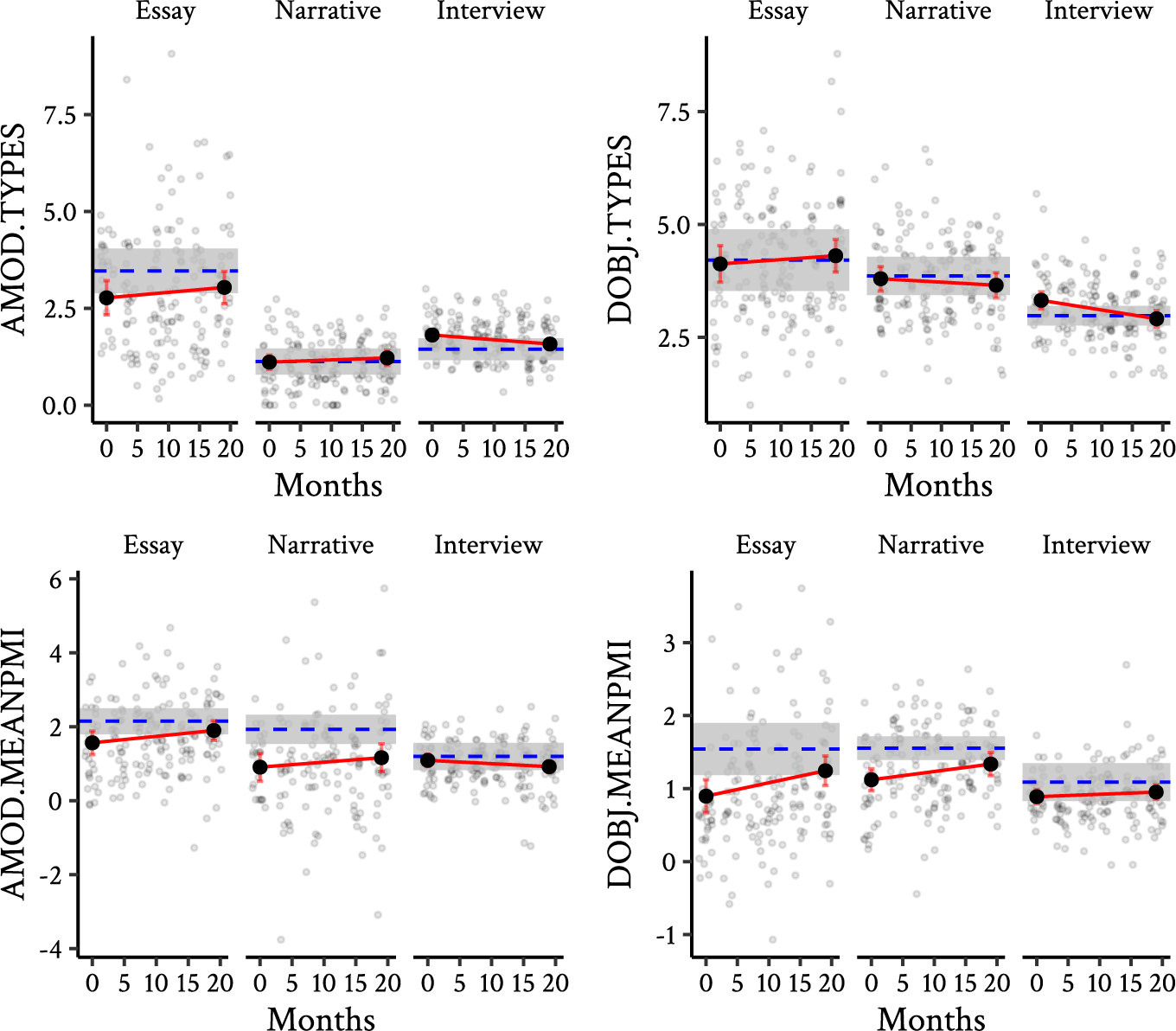
Figure 1. Predicted estimates and raw values of phraseological complexity measures over time (solid red line) compared to L1 benchmark (blue dashed line).
