INTRODUCTION
Widespread interest in fishers’ knowledge (FK) for fisheries science and management (Haggan et al. Reference Haggan, Neis and Baird2007) derives from two main perspectives. Firstly, FK is thought to contain useful information to improve the performance of fisheries management (Jentoft et al. Reference Jentoft, McCay and Wilson1998; Haggan & Neis Reference Haggan, Neis, Haggan, Neis and Baird2007). FK may encompass a finer spatial resolution and be more up to date than formal scientific knowledge (Rochet et al. Reference Rochet, Prigent, Bertrand, Carpentier, Coppin, Delpech, Fontenelle, Foucher, Mahé, Rostiaux and Trenkel2008). FK often also provides a longer historical perspective than other data sources (Dulvy & Polunin Reference Dulvy and Polunin2004; Ames Reference Ames, Haggan, Neis and Baird2007; Lavides et al. Reference Lavides, Polunin, Stead, Tabaranza, Comeros and Dongallo2009), especially in tropical reef fisheries (Johannes Reference Johannes1998) and has broader scope. For example FK may include information on ecological, social, technical and economic aspects of fisheries that have historically been neglected by conventional fisheries science (Moller et al. Reference Moller, Berkes, Lyver and Kislalioglu2004). Secondly, the process of co-management (Jentoft et al. Reference Jentoft, McCay and Wilson1998) relies on the development of institutions that facilitate knowledge exchange between stakeholders, scientists and managers to develop greater understanding and more efficient governance (Hoefnagel et al. Reference Hoefnagel, Burnett, Wilson, Motos and Wilson2006).
Fishers and scientists commonly have diverging perceptions of resources and may be suspicious of the reliability of one another's perceptions (Hall-Arber Reference Hall-Arber2003; Gray et al. Reference Gray, Hatchard, Daw and Stead2008), creating conflicts and barriers for integrating knowledge sources in multi-stakeholder arenas. Such ‘cognitive conflicts’ may be the main challenge in managing common pool natural resources (Adams et al. Reference Adams, Brockington, Dyson and Vira2003), while shared understandings can support collective action to sustain resources (Ostrom et al. Reference Ostrom, Burger, Field, Norgaard and Policansky1999). However, investigating discrepancies between scientific and fishers’ perceptions may also provide opportunities for expansion and revision of scientific knowledge (Johannes & Neis Reference Johannes, Neis, Haggan, Neis and Baird2007).
Many possible explanations exist for disagreement between scientists’ and fishers’ perceptions. Disputes often focus on the validity of either perception and the question of which is correct. Scientists may be sceptical when fishers dispute the severity of stock declines (termed the ‘you-would-say-that’ issue by Daw Reference Daw2008). Indeed, political conflicts or vested interests can incentivize fishers to bias their stated views either consciously or unconsciously (Harmon-Jones & Harmon-Jones Reference Harmon-Jones and Harmon-Jones2007). However, numerous examples exist in which fishers’ disputes of scientific findings have been shown to be based on more than strategic self interest (Haggan & Neis Reference Haggan, Neis, Haggan, Neis and Baird2007).
Disputes can arise simply because they are based on observations of different parts of the fisheries system. FK has been shown to vary according to the social context of fishers (Crona & Bodin Reference Crona and Bodin2006) or by the types of gear used (Gerhardinger et al. Reference Gerhardinger, Marenzi, Bertoncini, Medeiros and Hostim-Silva2006). Fishers and scientists may perceive the system at different scales (Berkes Reference Berkes2006), or through monitoring different variables (Verweij et al Reference Verweij, van Densen and Mol2010). Inaccuracies or biases can also affect both scientific and fishers’ perceptions, due to the context in which perceptions are formed.
Human perceptions are affected by psychological recall processes (Tversky & Kahneman Reference Tversky and Kahnema1973), existing beliefs and behaviours (Balcetis & Dunning Reference Balcetis and Dunning2007) and frameworks of understanding (Miller Reference Miller2000; Fazey et al. Reference Fazey, Fazey, Salisbury, Lindenmayer and Dovers2006). Perception of trends over time requires recall, and comparison of current and former conditions, while taking account of variation to distinguish long-term trends from short-term noise (van Densen Reference van Densen2001). The act of recalling past conditions is accomplished by a variety of cognitive heuristics (Tversky & Kahneman Reference Tversky and Kahneman1974). For example, the ‘availability heuristic’ is used when estimating the frequency of an event based on the ease with which it is recalled. As such, more available memories, which may be pleasant, unusual or emotive for the individual (Matlin Reference Matlin2004), will appear to be more frequent and have a greater influence on the perception of past conditions. The ‘shifting baseline syndrome’ has also been reported to affect fishers’ perceptions of deteriorating environmental conditions (Saenz-Arroyo et al. Reference Saenz-Arroyo, Roberts, Torre, Carino-Olvera and Enriquez-Andrade2005; Bunce et al. Reference Bunce, Rodwell, Gibb and Mee2007) and can operate at a societal level, as a result of younger generations being unaware of past abundance (termed generational amnesia), or as a result of individuals forgetting previous abundances (termed personal amnesia) (Papworth et al. Reference Papworth, Rist, Coad and Milner-Gulland2009).
Holm (Reference Holm2003) characterized the process of researching and recording FK as the construction of a decontextualized knowledge, which he referred to as FEK*. This useful distinction between in situ FK, and FEK* as the product of FK research, highlights inaccuracies or biases which may exist as artefacts of the process of generating FEK*. Recording FK can be affected by political or vested interests of individuals, fisheries management regimes, and methodological aspects of the research (Maurstad Reference Maurstad, Neis and Felt2000; Davis & Wagner Reference Davis and Wagner2003).
Scientific data are also subject to potential inaccuracies due to poor resolution, biases and incorrect assumptions, and fisheries science is well known to be subject to a range of uncertainties (Charles Reference Charles1998), and even the political and social context in which it is generated (Finlayson Reference Finlayson1994). Fisheries-dependent data collection can be biased by misreporting, poor sampling design and effort (over emphasis on certain landing sites or gears), and changes in gear use and targeting behaviour by fishers in response to various socioeconomic drivers (de Mutsert et al. Reference de Mutsert, Cowan, Essington and Hilborn2008). In many fisheries, effort is not monitored, or the resolution is low, while subtle changes in efficiency are difficult to monitor. For example, fishers can introduce or improve fishing technology (such as global positioning systems or fish-finders), change the targeting of fish or increase distance travelled, and trap soak times or effort exerted during fishing (Jennings et al. Reference Jennings, Kaiser and Reynolds2001). Such changes are almost impossible to record and standardize (Hilborn & Walters Reference Hilborn and Walters1992). Interviews with fishers have the advantage of being able to investigate such changes (see for example Neis et al. Reference Neis, Schneider, Felt, Haedrich, Fischer and Hutchings1999; Quirijns et al. Reference Quirijns, Poos and Rijnsdorp2008; Eigaard Reference Eigaard2009). In multispecies tropical fisheries, data collection is often aggregated at high taxonomic levels and large spatial scales. Underwater visual census (UVC) offers detailed fisheries-independent data, but biases in UVC may arise from spatial extent of sampling, habitat structure and fish behaviour (Edgar et al. Reference Edgar, Barrett and Morton2004), and the method can normally only sample a limited proportion of the available habitat.
Moving beyond the ‘who-is-right’ mode of understanding disputes between FK and science becomes possible with the appreciation that all types of knowledge are partial and affected by the context in which they are created (Murray et al. Reference Murray, Neis, Palmer and Schneider2008). Disputes may provide opportunities to expand the scope of knowledge available for resource management and revise scientific understandings, which may be based on too narrow a conception of the system (Hoffmann-Riem & Wynne Reference Hoffmann-Riem and Wynne2002).
This paper examines how perceptions of resource abundance in the Seychelles artisanal trap fishery differ according to fisher interviews and two common forms of scientific fisheries data, namely UVC by scientific divers and landings surveys conducted by fieldworkers. We compare the perceptions of catch, catch-per-unit-effort (CPUE), variance in catches and abundance trends over a 10-year period.
METHODS
Study sites
Artisanal trap fisheries of the inner Seychelles islands provide a rare opportunity among tropical artisanal fisheries of a long (10+ year) time span of catch and effort data, and UVC data on fish density and biomass.
The fishery is conducted from small boats with outboard engines of 15–40 hp, and uses three types of traditional bamboo traps (kasye). Kasye peze and kasye dormi (fixed traps) are both sturdily constructed and left for a soak time of up to three days. Kasye peze are unbaited and wedged amongst corals on the shallow reef flats, while kasye dormi are set outside the reef crest in depths of up to 60m and may be baited (Daw Reference Daw2008). Kasye lavol (active traps) have a lighter construction, are baited, and are placed in a variety of depths for several hours. Siganids, lethrinids and scarids are the most important families caught in traps but kasye dormi also catch substantial quantities of mullids and lutjanids. Octopus, labrids, acanthurids, serranids, haemulids, balistids, muraenids and pomacanthids are also fished, while chaetodontids and scorpaenids are frequently caught but discarded. Kasye lavol are used to target known spawning aggregations of Siganus sutor, in which case they catch this species almost exclusively (Robinson et al. Reference Robinson, Isidore, Marguerite, Öhman and Payet2004).
Trap-caught fish are used for local consumption and are typically sold by fishers themselves, in ‘packets’, of several fish tied together. The price of packets tends to be constant, but their weight and species composition can vary according to catches.
Three areas of Seychelles were chosen for this study to correspond with existing UVC data collection sites (Jennings et al. Reference Jennings, Grandcourt and Polunin1995; Fig. 1). We focused our analysis on the dominant trap type and season in each area to maximize the relationship between landings and UVC data (Table 1).
Table 1 The area and trap type distinctions used to define the three fisheries selected for this study, indicating the total number of fishers identified, interviewed and who refused to be interviewed. East Mahé landings records are from June to September only. Valid area/gear refers to fishers using selected gears within the area of the UVC surveys and landings data. Valid trends refers to fishers with ≥ 8 years of continuous gear use. Ten year trend figures from south-west Praslin include records from Grand Anse only.