In contrast to the diversity measures, which exhibited no clear growth, the sophistication of both direct objects and adjectival modifiers increased slightly over the study period. In the case of both essays and narratives, the PMI of units at the beginning of the study period was below the lower confidence interval for the L1 benchmark and moved closer toward the native benchmark over time. However, although the direction of the trend was positive for adjectival modifiers, it did not quite reach statistical significance, in contrast to the results of Edmonds and Gudmestad (Reference Edmonds and Gudmestad2021), who found a significant increase in the PMI of adjective-noun collocations in the LANGSNAP argumentative essays between presojourn and the second postsojourn collection 19 months later. Although the Edmonds and Gudemestad study used the same data set, direct comparison of the results is made difficult due to several methodological differences including the use of a different extraction method, a different reference corpus and a different statistical modeling approach. That being said, one point of similarity between the two studies is that growth of phraseological sophistication is very gradual. Edmonds and Gudmestad (Reference Edmonds and Gudmestad2021, p. 11) report a monthly increase of 0.04,Footnote 13 in the PMI of adjectival modifiers, compared to 0.01 (n.s.) in this study. Even when growth was found to be significant in the current study, in the case of direct objects, the change in phraseological complexity only amounted to a monthly increase in PMI of 0.01. This may also explain why previous research has often failed to find a significant effect of time on association strength. Yoon (Reference Yoon2016), for example, reported no significant increase in the PMI of verb + noun collocations over the course of a semester in either narrative or argumentative written texts. Similarly, Kim, Crossley, and Kyle (Reference Kim, Crossley and Kyle2018) found no significant effect of time on the PMI of ngrams in learner interviews over the course of one year. These results suggest that phraseological complexity indeed develops very slowly, and that a large quantity of target language contact does not necessarily guarantee improvement in phraseological complexity (see Arvidsson, Reference Arvidsson2019).
Interestingly, although the difference in development between the three tasks was not significantly different for the PMI of direct objects, as can be seen in Figure 1, the estimated marginal trend for both the essay (0.02) and the narrative (0.01) are larger than that of the interview (0.003). In other words, direct object sophistication in both the essays and the narrative seems to have developed slightly faster than in the interviews (but not significantly so). One possible explanation may be that the interview topics simply did not elicit the same type of sophisticated vocabulary as the essay and narrative. Indeed, we did find that there was a small topic effect for the sophistication of direct objects so it could be the case that the open-ended nature of the interviews allowed learners to avoid topics for which they lacked vocabulary. Compared to the interview, the essay and the narrative tasks are both somewhat more constrained and this may have pushed the learners to produce more sophisticated phraseological units if they had acquired them in the intervening time between tasks. This was particularly evident in the case of one learner who struggled at presojourn to produce the word conte (“fairy tale”) but a year later was able to produce the direct object relation lire_VER conte_NOM (“read fairy tale”; PMI = 2.89) when completing the same narrative task. Likewise, the same learner replaced the direct object faire_VER exercise_NOM (“do exercise”; PMI = 1.00) with the more sophisticated chasser_VER papillon_NOM (“‘hunt butterfly”; PMI = 4.37), which describes the actions of the character in the story more specifically. Such gaps in vocabulary knowledge may not have been as evident in the interview task because there was less of a need to produce specific phraseological units and learners may have been able to recycle phraseological units used by the interlocutor or reuse units with which they are comfortable. Again, these may be very useful communication strategies in their own right but they have consequences for the ability to observe the development of phraseological complexity over time to the extent that they mask vocabulary gaps and artificially raise phraseological complexity at earlier time points (cf. Paquot et al., Reference Paquot, Gablasova, Brezina, Naets, Lénko-Szymańska and Götz2022).
To sum up, no significant increase was observed in terms of phraseological diversity over the 21-month period, which may have been because learners had already reached a native benchmark of phraseological diversity. Phraseological sophistication, however, did show a small amount of growth over the same period but this growth was only significant for direct objects. These results show that even in the context of intensive L1 input, phraseological complexity is slow to develop and that even when it does occur, it may not be equally evident in all task types or for all types of phraseological units.