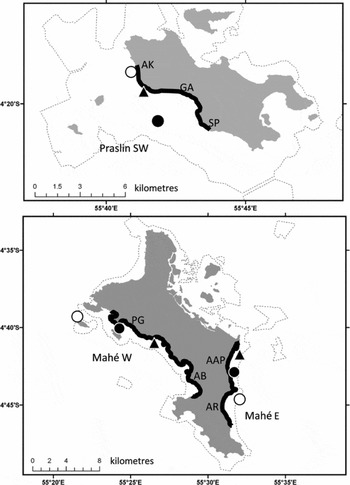
Figure 1 Seychelles study locations. Dark lines indicate the three areas of coastline where interviews were conducted. Shapes indicate underwater visual census sites in three different habitats (filled circles = coral, open circles = granite, triangles = patch reefs). Areas for the catch assessment survey are identified by abbreviations (AAP = Anse Aux Pins, AB = Anse Boileau, AR = Anse Royale, AK = Anse Kerlan, GA = Grande Anse, PG = Port Glaud, SP = South Praslin).
Data collection
Perceptions of fishers
Individual structured interviews were conducted during September–November 2005, focused on the dominant trap type used in each area (Table 1). In each area, a list of trap fishers was compiled from landing site visits and by speaking to fishers, local residents and Seychelles Fishing Authority (SFA) staff. All fishers that could be contacted were approached for interview. Individual trap fishers were asked about their typical catch and effort on a ‘good’, ‘poor’ and ‘normal’ day, their perception of trends over the past ten years (or since the start of their career if less than ten years), their typical catch and effort ten years previously, and several indicators of changes in effective effort (such as length of vessel, engine power, days fished per week, maximum depth fished, maximum distance travelled from landing site to fishing ground and trap soak time) currently and ten years previously (or since the start of their career if less than ten years). These formed part of an interview which also included understandings of fish stock dynamics and opinions on fisheries management (questionnaire adapted from Walmsley et al Reference Walmsley, Howard and Medley2005; Appendix, see supplementary material at Journals.cambridge.org/ENC). Catches were generally reported in packets, while effort was described by the number of trap hauls per day. Mean catch per unit effort (CPUE) was calculated by dividing the day's catch by the number of trap hauls. To ensure standardized data within each area and maximum overlap with the UVC data, fishers were only included in the analysis if they answered questions on the dominant trap type in each region and spent all of their fishing time within the regions (Fig. 1).
Catch assessment survey data
Landings data were extracted from the SFA artisanal fisheries catch assessment survey (CAS), a stratified catch and effort monitoring system that has been in place since 1985. Fieldworker effort is randomly distributed between 63 landing sites on Mahé, Praslin and La Digue, within site strata that are defined according to the number and type of boats active at the sites. Fieldworkers record fishing activities (number and types of vessels fishing, number of trips, and number and type of gears used) and landings are estimated from counts of the number of fish or packets, sample weights and estimated percentage taxonomic composition of catches (Mees Reference Mees1990).
The CAS distinguishes active traps (kasye lavol) from fixed traps, but not between the two types of fixed traps (kasye peze and skayse dormi). Interviews with trap fishers indicated that kasye dormi were predominantly used in west Mahé and that there is typically a seasonal pattern in the use of fixed traps in east Mahé, with kasye dormi being used in the calmer north-west monsoon and at least 75% of fishers exclusively using kasye peze inside the reef during the rougher south-east monsoon. Thus, to maximize the overlap between interview data on kasye peze, landings from fixed traps during the south-east monsoon (June–September) were selected. Records for fixed traps and active traps from west Mahé and south-west Praslin were taken from all months, assuming that they represented kasye dormi and kasye lavol, respectively. The few landings records from vessels without outboard engines were excluded, as all interviewees used outboard engines. Mean CPUE was calculated by dividing daily catch by the gear number. Some data (0.5–10% depending on area) were excluded, as the number of gears had apparently been entered erroneously as one, inflating estimates of CPUE.
Underwater visual census
Fisheries-independent indications of trends in fish biomass were obtained from UVC conducted in 1994 and 2005. Three sites, representing carbonate reef, granitic reef and patch reef habitats, were surveyed within each of the three areas (Fig. 1). At each site, 16 replicate 7-m radius point-count surveys of reef fishes were conducted. The abundance and size of 134 species of diurnally-active reef-associated fish were recorded at each count (Jennings et al. Reference Jennings, Grandcourt and Polunin1995; Graham et al. Reference Graham, Wilson, Jennings, Polunin, Bijoux and Robinson2006). Length calibration was achieved by estimating the lengths of a random assortment of lengths of PVC pipe before each day's sampling, until the observer was within an error range of 1cm; mean errors for 1994 and 2005 were 3.1% and 2.2%, respectively (Graham et al. Reference Graham, Wilson, Jennings, Polunin, Bijoux and Daw2007). Abundance-length data were converted to biomass using published length-weight relationships (Letourneur et al. Reference Letourneur, Kulbicki and Labrosse1998; Froese & Pauly Reference Froese and Pauly2006).
Data analysis
Catches as reported by fishers
Most fishers reported catches in numbers of packets. To allow comparison with landings data, packet numbers were converted to kg based on a sample of 239 original SFA fieldworker datasheets detailing weights of individual packets from July to December 1996 and from July 2004 until June 2005. Packets were significantly larger in 2004 (p < 0.001). Accordingly, estimates of current and past (ten years previous) catches from fisher interviews were converted by multiplying number of packets by 3.63 kg and 2.94 kg, respectively.
Contemporary catch and CPUE
For an indication of ‘current’ catches, a subset of landings data was taken for a three-year period prior to the interviews in order to provide enough data (n = 75–150), to indicate the frequency distribution and central tendency of catches in each area. To capture variability in catches, records representing the aggregate catch of more than one boat (c.10% of records) were removed. Frequency distributions of catch and CPUE were plotted for each area. Owing to the positively skewed nature of the landings data and the existence of outliers, median rather than mean values were chosen to compare with fishers’ perceptions of a ‘normal’ day's catch and CPUE.
For each interviewed fisher, the difference between median landings records, and their reported normal catch and catch per trap (dCatch and dCPUE), was calculated as a proportion of median landings values:
Proportional catch difference (dCatchfa) = (NCfa – Ma)/Ma
Where NCf is a normal day's catch in fishery a according to fisher f, and Ma is the median catch from landings data in fishery a.
For each fishery, the distribution of dCatch and dCPUE was tested for significant differences from zero (Wilcoxon signed ranks non-parametric test) to indicate whether reports of normal catches by fishers differed from median catches according to CAS data.
Time trends as perceived by catch assessment survey
For analysis of trends in landings data, records from between January 1995 and July 2005 were selected from the CAS database. Average catch per day and average catch per trap (CPUE) were analysed for trends. Visual assessment of data showed different trends between the three main landing sites in the south-west Praslin area, so only data from Grande Anse (where the majority of interviews were conducted) were included.
The presence of linear or non-linear trends in each time series was assessed by comparing generalized additive models (GAMs) fitted to each dataset using the gam function from the mgcv package in R. GAMs allow the visualization of non-linear relationships between dependent and multiple explanatory variables (Zuur et al. Reference Zuur, Ieno and Smith2007). Seasonality in catches was accounted for by the inclusion of a month smoother term in all models except those for the east Mahé kasye peze fishery, which only included the four months of the north-east monsoon. Three alternative models were fitted to the data from each area representing (1) no trend over time (month smoother term only), (2) a linear trend over time (month smoother and year linear terms) and (3) a non-linear trend over time (month and year smoother terms).
The degree to which each model fitted the data was assessed using Akaike's Information Criterion (AIC). Models explaining the greatest proportion of variance with fewest parameters were indicated by the lowest AIC (Burnham & Anderson Reference Burnham and Anderson2002). We calculated ΔAIC as the difference between each model and the best of the three models. Residual plots and graphical outputs of the GAM smoother term were examined. We did not account for autocorrelation between years, possibly leading to over estimation of the degree of fit, particularly for GAMs.
Where AIC scores indicated a linear trend with year (where the linear trend was favoured over the no-trend model, the modelled change was presented as annual change as a per cent of interpolated year 2000 values (change year−1 yr2000−1). We plotted graphical representations of the year smoother from the non-linear trend and obtained an indication of recent trends by presenting the slope of the GAM between the last two years as a percentage of the ten-year mean.
Time trends as perceived by underwater visual census
Total UVC-measured fish biomass, and biomass of target species (Table S1 in Appendix, see supplementary material at Journals.cambridge.org/ENC) that were large enough (> 6 cm body depth) to be caught by the inshore trap fishery (Graham et al. Reference Graham, Wilson, Jennings, Polunin, Bijoux and Daw2007), were analysed for differences between 1995 and 2005. Linear models with year, site (see Fig. 1) and site × year interaction were fitted for each area, to square-root-transformed data to reduce the influence of outliers. Models were selected for each area by AIC-based stepwise removal of terms (Zuur et al. Reference Zuur, Ieno and Smith2007) and the difference between years was indicated by the significance of the year term. If site × year interactions were retained in the model selection (suggesting a different trend at each site), trends were reported for each site. Where variances were unequal between years, Welch's t-tests, which do not assume equal variance, were used to test for a difference between years. All detected differences were converted to change year−1 yr2000−1.
Time trends as perceived by fishers
Qualitative indicators of fishers’ perceptions of trends were taken as the proportions of fishers who perceived a decline, no change or an increase at each site. Quantitative indicators of trends were also calculated for each fisher as the difference between reported contemporary catches and catches ten years previously (or at the start of their trap fishing activities if they had fished for less than 10 years). Only data from fishers with eight or more years of experience were used for quantitative trends. Six different quantitative trend indices were calculated as a result of using two different reports of current catches (‘normal’ and ‘good’ catches) and three different units to indicate catch, namely total daily catch, daily catch per trap in kg and daily catch per trap in the units used by interviewees (usually packets). Each of these trends were presented as change year−1 yr2000−1.
Indicators of effective effort
Fishers who had been fishing for at least five years were included in the analysis of indicators of effort efficiency. We calculated the proportion of interviewees who had increased, decreased or not changed with regards to each indicator and the percentage change from initial levels for each fisher. Changes for the sample were calculated in terms of mean percentage change and mean absolute change (such as miles).
RESULTS
Perceptions of current catches from interviews and landings
Landings data from all three fisheries showed a typical positively-skewed distribution of catches with extreme values at the higher end of the range (Fig. 2). The proportional difference between a fisher's normal catch and the median catch for that fishery (dCatch) was significantly greater than zero for all three fisheries (Table 2) indicating that most fishers’ reports of a ‘normal’ catch (converted to kg) were greater than the median of landings. Fishers reports tended to have greater extremes than landings; five of the 30 interviewees reported ‘normal’ catches that exceeded the 95% quantiles of landings data. ‘Poor’ catches were frequently lower than the 5% quantile of landings, and ‘good’ catches higher than the 95% quantile, particularly in east Mahé. Overall, one-third of reported ‘poor’ catches involved nothing being caught, but only two of the 362 landings records involved no catch, both from Praslin.