Conclusion
While we first set out to compare phraseological complexity across modes, it quickly became apparent that a simple oral-written binary approach was too simplistic. While the tasks in the current study differ with respect to modality, they differ in other important ways as well. For one, the oral and written tasks involve different communicative functions (Biber, Reference Biber2014), which we showed seem to have an influence on the type of phraseological units that are elicited. Tasks also differ with respect to performance conditions such as the amount of planning time and interactivity (Skehan, Reference Skehan2009b), both of which have been shown to have an influence on the complexity of learner productions (Ellis et al., Reference Ellis, Skehan, Li, Shintani, Lambert, Ellis, Skehan, Li, Shintani and Lambert2019). The fact that phraseological complexity tended to be higher in learners’ written tasks as compared to oral tasks could be due to a number of these task-related factors. In this study, the design of the corpus only allows us to speculate on the causes of the differences that we observed between the tasks. That being said, the comparison to native benchmark data did help to shed light on possible explanations.
If access to phraseological units is somewhat more proceduralized for native speakers as compared to the learners (De Bot, Reference De Bot1992), this may explain why writing seems to have showcased the phraseological complexity of learners more so than native speakers. Comparing the learner levels of phraseological diversity to the L1 benchmark also showed that the learners performed quite similarly to their L1 counterparts in some respects, which suggests that there is a limit to how phraseologically diverse a production needs to be to fulfill the requirements of a task. Similarly, although the rate of development was not significantly different between tasks, there was a general trend that suggested that development may not be equally evident in all tasks. If a task does not require sophisticated language, such language is not likely to be elicited from learners. To our knowledge, this is the first study to directly compare the effect of task type on phraseological complexity and the results speak to the importance of considering task variables when measuring phraseological complexity in L2 production. They also speak to the usefulness of including L1 benchmark data (cf. Housen & Kuiken, Reference Housen and Kuiken2009).
It is important, however, to consider the limitations of this study when interpreting these results. For one, the assumption of linear growth over time may be criticized, given the dynamic nature of linguistic development (De Bot & Larsen-Freeman, Reference De Bot, Larsen-Freeman, Verspoor, de Bot and Lowie2011). However, the main aim of this research was to determine the extent to which the longitudinal development of phraseological complexity differed across oral and written production tasks, so we feel that such an abstraction is merited. Although individual learners may have shown variability from any one time point to the next, the results here show that there was an overall increase in direct object PMI over time and this linear pattern did not show a large degree of variation between learners (evidenced by the small standard deviation in by-participant slopes for time in months). Moreover, approaches that fully capitalize on variability in L2 development, such as the dynamic systems approach (ibid.), require many more data collection points than the six that are available in the LANGSNAP data.
Another limitation is that to include both random slopes and random intercepts in the model, the individual prompts for the essays and narratives needed to be collapsed together. While this is not ideal, given that topic has been shown to have a strong effect on phraseological complexity (Paquot et al., Reference Paquot, Naets, Gries, Le Bruyn and Paquot2021), we believe that the generalizability gained by including random slopes and random intercepts outweighs this downside. In an attempt to control for topic, we removed the phraseological units that were directly provided in the prompts or the picture stories and we included cosine similarity in the model. This method allowed us to quantify text differences in a more fine-grained way than is possible by using a simple categorical variable for the different prompts. That being said, it is still a rather crude measure of textual similarity. Future studies would do well to investigate more sophisticated methods of controlling for topic differences (e.g., topic modeling as proposed by Murakami et al., Reference Murakami, Thompson, Hunston and Vajn2017).