Figure 2 Frequency distributions of daily catches and catch per unit effort from three different Seychelles trap fisheries from landings data (upper panels), and individual reports of catch and CPUE from fisher interviews (lower panels, circles = ‘normal’ day, triangles = ‘poor’ day, squares = ‘good’ day) lower panels show a ‘strip chart’ in which each fisher is represented by a different y axis value. Thick vertical lines indicate median, and dotted vertical lines indicate the 5% and 95% quantiles of landings data.
Table 2 Results of Wilcoxon signed rank tests (with estimated median values) of dCatch and dCPUE (differences between ‘normal’ catches and CPUE reported by fishers and median landings).

When converted to CPUE, fishers’ reports showed more agreement with landings data. Reports of ‘normal’ CPUE in east Mahé and south-west Praslin straddled the median landing (Fig. 2) and dCPUE did not differ significantly from zero (Table 2). dCPUE in west Mahé was lower than dCatch but still generally positive and differed significantly from zero at 10% level. The 95% quantile of CPUE tended to be straddled by reports of ‘good’ CPUE (Fig. 2).
Extreme reported values were apparent in each fishery, especially for catch. Half of the ‘good’ catch reports in east Mahé lay outside the range of the landings data, while one ‘normal’ report in west Mahé was similar to maximum recorded landings. However, expressing reported data as CPUE tended to reduce the occurrence and extent of extreme values. For example, catch reports included three ‘normal’ and 11 ‘good’ values that were larger than the maximum recorded landing, whereas for CPUE, only one ‘normal’ and two ‘good’ reports extended beyond maximum landings. Two reports of ‘good’ catches from south-west Praslin were two and three times greater than the maximum recorded landings, but were comparable to typical landings when presented as CPUE as they came from three hauls from a large number (ten) of traps.
Time trends according to catch assessment survey
Model selection indicated different trends in the three fisheries and, in the case of east Mahé, between catch and CPUE (Table 3). Highly significant year terms for west Mahé catch and CPUE and east Mahé CPUE (but not catch) were also reflected in lower AICc values for linear as compared with null models. All linear trends were positive, and equivalent to 2–15% change yr−1 yr2000−1. South-west Praslin showed no evidence of any long-term trend in CPUE, but a trend in catch was indicated by a marginally significant year term in the linear model, which was selected by AIC over the null model.
Table 3 Selection of candidate models for catch and CPUE of each fishery and indicators of trends where present. *The lowest (i.e. best) AIC between the linear models. Smooth terms are plotted with partial residuals (shaded areas correspond to two standard errors above and below the estimate of the smooth).

The GAMs were selected by AIC in all cases. Graphical representations of the GAM smoother terms gave an indication of the underlying trend in catches by year when seasonal trends were accounted for by the month term in the models (Table 3). All sites showed some evidence of declines over the last two years, ranging from 9% of mean values in west Mahé CPUE to 37% in east Mahé CPUE.
Time trends according to fishers’ perceptions
Most fishers (82%, n = 28) in all areas perceived that catches had declined (Table 4), but quantitative indices of trends gave different conclusions depending on which index was used (Fig. 3). Most fishers cited ‘normal’ catches that were less than previous catches (representing declines of up to 15% yr−1 yr2000−1), but roughly half of fishers’ ‘good’ catches were greater than previous catches. In terms of the different indices, daily catch suggested less of a decline than catch per trap, and catch per trap converted to kg indicated less severe declines than catch per trap in the fishers’ own units, owing to the different packet conversion for current and former times. ‘Good’ versus previous catch in kg (Fig. 3, top right), indicated no decline on average, while ‘normal’ versus former catch per trap in the fishers’ own units (Fig. 3, lower left) suggested the most pessimistic picture, in which only 1 fisher (from east Mahé) perceived an increase in catches and 13 perceived a decrease of up to 17% yr−1 yr2000−1.
Table 4 Perceptions of trends from UVC and fisher interviews in each fishery. Proportional changes are calculated as annual change as a percentage of the modelled value for year 2000. Shaded bars indicate responses based on 8–10 years experience, open bars indicate other time spans (nine with 3–7 years and one with 30 years due to leaving and returning to fishing).


Figure 3 Quantitative indicators of changes in catch from trap fisher interviews in three trap fisheries, calculated by comparing past catches with either ‘normal’ (left panel) or ‘good’ (right panel) reported catches; and calculated in three different catch units (daily catch converted to kg, CPUE converted to kg trap−1 and CPUE in original reported units trap−1). Asterisks indicate mean values. Vertical lines indicate zero change. Circles = east Mahé kasye peze, triangles = west Mahé kasye dormi, crosses = south-west Praslin kasye lavol.
Time trends according to UVC data
UVC data from 1994 and 2005 for east Mahé indicated declines in total fish biomass but not in biomass of trap fish (Welch t = 2.05, p = 0.04; Table 4). There was a significant site × year interaction at west Mahé, so the three sites were tested separately. Total biomass was significantly less in 2005 at the patch reef site (Welch t = 4.87, p < 0.001), but not at the other sites, while trap fish biomass was significantly lower at all sites (F = 4.98, p = 0.028), and much lower in 2005 at the patch reef site (Welch t = 3.60, p = 0.001). No significant trends were detected in south-west Praslin.
Comparisons of trend perceptions in the datasets
Qualitatively, 10-year trend indications from the three sources of information were very different. Fisher interviews indicated declines in all areas, UVC from some sites indicated declines and landings data showed increases over the ten years. Landings did, however, indicate a decline in catches over the final one to three years of the time series. When comparing between areas, west Mahé landings data showed the strongest evidence for an increase, the least severe decline according to fisher interviews, but the most evidence for a decline according to UVC. Meanwhile, south-west Praslin showed no evidence for a decline according to UVC, but had the most pessimistic quantitative indications from fishers.
Trends in fishing behaviour
For most behavioural indicators, the majority of fishers did not report changes, but more fishers reported increases in effort than decreases (Fig. 4). The only exception was ‘days fished per week’ which had decreased for 14% (n = 29) of fishers and increased for only 3%. Vessel fishing power had commonly increased, with 45% (n = 22) of fishers having upgraded to larger engines (nine fishers), or obtained engines for the first time (two fishers), and 41% of fishers fishing in larger vessels. Thirty-one per cent and 44% (n = 16) of the fishers had increased the number of traps fished and soak time, respectively, with an average increase of c. 25% in each. A minority of fishers had increased the depth or distance at which they fished, with an average extension of 1.4 nmi in their range.

Figure 4 Changes in quantitative indicators of fishing effective effort by trap fishing interviewees during the previous 5–10 years. Mean changes are reported firstly in absolute terms and then as a percentage. Bars indicate the proportion of the interviewees whose indicators had increased, remained constant or decreased. Days per week refers to days spent fishing. Soak time refers to time between setting and lifting traps, max depth refers to depth of water in which traps were set, max range refers to the maximum distance travelled to fishing grounds. *Percentage changes in distance and engine power are not displayed owing to low or zero initial values.
DISCUSSION
In this study we compared perceptions of artisanal trap catches and CPUE, as obtained from fisher interviews and structured landings surveys, and perceptions of trends over ten years according to interviews, landings surveys and UVC. Landings data indicated lower catch per day, but similar catch per trap to fishers’ stated ‘poor’, ‘normal’ and ‘good’ catches, both in terms of the central tendency of the data (median CPUE and ‘normal’ catch per trap), and the range of the data indicated by the frequency distributions and the fishers’ reports of ‘poor’ and ‘good’ catches. Indications of trends over 10 years however, differed widely, with fisher interviews indicating declines, landings data indicating no trends or increases, and UVC indicating no trends or a decline depending on the site and fraction of the fish community sampled.
Contemporary catch indications from landings and fisher interviews
The observed congruence between contemporary CPUE according to landings and catch per trap according to interviews is encouraging for situations in which time and resources are not available to initiate a structured landings recording programme. For Seychelles trap fisheries, it seems that if effort is carefully accounted for, a reasonable account of both the magnitude and the variability of contemporary catch per trap can be obtained from interviews with fishers (for example see Lunn & Dearden Reference Lunn and Dearden2006). However, similar studies over a larger range of fisheries are needed to investigate the generality of this finding.
Our findings emphasize the importance of detailed measurement of fishing effort. Catch per day gave a different and less compatible result than the more detailed catch per trap, because of variations in the quantity of traps used (2–14) and hauls made per day (1–3) by fishers. Extreme reports of high daily catches may have created suspicion of exaggeration by interviewees, but when the data were presented in terms of catch per trap, individuals’ answers fell comfortably within the range of the rest of the sample.
Answers to interview questions can be affected by how interviewees interpret the context and wording of questions (Schwarz Reference Schwarz1999). For example, a ‘normal’ catch may have been cited based on a range of catch experiences, which may include or exclude zero catches, or be focused on particular seasons or areas. Different cognitive heuristics may have been used to construct an answer, such as stating the first trip that is recalled from memory, calculating the desired catch to cover costs and make an acceptable profit, or attempting some form of averaging over the range of a number of recalled trips. To encourage respondents to use a similar anchoring-and-adjustment heuristic (Tversky & Kahneman Reference Tversky and Kahneman1974), and to remove some variability owing to fishers placing more or less emphasis on particularly good catches, we asked about ‘good’, ‘poor’ and then ‘normal’ catches. While not eliminating problems of question interpretation, this provided a more standardized measurement of the perception of current catches, and also provided some indication of catch variability. Variability is in itself of interest, as it affects fishers’ ability to perceive spatial or temporal trends in catches (Oostenbrugge et al. Reference Oostenbrugge, Densen, and Machiels2001; Pet-Soede et al. Reference Pet-Soede, Van Densen, Hiddink, Kuyl and Machiels2001; van Densen Reference van Densen2001), has implications for livelihoods and vulnerability, and has been shown to rise in response to increasing fishing pressure (McClanahan et al. Reference McClanahan, Hicks and Darling2008), potentially indicating loss of fish stock resilience (Hsieh et al. Reference Hsieh, Reiss, Hunter, Beddington, May and Sugihara2006). More generally, variance indicators have been proposed as predictors of ecological regime-shifts (Carpenter & Brock Reference Carpenter and Brock2006).