The scope of this study is also limited in that we have chosen to focus on only two types of phraseological units (as realized in the form of adjectival modifiers and direct objects). Given that this was one of the first studies to investigate phraseological complexity in L2 French, we felt that looking at these units was a good starting point, especially in light of previous phraseological complexity research in L2 English (e.g., Paquot, Reference Paquot2018, Reference Paquot2019). Of course, it would be disingenuous to claim that these two units alone represent the full extent of the phraseological complexity apparent in a given text and more work is clearly needed to increase the coverage of phraseological complexity measures, especially considering that adjectival modifiers and direct objects tended to relatively infrequent in the learner texts overall. In oral narratives, for example, the median number of adjectival modifiers was only 1.18 per 100 words (IQR = 0.92). This raises the question of how many phraseological units are needed to get a reliable picture of a text’s phraseological complexity. After all, if a text uses just one adjectival modifier with a high PMI, is that really enough to claim that it exhibits a high level of phraseological sophistication overall? Probably not. In a follow-up study to the present one, we have begun to address this very issue by broadening the scope of phraseological units under investigation beyond two-word collocations (Vandeweerd et al., Reference Vandeweerd, Housen and Paquotunder review).
Another challenge for phraseological complexity is how to operationalize the dimension of diversity. As discussed in the methodology section, measures that were originally designed for lexical items tend to be either strongly correlated with text-length overall (e.g., RTTR) or cannot be used because they require many more units than are attested in a typical text (e.g., MTLD). Using a moving-average method based on 100-word windows solved some of these problems but may have also introduced others (e.g., conflating low quantity and low diversity, biasing units at the beginning rather than the end of texts). Moving forward, it will be necessary to develop more innovative solutions to account for the unique challenges of phraseology rather than simply borrowing measures from other linguistic domains.
More broadly, because phraseological complexity is still a relatively new construct, there is also an urgent need to empirically demonstrate the validity of these measures more systematically. This could be done, for example, by grounding these measures in human judgements not just of proficiency but also of phraseological complexity (as suggested by Paquot, Reference Paquot2021). After all, just because a given complexity measure is correlated with proficiency or increases over time does not mean that it necessarily represents the construct it is intended to measure (see Pallotti, Reference Pallotti2015, Reference Pallotti, Winke and Brunfaut2021).
Despite these limitations, the results here do speak to the usefulness of phraseological complexity measures as indices of development in L2 French. Importantly, they also show that various factors besides development appear to influence the amount of phraseological complexity observed in a given text. These factors are similar to those discussed by Skehan (Reference Skehan, Richards, Daller, Malvern, Meara, Milton and Treffers-Daller2009a) in relation to lexical complexity, namely: performance conditions (e.g., modality), style, and other task influences (e.g., interactivity). At this point, we have only just begun to scratch the surface in our understanding of how such factors contribute to measures of phraseological complexity and this will be an important area to explore further in the future.
Acknowledgments
We would like to thank Héloïse Copin for her invaluable help with the manual annotation. We are also grateful to the LANGSNAP team at the University of Southampton who have kindly made their data publicly available for research purposes. Funding for this project was provided by the Fonds de la Recherche Scientifique—FNRS (Grant n° T.0086.18). The present research also benefited from computational resources made available on the Tier-1 supercomputer of the Fédération Wallonie-Bruxelles, infrastructure funded by the Walloon Region under the grant agreement n°1117545.
Data Availability Statement
The experiment in this article earned an Open Materials badge for transparent practices. The materials are available at: https://osf.io/bkfru/
Appendix
Definitions of phraseological units
-
• Adjectival modifiers (AMOD) were defined as adjectives that modify a noun. This also includes superlatives (e.g., la drogue la plus forte). Following Abeillé and Clément (Reference Abeillé and Clément2003), past participles in the attribute position are considered adjectives (e.g., enfants adoptés) except if they are followed by an agentive complement (e.g., les enfants adoptés par Jean et Luc). Present participles are considered adjectives if they agree with the noun they modify and they do not have a direct object (e.g., les erreurs existants). Quantifiers (e.g., certaines) and ordinal numbers (e.g., première) are not considered adjectives, nor are nouns that are modified by another (proper) noun (e.g., étudiants Erasmus). Compound words (e.g., fast food) are not considered adjectival modifiers.
-
• Direct objects (DOBJ) were defined as nouns that are the object of verbs. This does not include nouns modified by a relative clause (e.g., les impôts qu’on paie) or by a passive clause (les distributeurs ont été supprimés) but it does include objects of nonfinite verbs (e.g., concernant le mariage). The object of il y a is considered a direct object relation (e.g., il y a une bonne idée).