Perception of trends from UVC and fisher interviews
Trends perceived from fishers’ and UVC data were not in accord, which can be attributed to many factors affecting the two data sources, and the limited overlap between UVC and the fishery in terms of depths, habitats and species. Kasye peze fishers tend to fish shallower than the reef slopes targeted by UVC, while kasye dormi fishers can expand beyond the shallow fringing reefs targeted by UVC onto the expansive Mahé plateau. Graham et al. (Reference Graham, Wilson, Jennings, Polunin, Bijoux and Daw2007) estimated that only 50–60% of trap fishing grounds overlapped with habitats and depths sampled by these UVC data.
Filtering of the UVC data for ‘trap fish’ changed indications from ‘decline’ to ‘no detectable decline’ in east Mahé, and vice versa in west Mahé. Trends in west Mahé were different at the different sites, with severe declines indicated on the patch reef habitat. This illustrates the complexity of comparing independent indicators of fish biomass over a complex heterogeneous seascape and the potentially critical impact of choices of how to select and interpret scientific data for the trends perceived in a resource. If the patch-reef site was assumed to be representative of areas targeted by trap fishers, the perceptions of declines reported by fishers would be expected.
Perception of trends from landings data and fisher interviews
The importance of effort is again highlighted by different trends indicated by catch and CPUE from landings (Table 3). There was an increase in some individuals’ effective effort through increasing vessel power and soak times (Fig. 4). This may account for discrepancy between landings and fishers’ perceptions. Fishers may have perceived resource declines, while compensating for them by increasing effective effort. Such declines cannot be perceived from landings without detailed information on changes in fishing behaviour/technology and the spatial distribution of effort. Effective effort can also be reduced by a range of factors, including management regulations, fuel prices or, at a personal level, due to ageing, or deterioration of gear or vessels.
Although several fishers reported poor catches of zero, CAS data had few zero catches. This may be because fishers with zero catches returned directly to mooring/anchorage sites instead of the monitored landing sites, fishers avoided interviews with fieldworkers on zero-catch days, or fieldworkers preferentially sampled and interviewed fishers with landings over those without. For fisheries in which zero catches are common, the failure to record them may introduce biases in mean or raised estimates from landings data.
Few studies of the implications of cognitive processes of memory and recall currently exist in FK literature, but such processes may affect perceptions of resource trends. For example, the time window of ten years may have contributed to the differing perceptions of CPUE trends obtained from fisher interviews and CAS data. All six of the GAM smoother terms showed a decline in recent years (although caution should be taken with the interpretation of the ends of GAM smoothers; A. Zurr, personal communication 2006). Fishers answering questions about a ten-year time trend may have based their answer on recent trends that were easier to recall, even if they were questioned about trends over 10 years.
As with the majority of artisanal fisheries in the world (Berkes et al. Reference Berkes, Mahon, McConney, Pollnac and Pomeroy2001), catches from the Seychelles trap fishery are sold for profit. Changes in the gross revenue or profits from fishing may therefore be of greater importance to the fisher, and thus better remembered, than changes in quantity of catch (Matlin Reference Matlin2004). Increasing costs and/or decreasing fish prices could result in perceptions of a decline as profitability fell. In Seychelles, fishers’ profitability may have been negatively affected by macroeconomic difficulties that worsened over the study period, making imported equipment increasingly expensive, or by decreasing unit fish prices (as indicated by the increasing trend in packet size identified over the ten years). More generally, fluctuations in fuel prices, global market prices and government subsidies for fishers may affect perceptions of trends in resource abundance.
The availability heuristic (Tversky & Kahneman Reference Tversky and Kahneman1974) predicts that particularly large or unusual catches would dominate the perception of former catches. We compared ‘normal’ catches against reported former catches (assuming them also to be normal; Fig. 3, left). If these reported former catches do, in fact, refer to memorably good catches, then fishers would perceive more severe declines creating a ‘memory illusion’, in which declining trends are exaggerated or incorrectly perceived (Papworth et al. Reference Papworth, Rist, Coad and Milner-Gulland2009). Memory illusion operates in the opposite direction to the ‘shifting-baseline syndrome’, in which severity of declines is underestimated (Saenz-Arroyo et al. Reference Saenz-Arroyo, Roberts, Torre, Carino-Olvera and Enriquez-Andrade2005; Bunce et al. Reference Bunce, Rodwell, Gibb and Mee2007). Such potential biases arising from recall remain a challenge for the use of memory-based perceptions of trends, in the absence of records or repeated surveys. Biases are expected to increase with the length of time over which interviewees are asked to remember. Further research is required to investigate the magnitude and impact of these biases on trend perception. Cognitive science can be used to understand the processes, but to specifically understand them in fisheries, empirical research is needed to compare memory-based verbal reports of catches with independent records of the same individual's actual catches, in different contexts.
In conducting FK research and creating FEK*, FK is filtered and adjusted through the decisions, methods and assumptions of the researchers, which may have significant impacts (for example see Fig. 3). Assuming that reports of former catches were representative of memorably ‘good’ catches, and thus comparing them with contemporary ‘good’ catches (Fig. 3, right), would imply lesser declines. Different inferences may also be generated by the method used to process catch estimates from fishers. In this study, we found trends obtained from figures converted to kg were apparently more negative than trends calculated using fishers’ own units, and CPUE trends were more negative than catch trends (Fig. 3) owing to increasing trap numbers, thus demonstrating the critical impact that the method selected for FK processing and analysis can have on deriving conclusions. Changes in the assumptions made during the interpretation of fishers’ reports, may qualitatively alter the nature of the perceived trend (O'Donnell et al. Reference O'Donnell, Pajaro and Vincent2010).
Conflicts between scientific, or bureaucratic, perspectives on resources and those of resource users are common in fisheries (Gray et al. Reference Gray, Hatchard, Daw and Stead2008). In this case, fishers had more pessimistic perceptions of catch trends than scientific data, but, in spite of this, fishers were not supportive of introducing effort or catch controls, or limiting entry to the fishery (Daw Reference Daw2008). Thus, it is important to note that perceptions and knowledge are not the sole factors determining personal environmental behaviour (Kollmuss & Agyeman Reference Kollmuss and Agyeman2002), and preferences for potential management measures are complicated by a range of political factors, values, personal interests and power relations.
CONCLUSIONS
All three data sources gave different perceptions of trends in the biomass of fish and catches over the study period. Fishers’ experience of the fishery may have resulted in more pessimistic perceptions because of (1) increasing numbers of traps and other increases in effective effort, (2) an emphasis on recent catch declines, (3) psychological biases in the recall of past catches or (4) the increasing weight of fish packets effectively reducing the price of fish. Meanwhile, landings data may have perceived positive trends as a consequence of (1) monitoring of catch by kg rather than packets, (2) a long 10-year perspective, without emphasis on recent trends, (3) failure to record zero catches and (4) an inability to account for increasing efficiency. In addition, the trends implied by each dataset can be qualitatively affected by changing underlying assumptions about the types of fish measured by UVC or the meaning of fishers’ reports of previous catches. This illustrates how inferences can be critically impacted by the choice of methods and assumptions used to analyse either FK or scientific data. We have documented changes in effective effort, different trends identified over different timescales, habitats or species, changes in fish price and the existence of zero catches. Cognitive processes affecting trend perception are potentially highly significant, but were not measured or documented in this study and require further research.
An increasingly prevalent perspective suggests the importance of combining multiple information sources for monitoring and learning about social-ecological systems like fisheries (Folke et al. Reference Folke, Colding, Berkes, Berkes, Colding and Folke2003). Fishers may be able to perceive trends rapidly and at small scales, which, owing to subtle increases in fishing effort or efficiency, or aggregation over large scales, are not detectable by landings data (Neis et al. Reference Neis, Schneider, Felt, Haedrich, Fischer and Hutchings1999; Rochet et al. Reference Rochet, Prigent, Bertrand, Carpentier, Coppin, Delpech, Fontenelle, Foucher, Mahé, Rostiaux and Trenkel2008). Meanwhile, landings data may better detect large-scale trends from background variability by integrating the catches of many different fishers (van Densen Reference van Densen2001). UVC may be able to monitor actual changes in biomass of fish regardless of variability and change in catchability and fisher behaviour, but may have poor coverage or limited temporal, spatial, depth or taxonomic overlap with exploited fish populations. Neither scientific nor fishers’ perceptions can be considered to provide the ‘true’ picture of the resource. Each is partial in terms of spatial or taxonomic coverage, or the variables considered, and contextually contingent in terms of the methodology and the socioeconomic environment in which they are created. Consideration of various perceptions increases the awareness of the contingent nature of each, enables conflicts to be identified and addressed, and helps to avoid overconfidence in one signal.
Overconfidence in signals from trawler CPUE contributed to the overfishing and collapse of the northern cod fishery (Finlayson Reference Finlayson1994; Neis Reference Neis, Candow and Corbin1997). Further research should involve fishers and scientists in examining the reasons why perceptions are divergent, in order to improve understandings of trends in the fishery and to investigate issues such as how changes in effective effort influence the different perspectives. Stock assessments supported by various types of fishers’ knowledge are needed to provide a reliable and accepted status of the resource. Such processes also help to address ‘cognitive conflicts’ and facilitate social learning, which is thought to be important for co-management (Armitage et al. Reference Armitage, Plummer, Berkes, Arthur, Charles, Davidson-Hunt, Diduck, Doubleday, Johnson, Marschke, McConney, Pinkerton and Wollenberg2009).
In the case of the Seychelles artisanal trap fishery, new initiatives have recently been instigated to establish fisher organizations and co-management, which may provide a medium for such collaborative learning. Our research suggests that participatory research and monitoring to address diverging perceptions and understandings will be a key process supporting this initiative. In terms of specific recommendations, the scales of monitoring in co-management should be aligned with those over which fishers perceive the resource, and the participatory development of indicator systems may provide a more intuitive and acceptable scientific approach to monitoring and setting management targets.
ACKNOWLEDGEMENTS
We gratefully acknowledge the cooperation and time of Seychellois trap fishers, especially Peter Tirant and Hetson Oreddy. Vincent Savy, Gregory Berke, Maria Cedras and Christine Henriette assisted with fieldwork. Simon Jennings collected the 1994 UVC data. Selina Stead, Tim Gray, Tim McClanahan and Tony Hawkins provided advice and comments. The manuscript was substantially improved by comments from Nicholas Polunin, Ioan Fazey and two anonymous referees. Maps were provided by Calvin Gerry from Seychelles Fishing Authority. Funding was provided by the Western Indian Ocean Marine Science Association MASMA program, the Leverhulme Trust, a NERC/ESRC Ph.D. studentship (T. M. Daw) and a Fisheries Society of the British Isles Ph.D. studentship (N. A. J. Graham).